You are viewing a plain text version of this content. The canonical link for it is here.
Posted to commits@seatunnel.apache.org by ki...@apache.org on 2022/02/19 05:46:39 UTC
[incubator-seatunnel-website] branch main updated: [Feature]The blog module supports Chinese and English (#65)
This is an automated email from the ASF dual-hosted git repository.
kirs pushed a commit to branch main
in repository https://gitbox.apache.org/repos/asf/incubator-seatunnel-website.git
The following commit(s) were added to refs/heads/main by this push:
new 99fcd56 [Feature]The blog module supports Chinese and English (#65)
99fcd56 is described below
commit 99fcd5613f5a4fd62d1871a8098ebea29b5871bf
Author: Kerwin <37...@users.noreply.github.com>
AuthorDate: Sat Feb 19 13:46:34 2022 +0800
[Feature]The blog module supports Chinese and English (#65)
* The blog module supports Chinese and English
* update display chinese in the blog module
---
README.md | 1 +
README_ZH.md | 1 +
blog/2021-12-30-hdfs-to-clickhouse.md | 74 +++++------
blog/2021-12-30-hive-to-clickhouse.md | 50 +++----
blog/2021-12-30-spark-execute-elasticsearch.md | 90 ++++++-------
blog/2021-12-30-spark-execute-tidb.md | 93 +++++++------
blog/2021-12-30-spark-structured-streaming.md | 145 ++++++++++-----------
blog/2022-2-18-Meetup-vip.md | 112 ++++++++--------
.../2021-12-30-hdfs-to-clickhouse.md | 0
.../2021-12-30-hive-to-clickhouse.md | 2 +-
.../2021-12-30-spark-execute-elasticsearch.md | 0
.../2021-12-30-spark-execute-tidb.md | 0
.../2021-12-30-spark-structured-streaming.md | 6 +-
.../2022-2-18-Meetup-vip.md | 0
14 files changed, 288 insertions(+), 286 deletions(-)
diff --git a/README.md b/README.md
index 0db9dee..974c182 100644
--- a/README.md
+++ b/README.md
@@ -37,6 +37,7 @@ This website is compiled using node, using Docusaurus framework components
|-- i18n
| `-- zh-CN //Internationalized Chinese
| |-- code.json
+| |-- docusaurus-plugin-content-blog
| |-- docusaurus-plugin-content-docs
| |-- docusaurus-plugin-content-docs-community
| |-- docusaurus-plugin-content-docs-download
diff --git a/README_ZH.md b/README_ZH.md
index 2b75fda..5aaa814 100644
--- a/README_ZH.md
+++ b/README_ZH.md
@@ -37,6 +37,7 @@ asf-staging 官网测试环境 通过https://seatunnel.staged.apache.org 访问
|-- i18n
| -- zh-CN //国际化中文
| |-- code.json
+| |-- docusaurus-plugin-content-blog
| |-- docusaurus-plugin-content-docs
| |-- docusaurus-plugin-content-docs-community
| |-- docusaurus-plugin-content-docs-download
diff --git a/blog/2021-12-30-hdfs-to-clickhouse.md b/blog/2021-12-30-hdfs-to-clickhouse.md
index e11f74b..62d588b 100644
--- a/blog/2021-12-30-hdfs-to-clickhouse.md
+++ b/blog/2021-12-30-hdfs-to-clickhouse.md
@@ -1,24 +1,24 @@
---
slug: hdfs-to-clickhouse
-title: 如何快速地把 HDFS 中的数据导入 ClickHouse
+title: How to quickly import data from HDFS into ClickHouse
tags: [HDFS, ClickHouse]
---
-# 如何快速地把 HDFS 中的数据导入 ClickHouse
+# How to quickly import data from HDFS into ClickHouse
-ClickHouse 是面向 OLAP 的分布式列式 DBMS。我们部门目前已经把所有数据分析相关的日志数据存储至 ClickHouse 这个优秀的数据仓库之中,当前日数据量达到了 300 亿。
+ClickHouse is a distributed columnar DBMS for OLAP. Our department has now stored all log data related to data analysis in ClickHouse, an excellent data warehouse, and the current daily data volume has reached 30 billion.
-之前介绍的有关数据处理入库的经验都是基于实时数据流,数据存储在 Kafka 中,我们使用 Java 或者 Golang 将数据从 Kafka 中读取、解析、清洗之后写入 ClickHouse 中,这样可以实现数据的快速接入。然而在很多同学的使用场景中,数据都不是实时的,可能需要将 HDFS 或者是 Hive 中的数据导入 ClickHouse。有的同学通过编写 Spark 程序来实现数据的导入,那么是否有更简单、高效的方法呢。
+The experience of data processing and storage introduced earlier is based on real-time data streams. The data is stored in Kafka. We use Java or Golang to read, parse, and clean the data from Kafka and write it into ClickHouse, so that the data can be stored in ClickHouse. Quick access. However, in the usage scenarios of many students, the data is not real-time, and it may be necessary to import the data in HDFS or Hive into ClickHouse. Some students implement data import by writing Spar [...]
-目前开源社区上有一款工具 **Seatunnel**,项目地址 [https://github.com/apache/incubator-seatunnel](https://github.com/apache/incubator-seatunnel),可以快速地将 HDFS 中的数据导入 ClickHouse。
+At present, there is a tool **Seatunnel** in the open source community, the project address [https://github.com/apache/incubator-seatunnel](https://github.com/apache/incubator-seatunnel), can quickly Data in HDFS is imported into ClickHouse.
## HDFS To ClickHouse
-假设我们的日志存储在 HDFS 中,我们需要将日志进行解析并筛选出我们关心的字段,将对应的字段写入 ClickHouse 的表中。
+Assuming that our logs are stored in HDFS, we need to parse the logs and filter out the fields we care about, and write the corresponding fields into the ClickHouse table.
### Log Sample
-我们在 HDFS 中存储的日志格式如下, 是很常见的 Nginx 日志
+The log format we store in HDFS is as follows, which is a very common Nginx log
```shell
10.41.1.28 github.com 114.250.140.241 0.001s "127.0.0.1:80" [26/Oct/2018:03:09:32 +0800] "GET /Apache/Seatunnel HTTP/1.1" 200 0 "-" - "Dalvik/2.1.0 (Linux; U; Android 7.1.1; OPPO R11 Build/NMF26X)" "196" "-" "mainpage" "443" "-" "172.16.181.129"
@@ -26,7 +26,7 @@ ClickHouse 是面向 OLAP 的分布式列式 DBMS。我们部门目前已经把
### ClickHouse Schema
-我们的 ClickHouse 建表语句如下,我们的表按日进行分区
+Our ClickHouse table creation statement is as follows, our table is partitioned by day
```shell
CREATE TABLE cms.cms_msg
@@ -46,21 +46,21 @@ CREATE TABLE cms.cms_msg
## Seatunnel with ClickHouse
-接下来会给大家详细介绍,我们如何通过 Seatunnel 满足上述需求,将 HDFS 中的数据写入 ClickHouse 中。
+Next, I will introduce to you in detail how we can meet the above requirements through Seatunnel and write the data in HDFS into ClickHouse.
### Seatunnel
-[Seatunnel](https://github.com/apache/incubator-seatunnel) 是一个非常易用,高性能,能够应对海量数据的实时数据处理产品,它构建在Spark之上。Seatunnel 拥有着非常丰富的插件,支持从 Kafka、HDFS、Kudu 中读取数据,进行各种各样的数据处理,并将结果写入 ClickHouse、Elasticsearch 或者 Kafka 中。
+[Seatunnel](https://github.com/apache/incubator-seatunnel) is a very easy-to-use, high-performance, real-time data processing product that can deal with massive data. It is built on Spark. Seatunnel has a very rich set of plugins that support reading data from Kafka, HDFS, Kudu, performing various data processing, and writing the results to ClickHouse, Elasticsearch or Kafka.
### Prerequisites
-首先我们需要安装 Seatunnel,安装十分简单,无需配置系统环境变量
+First we need to install Seatunnel, the installation is very simple, no need to configure system environment variables
-1. 准备 Spark 环境
-2. 安装 Seatunnel
-3. 配置 Seatunnel
+1. Prepare the Spark environment
+2. Install Seatunnel
+3. Configure Seatunnel
-以下是简易步骤,具体安装可以参照 [Quick Start](/docs/quick-start)
+The following are simple steps, the specific installation can refer to [Quick Start](/docs/quick-start)
```shell
cd /usr/local
@@ -75,19 +75,19 @@ unzip seatunnel-1.1.1.zip
cd seatunnel-1.1.1
vim config/seatunnel-env.sh
-# 指定Spark安装路径
+# Specify the Spark installation path
SPARK_HOME=${SPARK_HOME:-/usr/local/spark-2.2.0-bin-hadoop2.7}
```
### seatunnel Pipeline
-我们仅需要编写一个 seatunnel Pipeline 的配置文件即可完成数据的导入。
+We only need to write a configuration file of seatunnel Pipeline to complete the data import.
-配置文件包括四个部分,分别是 Spark、Input、filter 和 Output。
+The configuration file consists of four parts, Spark, Input, filter and Output.
#### Spark
-这一部分是 Spark 的相关配置,主要配置 Spark 执行时所需的资源大小。
+This part is the related configuration of Spark, which mainly configures the size of the resources required for Spark to execute.
```shell
spark {
@@ -100,7 +100,7 @@ spark {
#### Input
-这一部分定义数据源,如下是从 HDFS 文件中读取 text 格式数据的配置案例。
+This part defines the data source. The following is a configuration example for reading data in text format from HDFS files.
```shell
input {
@@ -114,18 +114,18 @@ input {
#### Filter
-在 Filter 部分,这里我们配置一系列的转化,包括正则解析将日志进行拆分、时间转换将 HTTPDATE 转化为 ClickHouse 支持的日期格式、对 Number 类型的字段进行类型转换以及通过 SQL 进行字段筛减等
+In the Filter section, here we configure a series of transformations, including regular parsing to split the log, time transformation to convert HTTPDATE to the date format supported by ClickHouse, type conversion to Number type fields, and field filtering through SQL, etc.
```shell
filter {
- # 使用正则解析原始日志
+ # Parse raw logs using regular expressions
grok {
source_field = "raw_message"
pattern = '%{IP:ha_ip}\\s%{NOTSPACE:domain}\\s%{IP:remote_addr}\\s%{NUMBER:request_time}s\\s\"%{DATA:upstream_ip}\"\\s\\[%{HTTPDATE:timestamp}\\]\\s\"%{NOTSPACE:method}\\s%{DATA:url}\\s%{NOTSPACE:http_ver}\"\\s%{NUMBER:status}\\s%{NUMBER:body_bytes_send}\\s%{DATA:referer}\\s%{NOTSPACE:cookie_info}\\s\"%{DATA:user_agent}\"\\s%{DATA:uid}\\s%{DATA:session_id}\\s\"%{DATA:pool}\"\\s\"%{DATA:tag2}\"\\s%{DATA:tag3}\\s%{DATA:tag4}'
}
- # 将"dd/MMM/yyyy:HH:mm:ss Z"格式的数据转换为
- # "yyyy/MM/dd HH:mm:ss"格式的数据
+ # Convert data in "dd/MMM/yyyy:HH:mm:ss Z" format to
+ # Data in "yyyy/MM/dd HH:mm:ss" format
date {
source_field = "timestamp"
target_field = "datetime"
@@ -133,8 +133,8 @@ filter {
target_time_format = "yyyy/MM/dd HH:mm:ss"
}
- # 使用SQL筛选关注的字段,并对字段进行处理
- # 甚至可以通过过滤条件过滤掉不关心的数据
+ # Use SQL to filter the fields of interest and process the fields
+ # You can even filter out data you don't care about by filter conditions
sql {
table_name = "access"
sql = "select substring(date, 1, 10) as date, datetime, hostname, url, http_code, float(request_time), int(data_size), domain from access"
@@ -144,7 +144,7 @@ filter {
#### Output
-最后我们将处理好的结构化数据写入 ClickHouse
+Finally, we write the processed structured data to ClickHouse
```shell
output {
@@ -161,7 +161,7 @@ output {
### Running seatunnel
-我们将上述四部分配置组合成为我们的配置文件 `config/batch.conf`。
+We combine the above four-part configuration into our configuration file `config/batch.conf`.
```shell
vim config/batch.conf
@@ -184,14 +184,14 @@ input {
}
filter {
- # 使用正则解析原始日志
+ # Parse raw logs using regular expressions
grok {
source_field = "raw_message"
pattern = '%{IP:ha_ip}\\s%{NOTSPACE:domain}\\s%{IP:remote_addr}\\s%{NUMBER:request_time}s\\s\"%{DATA:upstream_ip}\"\\s\\[%{HTTPDATE:timestamp}\\]\\s\"%{NOTSPACE:method}\\s%{DATA:url}\\s%{NOTSPACE:http_ver}\"\\s%{NUMBER:status}\\s%{NUMBER:body_bytes_send}\\s%{DATA:referer}\\s%{NOTSPACE:cookie_info}\\s\"%{DATA:user_agent}\"\\s%{DATA:uid}\\s%{DATA:session_id}\\s\"%{DATA:pool}\"\\s\"%{DATA:tag2}\"\\s%{DATA:tag3}\\s%{DATA:tag4}'
}
- # 将"dd/MMM/yyyy:HH:mm:ss Z"格式的数据转换为
- # "yyyy/MM/dd HH:mm:ss"格式的数据
+ # Convert data in "dd/MMM/yyyy:HH:mm:ss Z" format to
+ # Data in "yyyy/MM/dd HH:mm:ss" format
date {
source_field = "timestamp"
target_field = "datetime"
@@ -199,8 +199,8 @@ filter {
target_time_format = "yyyy/MM/dd HH:mm:ss"
}
- # 使用SQL筛选关注的字段,并对字段进行处理
- # 甚至可以通过过滤条件过滤掉不关心的数据
+ # Use SQL to filter the fields of interest and process the fields
+ # You can even filter out data you don't care about by filter conditions
sql {
table_name = "access"
sql = "select substring(date, 1, 10) as date, datetime, hostname, url, http_code, float(request_time), int(data_size), domain from access"
@@ -219,7 +219,7 @@ output {
}
```
-执行命令,指定配置文件,运行 Seatunnel,即可将数据写入 ClickHouse。这里我们以本地模式为例。
+Execute the command, specify the configuration file, and run Seatunnel to write data to ClickHouse. Here we take the local mode as an example.
```shell
./bin/start-seatunnel.sh --config config/batch.conf -e client -m 'local[2]'
@@ -227,10 +227,10 @@ output {
## Conclusion
-在这篇文章中,我们介绍了如何使用 Seatunnel 将 HDFS 中的 Nginx 日志文件导入 ClickHouse 中。仅通过一个配置文件便可快速完成数据的导入,无需编写任何代码。除了支持 HDFS 数据源之外,Seatunnel 同样支持将数据从 Kafka 中实时读取处理写入 ClickHouse 中。我们的下一篇文章将会介绍,如何将 Hive 中的数据快速导入 ClickHouse 中。
+In this post, we covered how to import Nginx log files from HDFS into ClickHouse using Seatunnel. Data can be imported quickly with only one configuration file without writing any code. In addition to supporting HDFS data sources, Seatunnel also supports real-time reading and processing of data from Kafka to ClickHouse. Our next article will describe how to quickly import data from Hive into ClickHouse.
-当然,Seatunnel 不仅仅是 ClickHouse 数据写入的工具,在 Elasticsearch 以及 Kafka等 数据源的写入上同样可以扮演相当重要的角色。
+Of course, Seatunnel is not only a tool for ClickHouse data writing, but also plays a very important role in the writing of data sources such as Elasticsearch and Kafka.
-希望了解 Seatunnel 和 ClickHouse、Elasticsearch、Kafka 结合使用的更多功能和案例,可以直接进入官网 [https://seatunnel.apache.org/](https://seatunnel.apache.org/)
+If you want to know more functions and cases of Seatunnel combined with ClickHouse, Elasticsearch and Kafka, you can go directly to the official website [https://seatunnel.apache.org/](https://seatunnel.apache.org/)
-- Power by [InterestingLab](https://github.com/InterestingLab)
diff --git a/blog/2021-12-30-hive-to-clickhouse.md b/blog/2021-12-30-hive-to-clickhouse.md
index 2289991..402ec12 100644
--- a/blog/2021-12-30-hive-to-clickhouse.md
+++ b/blog/2021-12-30-hive-to-clickhouse.md
@@ -1,22 +1,22 @@
---
slug: hive-to-clickhouse
-title: 如何快速地把 Hive 中的数据导入 ClickHouse
+title: How to quickly import data from Hive into ClickHouse
tags: [Hive, ClickHouse]
---
-ClickHouse是面向OLAP的分布式列式DBMS。我们部门目前已经把所有数据分析相关的日志数据存储至ClickHouse这个优秀的数据仓库之中,当前日数据量达到了300亿。
+ClickHouse is a distributed columnar DBMS for OLAP. Our department has stored all log data related to data analysis in ClickHouse, an excellent data warehouse, and the current daily data volume has reached 30 billion.
-在之前的文章 [如何快速地把HDFS中的数据导入ClickHouse](2021-12-30-hdfs-to-clickhouse.md) 中我们提到过使用 Seatunnel [https://github.com/apache/incubator-seatunnel](https://github.com/apache/incubator-seatunnel) 对HDFS中的数据经过很简单的操作就可以将数据写入ClickHouse。HDFS中的数据一般是非结构化的数据,那么针对存储在Hive中的结构化数据,我们应该怎么操作呢?
+In the previous article [How to quickly import data from HDFS into ClickHouse] (2021-12-30-hdfs-to-clickhouse.md), we mentioned the use of Seatunnel [https://github.com/apache/incubator -seatunnel](https://github.com/apache/incubator-seatunnel) After a very simple operation on the data in HDFS, the data can be written to ClickHouse. The data in HDFS is generally unstructured data, so what should we do with the structured data stored in Hive?

## Hive to ClickHouse
-假定我们的数据已经存储在Hive中,我们需要读取Hive表中的数据并筛选出我们关心的字段,或者对字段进行转换,最后将对应的字段写入ClickHouse的表中。
+Assuming that our data has been stored in Hive, we need to read the data in the Hive table and filter out the fields we care about, or convert the fields, and finally write the corresponding fields into the ClickHouse table.
### Hive Schema
-我们在Hive中存储的数据表结构如下,存储的是很常见的Nginx日志
+The structure of the data table we store in Hive is as follows, which stores common Nginx logs.
```
CREATE TABLE `nginx_msg_detail`(
@@ -40,7 +40,7 @@ CREATE TABLE `nginx_msg_detail`(
### ClickHouse Schema
-我们的ClickHouse建表语句如下,我们的表按日进行分区
+Our ClickHouse table creation statement is as follows, our table is partitioned by day
```
CREATE TABLE cms.cms_msg
@@ -59,27 +59,28 @@ CREATE TABLE cms.cms_msg
## Seatunnel with ClickHouse
-接下来会给大家介绍,我们如何通过 Seatunnel 将Hive中的数据写入ClickHouse中。
+Next, I will introduce to you how we write data from Hive to ClickHouse through Seatunnel.
### Seatunnel
-[Seatunnel](https://github.com/apache/incubator-seatunnel) 是一个非常易用,高性能,能够应对海量数据的实时数据处理产品,它构建在Spark之上。Seatunnel 拥有着非常丰富的插件,支持从Kafka、HDFS、Kudu中读取数据,进行各种各样的数据处理,并将结果写入ClickHouse、Elasticsearch或者Kafka中。
+[Seatunnel](https://github.com/apache/incubator-seatunnel) is a very easy-to-use, high-performance, real-time data processing product that can deal with massive data. It is built on Spark. Seatunnel has a very rich set of plug-ins that support reading data from Kafka, HDFS, and Kudu, performing various data processing, and writing the results to ClickHouse, Elasticsearch or Kafka.
-Seatunnel的环境准备以及安装步骤这里就不一一赘述了,具体安装步骤可以参考上一篇文章或者访问 [Seatunnel Docs](/docs/introduction)
+The environment preparation and installation steps of Seatunnel will not be repeated here. For specific installation steps, please refer to the previous article or visit [Seatunnel Docs](/docs/introduction)
### Seatunnel Pipeline
-我们仅需要编写一个Seatunnel Pipeline的配置文件即可完成数据的导入。
+We only need to write a configuration file of Seatunnel Pipeline to complete the data import.
-配置文件包括四个部分,分别是Spark、Input、filter和Output。
+The configuration file includes four parts, namely Spark, Input, filter and Output.
#### Spark
-这一部分是Spark的相关配置,主要配置Spark执行时所需的资源大小。
+This part is the related configuration of Spark, which mainly configures the resource size required for Spark execution.
+
```
spark {
- // 这个配置必需填写
+ // This configuration is required
spark.sql.catalogImplementation = "hive"
spark.app.name = "seatunnel"
spark.executor.instances = 2
@@ -90,7 +91,7 @@ spark {
#### Input
-这一部分定义数据源,如下是从Hive文件中读取text格式数据的配置案例。
+This part defines the data source. The following is a configuration example of reading data in text format from a Hive file.
```
input {
@@ -101,15 +102,15 @@ input {
}
```
-看,很简单的一个配置就可以从Hive中读取数据了。其中`pre_sql`是从Hive中读取数据SQL,`table_name`是将读取后的数据,注册成为Spark中临时表的表名,可为任意字段。
+See, a very simple configuration can read data from Hive. `pre_sql` is the SQL to read data from Hive, and `table_name` is the name of the table that will register the read data as a temporary table in Spark, which can be any field.
-需要注意的是,必须保证hive的metastore是在服务状态。
+It should be noted that it must be ensured that the metastore of hive is in the service state.
-在Cluster、Client、Local模式下运行时,必须把`hive-site.xml`文件置于提交任务节点的$HADOOP_CONF目录下
+When running in Cluster, Client, Local mode, the `hive-site.xml` file must be placed in the $HADOOP_CONF directory of the submit task node
#### Filter
-在Filter部分,这里我们配置一系列的转化,我们这里把不需要的minute和hour字段丢弃。当然我们也可以在读取Hive的时候通过`pre_sql`不读取这些字段
+In the Filter section, here we configure a series of transformations, and here we discard the unnecessary minute and hour fields. Of course, we can also not read these fields through `pre_sql` when reading Hive
```
filter {
@@ -120,7 +121,8 @@ filter {
```
#### Output
-最后我们将处理好的结构化数据写入ClickHouse
+
+Finally, we write the processed structured data to ClickHouse
```
output {
@@ -137,7 +139,7 @@ output {
### Running Seatunnel
-我们将上述四部分配置组合成为我们的配置文件`config/batch.conf`。
+We combine the above four-part configuration into our configuration file `config/batch.conf`.
vim config/batch.conf
@@ -147,7 +149,7 @@ spark {
spark.executor.instances = 2
spark.executor.cores = 1
spark.executor.memory = "1g"
- // 这个配置必需填写
+ // This configuration is required
spark.sql.catalogImplementation = "hive"
}
input {
@@ -173,15 +175,15 @@ output {
}
```
-执行命令,指定配置文件,运行 Seatunnel,即可将数据写入ClickHouse。这里我们以本地模式为例。
+Execute the command, specify the configuration file, and run Seatunnel to write data to ClickHouse. Here we take the local mode as an example.
./bin/start-seatunnel.sh --config config/batch.conf -e client -m 'local[2]'
## Conclusion
-在这篇文章中,我们介绍了如何使用 Seatunnel 将Hive中的数据导入ClickHouse中。仅仅通过一个配置文件便可快速完成数据的导入,无需编写任何代码,十分简单。
+In this post, we covered how to import data from Hive into ClickHouse using Seatunnel. The data import can be completed quickly through only one configuration file without writing any code, which is very simple.
-希望了解 Seatunnel 与ClickHouse、Elasticsearch、Kafka、Hadoop结合使用的更多功能和案例,可以直接进入官网 [https://seatunnel.apache.org/](https://seatunnel.apache.org/)
+If you want to know more functions and cases of Seatunnel combined with ClickHouse, Elasticsearch, Kafka, Hadoop, you can go directly to the official website [https://seatunnel.apache.org/](https://seatunnel.apache.org/)
-- Power by [InterestingLab](https://github.com/InterestingLab)
diff --git a/blog/2021-12-30-spark-execute-elasticsearch.md b/blog/2021-12-30-spark-execute-elasticsearch.md
index 1ed633d..d24dd22 100644
--- a/blog/2021-12-30-spark-execute-elasticsearch.md
+++ b/blog/2021-12-30-spark-execute-elasticsearch.md
@@ -1,38 +1,38 @@
---
slug: spark-execute-elasticsearch
-title: 如何使用 Spark 快速将数据写入 Elasticsearch
+title: How to quickly write data to Elasticsearch using Spark
tags: [Spark, Kafka, Elasticsearch]
---
-说到数据写入 Elasticsearch,最先想到的肯定是Logstash。Logstash因为其简单上手、可扩展、可伸缩等优点被广大用户接受。但是尺有所短,寸有所长,Logstash肯定也有它无法适用的应用场景,比如:
+When it comes to writing data to Elasticsearch, the first thing that comes to mind must be Logstash. Logstash is accepted by the majority of users because of its simplicity, scalability, and scalability. However, the ruler is shorter and the inch is longer, and Logstash must have application scenarios that it cannot apply to, such as:
-* 海量数据ETL
-* 海量数据聚合
-* 多源数据处理
+* Massive data ETL
+* Massive data aggregation
+* Multi-source data processing
-为了满足这些场景,很多同学都会选择Spark,借助Spark算子进行数据处理,最后将处理结果写入Elasticsearch。
+In order to meet these scenarios, many students will choose Spark, use Spark operators to process data, and finally write the processing results to Elasticsearch.
-我们部门之前利用Spark对Nginx日志进行分析,统计我们的Web服务访问情况,将Nginx日志每分钟聚合一次最后将结果写入Elasticsearch,然后利用Kibana配置实时监控Dashboard。Elasticsearch和Kibana都很方便、实用,但是随着类似需求越来越多,如何快速通过Spark将数据写入Elasticsearch成为了我们的一大问题。
+Our department used Spark to analyze Nginx logs, counted our web service access, aggregated Nginx logs every minute and finally wrote the results to Elasticsearch, and then used Kibana to configure real-time monitoring of the Dashboard. Both Elasticsearch and Kibana are convenient and practical, but with more and more similar requirements, how to quickly write data to Elasticsearch through Spark has become a big problem for us.
-今天给大家推荐一款能够实现数据快速写入的黑科技 Seatunnel [https://github.com/apache/incubator-seatunnel](https://github.com/apache/incubator-seatunnel) 一个非常易用,高性能,能够应对海量数据的实时数据处理产品,它构建在Spark之上,简单易用,灵活配置,无需开发。
+Today, I would like to recommend a black technology Seatunnel [https://github.com/apache/incubator-seatunnel](https://github.com/apache/incubator-seatunnel) that can realize fast data writing. It is very easy to use , a high-performance, real-time data processing product that can deal with massive data. It is built on Spark and is easy to use, flexibly configured, and requires no development.

