You are viewing a plain text version of this content. The canonical link for it is here.
Posted to commits@dolphinscheduler.apache.org by zh...@apache.org on 2022/03/31 08:43:16 UTC
[dolphinscheduler-website] 01/01: [init] history docs first commit
This is an automated email from the ASF dual-hosted git repository.
zhongjiajie pushed a commit to branch history-docs
in repository https://gitbox.apache.org/repos/asf/dolphinscheduler-website.git
commit f777b12d3a0fa398b51210f034a749467303aaf3
Author: Jiajie Zhong <zh...@hotmail.com>
AuthorDate: Thu Mar 31 16:42:08 2022 +0800
[init] history docs first commit
---
.asf.yaml | 45 -
.babelrc | 22 -
.dlc.json | 36 -
.docsite | 0
.eslintrc | 37 -
.gitignore | 30 +
.htaccess | 23 -
.nojekyll | 0
README.md | 79 +-
asset/dolphinscheduler-netutils.jar | Bin 62446 -> 0 bytes
blog/en-us/Apache-DolphinScheduler-2.0.1.md | 274 --
blog/en-us/Apache_dolphinScheduler_2.0.2.md | 122 -
blog/en-us/Apache_dolphinScheduler_2.0.3.md | 146 -
blog/en-us/Apache_dolphinScheduler_2.0.5.md | 70 -
blog/en-us/Awarded_most_popular_project_in_2021.md | 77 -
blog/en-us/Board_of_Directors_Report.md | 99 -
blog/en-us/DAG.md | 166 --
blog/en-us/DS-2.0-alpha-release.md | 80 -
blog/en-us/DS_run_in_windows.md | 102 -
.../DolphinScheduler-Vulnerability-Explanation.md | 62 -
...hinScheduler_Kubernetes_Technology_in_action.md | 458 ---
blog/en-us/Eavy_Info.md | 96 -
...nd_practice_of_Tujia_Big_Data_Platform_Based.md | 169 --
blog/en-us/FAQ.md | 13 -
blog/en-us/Hangzhou_cisco.md | 138 -
...H_process_10_000+_workflow_instances_per_day.md | 175 --
.../Introducing-Apache-DolphinScheduler-1.3.9.md | 83 -
blog/en-us/Json_Split.md | 103 -
blog/en-us/K8s_Cisco_Hangzhou.md | 176 --
blog/en-us/Lizhi-case-study.md | 112 -
blog/en-us/Meetup_2022_02_26.md | 77 -
blog/en-us/Twos.md | 34 -
blog/en-us/YouZan-case-study.md | 387 ---
blog/en-us/architecture-design.md | 319 --
blog/en-us/meetup_2019_10_26.md | 15 -
blog/en-us/meetup_2019_12_08.md | 63 -
blog/zh-cn/Apache-DolphinScheduler-2.0.1.md | 265 --
blog/zh-cn/Apache_dolphinScheduler_2.0.2.md | 131 -
blog/zh-cn/Apache_dolphinScheduler_2.0.3.md | 121 -
blog/zh-cn/Apache_dolphinScheduler_2.0.5.md | 75 -
blog/zh-cn/Awarded_most_popular_project_in_2021.md | 56 -
blog/zh-cn/Board_of_Directors_Report.md | 80 -
blog/zh-cn/DAG.md | 167 --
blog/zh-cn/DS-2.0-alpha-release.md | 99 -
blog/zh-cn/DS_architecture_evolution.md | 135 -
blog/zh-cn/DS_run_in_windows.md | 107 -
...hinScheduler_Kubernetes_Technology_in_action.md | 457 ---
...203\205\345\206\265\350\257\264\346\230\216.md" | 43 -
blog/zh-cn/Eavy_Info.md | 109 -
...nd_practice_of_Tujia_Big_Data_Platform_Based.md | 145 -
blog/zh-cn/Hangzhou_cisco.md | 140 -
blog/zh-cn/Hangzhou_cisco.md.md | 140 -
...H_process_10_000+_workflow_instances_per_day.md | 156 -
blog/zh-cn/K8s_Cisco_Hangzhou.md | 116 -
blog/zh-cn/Lizhi-case-study.md | 135 -
blog/zh-cn/Meetup_2022_02_26.md | 89 -
blog/zh-cn/Twos.md | 44 -
blog/zh-cn/YouZan-case-study.md | 240 --
blog/zh-cn/about_blocking_task.md | 155 -
blog/zh-cn/architecture-design.md | 304 --
blog/zh-cn/cicd_workflow.md | 287 --
blog/zh-cn/dolphinscheduler_json.md | 799 -----
blog/zh-cn/ipalfish_tech_platform.md | 249 --
blog/zh-cn/json_split.md | 106 -
blog/zh-cn/live_online_2020_05_26.md | 28 -
blog/zh-cn/meetup_2019_10_26.md | 15 -
blog/zh-cn/meetup_2019_12_08.md | 56 -
blog/zh-cn/new_committer_wenjun.md | 50 -
blog/zh-cn/ut-guideline.md | 437 ---
blog/zh-cn/ut-template.md | 408 ---
community/en-us/DSIP.md | 91 -
community/en-us/development/DS-License.md | 42 -
community/en-us/development/become-a-committer.md | 11 -
community/en-us/development/code-conduct.md | 68 -
community/en-us/development/commit-message.md | 94 -
community/en-us/development/contribute.md | 40 -
community/en-us/development/document.md | 60 -
community/en-us/development/issue.md | 136 -
community/en-us/development/microbench.md | 100 -
community/en-us/development/pull-request.md | 94 -
community/en-us/development/submit-code.md | 63 -
community/en-us/development/subscribe.md | 23 -
community/en-us/development/unit-test.md | 118 -
community/en-us/join/e2e-guide.md | 36 -
community/en-us/join/review.md | 151 -
community/en-us/release-post.md | 47 -
community/en-us/release-prepare.md | 27 -
community/en-us/release.md | 469 ---
community/en-us/security.md | 8 -
community/en-us/team.md | 70 -
community/zh-cn/DSIP.md | 85 -
community/zh-cn/development/DS-License.md | 104 -
community/zh-cn/development/become-a-committer.md | 12 -
community/zh-cn/development/code-conduct.md | 68 -
community/zh-cn/development/commit-message.md | 89 -
community/zh-cn/development/contribute.md | 42 -
