You are viewing a plain text version of this content. The canonical link for it is here.
Posted to commits@mxnet.apache.org by GitBox <gi...@apache.org> on 2022/02/11 14:33:41 UTC
[GitHub] [incubator-mxnet] bgawrych commented on a change in pull request #20856: [website] Add CPU quantization tutorial
bgawrych commented on a change in pull request #20856:
URL: https://github.com/apache/incubator-mxnet/pull/20856#discussion_r804705218
##########
File path: docs/python_docs/python/tutorials/performance/backend/dnnl/dnnl_quantization.md
##########
@@ -0,0 +1,304 @@
+<!--- Licensed to the Apache Software Foundation (ASF) under one -->
+<!--- or more contributor license agreements. See the NOTICE file -->
+<!--- distributed with this work for additional information -->
+<!--- regarding copyright ownership. The ASF licenses this file -->
+<!--- to you under the Apache License, Version 2.0 (the -->
+<!--- "License"); you may not use this file except in compliance -->
+<!--- with the License. You may obtain a copy of the License at -->
+
+<!--- http://www.apache.org/licenses/LICENSE-2.0 -->
+
+<!--- Unless required by applicable law or agreed to in writing, -->
+<!--- software distributed under the License is distributed on an -->
+<!--- "AS IS" BASIS, WITHOUT WARRANTIES OR CONDITIONS OF ANY -->
+<!--- KIND, either express or implied. See the License for the -->
+<!--- specific language governing permissions and limitations -->
+<!--- under the License. -->
+
+## Introduction
+
+After successful model building and achieving desired accuracy on the test data, often the next step is to optimize inference to deploy the model to production. One of the key features of usable model is to have as small latency as possible to be able to provide services to large number of customers simultaneously. In addition to customer satisfaction, with well optimized model, hardware load is reduced which also reduces energy costs needed to perform inference.
+
+Two main types of software optimizations can be characerized as:
+- memory-bound optimizations - main objective of these optimizations is to reduce the amount of memory operations (reads and writes) - it is done by e.g. chaining operations which can be performed one after another immediately, where input of every subsequent operation is the output of the previous one (example: ReLU activation after convolution),
+- compute-bound optimizations - these optimizations are mainly made on operations which require large number of CPU cycles to complete, like FullyConnected and Convolution. One of the methods to speedup compute-bound operations is to lower computation precision - this type of optimization is called quantization.
+
+In version 2.0 of the Apache MXNet (incubating) GluonAPI2.0 replaced Symbolic API known from versions 1.x, thus there are some differences between API to perform graph fusion and quantization.
+
+## Operator Fusion
+
+Models are often represented as a directed graph of operations (represented by nodes) and data flow (represented as edges). This way of visualizing helps a lot when searching for common patterns in whole model which can be optimized by fusion. Example:
+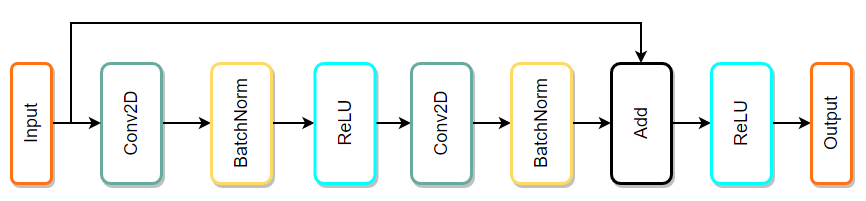
+
+
+The simplest way to explain what fusion is and how it works is to present an example. Image above depicts a sequence of popular operations taken from ResNet architecture. This type of architecture is built with many similar blocks called residual blocks. Some possible fusion patterns are:
+
+- Conv2D + BatchNorm => Fusing BatchNorm with Convolution can be performed by modifing weights and bias of Convolution - this way BatchNorm is completely contained within Convolution which makes BatchNorm zero time operation. Only cost of fusing is time needed to prepare weights and bias in Convolution based on BatchNorm parameters.
+- Conv2D + ReLU => this type of fusion is very popular also with other layers (e.g. FullyConnected + Activation). It is very simple idea where before writing data to output, activation is performed on that data. Main benefit of this fusion is that, there is no need to read and write back data in other layer only to perform simple activation function.