## Kafka to Elasticsearch
-和Logstash一样,Seatunnel同样支持多种类型的数据输入,这里我们以最常见的Kakfa作为输入源为例,讲解如何使用 Seatunnel 将数据快速写入Elasticsearch
+Like Logstash, Seatunnel also supports multiple types of data input. Here we take the most common Kakfa as the input source as an example to explain how to use Seatunnel to quickly write data to Elasticsearch
### Log Sample
-原始日志格式如下:
+The original log format is as follows:
```
127.0.0.1 elasticsearch.cn 114.250.140.241 0.001s "127.0.0.1:80" [26/Oct/2018:21:54:32 +0800] "GET /article HTTP/1.1" 200 123 "-" - "Dalvik/2.1.0 (Linux; U; Android 7.1.1; OPPO R11 Build/NMF26X)"
```
### Elasticsearch Document
-我们想要统计,一分钟每个域名的访问情况,聚合完的数据有以下字段:
+We want to count the visits of each domain name in one minute. The aggregated data has the following fields:
```
domain String
hostname String
@@ -43,20 +43,20 @@ count int
## Seatunnel with Elasticsearch
-接下来会给大家详细介绍,我们如何通过 Seatunnel 读取Kafka中的数据,对数据进行解析以及聚合,最后将处理结果写入Elasticsearch中。
+Next, I will introduce you in detail, how we read the data in Kafka through Seatunnel, parse and aggregate the data, and finally write the processing results into Elasticsearch.
### Seatunnel
-[Seatunnel](https://github.com/apache/incubator-seatunnel) 同样拥有着非常丰富的插件,支持从Kafka、HDFS、Hive中读取数据,进行各种各样的数据处理,并将结果写入Elasticsearch、Kudu或者Kafka中。
+[Seatunnel](https://github.com/apache/incubator-seatunnel) also has a very rich plug-in that supports reading data from Kafka, HDFS, Hive, performing various data processing, and converting the results Write to Elasticsearch, Kudu or Kafka.
### Prerequisites
-首先我们需要安装seatunnel,安装十分简单,无需配置系统环境变量
-1. 准备Spark环境
-2. 安装 Seatunnel
-3. 配置 Seatunnel
+First of all, we need to install seatunnel, the installation is very simple, no need to configure system environment variables
+1. Prepare the Spark environment
+2. Install Seatunnel
+3. Configure Seatunnel
-以下是简易步骤,具体安装可以参照 [Quick Start](/docs/quick-start)
+The following are simple steps, the specific installation can refer to [Quick Start](/docs/quick-start)
```yaml
cd /usr/local
@@ -67,20 +67,20 @@ unzip seatunnel-1.1.1.zip
cd seatunnel-1.1.1
vim config/seatunnel-env.sh
-# 指定Spark安装路径
+# Specify the Spark installation path
SPARK_HOME=${SPARK_HOME:-/usr/local/spark-2.2.0-bin-hadoop2.7}
```
### Seatunnel Pipeline
-与Logstash一样,我们仅需要编写一个Seatunnel Pipeline的配置文件即可完成数据的导入,相信了解Logstash的朋友可以很快入手 Seatunnel 配置。
+Like Logstash, we only need to write a configuration file of Seatunnel Pipeline to complete the data import. I believe that friends who know Logstash can start Seatunnel configuration soon.
-配置文件包括四个部分,分别是Spark、Input、filter和Output。
+The configuration file includes four parts, namely Spark, Input, filter and Output.
#### Spark
-这一部分是Spark的相关配置,主要配置Spark执行时所需的资源大小。
+This part is the related configuration of Spark, which mainly configures the resource size required for Spark execution.
```
spark {
spark.app.name = "seatunnel"
@@ -93,7 +93,7 @@ spark {
#### Input
-这一部分定义数据源,如下是从Kafka中读取数据的配置案例,
+This part defines the data source. The following is a configuration example of reading data from Kafka,
```
kafkaStream {
@@ -106,24 +106,24 @@ kafkaStream {
#### Filter
-在Filter部分,这里我们配置一系列的转化,包括正则解析将日志进行拆分、时间转换将HTTPDATE转化为Elasticsearch支持的日期格式、对Number类型的字段进行类型转换以及通过SQL进行数据聚合
+In the Filter section, here we configure a series of conversions, including regular parsing to split logs, time conversion to convert HTTPDATE to a date format supported by Elasticsearch, type conversion for fields of type Number, and data aggregation through SQL
```yaml
filter {
- # 使用正则解析原始日志
- # 最开始数据都在raw_message字段中
+ # Parse the original log using regex
+ # The initial data is in the raw_message field
grok {
source_field = "raw_message"
pattern = '%{NOTSPACE:hostname}\\s%{NOTSPACE:domain}\\s%{IP:remote_addr}\\s%{NUMBER:request_time}s\\s\"%{DATA:upstream_ip}\"\\s\\[%{HTTPDATE:timestamp}\\]\\s\"%{NOTSPACE:method}\\s%{DATA:url}\\s%{NOTSPACE:http_ver}\"\\s%{NUMBER:status}\\s%{NUMBER:body_bytes_send}\\s%{DATA:referer}\\s%{NOTSPACE:cookie_info}\\s\"%{DATA:user_agent}'
}
- # 将"dd/MMM/yyyy:HH:mm:ss Z"格式的数据转换为
- # Elasticsearch中支持的格式
+ # Convert data in "dd/MMM/yyyy:HH:mm:ss Z" format to
+ # format supported in Elasticsearch
date {
source_field = "timestamp"
target_field = "datetime"
source_time_format = "dd/MMM/yyyy:HH:mm:ss Z"
target_time_format = "yyyy-MM-dd'T'HH:mm:ss.SSS+08:00"
}
- ## 利用SQL对数据进行聚合
+ ## Aggregate data with SQL
sql {
table_name = "access_log"
sql = "select domain, hostname, int(status), datetime, count(*) from access_log group by domain, hostname, status, datetime"
@@ -132,7 +132,7 @@ filter {
```
#### Output
-最后我们将处理好的结构化数据写入Elasticsearch。
+Finally, we write the processed structured data to Elasticsearch.
```yaml
output {
@@ -147,7 +147,7 @@ output {
### Running Seatunnel
-我们将上述四部分配置组合成为我们的配置文件 `config/batch.conf`。
+We combine the above four-part configuration into our configuration file `config/batch.conf`.
vim config/batch.conf
@@ -168,21 +168,21 @@ input {
}
}
filter {
- # 使用正则解析原始日志
- # 最开始数据都在raw_message字段中
+ # Parse the original log using regex
+ # The initial data is in the raw_message field
grok {
source_field = "raw_message"
pattern = '%{IP:hostname}\\s%{NOTSPACE:domain}\\s%{IP:remote_addr}\\s%{NUMBER:request_time}s\\s\"%{DATA:upstream_ip}\"\\s\\[%{HTTPDATE:timestamp}\\]\\s\"%{NOTSPACE:method}\\s%{DATA:url}\\s%{NOTSPACE:http_ver}\"\\s%{NUMBER:status}\\s%{NUMBER:body_bytes_send}\\s%{DATA:referer}\\s%{NOTSPACE:cookie_info}\\s\"%{DATA:user_agent}'
}
- # 将"dd/MMM/yyyy:HH:mm:ss Z"格式的数据转换为
- # Elasticsearch中支持的格式
+ # Convert data in "dd/MMM/yyyy:HH:mm:ss Z" format to
+ # format supported in Elasticsearch
date {
source_field = "timestamp"
target_field = "datetime"
source_time_format = "dd/MMM/yyyy:HH:mm:ss Z"
target_time_format = "yyyy-MM-dd'T'HH:mm:00.SSS+08:00"
}
- ## 利用SQL对数据进行聚合
+ ## Aggregate data with SQL
sql {
table_name = "access_log"
sql = "select domain, hostname, status, datetime, count(*) from access_log group by domain, hostname, status, datetime"
@@ -198,11 +198,11 @@ output {
}
```
-执行命令,指定配置文件,运行 Seatunnel,即可将数据写入Elasticsearch。这里我们以本地模式为例。
+Execute the command, specify the configuration file, and run Seatunnel to write data to Elasticsearch. Here we take the local mode as an example.
./bin/start-seatunnel.sh --config config/batch.conf -e client -m 'local[2]'
-最后,写入Elasticsearch中的数据如下,再配上Kibana就可以实现Web服务的实时监控了^_^.
+Finally, the data written into Elasticsearch is as follows, and with Kibana, real-time monitoring of web services can be realized ^_^.
```
"_source": {
@@ -216,16 +216,16 @@ output {
## Conclusion
-在这篇文章中,我们介绍了如何通过 Seatunnel 将Kafka中的数据写入Elasticsearch中。仅仅通过一个配置文件便可快速运行一个Spark Application,完成数据的处理、写入,无需编写任何代码,十分简单。
+In this post, we introduced how to write data from Kafka to Elasticsearch via Seatunnel. You can quickly run a Spark Application with only one configuration file, complete data processing and writing, and do not need to write any code, which is very simple.
-当数据处理过程中有遇到Logstash无法支持的场景或者Logstah性能无法达到预期的情况下,都可以尝试使用 Seatunnel 解决问题。
+When there are scenarios that Logstash cannot support or the performance of Logstah cannot meet expectations during data processing, you can try to use Seatunnel to solve the problem.
-希望了解 Seatunnel 与Elasticsearch、Kafka、Hadoop结合使用的更多功能和案例,可以直接进入官网 [https://seatunnel.apache.org/](https://seatunnel.apache.org/)
+If you want to know more functions and cases of using Seatunnel in combination with Elasticsearch, Kafka and Hadoop, you can go directly to the official website [https://seatunnel.apache.org/](https://seatunnel.apache.org/)
-**我们近期会再发布一篇《如何用Spark和Elasticsearch做交互式数据分析》,敬请期待.**
+**We will publish another article "How to Use Spark and Elasticsearch for Interactive Data Analysis" in the near future, so stay tuned.**
## Contract us
-* 邮件列表 : **dev@seatunnel.apache.org**. 发送任意内容至 `dev-subscribe@seatunnel.apache.org`, 按照回复订阅邮件列表。
-* Slack: 发送 `Request to join SeaTunnel slack` 邮件到邮件列表 (`dev@seatunnel.apache.org`), 我们会邀请你加入(在此之前请确认已经注册Slack).
-* [bilibili B站 视频](https://space.bilibili.com/1542095008)
+* Mailing list : **dev@seatunnel.apache.org**. Send anything to `dev-subscribe@seatunnel.apache.org` and subscribe to the mailing list according to the replies.
+* Slack: Send a `Request to join SeaTunnel slack` email to the mailing list (`dev@seatunnel.apache.org`), and we will invite you to join (please make sure you are registered with Slack before doing so).
+* [bilibili B station video](https://space.bilibili.com/1542095008)
diff --git a/blog/2021-12-30-spark-execute-tidb.md b/blog/2021-12-30-spark-execute-tidb.md
index 90233a4..b3e6359 100644
--- a/blog/2021-12-30-spark-execute-tidb.md
+++ b/blog/2021-12-30-spark-execute-tidb.md
@@ -1,36 +1,36 @@
---
slug: spark-execute-tidb
-title: 怎么用 Spark 在 TiDB 上做 OLAP 分析
+title: How to use Spark to do OLAP analysis on TiDB
tags: [Spark, TiDB]
---
-# 怎么用Spark在TiDB上做OLAP分析
+# How to use Spark to do OLAP analysis on TiDB
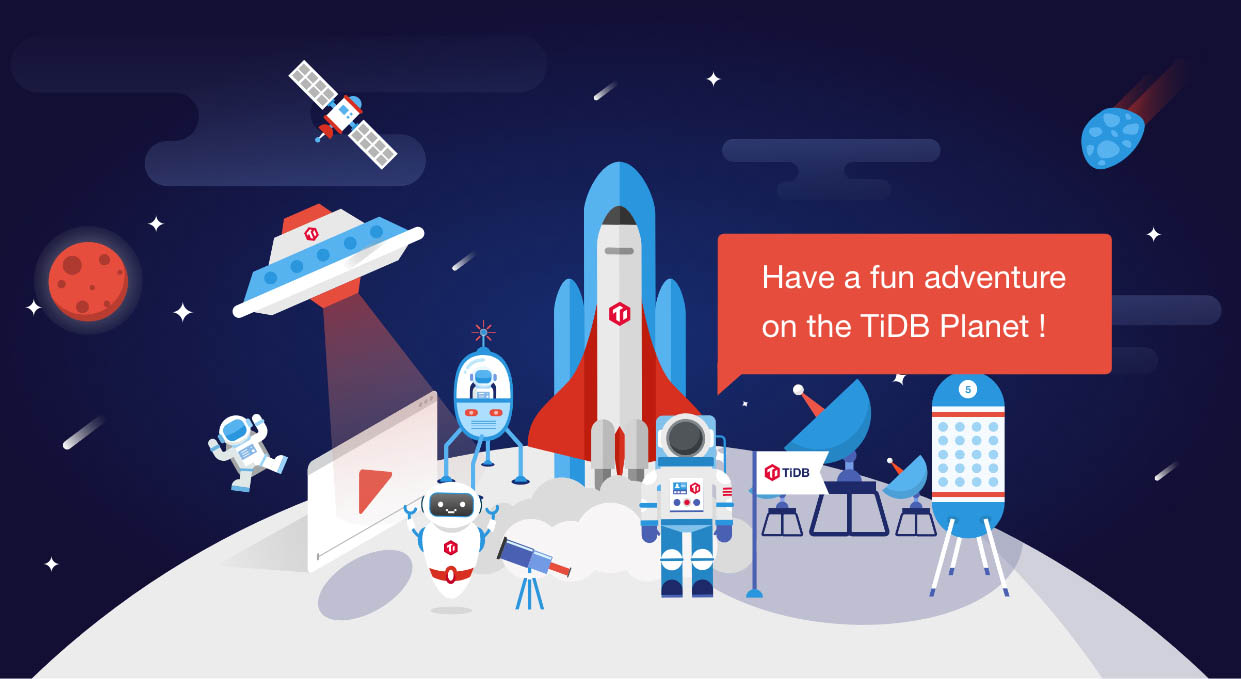
-[TiDB](https://github.com/pingcap/tidb) 是一款定位于在线事务处理/在线分析处理的融合型数据库产品,实现了一键水平伸缩,强一致性的多副本数据安全,分布式事务,实时 OLAP 等重要特性。
+[TiDB](https://github.com/pingcap/tidb) is a fusion database product targeting online transaction processing/online analytical processing. Distributed transactions, real-time OLAP and other important features.
-TiSpark 是 PingCAP 为解决用户复杂 OLAP 需求而推出的产品。它借助 Spark 平台,同时融合 TiKV 分布式集群的优势。
+TiSpark is a product launched by PingCAP to solve the complex OLAP needs of users. It uses the Spark platform and integrates the advantages of TiKV distributed clusters.
-直接使用 TiSpark 完成 OLAP 操作需要了解 Spark,还需要一些开发工作。那么,有没有一些开箱即用的工具能帮我们更快速地使用 TiSpark 在 TiDB 上完成 OLAP 分析呢?
+Completing OLAP operations with TiSpark directly requires knowledge of Spark and some development work. So, are there some out-of-the-box tools that can help us use TiSpark to complete OLAP analysis on TiDB more quickly?
-目前开源社区上有一款工具 **Seatunnel**,项目地址 [https://github.com/apache/incubator-seatunnel](https://github.com/apache/incubator-seatunnel) ,可以基于Spark,在 TiSpark 的基础上快速实现 TiDB 数据读取和 OLAP 分析。
+At present, there is a tool **Seatunnel** in the open source community, the project address [https://github.com/apache/incubator-seatunnel](https://github.com/apache/incubator-seatunnel), which can be based on Spark, Quickly implement TiDB data reading and OLAP analysis based on TiSpark.
-## 使用 Seatunnel 操作TiDB
+## Operating TiDB with Seatunnel
-在我们线上有这么一个需求,从 TiDB 中读取某一天的网站访问数据,统计每个域名以及服务返回状态码的访问次数,最后将统计结果写入 TiDB 另外一个表中。 我们来看看 Seatunnel 是如何实现这么一个功能的。
+We have such a requirement online. Read the website access data of a certain day from TiDB, count the number of visits of each domain name and the status code returned by the service, and finally write the statistical results to another table in TiDB. Let's see how Seatunnel implements such a function.
### Seatunnel
-[Seatunnel](https://github.com/apache/incubator-seatunnel) 是一个非常易用,高性能,能够应对海量数据的实时数据处理产品,它构建在 Spark 之上。Seatunnel 拥有着非常丰富的插件,支持从 TiDB、Kafka、HDFS、Kudu 中读取数据,进行各种各样的数据处理,然后将结果写入 TiDB、ClickHouse、Elasticsearch 或者 Kafka 中。
+[Seatunnel](https://github.com/apache/incubator-seatunnel) is a very easy-to-use, high-performance, real-time data processing product that can deal with massive data. It is built on Spark. Seatunnel has a very rich set of plugins that support reading data from TiDB, Kafka, HDFS, Kudu, perform various data processing, and then write the results to TiDB, ClickHouse, Elasticsearch or Kafka.
-#### 准备工作
+#### Ready to work
-##### 1. TiDB 表结构介绍
+##### 1. Introduction to TiDB table structure
-**Input**(存储访问日志的表)
+**Input** (table where access logs are stored)
```
CREATE TABLE access_log (
@@ -60,7 +60,7 @@ CREATE TABLE access_log (
+-----------------+--------------+------+------+---------+-------+
```
-**Output**(存储结果数据的表)
+**Output** (table where result data is stored)
```
CREATE TABLE access_collect (