community/zh-cn/development/document.md | 60 -
community/zh-cn/development/issue.md | 217 --
community/zh-cn/development/microbench.md | 98 -
community/zh-cn/development/pull-request.md | 95 -
community/zh-cn/development/submit-code.md | 71 -
community/zh-cn/development/subscribe.md | 25 -
community/zh-cn/development/unit-test.md | 110 -
community/zh-cn/join/e2e-guide.md | 36 -
community/zh-cn/join/review.md | 139 -
community/zh-cn/release-post.md | 47 -
community/zh-cn/release-prepare.md | 27 -
community/zh-cn/release.md | 487 ---
community/zh-cn/security.md | 8 -
community/zh-cn/team.md | 72 -
development/en-us/api-standard.md | 100 -
development/en-us/architecture-design.md | 315 --
.../en-us/backend/mechanism/global-parameter.md | 61 -
development/en-us/backend/mechanism/overview.md | 6 -
development/en-us/backend/mechanism/task/switch.md | 8 -
development/en-us/backend/spi/alert.md | 75 -
development/en-us/backend/spi/datasource.md | 23 -
development/en-us/backend/spi/registry.md | 27 -
development/en-us/backend/spi/task.md | 15 -
development/en-us/development-environment-setup.md | 159 -
development/en-us/e2e-test.md | 198 --
development/en-us/frontend-development.md | 639 ----
development/en-us/have-questions.md | 65 -
development/zh-cn/api-standard.md | 102 -
development/zh-cn/architecture-design.md | 302 --
.../zh-cn/backend/mechanism/global-parameter.md | 61 -
development/zh-cn/backend/mechanism/overview.md | 6 -
development/zh-cn/backend/mechanism/task/switch.md | 8 -
development/zh-cn/backend/spi/alert.md | 71 -
development/zh-cn/backend/spi/datasource.md | 23 -
development/zh-cn/backend/spi/registry.md | 26 -
development/zh-cn/backend/spi/task.md | 15 -
development/zh-cn/development-environment-setup.md | 155 -
development/zh-cn/e2e-test.md | 195 --
development/zh-cn/frontend-development.md | 639 ----
development/zh-cn/have-questions.md | 65 -
{site_config => docs/1.2.0/configs}/docs1-2-0.js | 0
.../docs/en}/backend-deployment.md | 0
.../docs/en}/cluster-deployment.md | 0
.../docs/en}/frontend-deployment.md | 0
.../docs/en}/hardware-environment.md | 0
.../user_doc => 1.2.0/docs/en}/metadata-1.2.md | 0
.../user_doc => 1.2.0/docs/en}/quick-start.md | 0
.../docs/en}/standalone-deployment.md | 0
.../user_doc => 1.2.0/docs/en}/system-manual.md | 0
.../1.2.1/user_doc => 1.2.0/docs/en}/upgrade.md | 0
.../docs/zh}/backend-deployment.md | 0
.../docs/zh}/cluster-deployment.md | 0
.../user_doc => 1.2.0/docs/zh}/deployparam.md | 0
.../docs/zh}/frontend-deployment.md | 0
.../docs/zh}/hardware-environment.md | 0
.../docs/zh}/masterserver-code-analysis.md | 0
.../user_doc => 1.2.0/docs/zh}/metadata-1.2.md | 0
.../user_doc => 1.2.0/docs/zh}/quick-start.md | 0
.../docs/zh}/standalone-deployment.md | 0
.../user_doc => 1.2.0/docs/zh}/system-manual.md | 0
.../1.2.1/user_doc => 1.2.0/docs/zh}/upgrade.md | 0
{site_config => docs/1.2.1/configs}/docs1-2-1.js | 0
.../docs/en}/architecture-design.md | 0
.../docs/en}/backend-deployment.md | 0
.../docs/en}/frontend-deployment.md | 0
.../docs/en}/hardware-environment.md | 0
.../user_doc => 1.2.1/docs/en}/metadata-1.2.md | 0
.../docs/en}/plugin-development.md | 0
.../user_doc => 1.2.1/docs/en}/quick-start.md | 0
.../user_doc => 1.2.1/docs/en}/system-manual.md | 0
.../1.2.0/user_doc => 1.2.1/docs/en}/upgrade.md | 0
.../docs/zh}/architecture-design.md | 0
.../docs/zh}/backend-deployment.md | 0
.../docs/zh}/cluster-deployment.md | 0
.../user_doc => 1.2.1/docs/zh}/deployparam.md | 0
.../docs/zh}/frontend-deployment.md | 0
.../docs/zh}/hardware-environment.md | 0
.../user_doc => 1.2.1/docs/zh}/metadata-1.2.md | 0
.../1.2.1/user_doc => 1.2.1/docs/zh}/microbench.md | 0
.../docs/zh}/plugin-development.md | 0
.../user_doc => 1.2.1/docs/zh}/quick-start.md | 0
.../docs/zh}/standalone-deployment.md | 0
.../user_doc => 1.2.1/docs/zh}/system-manual.md | 0
.../1.2.0/user_doc => 1.2.1/docs/zh}/upgrade.md | 0
{site_config => docs/1.3.1/configs}/docs1-3-1.js | 0
.../docs/en}/architecture-design.md | 664 ++---
.../docs/en}/cluster-deployment.md | 0
.../docs/en}/configuration-file.md | 812 ++---
.../docs/en}/hardware-environment.md | 0
.../user_doc => 1.3.1/docs/en}/metadata-1.3.md | 0
.../user_doc => 1.3.1/docs/en}/quick-start.md | 0
.../docs/en}/standalone-deployment.md | 0
.../user_doc => 1.3.1/docs/en}/system-manual.md | 0
.../user_doc => 1.3.1/docs/en}/task-structure.md | 2272 +++++++-------
.../1.3.1/user_doc => 1.3.1/docs/en}/upgrade.md | 0
.../docs/zh}/architecture-design.md | 0
.../docs/zh}/cluster-deployment.md | 0
.../docs/zh}/configuration-file.md | 0
.../docs/zh}/hardware-environment.md | 0
.../user_doc => 1.3.1/docs/zh}/metadata-1.3.md | 0