+- Conv2D + Add => even simpler idea than the previous ones - instead of overwriting output memory, results are added to the output memory. In the simplest terms: `out_mem = conv_result` is replaced by `out_mem += conv_result`.
+
+Above examples are presented as atomic ones, but often they can be combined together, thus two patterns that can be fused in above example are:
+- Conv2D + BatchNorm + ReLU
+- Conv2D + BatchNorm + Add + ReLU
+
+After fusing all patterns, computational graph will be changed to the following one:
+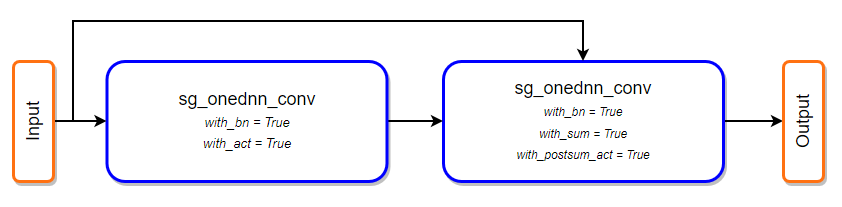
+
+
+
+### Operator fusion in MXNet
+Since the version 1.6 of MXNet built with oneDNN support, operator fusion had been enabled by default for executing model with Module API, however in version 2.0 it has been decided to remove setting this feature by environment flag and replace it by aware user API call.
+
+To fuse model in MXNet 2.0 there are two requirements:
+- the model must be defined as a subclass of HybridBlock or Symbol,
+- the model must have specific operator patterns which can be fused.
+
+As an example we define example network (sample block from ResNet architecture):
+
+```
+import mxnet as mx
+from mxnet.gluon import nn
+
+class SampleBlock(nn.HybridBlock):
+ def __init__(self):
+ super(SampleBlock, self).__init__()
+ self.conv1 = nn.Conv2D(channels=64, kernel_size=3, strides=1, padding=1,
+ use_bias=False, in_channels=64)
+ self.bn1 = nn.BatchNorm()
+ self.conv2 = nn.Conv2D(channels=64, kernel_size=3, strides=1, padding=1,
+ use_bias=False, in_channels=64)
+ self.bn2 = nn.BatchNorm()
+
+ def forward(self, x):
+ out = mx.npx.activation(self.bn1(self.conv1(x)), 'relu')
+ out = self.bn2(self.conv2(out))
+ out = mx.npx.activation(out + x, 'relu')
+ return out
+
+net = SampleBlock()
+net.initialize()
+
+data = mx.np.zeros(shape=(1,64,224,224))
+# run fusion
+net.optimize_for(data, backend='ONEDNN')
+
+# We can check fusion by plotting current symbol of our optimized network
+sym, _ = net.export(None)
+graph = mx.viz.plot_network(sym, save_format='jpg')
+graph.view()
+```
+Both HybridBlock and Symbol classes provide API to easily run fusion of operators. Single line of code is enabling fusion passes on model:
+```
+net.optimize_for(data, backend='ONEDNN')
+```
+
+*optimize_for* function is available also as Symbol class method. Example call to this API is shown below. Notice that Symbol’s *optimize_for* method is not done in-place, so assigning it to a new variable is required:
+
+```
+optimized_symbol = sym.optimize_for(backend='ONEDNN')
+```
+
+For the above model definition in a naive benchmark with artificial data, we can gain up to 1.75x speedup without any accuracy loss on our testing machine with Intel(R) Core(TM) i9-9940X.
+
+
+## Quantization
+As mentioned in the introduction, precision reduction is another very popular method of improving performance of workloads and, what is important, in most cases is combined together with operator fusion which improves performance even more. In training precision reduction utilizes 16 bit data types like bfloat or float16, but for inference great results can be achieved using int8.
Review comment:
```suggestion
## Quantization
As mentioned in the introduction, precision reduction is another very popular method of improving performance of workloads and, what is important, in most cases is combined together with operator fusion which improves performance even more. In training precision reduction utilizes 16 bit data types like bfloat or float16, but for inference great results can be achieved using int8.
```
--
This is an automated message from the Apache Git Service.
To respond to the message, please log on to GitHub and use the
URL above to go to the specific comment.
To unsubscribe, e-mail: commits-unsubscribe@mxnet.apache.org
For queries about this service, please contact Infrastructure at:
users@infra.apache.org