@@ -82,46 +82,46 @@ CREATE TABLE access_collect (
+--------+-------------+------+------+---------+-------+
```
-##### 2. 安装 Seatunnel
+##### 2. Install Seatunnel
-有了 TiDB 输入和输出表之后, 我们需要安装 Seatunnel,安装十分简单,无需配置系统环境变量
-1. 准备 Spark环境
-2. 安装 Seatunnel
-3. 配置 Seatunnel
+After we have the input and output tables of TiDB, we need to install Seatunnel. The installation is very simple, and there is no need to configure system environment variables
+1. Prepare the Spark environment
+2. Install Seatunnel
+3. Configure Seatunnel
-以下是简易步骤,具体安装可以参照 [Quick Start](/docs/quick-start)
+The following are simple steps, the specific installation can refer to [Quick Start](/docs/quick-start)
```
-# 下载安装Spark
+# Download and install Spark
cd /usr/local
wget https://archive.apache.org/dist/spark/spark-2.1.0/spark-2.1.0-bin-hadoop2.7.tgz
tar -xvf https://archive.apache.org/dist/spark/spark-2.1.0/spark-2.1.0-bin-hadoop2.7.tgz
wget
-# 下载安装seatunnel
+# Download and install seatunnel
https://github.com/InterestingLab/seatunnel/releases/download/v1.2.0/seatunnel-1.2.0.zip
unzip seatunnel-1.2.0.zip
cd seatunnel-1.2.0
vim config/seatunnel-env.sh
-# 指定Spark安装路径
+# Specify the Spark installation path
SPARK_HOME=${SPARK_HOME:-/usr/local/spark-2.1.0-bin-hadoop2.7}
```
-### 实现 Seatunnel 处理流程
+### Implement the Seatunnel processing flow
-我们仅需要编写一个 Seatunnel 配置文件即可完成数据的读取、处理、写入。
+We only need to write a Seatunnel configuration file to read, process, and write data.
-Seatunnel 配置文件由四个部分组成,分别是 `Spark`、`Input`、`Filter` 和 `Output`。`Input` 部分用于指定数据的输入源,`Filter` 部分用于定义各种各样的数据处理、聚合,`Output` 部分负责将处理之后的数据写入指定的数据库或者消息队列。
+The Seatunnel configuration file consists of four parts, `Spark`, `Input`, `Filter` and `Output`. The `Input` part is used to specify the input source of the data, the `Filter` part is used to define various data processing and aggregation, and the `Output` part is responsible for writing the processed data to the specified database or message queue.
-整个处理流程为 `Input` -> `Filter` -> `Output`,整个流程组成了 Seatunnel 的 处理流程(Pipeline)。
+The whole processing flow is `Input` -> `Filter` -> `Output`, which constitutes the processing flow (Pipeline) of Seatunnel.
-> 以下是一个具体配置,此配置来源于线上实际应用,但是为了演示有所简化。
+> The following is a specific configuration, which is derived from an online practical application, but simplified for demonstration.
##### Input (TiDB)
-这里部分配置定义输入源,如下是从 TiDB 一张表中读取数据。
+This part of the configuration defines the input source. The following is to read data from a table in TiDB.
input {
tidb {
@@ -133,7 +133,7 @@ Seatunnel 配置文件由四个部分组成,分别是 `Spark`、`Input`、`Fil
##### Filter
-在Filter部分,这里我们配置一系列的转化, 大部分数据分析的需求,都是在Filter完成的。Seatunnel 提供了丰富的插件,足以满足各种数据分析需求。这里我们通过 SQL 插件完成数据的聚合操作。
+In the Filter section, here we configure a series of transformations, most of the data analysis requirements are completed in the Filter. Seatunnel provides a wealth of plug-ins enough to meet various data analysis needs. Here we complete the data aggregation operation through the SQL plugin.
filter {
sql {
@@ -145,7 +145,7 @@ Seatunnel 配置文件由四个部分组成,分别是 `Spark`、`Input`、`Fil
##### Output (TiDB)
-最后, 我们将处理后的结果写入TiDB另外一张表中。TiDB Output是通过JDBC实现的
+Finally, we write the processed results to another table in TiDB. TiDB Output is implemented through JDBC
output {
tidb {
@@ -159,10 +159,9 @@ Seatunnel 配置文件由四个部分组成,分别是 `Spark`、`Input`、`Fil
##### Spark
-这一部分是 Spark 的相关配置,主要配置 Spark 执行时所需的资源大小以及其他 Spark 配置。
-
-我们的 TiDB Input 插件是基于 TiSpark 实现的,而 TiSpark 依赖于 TiKV 集群和 Placement Driver (PD)。因此我们需要指定 PD 节点信息以及 TiSpark 相关配置`spark.tispark.pd.addresses`和`spark.sql.extensions`。
+This part is related to Spark configuration. It mainly configures the resource size required for Spark execution and other Spark configurations.
+Our TiDB Input plugin is implemented based on TiSpark, which relies on TiKV cluster and Placement Driver (PD). So we need to specify PD node information and TiSpark related configuration `spark.tispark.pd.addresses` and `spark.sql.extensions`.
spark {
spark.app.name = "seatunnel-tidb"
@@ -175,9 +174,9 @@ Seatunnel 配置文件由四个部分组成,分别是 `Spark`、`Input`、`Fil
}
-#### 运行 Seatunnel
+#### Run Seatunnel
-我们将上述四部分配置组合成我们最终的配置文件 `conf/tidb.conf`
+We combine the above four parts into our final configuration file `conf/tidb.conf`
```
spark {
@@ -213,7 +212,7 @@ output {
}
```
-执行命令,指定配置文件,运行 Seatunnel ,即可实现我们的数据处理逻辑。
+Execute the command, specify the configuration file, and run Seatunnel to implement our data processing logic.
* Local
@@ -227,9 +226,9 @@ output {
> ./bin/start-seatunnel.sh --config config/tidb.conf --deploy-mode cluster -master yarn
-如果是本机测试验证逻辑,用本地模式(Local)就可以了,一般生产环境下,都是使用`yarn-client`或者`yarn-cluster`模式。
+If it is a local test and verification logic, you can use the local mode (Local). Generally, in the production environment, the `yarn-client` or `yarn-cluster` mode is used.
-#### 检查结果
+#### test result
```
mysql> select * from access_collect;
@@ -244,20 +243,20 @@ mysql> select * from access_collect;
-## 总结
+## Conclusion
-在这篇文章中,我们介绍了如何使用 Seatunnel 从 TiDB 中读取数据,做简单的数据处理之后写入 TiDB 另外一个表中。仅通过一个配置文件便可快速完成数据的导入,无需编写任何代码。
+In this article, we introduced how to use Seatunnel to read data from TiDB, do simple data processing and write it to another table in TiDB. Data can be imported quickly with only one configuration file without writing any code.
-除了支持 TiDB 数据源之外,Seatunnel 同样支持Elasticsearch, Kafka, Kudu, ClickHouse等数据源。
+In addition to supporting TiDB data sources, Seatunnel also supports Elasticsearch, Kafka, Kudu, ClickHouse and other data sources.
-**于此同时,我们正在研发一个重要功能,就是在 Seatunnel 中,利用 TiDB 的事务特性,实现从 Kafka 到 TiDB 流式数据处理,并且支持端(Kafka)到端(TiDB)的 Exactly-Once 数据一致性。**
+**At the same time, we are developing an important function, which is to use the transaction features of TiDB in Seatunnel to realize streaming data processing from Kafka to TiDB, and support Exactly-Once data from end (Kafka) to end (TiDB). consistency. **
-希望了解 Seatunnel 和 TiDB,ClickHouse、Elasticsearch、Kafka结合使用的更多功能和案例,可以直接进入官网 [https://seatunnel.apache.org/](https://seatunnel.apache.org/)
+If you want to know more functions and cases of Seatunnel combined with TiDB, ClickHouse, Elasticsearch and Kafka, you can go directly to the official website [https://seatunnel.apache.org/](https://seatunnel.apache.org/)
-## 联系我们
-* 邮件列表 : **dev@seatunnel.apache.org**. 发送任意内容至 `dev-subscribe@seatunnel.apache.org`, 按照回复订阅邮件列表。
-* Slack: 发送 `Request to join SeaTunnel slack` 邮件到邮件列表 (`dev@seatunnel.apache.org`), 我们会邀请你加入(在此之前请确认已经注册Slack).
-* [bilibili B站 视频](https://space.bilibili.com/1542095008)
+## Contract us
+* Mailing list : **dev@seatunnel.apache.org**. Send anything to `dev-subscribe@seatunnel.apache.org` and subscribe to the mailing list according to the replies.
+* Slack: Send a `Request to join SeaTunnel slack` email to the mailing list (`dev@seatunnel.apache.org`), and we will invite you to join (please make sure you are registered with Slack before doing so).
+* [bilibili B station video](https://space.bilibili.com/1542095008)
-- Power by [InterestingLab](https://github.com/InterestingLab)
diff --git a/blog/2021-12-30-spark-structured-streaming.md b/blog/2021-12-30-spark-structured-streaming.md
index 4901519..4ecdf0c 100644
--- a/blog/2021-12-30-spark-structured-streaming.md
+++ b/blog/2021-12-30-spark-structured-streaming.md
@@ -1,34 +1,34 @@
---
slug: spark-structured-streaming
-title: 如何支持的 Spark StructuredStreaming
+title: How to support Spark StructuredStreaming
tags: [Spark, StructuredStreaming]
---
-# Seatunnel 最近支持的 StructuredStreaming 怎么用
+# How to use StructuredStreaming recently supported by Seatunnel
-### 前言
+### Foreword
-StructuredStreaming是Spark 2.0以后新开放的一个模块,相比SparkStreaming,它有一些比较突出的优点:<br/>   一、它能做到更低的延迟;<br/>
-  二、可以做实时的聚合,例如实时计算每天每个商品的销售总额;<br/>
-  三、可以做流与流之间的关联,例如计算广告的点击率,需要将广告的曝光记录和点击记录关联。<br/>
-以上几点如果使用SparkStreaming来实现可能会比较麻烦或者说是很难实现,但是使用StructuredStreaming实现起来会比较轻松。
-### 如何使用StructuredStreaming
-可能你没有详细研究过StructuredStreaming,但是发现StructuredStreaming能很好的解决你的需求,如何快速利用StructuredStreaming来解决你的需求?目前社区有一款工具 **Seatunnel**,项目地址:[https://github.com/apache/incubator-seatunnel](https://github.com/apache/incubator-seatunnel) ,
-可以高效低成本的帮助你利用StructuredStreaming来完成你的需求。
+StructuredStreaming is a newly opened module after Spark 2.0. Compared with SparkStreaming, it has some prominent advantages:<br/>   First, it can achieve lower latency;<br/>
+  Second, real-time aggregation can be done, such as real-time calculation of the total sales of each commodity every day;<br/>
+  Third, you can do the association between streams, for example, to calculate the click rate of an advertisement, you need to associate the exposure record of the advertisement with the click record. <br/>
+The above points may be cumbersome or difficult to implement if using SparkStreaming, but it will be easier to implement using StructuredStreaming.
+### How to use StructuredStreaming
+Maybe you have not studied StructuredStreaming in detail, but found that StructuredStreaming can solve your needs very well. How to quickly use StructuredStreaming to solve your needs? Currently there is a tool **Seatunnel** in the community, the project address: [https://github.com/apache/incubator-seatunnel](https://github.com/apache/incubator-seatunnel) ,
+It can help you use StructuredStreaming to complete your needs efficiently and at low cost.
### Seatunnel
-Seatunnel 是一个非常易用,高性能,能够应对海量数据的实时数据处理产品,它构建在Spark之上。Seatunnel 拥有着非常丰富的插件,支持从Kafka、HDFS、Kudu中读取数据,进行各种各样的数据处理,并将结果写入ClickHouse、Elasticsearch或者Kafka中
+Seatunnel is a very easy-to-use, high-performance, real-time data processing product that can deal with massive data. It is built on Spark. Seatunnel has a very rich set of plug-ins, supports reading data from Kafka, HDFS, Kudu, performs various data processing, and writes the results to ClickHouse, Elasticsearch or Kafka
-### 准备工作
+### Ready to work
-首先我们需要安装 Seatunnel,安装十分简单,无需配置系统环境变量
+First we need to install Seatunnel, the installation is very simple, no need to configure system environment variables
-1. 准备Spark环境
-2. 安装 Seatunnel
-3. 配置 Seatunnel
+1. Prepare the Spark environment
+2. Install Seatunnel
+3. Configure Seatunnel
-以下是简易步骤,具体安装可以参照 [Quick Start](/docs/quick-start)
+The following are simple steps, the specific installation can refer to [Quick Start](/docs/quick-start)
```
cd /usr/local
@@ -39,19 +39,19 @@ unzip seatunnel-1.3.0.zip
cd seatunnel-1.3.0
vim config/seatunnel-env.sh
-# 指定Spark安装路径
+# Specify the Spark installation path
SPARK_HOME=${SPARK_HOME:-/usr/local/spark-2.2.0-bin-hadoop2.7}
```
### Seatunnel Pipeline
-我们仅需要编写一个 Seatunnel Pipeline的配置文件即可完成数据的导入。
+We only need to write a configuration file of Seatunnel Pipeline to complete the data import.
-配置文件包括四个部分,分别是Spark、Input、filter和Output。
+The configuration file includes four parts, namely Spark, Input, filter and Output.
#### Spark
-这一部分是Spark的相关配置,主要配置Spark执行时所需的资源大小。
+This part is the related configuration of Spark, which mainly configures the resource size required for Spark execution.
```
spark {
@@ -64,7 +64,7 @@ spark {
#### Input
-下面是一个从kafka读取数据的例子
+Below is an example of reading data from kafka
```
kafkaStream {
@@ -74,12 +74,12 @@ kafkaStream {
}
```
-通过上面的配置就可以读取kafka里的数据了 ,topics是要订阅的kafka的topic,同时订阅多个topic可以以逗号隔开,consumer.bootstrap.servers就是Kafka的服务器列表,schema是可选项,因为StructuredStreaming从kafka读取到的值(官方固定字段value)是binary类型的,详见http://spark.apache.org/docs/latest/structured-streaming-kafka-integration.html
-但是如果你确定你kafka里的数据是json字符串的话,你可以指定schema,input插件将按照你指定的schema解析
+Through the above configuration, the data in kafka can be read. Topics is the topic of kafka to be subscribed to. Subscribing to multiple topics at the same time can be separated by commas. Consumer.bootstrap.servers is the list of Kafka servers, and schema is optional. Because the value read by StructuredStreaming from kafka (official fixed field value) is of binary type, see http://spark.apache.org/docs/latest/structured-streaming-kafka-integration.html