.../user_doc => 1.3.1/docs/zh}/quick-start.md | 0
.../docs/zh}/standalone-deployment.md | 0
.../user_doc => 1.3.1/docs/zh}/system-manual.md | 0
.../user_doc => 1.3.1/docs/zh}/task-structure.md | 0
.../1.3.1/user_doc => 1.3.1/docs/zh}/upgrade.md | 0
{site_config => docs/1.3.2/configs}/docs1-3-2.js | 0
.../docs/en}/architecture-design.md | 664 ++---
.../docs/en}/cluster-deployment.md | 0
.../docs/en}/configuration-file.md | 815 ++---
.../docs/en}/expansion-reduction.md | 0
.../docs/en}/hardware-environment.md | 0
.../user_doc => 1.3.2/docs/en}/metadata-1.3.md | 0
.../user_doc => 1.3.2/docs/en}/quick-start.md | 0
.../docs/en}/standalone-deployment.md | 0
.../user_doc => 1.3.2/docs/en}/system-manual.md | 0
.../user_doc => 1.3.2/docs/en}/task-structure.md | 2272 +++++++-------
.../1.3.6/user_doc => 1.3.2/docs/en}/upgrade.md | 0
.../docs/zh}/architecture-design.md | 0
.../docs/zh}/cluster-deployment.md | 0
.../docs/zh}/configuration-file.md | 0
.../docs/zh}/expansion-reduction.md | 0
.../docs/zh}/hardware-environment.md | 0
.../user_doc => 1.3.2/docs/zh}/metadata-1.3.md | 0
.../user_doc => 1.3.2/docs/zh}/quick-start.md | 0
.../docs/zh}/standalone-deployment.md | 0
.../user_doc => 1.3.2/docs/zh}/system-manual.md | 0
.../user_doc => 1.3.2/docs/zh}/task-structure.md | 0
.../1.3.5/user_doc => 1.3.2/docs/zh}/upgrade.md | 0
{site_config => docs/1.3.3/configs}/docs1-3-3.js | 0
.../docs/en}/architecture-design.md | 664 ++---
.../docs/en}/cluster-deployment.md | 0
.../docs/en}/configuration-file.md | 815 +++--
.../docs/en}/expansion-reduction.md | 0
.../docs/en}/hardware-environment.md | 0
.../user_doc => 1.3.3/docs/en}/metadata-1.3.md | 0
.../user_doc => 1.3.3/docs/en}/quick-start.md | 0
.../docs/en}/standalone-deployment.md | 0
.../user_doc => 1.3.3/docs/en}/system-manual.md | 0
.../user_doc => 1.3.3/docs/en}/task-structure.md | 2272 +++++++-------
.../1.3.5/user_doc => 1.3.3/docs/en}/upgrade.md | 0
.../docs/zh}/architecture-design.md | 0
.../docs/zh}/cluster-deployment.md | 0
.../docs/zh}/configuration-file.md | 0
.../docs/zh}/expansion-reduction.md | 0
.../docs/zh}/hardware-environment.md | 0
.../user_doc => 1.3.3/docs/zh}/metadata-1.3.md | 0
.../user_doc => 1.3.3/docs/zh}/quick-start.md | 0
.../docs/zh}/standalone-deployment.md | 0
.../user_doc => 1.3.3/docs/zh}/system-manual.md | 0
.../user_doc => 1.3.3/docs/zh}/task-structure.md | 0
.../1.3.9/user_doc => 1.3.3/docs/zh}/upgrade.md | 0
{site_config => docs/1.3.4/configs}/docs1-3-4.js | 0
.../docs/en}/architecture-design.md | 664 ++---
.../docs/en}/cluster-deployment.md | 0
.../docs/en}/configuration-file.md | 0
.../docs/en}/docker-deployment.md | 0
.../docs/en}/expansion-reduction.md | 0
.../docs/en}/hardware-environment.md | 0
.../architecture => 1.3.4/docs/en}/load-balance.md | 0
.../user_doc => 1.3.4/docs/en}/metadata-1.3.md | 0
.../user_doc => 1.3.4/docs/en}/quick-start.md | 0
.../docs/en}/standalone-deployment.md | 0
.../user_doc => 1.3.4/docs/en}/system-manual.md | 0
.../user_doc => 1.3.4/docs/en}/task-structure.md | 0
.../1.3.4/user_doc => 1.3.4/docs/en}/upgrade.md | 0
.../docs/zh}/architecture-design.md | 0
.../docs/zh}/cluster-deployment.md | 0
.../docs/zh}/configuration-file.md | 0
.../docs/zh}/docker-deployment.md | 0
.../docs/zh}/expansion-reduction.md | 0
.../docs/zh}/hardware-environment.md | 0
.../user_doc => 1.3.4/docs/zh}/load-balance.md | 0
.../user_doc => 1.3.4/docs/zh}/metadata-1.3.md | 0
.../user_doc => 1.3.4/docs/zh}/quick-start.md | 0
.../docs/zh}/standalone-deployment.md | 0
.../user_doc => 1.3.4/docs/zh}/system-manual.md | 0
.../docs/zh}/task-structure.md | 0
.../1.3.8/user_doc => 1.3.4/docs/zh}/upgrade.md | 0
{site_config => docs/1.3.5/configs}/docs1-3-5.js | 0
.../docs/en}/architecture-design.md | 664 ++---
.../docs/en}/cluster-deployment.md | 0
.../docs/en}/configuration-file.md | 0
.../docs/en}/docker-deployment.md | 0
.../docs/en}/expansion-reduction.md | 0
.../docs/en}/hardware-environment.md | 0
.../docs/en}/kubernetes-deployment.md | 0
.../architecture => 1.3.5/docs/en}/load-balance.md | 0
.../user_doc => 1.3.5/docs/en}/metadata-1.3.md | 0
.../1.3.5/user_doc => 1.3.5/docs/en}/open-api.md | 0
.../user_doc => 1.3.5/docs/en}/quick-start.md | 0
.../docs/en}/standalone-deployment.md | 0
.../user_doc => 1.3.5/docs/en}/system-manual.md | 0
.../user_doc => 1.3.5/docs/en}/task-structure.md | 0
.../1.3.3/user_doc => 1.3.5/docs/en}/upgrade.md | 0
.../docs/zh}/architecture-design.md | 0
.../docs/zh}/cluster-deployment.md | 0
.../docs/zh}/configuration-file.md | 0
.../docs/zh}/docker-deployment.md | 0
.../docs/zh}/expansion-reduction.md | 0
.../docs/zh}/hardware-environment.md | 0
.../docs/zh}/kubernetes-deployment.md | 0
.../user_doc => 1.3.5/docs/zh}/load-balance.md | 0
.../user_doc => 1.3.5/docs/zh}/metadata-1.3.md | 0
.../1.3.5/user_doc => 1.3.5/docs/zh}/open-api.md | 0
.../user_doc => 1.3.5/docs/zh}/quick-start.md | 0
.../docs/zh}/standalone-deployment.md | 0
.../user_doc => 1.3.5/docs/zh}/system-manual.md | 0
.../docs/zh}/task-structure.md | 0
.../1.3.2/user_doc => 1.3.5/docs/zh}/upgrade.md | 0
{site_config => docs/1.3.6/configs}/docs1-3-6.js | 0
.../docs/en}/ambari-integration.md | 0
.../docs/en}/architecture-design.md | 664 ++---
.../docs/en}/cluster-deployment.md | 0
.../docs/en}/configuration-file.md | 0
.../docs/en}/docker-deployment.md | 0
.../docs/en}/expansion-reduction.md | 0
.../1.3.6/user_doc => 1.3.6/docs/en}/flink-call.md | 0
.../docs/en}/hardware-environment.md | 0
.../docs/en}/kubernetes-deployment.md | 0
.../architecture => 1.3.6/docs/en}/load-balance.md | 0
.../user_doc => 1.3.6/docs/en}/metadata-1.3.md | 0
.../user_doc/guide => 1.3.6/docs/en}/open-api.md | 2 +-
.../user_doc => 1.3.6/docs/en}/quick-start.md | 0
.../docs/en}/skywalking-agent-deployment.md | 0
.../docs/en}/standalone-deployment.md | 0
.../user_doc => 1.3.6/docs/en}/system-manual.md | 0
.../user_doc => 1.3.6/docs/en}/task-structure.md | 0
.../1.3.2/user_doc => 1.3.6/docs/en}/upgrade.md | 0
.../docs/zh}/architecture-design.md | 0
.../docs/zh}/cluster-deployment.md | 0
.../docs/zh}/configuration-file.md | 0
.../docs/zh}/docker-deployment.md | 0
.../docs/zh}/expansion-reduction.md | 0
.../user_doc/guide => 1.3.6/docs/zh}/flink-call.md | 0
.../docs/zh}/hardware-environment.md | 0
.../docs/zh}/kubernetes-deployment.md | 0
.../architecture => 1.3.6/docs/zh}/load-balance.md | 0
.../user_doc => 1.3.6/docs/zh}/metadata-1.3.md | 0
.../user_doc/guide => 1.3.6/docs/zh}/open-api.md | 0
.../user_doc => 1.3.6/docs/zh}/quick-start.md | 0
.../docs/zh}/skywalking-agent-deployment.md | 0
.../docs/zh}/standalone-deployment.md | 0
.../user_doc => 1.3.6/docs/zh}/system-manual.md | 0
.../docs/zh}/task-structure.md | 0
.../1.3.6/user_doc => 1.3.6/docs/zh}/upgrade.md | 0
{site_config => docs/1.3.8/configs}/docs1-3-8.js | 0
.../docs/en}/ambari-integration.md | 0
.../docs/en}/architecture-design.md | 0
.../docs/en}/cluster-deployment.md | 0
.../docs/en}/configuration-file.md | 0
.../docs/en}/docker-deployment.md | 0
.../docs/en}/expansion-reduction.md | 0
.../user_doc/guide => 1.3.8/docs/en}/flink-call.md | 0
.../docs/en}/hardware-environment.md | 0
.../docs/en}/kubernetes-deployment.md | 0
.../architecture => 1.3.8/docs/en}/load-balance.md | 0
.../user_doc => 1.3.8/docs/en}/metadata-1.3.md | 0
.../user_doc/guide => 1.3.8/docs/en}/open-api.md | 0
.../docs/en}/parameters-introduction.md | 0
.../user_doc => 1.3.8/docs/en}/quick-start.md | 0
.../docs/en}/skywalking-agent-deployment.md | 0
.../docs/en}/standalone-deployment.md | 0
.../user_doc => 1.3.8/docs/en}/system-manual.md | 0
.../docs/en}/task-structure.md | 0
.../1.3.9/user_doc => 1.3.8/docs/en}/upgrade.md | 0
.../docs/zh}/architecture-design.md | 0
.../docs/zh}/cluster-deployment.md | 0
.../docs/zh}/configuration-file.md | 0
.../docs/zh}/docker-deployment.md | 0
.../docs/zh}/expansion-reduction.md | 0
.../user_doc/guide => 1.3.8/docs/zh}/flink-call.md | 0
.../docs/zh}/hardware-environment.md | 0
.../docs/zh}/kubernetes-deployment.md | 0
.../architecture => 1.3.8/docs/zh}/load-balance.md | 0
.../user_doc => 1.3.8/docs/zh}/metadata-1.3.md | 0
.../user_doc/guide => 1.3.8/docs/zh}/open-api.md | 0
.../docs/zh}/parameters-introduction.md | 172 +-
.../user_doc => 1.3.8/docs/zh}/quick-start.md | 0
.../docs/zh}/skywalking-agent-deployment.md | 0
.../docs/zh}/standalone-deployment.md | 0
.../user_doc => 1.3.8/docs/zh}/system-manual.md | 0
.../docs/zh}/task-structure.md | 0
.../1.3.4/user_doc => 1.3.8/docs/zh}/upgrade.md | 0
{site_config => docs/1.3.9/configs}/docs1-3-9.js | 0
.../docs/en}/ambari-integration.md | 0
.../docs/en}/architecture-design.md | 0
.../docs/en}/cluster-deployment.md | 0
.../docs/en}/configuration-file.md | 0
.../docs/en}/docker-deployment.md | 0
.../docs/en}/expansion-reduction.md | 0
.../user_doc/guide => 1.3.9/docs/en}/flink-call.md | 0
.../docs/en}/hardware-environment.md | 0
.../docs/en}/kubernetes-deployment.md | 0
.../user_doc => 1.3.9/docs/en}/load-balance.md | 0
.../user_doc => 1.3.9/docs/en}/metadata-1.3.md | 0
.../user_doc/guide => 1.3.9/docs/en}/open-api.md | 0
.../docs/en}/parameters-introduction.md | 0
.../user_doc => 1.3.9/docs/en}/quick-start.md | 0
.../docs/en}/skywalking-agent-deployment.md | 0
.../docs/en}/standalone-deployment.md | 0
.../docs/en}/standalone-server.md | 0
.../user_doc => 1.3.9/docs/en}/system-manual.md | 0