+But if you are sure that the data in your kafka is a json string, you can specify the schema, and the input plugin will parse it according to the schema you specify
#### Filter
-下面是一个简单的filter例子
+Here is a simple filter example
```
filter{
@@ -89,11 +89,11 @@ filter{
}
}
```
-`table_name`是注册成的临时表名,以便于在下面的sql使用
+`table_name` is the registered temporary table name for easy use in the following sql
#### Output
-处理好的数据往外输出,假设我们的输出也是kafka
+The processed data is output, assuming that our output is also kafka
```
output{
@@ -106,30 +106,30 @@ output{
}
```
-`topic` 是你要输出的topic,` producer.bootstrap.servers`是kafka集群列表,`streaming_output_mode`是StructuredStreaming的一个输出模式参数,有三种类型`append|update|complete`,具体使用参见文档http://spark.apache.org/docs/latest/structured-streaming-programming-guide.html#output-modes
+`topic` is the topic you want to output, `producer.bootstrap.servers` is a list of kafka clusters, `streaming_output_mode` is an output mode parameter of StructuredStreaming, there are three types of `append|update|complete`, for details, see the documentation http: //spark.apache.org/docs/latest/structured-streaming-programming-guide.html#output-modes
-`checkpointLocation`是StructuredStreaming的checkpoint路径,如果配置了的话,这个目录会存储程序的运行信息,比如程序退出再启动的话会接着上次的offset进行消费。
+`checkpointLocation` is the checkpoint path of StructuredStreaming. If configured, this directory will store the running information of the program. For example, if the program exits and restarts, it will continue to consume the last offset.
-### 场景分析
+### Scenario Analysis
-以上就是一个简单的例子,接下来我们就来介绍的稍微复杂一些的业务场景
+The above is a simple example. Next, we will introduce a slightly more complex business scenario.
-#### 场景一:实时聚合场景
+#### Scenario 1: Real-time aggregation scenario
-假设现在有一个商城,上面有10种商品,现在需要实时求每天每种商品的销售额,甚至是求每种商品的购买人数(不要求十分精确)。
-这么做的巨大的优势就是海量数据可以在实时处理的时候,完成聚合,再也不需要先将数据写入数据仓库,再跑离线的定时任务进行聚合,
-操作起来还是很方便的。
+Suppose there is now a mall with 10 kinds of products on it, and now it is necessary to find the daily sales of each product in real time, and even to find the number of buyers of each product (not very precise).
+The huge advantage of this is that massive data can be aggregated during real-time processing, and there is no need to write data into the data warehouse first, and then run offline scheduled tasks for aggregation.
+It is still very convenient to operate.
-kafka的数据如下
+The data of kafka is as follows
```
{"good_id":"abc","price":300,"user_id":123456,"time":1553216320}
```
-那我们该怎么利用 Seatunnel 来完成这个需求呢,当然还是只需要配置就好了。
+So how do we use Seatunnel to fulfill this requirement, of course, we only need to configure it.
```
-#spark里的配置根据业务需求配置
+#The configuration in spark is configured according to business requirements
spark {
spark.app.name = "seatunnel"
spark.executor.instances = 2
@@ -137,7 +137,7 @@ spark {
spark.executor.memory = "1g"
}
-#配置input
+#configure input
input {
kafkaStream {
topics = "good_topic"
@@ -146,28 +146,28 @@ input {
}
}
-#配置filter
+#configure filter
filter {
- #在程序做聚合的时候,内部会去存储程序从启动开始的聚合状态,久而久之会导致OOM,如果设置了watermark,程序自动的会去清理watermark之外的状态
- #这里表示使用ts字段设置watermark,界限为1天
+ #When the program is doing aggregation, it will internally store the aggregation state of the program since startup, which will lead to OOM over time. If the watermark is set, the program will automatically clean up the state other than the watermark.
+ #Here means use the ts field to set the watermark, the limit is 1 day
Watermark {
time_field = "time"
- time_type = "UNIX" #UNIX表示时间字段为10为的时间戳,还有其他的类型详细可以查看插件文档
- time_pattern = "yyyy-MM-dd" #这里之所以要把ts对其到天是因为求每天的销售额,如果是求每小时的销售额可以对其到小时`yyyy-MM-dd HH`
+ time_type = "UNIX" #UNIX represents a timestamp with a time field of 10, and other types can be found in the plugin documentation for details.
+ time_pattern = "yyyy-MM-dd" #The reason why the ts is assigned to the day is because the daily sales are sought, if the hourly sales are sought, the hour can be assigned `yyyy-MM-dd HH`
delay_threshold = "1 day"
- watermark_field = "ts" #设置watermark之后会新增一个字段,`ts`就是这个字段的名字
+ watermark_field = "ts" #After setting the watermark, a new field will be added, `ts` is the name of this field
}
- #之所以要group by ts是要让watermark生效,approx_count_distinct是一个估值,并不是精确的count_distinct
+ #The reason for group by ts is to make the watermark take effect, approx_count_distinct is an estimate, not an exact count_distinct
sql {
table_name = "good_table_2"
sql = "select good_id,sum(price) total, approx_count_distinct(user_id) person from good_table_2 group by ts,good_id"
}
}
-#接下来我们选择将结果实时输出到Kafka
+#Next we choose to output the results to Kafka in real time
output{
kafka {
topic = "seatunnel"
@@ -178,22 +178,22 @@ output{
}
```
-如上配置完成,启动 Seatunnel,就可以获取你想要的结果了。
+The above configuration is complete, start Seatunnel, and you can get the results you want.
-#### 场景二:多个流关联场景
+#### Scenario 2: Multiple stream association scenarios
-假设你在某个平台投放了广告,现在要实时计算出每个广告的CTR(点击率),数据分别来自两个topic,一个是广告曝光日志,一个是广告点击日志,
-此时我们就需要把两个流数据关联到一起做计算,而 Seatunnel 最近也支持了此功能,让我们一起看一下该怎么做:
+Suppose you have placed an advertisement on a certain platform, and now you need to calculate the CTR (click-through rate) of each advertisement in real time. The data comes from two topics, one is the advertisement exposure log, and the other is the advertisement click log.
+At this point, we need to associate the two stream data together for calculation, and Seatunnel also supports this function recently, let's take a look at how to do it:
-点击topic数据格式
+Click on topic data format
```
{"ad_id":"abc","click_time":1553216320,"user_id":12345}
```
-曝光topic数据格式
+Exposure topic data format
```
{"ad_id":"abc","show_time":1553216220,"user_id":12345}
@@ -201,7 +201,7 @@ output{
```
```
-#spark里的配置根据业务需求配置
+#The configuration in spark is configured according to business requirements
spark {
spark.app.name = "seatunnel"
spark.executor.instances = 2
@@ -209,7 +209,7 @@ spark {
spark.executor.memory = "1g"
}
-#配置input
+#configure input
input {
kafkaStream {
@@ -229,16 +229,16 @@ input {
filter {
- #左关联右表必须设置watermark
- #右关左右表必须设置watermark
+ #Left association right table must set watermark
+ #Right off left and right tables must set watermark
#http://spark.apache.org/docs/latest/structured-streaming-programming-guide.html#inner-joins-with-optional-watermarking
Watermark {
- source_table_name = "click_table" #这里可以指定为某个临时表添加watermark,不指定的话就是为input中的第一个
+ source_table_name = "click_table" #Here you can specify to add a watermark to a temporary table. If you don't specify it, it will be the first one in the input.
time_field = "time"
time_type = "UNIX"
delay_threshold = "3 hours"
watermark_field = "ts"
- result_table_name = "click_table_watermark" #添加完watermark之后可以注册成临时表,方便后续在sql中使用
+ result_table_name = "click_table_watermark" #After adding the watermark, it can be registered as a temporary table, which is convenient for subsequent use in sql
}
Watermark {
@@ -258,30 +258,29 @@ filter {
}
-#接下来我们选择将结果实时输出到Kafka
+#Next we choose to output the results to Kafka in real time
output {
kafka {
topic = "seatunnel"
producer.bootstrap.servers = "localhost:9092"
- streaming_output_mode = "append" #流关联只支持append模式
+ streaming_output_mode = "append" #Stream association only supports append mode
checkpointLocation = "/your/path"
}
}
```
+Through configuration, the case of stream association is also completed here.
-通过配置,到这里流关联的案例也完成了。
+### Conclusion
+Through configuration, you can quickly use StructuredStreaming for real-time data processing, but you still need to understand some concepts of StructuredStreaming, such as the watermark mechanism, and the output mode of the program.
-### 结语
-通过配置能很快的利用StructuredStreaming做实时数据处理,但是还是需要对StructuredStreaming的一些概念了解,比如其中的watermark机制,还有程序的输出模式。
+Finally, Seatunnel also supports spark streaming and spark batching of course.
+If you are also interested in these two, you can read our previous article "[How to quickly import data from Hive into ClickHouse](2021-12-30-hive-to-clickhouse.md)",
+"[Excellent data engineer, how to use Spark to do OLAP analysis on TiDB] (2021-12-30-spark-execute-tidb.md)",
+"[How to use Spark to quickly write data to Elasticsearch] (2021-12-30-spark-execute-elasticsearch.md)"
-最后,Seatunnel 当然还支持spark streaming和spark 批处理。
-如果你对这两个也感兴趣的话,可以阅读我们以前发布的文章《[如何快速地将Hive中的数据导入ClickHouse](2021-12-30-hive-to-clickhouse.md)》、
-《[优秀的数据工程师,怎么用Spark在TiDB上做OLAP分析](2021-12-30-spark-execute-tidb.md)》、
-《[如何使用Spark快速将数据写入Elasticsearch](2021-12-30-spark-execute-elasticsearch.md)》
-
-希望了解 Seatunnel 和 HBase, ClickHouse、Elasticsearch、Kafka、MySQL 等数据源结合使用的更多功能和案例,可以直接进入官网 [https://seatunnel.apache.org/](https://seatunnel.apache.org/)
+If you want to know more functions and cases of Seatunnel combined with HBase, ClickHouse, Elasticsearch, Kafka, MySQL and other data sources, you can go directly to the official website [https://seatunnel.apache.org/](https://seatunnel.apache. org/)
## 联系我们
-* 邮件列表 : **dev@seatunnel.apache.org**. 发送任意内容至 `dev-subscribe@seatunnel.apache.org`, 按照回复订阅邮件列表。
-* Slack: 发送 `Request to join SeaTunnel slack` 邮件到邮件列表 (`dev@seatunnel.apache.org`), 我们会邀请你加入(在此之前请确认已经注册Slack).
-* [bilibili B站 视频](https://space.bilibili.com/1542095008)
+* Mailing list : **dev@seatunnel.apache.org**. Send anything to `dev-subscribe@seatunnel.apache.org` and subscribe to the mailing list according to the replies.
+* Slack: Send a `Request to join SeaTunnel slack` email to the mailing list (`dev@seatunnel.apache.org`), and we will invite you to join (please make sure you are registered with Slack before doing so).
+* [bilibili B station video](https://space.bilibili.com/1542095008)
diff --git a/blog/2022-2-18-Meetup-vip.md b/blog/2022-2-18-Meetup-vip.md
index 3007fd6..8060034 100644
--- a/blog/2022-2-18-Meetup-vip.md
+++ b/blog/2022-2-18-Meetup-vip.md
@@ -1,100 +1,100 @@
---
-slug: SeaTunnel 在唯品会的实践
-title: SeaTunnel 在唯品会的实践
+slug: The practice of SeaTunnel in Vip
+title: The practice of SeaTunnel in Vip
tags:
-- 唯品会
+- Vip
- ClickHouse
---
-分享嘉宾:唯品会 资深大数据工程师 王玉
-讲稿整理:张德通
+Guest speaker: Vip Senior Big Data Engineer Wang Yu
+Lecture preparation: Zhang Detong
-导读: 唯品会早在1.0版本时就引用了SeaTunnel,我们使用SeaTunnel进行一些Hive到ClickHouse之间数据交互的工作。
-今天的介绍会围绕下面几点展开:
+Introduction: Vip referenced SeaTunnel as early as version 1.0. We use SeaTunnel to perform some data interaction work between Hive and ClickHouse.
+Today's presentation will focus on the following points:
-* ClickHouse数据导入的需求和痛点;
-* ClickHouse出仓入仓工具选型;
-* Hive to ClickHouse;
-* ClickHouse to Hive;
-* SeaTunnel与唯品会数据平台的集成;
-* 未来展望;
+* Requirements and pain points of ClickHouse data import;
+* Selection of ClickHouse warehousing and warehousing tools;
+* Hive to ClickHouse;
+* ClickHouse to Hive;
+* Integration of SeaTunnel and Vipshop data platform;
+* Future outlook;
-# ClickHouse数据导入的需求和痛点
-## 1.唯品会数据OLAP架构
-图中是唯品会OLAP架构,我们负责的模块是图中的数据服务和计算引擎两大部分。底层依赖的数据仓库分为离线数仓、实时数仓和湖仓。计算引擎方面,我们使用Presto、Kylin和Clickhouse。虽然Clickhouse是一个存储一体的OLAP数据库,我们为了利用Clickhouse的优秀计算性能而将它归入了计算引擎部分。基于OLAP组件之上,我们提供了SQL类数据服务和非SQL的唯品会自主分析,为不同智能服务。例如非SQL服务是为BI和商务提供更贴近业务的数据分析的服务。在数据服务至上抽象了多个数据应用。
+# Requirements and pain points of ClickHouse data import
+## 1. Vipshop Data OLAP Architecture
+The picture shows the OLAP architecture of Vipshop. The modules we are responsible for are the data service and the computing engine in the picture. The underlying data warehouses are divided into offline data warehouses, real-time data warehouses, and lake warehouses. For computing engines, we use Presto, Kylin and Clickhouse. Although Clickhouse is a storage-integrated OLAP database, we have included it in the computing engine part in order to take advantage of Clickhouse's excellent c [...]