.../docs/en}/task-structure.md | 0
.../1.3.8/user_doc => 1.3.9/docs/en}/upgrade.md | 0
.../docs/zh}/architecture-design.md | 0
.../docs/zh}/cluster-deployment.md | 0
.../docs/zh}/configuration-file.md | 0
.../docs/zh}/docker-deployment.md | 0
.../docs/zh}/expansion-reduction.md | 0
.../user_doc/guide => 1.3.9/docs/zh}/flink-call.md | 0
.../docs/zh}/hardware-environment.md | 0
.../docs/zh}/kubernetes-deployment.md | 0
.../architecture => 1.3.9/docs/zh}/load-balance.md | 0
.../user_doc => 1.3.9/docs/zh}/metadata-1.3.md | 0
.../user_doc/guide => 1.3.9/docs/zh}/open-api.md | 0
.../docs/zh}/parameters-introduction.md | 172 +-
.../user_doc => 1.3.9/docs/zh}/quick-start.md | 0
.../docs/zh}/skywalking-agent-deployment.md | 0
.../docs/zh}/standalone-deployment.md | 0
.../docs/zh}/standalone-server.md | 0
.../user_doc => 1.3.9/docs/zh}/system-manual.md | 0
.../docs/zh}/task-structure.md | 0
.../1.3.3/user_doc => 1.3.9/docs/zh}/upgrade.md | 0
{site_config => docs/2.0.0/configs}/docs2-0-0.js | 0
.../About_DolphinScheduler.md | 0
.../docs/en}/architecture/configuration.md | 0
.../docs/en}/architecture/design.md | 0
.../docs/en}/architecture/designplus.md | 0
.../docs/en/architecture}/load-balance.md | 0
.../docs/en}/architecture/metadata.md | 0
.../docs/en}/architecture/task-structure.md | 0
.../en}/guide/alert/alert_plugin_user_guide.md | 0
.../docs/en}/guide/alert/enterprise-wechat.md | 0
.../docs/en}/guide/datasource/hive.md | 0
.../docs/en}/guide/datasource/introduction.md | 0
.../docs/en}/guide/datasource/mysql.md | 0
.../docs/en}/guide/datasource/postgresql.md | 0
.../docs/en}/guide/datasource/spark.md | 0
.../docs/en}/guide/expansion-reduction.md | 0
.../user_doc => 2.0.0/docs/en}/guide/flink-call.md | 0
.../user_doc => 2.0.0/docs/en}/guide/homepage.md | 0
.../docs/en}/guide/installation/cluster.md | 0
.../docs/en}/guide/installation/docker.md | 0
.../docs/en}/guide/installation/hardware.md | 0
.../docs/en}/guide/installation/kubernetes.md | 0
.../docs/en}/guide/installation/pseudo-cluster.md | 0
.../docs/en}/guide/installation/standalone.md | 0
.../docs/en}/guide/introduction.md | 0
.../user_doc => 2.0.0/docs/en}/guide/monitor.md | 0
.../en}/guide/observability/skywalking-agent.md | 0
.../user_doc => 2.0.0/docs/en}/guide/open-api.md | 0
.../docs/en}/guide/parameter/built-in.md | 0
.../docs/en}/guide/parameter/context.md | 0
.../docs/en}/guide/parameter/global.md | 0
.../docs/en}/guide/parameter/local.md | 0
.../docs/en}/guide/parameter/priority.md | 0
.../docs/en}/guide/project/project-list.md | 0
.../docs/en}/guide/project/task-instance.md | 0
.../docs/en}/guide/project/workflow-definition.md | 0
.../docs/en}/guide/project/workflow-instance.md | 0
.../docs/en}/guide/quick-start.md | 0
.../user_doc => 2.0.0/docs/en}/guide/resource.md | 0
.../user_doc => 2.0.0/docs/en}/guide/security.md | 0
.../docs/en}/guide/task/conditions.md | 0
.../user_doc => 2.0.0/docs/en}/guide/task/datax.md | 0
.../docs/en}/guide/task/dependent.md | 0
.../user_doc => 2.0.0/docs/en}/guide/task/flink.md | 0
.../user_doc => 2.0.0/docs/en}/guide/task/http.md | 0
.../docs/en}/guide/task/map-reduce.md | 0
.../docs/en}/guide/task/pigeon.md | 0
.../docs/en}/guide/task/python.md | 0
.../user_doc => 2.0.0/docs/en}/guide/task/shell.md | 0
.../user_doc => 2.0.0/docs/en}/guide/task/spark.md | 0
.../user_doc => 2.0.0/docs/en}/guide/task/sql.md | 0
.../docs/en}/guide/task/stored-procedure.md | 0
.../docs/en}/guide/task/sub-process.md | 0
.../docs/en}/guide/task/switch.md | 0
.../user_doc => 2.0.0/docs/en}/guide/upgrade.md | 0
.../About_DolphinScheduler.md | 0
.../docs/zh}/architecture/configuration.md | 0
.../docs/zh}/architecture/design.md | 0
.../docs/zh}/architecture/designplus.md | 0
.../docs/zh}/architecture/load-balance.md | 0
.../docs/zh}/architecture/metadata.md | 0
.../docs/zh}/architecture/task-structure.md | 0
.../zh}/guide/alert/alert_plugin_user_guide.md | 0
.../docs/zh}/guide/alert/enterprise-wechat.md | 0
.../docs/zh}/guide/datasource/hive.md | 0
.../docs/zh}/guide/datasource/introduction.md | 0
.../docs/zh}/guide/datasource/mysql.md | 0
.../docs/zh}/guide/datasource/postgresql.md | 0
.../docs/zh}/guide/datasource/spark.md | 0
.../docs/zh}/guide/expansion-reduction.md | 0
.../user_doc => 2.0.0/docs/zh}/guide/flink-call.md | 0
.../user_doc => 2.0.0/docs/zh}/guide/homepage.md | 0
.../docs/zh}/guide/installation/cluster.md | 0
.../docs/zh}/guide/installation/docker.md | 0
.../docs/zh/guide/installation}/hardware.md | 0
.../docs/zh}/guide/installation/kubernetes.md | 0
.../docs/zh}/guide/installation/pseudo-cluster.md | 0
.../docs/zh}/guide/installation/standalone.md | 0
.../docs/zh}/guide/introduction.md | 0
.../user_doc => 2.0.0/docs/zh}/guide/monitor.md | 0
.../zh}/guide/observability/skywalking-agent.md | 0
.../user_doc => 2.0.0/docs/zh}/guide/open-api.md | 0
.../docs/zh}/guide/parameter/built-in.md | 0
.../docs/zh}/guide/parameter/context.md | 0
.../docs/zh}/guide/parameter/global.md | 0
.../docs/zh}/guide/parameter/local.md | 0
.../docs/zh}/guide/parameter/priority.md | 0
.../docs/zh}/guide/project/project-list.md | 0
.../docs/zh}/guide/project/task-instance.md | 0
.../docs/zh}/guide/project/workflow-definition.md | 0
.../docs/zh}/guide/project/workflow-instance.md | 0
.../docs/zh}/guide/quick-start.md | 0
.../user_doc => 2.0.0/docs/zh}/guide/resource.md | 0
.../user_doc => 2.0.0/docs/zh}/guide/security.md | 0
.../docs/zh}/guide/task/conditions.md | 0
.../user_doc => 2.0.0/docs/zh}/guide/task/datax.md | 0
.../docs/zh}/guide/task/dependent.md | 0
.../user_doc => 2.0.0/docs/zh}/guide/task/flink.md | 0
.../user_doc => 2.0.0/docs/zh}/guide/task/http.md | 0
.../docs/zh}/guide/task/map-reduce.md | 0
.../docs/zh}/guide/task/pigeon.md | 0
.../docs/zh}/guide/task/python.md | 0
.../user_doc => 2.0.0/docs/zh}/guide/task/shell.md | 0
.../user_doc => 2.0.0/docs/zh}/guide/task/spark.md | 0
.../user_doc => 2.0.0/docs/zh}/guide/task/sql.md | 0
.../docs/zh}/guide/task/stored-procedure.md | 0
.../docs/zh}/guide/task/sub-process.md | 0
.../docs/zh}/guide/task/switch.md | 0
.../user_doc => 2.0.0/docs/zh}/guide/upgrade.md | 0
{site_config => docs/2.0.1/configs}/docs2-0-1.js | 0
.../About_DolphinScheduler.md | 0
.../docs/en}/architecture/configuration.md | 0
.../docs/en}/architecture/design.md | 0
.../docs/en}/architecture/designplus.md | 0
.../docs/en/architecture}/load-balance.md | 0
.../docs/en}/architecture/metadata.md | 0
.../docs/en}/architecture/task-structure.md | 0
.../en}/guide/alert/alert_plugin_user_guide.md | 0
.../docs/en}/guide/alert/enterprise-wechat.md | 0
.../docs/en}/guide/datasource/hive.md | 0
.../docs/en}/guide/datasource/introduction.md | 0
.../docs/en}/guide/datasource/mysql.md | 0
.../docs/en}/guide/datasource/postgresql.md | 0
.../docs/en}/guide/datasource/spark.md | 0
.../docs/en}/guide/expansion-reduction.md | 0
.../user_doc => 2.0.1/docs/en}/guide/flink-call.md | 0
.../user_doc => 2.0.1/docs/en}/guide/homepage.md | 0
.../docs/en}/guide/installation/cluster.md | 0
.../docs/en}/guide/installation/docker.md | 0
.../docs/en}/guide/installation/hardware.md | 0
.../docs/en}/guide/installation/kubernetes.md | 0
.../docs/en}/guide/installation/pseudo-cluster.md | 0
.../docs/en}/guide/installation/standalone.md | 0
.../docs/en}/guide/introduction.md | 0
.../user_doc => 2.0.1/docs/en}/guide/monitor.md | 0
.../en}/guide/observability/skywalking-agent.md | 0
.../user_doc => 2.0.1/docs/en}/guide/open-api.md | 0
.../docs/en}/guide/parameter/built-in.md | 0
.../docs/en}/guide/parameter/context.md | 0
.../docs/en}/guide/parameter/global.md | 0
.../docs/en}/guide/parameter/local.md | 0
.../docs/en}/guide/parameter/priority.md | 0
.../docs/en}/guide/project/project-list.md | 0
.../docs/en}/guide/project/task-instance.md | 0
.../docs/en}/guide/project/workflow-definition.md | 0
.../docs/en}/guide/project/workflow-instance.md | 0
.../docs/en}/guide/quick-start.md | 0
.../user_doc => 2.0.1/docs/en}/guide/resource.md | 0
.../user_doc => 2.0.1/docs/en}/guide/security.md | 0
.../docs/en}/guide/task/conditions.md | 0
.../user_doc => 2.0.1/docs/en}/guide/task/datax.md | 0
.../docs/en}/guide/task/dependent.md | 0
.../user_doc => 2.0.1/docs/en}/guide/task/flink.md | 0
.../user_doc => 2.0.1/docs/en}/guide/task/http.md | 0
.../docs/en}/guide/task/map-reduce.md | 0
.../docs/en}/guide/task/pigeon.md | 0
.../docs/en}/guide/task/python.md | 0
.../user_doc => 2.0.1/docs/en}/guide/task/shell.md | 0
.../user_doc => 2.0.1/docs/en}/guide/task/spark.md | 0
.../user_doc => 2.0.1/docs/en}/guide/task/sql.md | 0
.../docs/en}/guide/task/stored-procedure.md | 0
.../docs/en}/guide/task/sub-process.md | 0
.../docs/en}/guide/task/switch.md | 0
.../user_doc => 2.0.1/docs/en}/guide/upgrade.md | 0
.../About_DolphinScheduler.md | 0
.../docs/zh}/architecture/configuration.md | 0
.../docs/zh}/architecture/design.md | 0
.../docs/zh}/architecture/designplus.md | 0
.../docs/zh}/architecture/load-balance.md | 0
.../docs/zh}/architecture/metadata.md | 0
.../docs/zh/architecture}/task-structure.md | 0
.../zh}/guide/alert/alert_plugin_user_guide.md | 0
.../docs/zh}/guide/alert/enterprise-wechat.md | 0
.../docs/zh}/guide/datasource/hive.md | 0
.../docs/zh}/guide/datasource/introduction.md | 0
.../docs/zh}/guide/datasource/mysql.md | 0
.../docs/zh}/guide/datasource/postgresql.md | 0
.../docs/zh}/guide/datasource/spark.md | 0