-## 2.需求
-我们通过Presto Connector和Spark组件,把底层的Hive、Kudu、Alluxio组件打通。大数据组件之间可以互相导入导出数据,可以根据数据分析的需求和场景任意利用合适的组件分析数据。但我们引入Clickhouse时,它是一个数据孤岛,数据的导入和导出比较困难。Hive和Clickhouse之间需要做很多工作才能实现导入导出。我们的第一个数据导入导出需求就是提升导入导出效率,把Clickhouse纳入大数据体系中。
+## 2. Requirements
+We connect the underlying Hive, Kudu, and Alluxio components through Presto Connector and Spark components. Big data components can import and export data to and from each other, and you can use appropriate components to analyze data according to the needs and scenarios of data analysis. But when we introduced Clickhouse, it was a data island, and it was difficult to import and export data. There is a lot of work between Hive and Clickhouse to implement import and export. Our first data [...]

-第二个需求是Presto跑SQL比较慢,图中是一个慢SQL的例子。图中的SQL where条件设置了日期、时间范围和具体过滤条件,这类SQL使用由于Presto使用分区粒度下推,运行比较慢。即使用Hive的Bucket表和分桶等其他方式优化后也是几秒的返回时间、不能满足业务要求。这种情况下,我们需要利用Clickhouse做离线的OLAP计算加速。
+The second requirement is that Presto runs SQL relatively slowly. The figure shows an example of slow SQL. The SQL where condition in the figure sets the date, time range, and specific filter conditions. This kind of SQL usage runs slowly because Presto uses partition granularity to push down. Even after optimization by other methods such as Hive's bucket table and bucketing, the return time is a few seconds, which cannot meet business requirements. In this case, we need to use Clickhous [...]