.../docs/zh}/guide/expansion-reduction.md | 0
.../user_doc => 2.0.1/docs/zh}/guide/flink-call.md | 0
.../user_doc => 2.0.1/docs/zh}/guide/homepage.md | 0
.../docs/zh}/guide/installation/cluster.md | 0
.../docs/zh}/guide/installation/docker.md | 0
.../docs/zh}/guide/installation/hardware.md | 0
.../docs/zh}/guide/installation/kubernetes.md | 0
.../docs/zh}/guide/installation/pseudo-cluster.md | 0
.../docs/zh}/guide/installation/standalone.md | 0
.../docs/zh}/guide/introduction.md | 0
.../user_doc => 2.0.1/docs/zh}/guide/monitor.md | 0
.../zh}/guide/observability/skywalking-agent.md | 0
.../user_doc => 2.0.1/docs/zh}/guide/open-api.md | 0
.../docs/zh}/guide/parameter/built-in.md | 0
.../docs/zh}/guide/parameter/context.md | 0
.../docs/zh}/guide/parameter/global.md | 0
.../docs/zh}/guide/parameter/local.md | 0
.../docs/zh}/guide/parameter/priority.md | 0
.../docs/zh}/guide/project/project-list.md | 0
.../docs/zh}/guide/project/task-instance.md | 0
.../docs/zh}/guide/project/workflow-definition.md | 0
.../docs/zh}/guide/project/workflow-instance.md | 0
.../docs/zh}/guide/quick-start.md | 0
.../user_doc => 2.0.1/docs/zh}/guide/resource.md | 0
.../user_doc => 2.0.1/docs/zh}/guide/security.md | 0
.../docs/zh}/guide/task/conditions.md | 0
.../user_doc => 2.0.1/docs/zh}/guide/task/datax.md | 0
.../docs/zh}/guide/task/dependent.md | 0
.../user_doc => 2.0.1/docs/zh}/guide/task/flink.md | 0
.../user_doc => 2.0.1/docs/zh}/guide/task/http.md | 0
.../docs/zh}/guide/task/map-reduce.md | 0
.../docs/zh}/guide/task/pigeon.md | 0
.../docs/zh}/guide/task/python.md | 0
.../user_doc => 2.0.1/docs/zh}/guide/task/shell.md | 0
.../user_doc => 2.0.1/docs/zh}/guide/task/spark.md | 0
.../user_doc => 2.0.1/docs/zh}/guide/task/sql.md | 0
.../docs/zh}/guide/task/stored-procedure.md | 0
.../docs/zh}/guide/task/sub-process.md | 0
.../docs/zh}/guide/task/switch.md | 0
.../user_doc => 2.0.1/docs/zh}/guide/upgrade.md | 0
{site_config => docs/2.0.2/configs}/docs2-0-2.js | 0
.../About_DolphinScheduler.md | 0
.../docs/en}/architecture/configuration.md | 0
.../docs/en}/architecture/design.md | 0
.../docs/en}/architecture/designplus.md | 0
.../docs/en/architecture}/load-balance.md | 0
.../docs/en}/architecture/metadata.md | 0
.../docs/en/architecture}/task-structure.md | 0
.../en}/guide/alert/alert_plugin_user_guide.md | 0
.../docs/en}/guide/alert/enterprise-wechat.md | 0
.../docs/en}/guide/datasource/hive.md | 0
.../docs/en}/guide/datasource/introduction.md | 0
.../docs/en}/guide/datasource/mysql.md | 0
.../docs/en}/guide/datasource/postgresql.md | 0
.../docs/en}/guide/datasource/spark.md | 0
.../docs/en}/guide/expansion-reduction.md | 0
.../user_doc => 2.0.2/docs/en/guide}/flink-call.md | 0
.../user_doc => 2.0.2/docs/en}/guide/homepage.md | 0
.../docs/en}/guide/installation/cluster.md | 0
.../docs/en}/guide/installation/docker.md | 0
.../docs/en}/guide/installation/hardware.md | 0
.../docs/en}/guide/installation/kubernetes.md | 0
.../docs/en}/guide/installation/pseudo-cluster.md | 0
.../docs/en}/guide/installation/standalone.md | 0
.../docs/en}/guide/introduction.md | 0
.../user_doc => 2.0.2/docs/en}/guide/monitor.md | 0
.../en}/guide/observability/skywalking-agent.md | 0
.../user_doc => 2.0.2/docs/en/guide}/open-api.md | 0
.../docs/en}/guide/parameter/built-in.md | 0
.../docs/en}/guide/parameter/context.md | 0
.../docs/en}/guide/parameter/global.md | 0
.../docs/en}/guide/parameter/local.md | 0
.../docs/en}/guide/parameter/priority.md | 0
.../docs/en}/guide/project/project-list.md | 0
.../docs/en}/guide/project/task-instance.md | 0
.../docs/en}/guide/project/workflow-definition.md | 0
.../docs/en}/guide/project/workflow-instance.md | 0
.../docs/en}/guide/quick-start.md | 0
.../user_doc => 2.0.2/docs/en}/guide/resource.md | 0
.../user_doc => 2.0.2/docs/en}/guide/security.md | 0
.../docs/en}/guide/task/conditions.md | 0
.../user_doc => 2.0.2/docs/en}/guide/task/datax.md | 0
.../docs/en}/guide/task/dependent.md | 0
.../user_doc => 2.0.2/docs/en}/guide/task/flink.md | 0
.../user_doc => 2.0.2/docs/en}/guide/task/http.md | 0
.../docs/en}/guide/task/map-reduce.md | 0
.../docs/en}/guide/task/pigeon.md | 0
.../docs/en}/guide/task/python.md | 0
.../user_doc => 2.0.2/docs/en}/guide/task/shell.md | 0
.../user_doc => 2.0.2/docs/en}/guide/task/spark.md | 0
.../user_doc => 2.0.2/docs/en}/guide/task/sql.md | 0
.../docs/en}/guide/task/stored-procedure.md | 0
.../docs/en}/guide/task/sub-process.md | 0
.../docs/en}/guide/task/switch.md | 0
.../user_doc => 2.0.2/docs/en}/guide/upgrade.md | 0
.../About_DolphinScheduler.md | 0
.../docs/zh}/architecture/configuration.md | 0
.../docs/zh}/architecture/design.md | 0