-我们的实时数据是通过Kafka、Flink SQL方式写入到Clickhouse中。但分析时只用实时数据是不够的,需要用Hive维度表和已经ETL计算号的T+1实时表一起在Clickhouse中做加速运输。这需要把Hive的数据导入到Clickhouse中,这就是我们的第三个需求。
+Our real-time data is written to Clickhouse through Kafka and Flink SQL. However, it is not enough to use real-time data for analysis. It is necessary to use the Hive dimension table and the T+1 real-time table with the ETL calculation number for accelerated transportation in Clickhouse. This requires importing Hive data into Clickhouse, which is our third requirement.

-## 3.痛点
-首先,我们引入一项数据组件时要考虑其性能。Hive表粒度是五分钟,是否有组件可以支撑五分钟内完成一个短小ETL流程并把ETL结果导入到Clickhouse中?第二,我们需要保证数据质量,数据的准确性需要有保障。Hive和Clickhouse的数据条数需要保障一致性,如果数据质量出问题能否通过重跑等机制修复数据?第三,数据导入需要支持的数据类型是否完备?不同数据库之间的数据类型和一些机制不同,我们有HiperLogLog和BitMap这类在某一存储引擎中利用得比较多得数据类型,是否可以正确传输和识别,且可以较好地使用。
+## 3. Pain points
+First, we introduce a data component to consider its performance. The granularity of the Hive table is five minutes. Is there a component that can support a short ETL process and import the ETL results into Clickhouse within five minutes? Second, we need to ensure the quality of the data, and the accuracy of the data needs to be guaranteed. The number of data entries in Hive and Clickhouse needs to be consistent. If there is a problem with the data quality, can the data be repaired by re [...]
-# ClickHouse和Hive出仓入仓工具的选型
-基于数据业务上的痛点,我们对数据出仓入仓工具进行了对比和选择。我们主要在开源工具中进行选择,没有考虑商业出入仓工具,主要对比DataX、SeaTunnel和编写Spark程序并用jdbc插入ClickHouse这三个方案中取舍。
-SeaTunnel和Spark依赖唯品会自己的Yarn集群,可以直接实现分布式读取和写入。DataX是非分布式的,且Reader、Writer之间的启动过程耗时时间长,性能普通,SeaTunnel和Spark处理数据的性能可以达到DataX的数倍。
-十亿以上的数据可以平稳地在SeaTunnel和Spark中运行,DataX在数据量大以后性能压力大,处理十亿以上数据吃力。
-在读写插件扩展性方面,SeaTunnel支持了多种数据源,支持用户开发插件。SeaTunnel支持了数据导入Redis。
-稳定性上,SeaTunnel和DataX由于是自成体系的工具,稳定性会更好。Spark的稳定性方面需要关注代码质量。
+# Selection of ClickHouse and Hive warehousing and warehousing tools
+Based on the pain points in the data business, we have compared and selected data warehouse and warehouse tools. We mainly choose among open source tools, without considering commercial warehouse entry and exit tools, we mainly compare DataX, SeaTunnel, and write Spark programs and use jdbc to insert ClickHouse among the three options.
+SeaTunnel and Spark rely on Vipshop's own Yarn cluster, which can directly implement distributed reading and writing. DataX is non-distributed, and the startup process between Reader and Writer takes a long time, and the performance is ordinary. The performance of SeaTunnel and Spark for data processing can reach several times that of DataX.
+Data of more than one billion can run smoothly in SeaTunnel and Spark. DataX has great performance pressure after the amount of data is large, and it is difficult to process data of more than one billion.
+In terms of read and write plug-in scalability, SeaTunnel supports a variety of data sources and supports users to develop plug-ins. SeaTunnel supports data import into Redis.
+In terms of stability, since SeaTunnel and DataX are self-contained tools, the stability will be better. The stability aspect of Spark requires attention to code quality.

-我们的曝光表数据量每天在几十亿级,我们有5min内完成数据处理的性能要求,我们我们存在数据导入导出到Redis的需求,我们需要导入导出工具可以接入到数据平台上进行任务调度。 出于数据量级、性能、可扩展性、平台兼容性几方面的考虑,我们选择了SeaTunnel作为我们的数仓导入导出工具。
-# Hive数据导入到ClickHouse
-下面将介绍我们对SeaTunnel的使用。
-图中是一张Hive表,它是我们三级的商品维度表,包含品类商品、维度品类和用户人群信息。表的主键是一个三级品类ct_third_id,下面的value是两个uid的位图,是用户id的bitmap类型,我们要把这个Hive表导入到Clickhouse。
+The amount of data in our exposure table is in the billions of levels every day. We have the performance requirement to complete data processing within 5 minutes. We have the need to import and export data to Redis. We need import and export tools that can be connected to the data platform for task scheduling. . For the consideration of data volume, performance, scalability, and platform compatibility, we chose SeaTunnel as our data warehouse import and export tool.
+# Import Hive data into ClickHouse
+The following will introduce our use of SeaTunnel.
+The picture is a Hive table, which is our three-level product dimension table, including category products, dimension categories, and user population information. The primary key of the table is a third-level category ct_third_id, and the following value is the bitmap of two uids, which is the bitmap type of the user id. We need to import this Hive table into Clickhouse.

-SeaTunnel安装简单,官网文档有介绍如何安装。下图中是SeaTunnel的配置,配置中env、source和sink是必不可少的。env部分,图中的例子是Spark配置,配置了包括并发度等,可以调整这些参数。source部分是数据来源,这里配置了Hive数据源,包括一条Hive Select语句,Spark运行source配置中的SQL把数据读出,此处支持UDF进行简单ETL;sink部分配置了Clickhouse,可以看到output_type=rowbinary,rowbinary是唯品会自研加速方案;pre_sql和check_sql是自研的用于数据校验的功能,后面也会详细介绍;clickhouse.socket_timeout和bulk_size都是可以根据实际情况进行调整的。
+SeaTunnel is easy to install, and the official website documentation describes how to install it. The figure below shows the configuration of SeaTunnel. In the configuration, env, source and sink are essential. In the env part, the example in the figure is the Spark configuration. The configuration includes concurrency, etc. These parameters can be adjusted. The source part is the data source. The Hive data source is configured here, including a Hive Select statement. Spark runs the SQL [...]

-运行SeaTunnel,执行sh脚本文件、配置conf文件地址和yarn信息,后即可。
+Run SeaTunnel, execute the sh script file, configure the conf file address and yarn information, and then you can.

-运行过程中会产生Spark日志,运行成功和运行中错误都可以在日志中查看。
+Spark logs are generated during the running process, and both successful running and running errors can be viewed in the logs.

-为了更贴合业务,唯品会对SeaTunnel做了一些改进。我们的ETL任务都是需要重跑的,我们支持了pre_sql和check_sql实现数据的重跑和对数。主要流程是在数据准备好后,执行pre_sql进行预处理,在Clickhouse中执行删除旧分区数据、存放到某一目录下在失败时恢复该分区、rename这类操作。check_sql会检验,校验通过后整个流程结束;如果检验不通过,根据配置进行重跑,重跑不通过则报警到对应负责人。
+In order to better fit the business, Vipshop will make some improvements to SeaTunnel. All our ETL tasks need to be rerun. We support pre_sql and check_sql to implement data rerun and logarithm. The main process is to execute pre_sql for preprocessing after the data is ready, delete the old partition data in Clickhouse, store it in a directory, and restore the partition and rename when it fails. check_sql will check, and the whole process will end after the check is passed; if the check [...]

-唯品会基于1.0版本SeaTunnel增加了RowBinary做加速,也让HuperLogLog和BinaryBitmap的二进制文件能更容易地从Hive导入到Clickhouse。我们在ClickHouse-jdbc、bulk_size、Hive-source几处进行了修改。使用CK-jdbc的extended api,以rowbinary方式将数据写入CK,bulk_size引入了以rowbinary方式写入CK的控制逻辑,Hive-source
-RDD以HashPartitioner进行分区将数据打散,防止数据倾斜。
+Based on the 1.0 version of SeaTunnel, Vipshop has added RowBinary for acceleration, and it also makes it easier to import the binary files of HuperLogLog and BinaryBitmap from Hive to Clickhouse. We made changes in ClickHouse-jdbc, bulk_size, Hive-source. Use the extended api of CK-jdbc to write data to CK in rowbinary mode. Bulk_size introduces the control logic for writing to CK in rowbinary mode. Hive-source
+RDD is partitioned with HashPartitioner to break up data to prevent data from being skewed.
-我们还让SeaTunnel支持了多类型,为了圈人群的功能,需要在Clickhouse、Preso、Spark中实现对应的方法。我们在Clickhouse-jdbc中增加支持Batch特性的Callback、HttpEntity、RowBinaryStream,在Clickhouse-jdbc和Clickhouse-sink代码中增加了bitmap类型映射,在Presto和Spark中实现了Clickhouse的Hyperloglog和Bitmap的function的UDF。
-前面的配置中,Clickhouse-sink部分可以指定表名,这里有写入本地表和分布式表的差异。写入分布式表的性能比写入本地表差对Clickhouse集群的压力会更大,但在计算曝光表、流量表,ABTest等场景中需要两表Join,两张表量级均在几十亿。这时我们希望Join key落在本机,Join成本更小。我们建表时在Clickhouse的分布式表分布规则中配置murmurHash64规则,然后在Seatunnel的sink里直接配置分布式表,把写入规则交给Clickhouse,利用了分布式表的特性进行写入。写入本地表对Clickhouse的压力会更小,写入的性能也会更好。我们在Seatunnel里,根据sink的本地表,去Clickhouse的System.cluster表里获取表的分布信息和机器分布host。然后根据均分规则写入这些host。把数据分布式写入的事情放到Seatunnel里来做。
-针对本地表和分布式表的写入,我们未来的改造方向是在Seatunnel实现一致性哈希,直接按照一定规则写如Clickhouse、不依赖Clickhouse自身做数据分发,改善Clickhouse高CPU负载问题。
+We also let SeaTunnel support multiple types. In order to circle the crowd, corresponding methods need to be implemented in Clickhouse, Preso, and Spark. We added Callback, HttpEntity, and RowBinaryStream that support Batch feature to Clickhouse-jdbc, added bitmap type mapping to Clickhouse-jdbc and Clickhouse-sink code, and implemented UDF of Clickhouse's Hyperloglog and Bitmap functions in Presto and Spark.
+In the previous configuration, the clickhouse-sink part can specify the table name, and here is the difference between writing to the local table and the distributed table. The performance of writing to a distributed table is worse than that of writing to a local table, which will put more pressure on the Clickhouse cluster. However, in scenarios such as exposure meter, flow meter, and ABTest, two tables are required to join, and both tables are in the order of billions. . At this time, [...]
+For the writing of local tables and distributed tables, our future transformation direction is to implement consistent hashing in Seatunnel, write directly according to certain rules, such as Clickhouse, without relying on Clickhouse itself for data distribution, and improve Clickhouse's high CPU load problem.
-# ClickHouse数据导入到Hive
-我们有圈人群的需求,每天唯品会为供应商圈20万个人群,比如80后、高富帅、白富美的人群集合。这些在Clickhouse中的Bitmap人群信息需要导出到Hive表,在Hive中与其他ETL任务进行配合,最后推到PIKA交给外部媒体使用。我们使SeaTunnel将Clickhouse Bitmap人群数据反推到Hive。
+# ClickHouse data import into Hive
+We have the needs of people in the circle. Every day, Vipshop gathers 200,000 people in the supplier circle, such as people born in the 1980s, Gao Fushuai, and Bai Fumei. These Bitmap crowd information in Clickhouse needs to be exported to the Hive table, coordinated with other ETL tasks in Hive, and finally pushed to PIKA for use by external media. We made SeaTunnel back-push Clickhouse Bitmap crowd data to Hive.

-图中是SeaTunnel配置,我们把source配置为Clickhouse、sink配置为Hive,数据校验也配置在Hive内。
+The figure shows the SeaTunnel configuration. We configure the source as Clickhouse, the sink as Hive, and the data verification is also configured in Hive.

-由于我们接入SeaTunnel较早,我们对一些模块间进行了加工,包括新增plugin-spark-sink-hive模块、plugin-spark-source-ClickHouse模块,重写Spark Row相关方法,使其能封装经过Schem映射后的Clickhouse数据,重新构造StructField并生成最终需要落地Hive的DataFrame。最新版本已经有了很多source和sink组件,在SeaTunnel使用上更方便。现在也可以在SeaTunnel中直接集成Flink connector。
+Since we access SeaTunnel earlier, we have processed some modules, including adding plugin-spark-sink-hive module, plugin-spark-source-ClickHouse module, and rewriting Spark Row related methods so that they can be packaged through The Clickhouse data mapped by Schem, reconstruct the StructField and generate the DataFrame that finally needs to land on Hive. The latest version has many source and sink components, which is more convenient to use in SeaTunnel. It is now also possible to inte [...]
-# SeaTunnel与唯品会数据平台的集成
-各个公司都有自己的调度系统,例如白鲸、宙斯。唯品会的调度工具是数坊,调度工具中集成了数据传输工具。下面是调度系统架构图,其中包含各类数据的出入仓。
+# Integration of SeaTunnel and Vipshop Data Platform
+Each company has its own scheduling system, such as Beluga, Zeus. The scheduling tool of Vipshop is Shufang, and the scheduling tool integrates the data transmission tool. The following is the architecture diagram of the scheduling system, which includes the entry and exit of various types of data.

-SeaTunnel任务类型集成到平台中,图中是数坊的定时任务截图,可以看到选中的部分,是一个配置好的SeaTunnel任务,负责人、最近一次耗时,前后依赖任务的血缘信息,消耗的资源信息。下面展示了历史运行实例信息。
+The SeaTunnel task type is integrated into the platform. The picture is a screenshot of the scheduled task of Shufang. You can see that the selected part is a configured SeaTunnel task. resource information. The following shows the historical running instance information.

-我们把SeaTunnel集成到了调度系统中,数坊调度Master会根据任务类型把任务分配到对应的Agent上,根据Agent负载情况分配到合适的机器上运行,管控器把前台的任务调度配置和信息拉取到后生成SeaTunnel cluster,在类似于k8s pod、cgroup隔离的虚拟环境内进行执行。运行结果会由调度平台的数据质量监控判断任务是否完成、是否运行成功,失败时进行重跑和告警。
+We integrated SeaTunnel into the scheduling system. The Shufang Scheduling Master will assign tasks to the corresponding Agents according to the task types, and assign them to the appropriate machines to run according to the Agent load. The controller pulls the task scheduling configuration and information in the foreground. After arriving, a SeaTunnel cluster is generated and executed in a virtual environment similar to k8s pod and cgroup isolation. The running result will be judged by [...]

-SeaTunnel本身是一个工具化的组件,是为了进行数据血缘,数据质量,历史记录,高警监控,还包括资源分配这些信息的管控。我们把SeaTunnel集成到平台中,可以利用平台优势利用好SeaTunnel。
-圈存人群中利用了SeaTunnel进行处理。我们通过打点数据,把圈存人群按照路径和使用情况分为不同的人,或称千人千面,把用户打上标签,圈出的某一类人群推送给用户、分析师和供应商。
+SeaTunnel itself is a tool-based component, which is used to manage and control data blood relationship, data quality, historical records, high-alert monitoring, and resource allocation. We integrate SeaTunnel into the platform, and we can take advantage of the platform to take advantage of SeaTunnel.
+SeaTunnel is used for processing in the deposit crowd. By managing data, we divide the circled people into different people according to their paths and usage conditions, or thousands of people and thousands of faces, tag users, and push a certain type of people circled to users, analysts and suppliers.

-流量进入Kafka,通过Flink入仓,再通过ETL形成用户标签表,用户标签表生成后,我们通过Presto实现了的BitMap方法,把数据打成Hive中的宽表。用户通过在人群系统页面中框选词条创建任务,提交腾群,生成SQL查询Clickhouse BitMap。Clickhouse的BitMap查询速度非常快,由天生优势,我们需要把Hive的BitMap表通过SeaTunnel导入到Clickhouse中。圈完人群后我们需要把表落地,形成Clickhouse的一个分区或一条记录,再把生成的结果BitMap表通过SeaTunnel存储到Hive中去。最后同步工具会将Hive的BitMap人群结果同步给外部媒体仓库Pika。每天圈20w个人群左右。
-整个过程中SeaTunnel负责把数据从Hive导出到Clickhouse,Clickhouse的ETL流程完成后SeaTunnel把数据从Clickhouse导出到Hive。
-为了完成这样的需求,我们在Presto和Spark端现ClickHouse的Hyperloglog和BitMap的function的UDF;我们还开发Seatunnel接口,使得用户在ClickHouse里使用Bitmap方法圈出来的人群,可以直接通过Seatunnel写入Hive表,无需中间落地步骤。用户也可以在Hive里通过spark圈人群或者反解人群bitmap,调用SeaTunnel接口,使数据直接传输到ClickHouse的结果表,而无需中间落地。
-# 后续工作
-后续我们会进一步改善Clickhouse写入数据时CPU负载高的问题,下一步会在SeaTunnel中实现Clickhouse数据源和读取端的CK-local模式,读写分离,减轻Clickhouse压力。未来我们也会增加更多sink支持,如数据推送到Pika和相应的数据检查。
+The traffic enters Kafka, enters the warehouse through Flink, and then forms a user label table through ETL. After the user label table is generated, we use the BitMap method implemented by Presto to type the data into a wide table in Hive. Users create tasks by box-selecting entries on the crowd system page, submit to Tengqun, and generate SQL query Clickhouse BitMap. Clickhouse's BitMap query speed is very fast. Due to its inherent advantages, we need to import Hive's BitMap table into [...]
+During the whole process, SeaTunnel is responsible for exporting the data from Hive to Clickhouse. After the ETL process of Clickhouse is completed, SeaTunnel exports the data from Clickhouse to Hive.
+In order to fulfill this requirement, we implemented UDFs of ClickHouse's Hyperloglog and BitMap functions on Presto and Spark; we also developed the Seatunnel interface, so that the crowds circled by users using the Bitmap method in ClickHouse can be directly written to the Hive table through Seatunnel , without an intermediate landing step. Users can also call the SeaTunnel interface through spark to circle the crowd or reverse the crowd bitmap in Hive, so that the data can be directly [...]
+# Follow-up
+In the future, we will further improve the problem of high CPU load when Clickhouse writes data. In the next step, we will implement the CK-local mode of Clickhouse data source and read end in SeaTunnel, separate read and write, and reduce Clickhouse pressure. In the future we will also add more sink support, such as data push to Pika and corresponding data checking.
diff --git a/blog/2021-12-30-hdfs-to-clickhouse.md b/i18n/zh-CN/docusaurus-plugin-content-blog/2021-12-30-hdfs-to-clickhouse.md
similarity index 100%
copy from blog/2021-12-30-hdfs-to-clickhouse.md
copy to i18n/zh-CN/docusaurus-plugin-content-blog/2021-12-30-hdfs-to-clickhouse.md
diff --git a/blog/2021-12-30-hive-to-clickhouse.md b/i18n/zh-CN/docusaurus-plugin-content-blog/2021-12-30-hive-to-clickhouse.md
similarity index 92%
copy from blog/2021-12-30-hive-to-clickhouse.md
copy to i18n/zh-CN/docusaurus-plugin-content-blog/2021-12-30-hive-to-clickhouse.md
index 2289991..b3c1f2d 100644
--- a/blog/2021-12-30-hive-to-clickhouse.md
+++ b/i18n/zh-CN/docusaurus-plugin-content-blog/2021-12-30-hive-to-clickhouse.md
@@ -6,7 +6,7 @@ tags: [Hive, ClickHouse]
ClickHouse是面向OLAP的分布式列式DBMS。我们部门目前已经把所有数据分析相关的日志数据存储至ClickHouse这个优秀的数据仓库之中,当前日数据量达到了300亿。
-在之前的文章 [如何快速地把HDFS中的数据导入ClickHouse](2021-12-30-hdfs-to-clickhouse.md) 中我们提到过使用 Seatunnel [https://github.com/apache/incubator-seatunnel](https://github.com/apache/incubator-seatunnel) 对HDFS中的数据经过很简单的操作就可以将数据写入ClickHouse。HDFS中的数据一般是非结构化的数据,那么针对存储在Hive中的结构化数据,我们应该怎么操作呢?
+在之前的文章 [如何快速地把HDFS中的数据导入ClickHouse](i18n/zh-CN/docusaurus-plugin-content-blog/current/2021-12-30-hdfs-to-clickhouse.mdtent-blog/current/2021-12-30-hdfs-to-clickhouse.md) 中我们提到过使用 Seatunnel [https://github.com/apache/incubator-seatunnel](https://github.com/apache/incubator-seatunnel) 对HDFS中的数据经过很简单的操作就可以将数据写入ClickHouse。HDFS中的数据一般是非结构化的数据,那么针对存储在Hive中的结构化数据,我们应该怎么操作呢?

diff --git a/blog/2021-12-30-spark-execute-elasticsearch.md b/i18n/zh-CN/docusaurus-plugin-content-blog/2021-12-30-spark-execute-elasticsearch.md
similarity index 100%
copy from blog/2021-12-30-spark-execute-elasticsearch.md
copy to i18n/zh-CN/docusaurus-plugin-content-blog/2021-12-30-spark-execute-elasticsearch.md
diff --git a/blog/2021-12-30-spark-execute-tidb.md b/i18n/zh-CN/docusaurus-plugin-content-blog/2021-12-30-spark-execute-tidb.md
similarity index 100%
copy from blog/2021-12-30-spark-execute-tidb.md
copy to i18n/zh-CN/docusaurus-plugin-content-blog/2021-12-30-spark-execute-tidb.md
diff --git a/blog/2021-12-30-spark-structured-streaming.md b/i18n/zh-CN/docusaurus-plugin-content-blog/2021-12-30-spark-structured-streaming.md
similarity index 95%
copy from blog/2021-12-30-spark-structured-streaming.md
copy to i18n/zh-CN/docusaurus-plugin-content-blog/2021-12-30-spark-structured-streaming.md
index 4901519..af4363a 100644
--- a/blog/2021-12-30-spark-structured-streaming.md
+++ b/i18n/zh-CN/docusaurus-plugin-content-blog/2021-12-30-spark-structured-streaming.md
@@ -275,9 +275,9 @@ output {
通过配置能很快的利用StructuredStreaming做实时数据处理,但是还是需要对StructuredStreaming的一些概念了解,比如其中的watermark机制,还有程序的输出模式。
最后,Seatunnel 当然还支持spark streaming和spark 批处理。
-如果你对这两个也感兴趣的话,可以阅读我们以前发布的文章《[如何快速地将Hive中的数据导入ClickHouse](2021-12-30-hive-to-clickhouse.md)》、
-《[优秀的数据工程师,怎么用Spark在TiDB上做OLAP分析](2021-12-30-spark-execute-tidb.md)》、
-《[如何使用Spark快速将数据写入Elasticsearch](2021-12-30-spark-execute-elasticsearch.md)》
+如果你对这两个也感兴趣的话,可以阅读我们以前发布的文章《[如何快速地将Hive中的数据导入ClickHouse](i18n/zh-CN/docusaurus-plugin-content-blog/current/2021-12-30-hive-to-clickhouse.mdtent-blog/current/2021-12-30-hive-to-clickhouse.md)》、
+《[优秀的数据工程师,怎么用Spark在TiDB上做OLAP分析](i18n/zh-CN/docusaurus-plugin-content-blog/current/2021-12-30-spark-execute-tidb.mdtent-blog/current/2021-12-30-spark-execute-tidb.md)》、
+《[如何使用Spark快速将数据写入Elasticsearch](i18n/zh-CN/docusaurus-plugin-content-blog/2021-12-30-spark-execute-elasticsearch.md/current/2021-12-30-spark-execute-elasticsearch.md)》
希望了解 Seatunnel 和 HBase, ClickHouse、Elasticsearch、Kafka、MySQL 等数据源结合使用的更多功能和案例,可以直接进入官网 [https://seatunnel.apache.org/](https://seatunnel.apache.org/)
diff --git a/blog/2022-2-18-Meetup-vip.md b/i18n/zh-CN/docusaurus-plugin-content-blog/2022-2-18-Meetup-vip.md
similarity index 100%
copy from blog/2022-2-18-Meetup-vip.md
copy to i18n/zh-CN/docusaurus-plugin-content-blog/2022-2-18-Meetup-vip.md