.../docs/zh}/architecture/designplus.md | 0
.../docs/zh}/architecture/load-balance.md | 0
.../docs/zh}/architecture/metadata.md | 0
.../docs/zh/architecture}/task-structure.md | 0
.../zh}/guide/alert/alert_plugin_user_guide.md | 0
.../docs/zh}/guide/alert/enterprise-wechat.md | 0
.../docs/zh}/guide/datasource/hive.md | 0
.../docs/zh}/guide/datasource/introduction.md | 0
.../docs/zh}/guide/datasource/mysql.md | 0
.../docs/zh}/guide/datasource/postgresql.md | 0
.../docs/zh}/guide/datasource/spark.md | 0
.../docs/zh}/guide/expansion-reduction.md | 0
.../user_doc => 2.0.2/docs/zh}/guide/flink-call.md | 0
.../user_doc => 2.0.2/docs/zh}/guide/homepage.md | 0
.../docs/zh}/guide/installation/cluster.md | 0
.../docs/zh}/guide/installation/docker.md | 0
.../docs/zh}/guide/installation/hardware.md | 0
.../docs/zh}/guide/installation/kubernetes.md | 0
.../docs/zh}/guide/installation/pseudo-cluster.md | 0
.../docs/zh}/guide/installation/standalone.md | 0
.../docs/zh}/guide/introduction.md | 0
.../user_doc => 2.0.2/docs/zh}/guide/monitor.md | 0
.../zh}/guide/observability/skywalking-agent.md | 0
.../user_doc => 2.0.2/docs/zh}/guide/open-api.md | 0
.../docs/zh}/guide/parameter/built-in.md | 0
.../docs/zh}/guide/parameter/context.md | 0
.../docs/zh}/guide/parameter/global.md | 0
.../docs/zh}/guide/parameter/local.md | 0
.../docs/zh}/guide/parameter/priority.md | 0
.../docs/zh}/guide/project/project-list.md | 0
.../docs/zh}/guide/project/task-instance.md | 0
.../docs/zh}/guide/project/workflow-definition.md | 0
.../docs/zh}/guide/project/workflow-instance.md | 0
.../docs/zh}/guide/quick-start.md | 0
.../user_doc => 2.0.2/docs/zh}/guide/resource.md | 0
.../user_doc => 2.0.2/docs/zh}/guide/security.md | 0
.../docs/zh}/guide/task/conditions.md | 0
.../user_doc => 2.0.2/docs/zh}/guide/task/datax.md | 0
.../docs/zh}/guide/task/dependent.md | 0
.../user_doc => 2.0.2/docs/zh}/guide/task/flink.md | 0
.../user_doc => 2.0.2/docs/zh}/guide/task/http.md | 0
.../docs/zh}/guide/task/map-reduce.md | 0
.../docs/zh}/guide/task/pigeon.md | 0
.../docs/zh}/guide/task/python.md | 0
.../user_doc => 2.0.2/docs/zh}/guide/task/shell.md | 0
.../user_doc => 2.0.2/docs/zh}/guide/task/spark.md | 0
.../user_doc => 2.0.2/docs/zh}/guide/task/sql.md | 0
.../docs/zh}/guide/task/stored-procedure.md | 0
.../docs/zh}/guide/task/sub-process.md | 0
.../docs/zh}/guide/task/switch.md | 0
.../user_doc => 2.0.2/docs/zh}/guide/upgrade.md | 0
{site_config => docs/2.0.3/configs}/docs2-0-3.js | 0
.../About_DolphinScheduler.md | 0
.../docs/en}/architecture/cache.md | 0
.../docs/en}/architecture/configuration.md | 0
.../docs/en}/architecture/design.md | 0
.../docs/en}/architecture/designplus.md | 0
.../docs/en}/architecture/load-balance.md | 0
.../docs/en}/architecture/metadata.md | 0
.../docs/en}/architecture/task-structure.md | 0
.../en}/guide/alert/alert_plugin_user_guide.md | 0
.../docs/en}/guide/alert/enterprise-wechat.md | 0
.../docs/en}/guide/datasource/hive.md | 0
.../docs/en}/guide/datasource/introduction.md | 0
.../docs/en}/guide/datasource/mysql.md | 0
.../docs/en}/guide/datasource/postgresql.md | 0
.../docs/en}/guide/datasource/spark.md | 0
.../docs/en}/guide/expansion-reduction.md | 0
.../user_doc => 2.0.3/docs/en}/guide/flink-call.md | 0
.../user_doc => 2.0.3/docs/en}/guide/homepage.md | 0
.../docs/en}/guide/installation/cluster.md | 0
.../docs/en}/guide/installation/docker.md | 0
.../docs/en}/guide/installation/hardware.md | 0
.../docs/en}/guide/installation/kubernetes.md | 0
.../docs/en}/guide/installation/pseudo-cluster.md | 0
.../docs/en}/guide/installation/standalone.md | 0
.../docs/en}/guide/introduction.md | 0
.../user_doc => 2.0.3/docs/en}/guide/monitor.md | 0
.../en}/guide/observability/skywalking-agent.md | 0
.../user_doc => 2.0.3/docs/en}/guide/open-api.md | 0
.../docs/en}/guide/parameter/built-in.md | 0
.../docs/en}/guide/parameter/context.md | 0
.../docs/en}/guide/parameter/global.md | 0
.../docs/en}/guide/parameter/local.md | 0
.../docs/en}/guide/parameter/priority.md | 0
.../docs/en}/guide/project/project-list.md | 0
.../docs/en}/guide/project/task-instance.md | 0
.../docs/en}/guide/project/workflow-definition.md | 0
.../docs/en}/guide/project/workflow-instance.md | 0
.../docs/en}/guide/quick-start.md | 0
.../user_doc => 2.0.3/docs/en}/guide/resource.md | 0
.../user_doc => 2.0.3/docs/en}/guide/security.md | 0
.../docs/en}/guide/task/conditions.md | 0
.../user_doc => 2.0.3/docs/en}/guide/task/datax.md | 0
.../docs/en}/guide/task/dependent.md | 0
.../user_doc => 2.0.3/docs/en}/guide/task/flink.md | 0
.../user_doc => 2.0.3/docs/en}/guide/task/http.md | 0
.../docs/en}/guide/task/map-reduce.md | 0
.../docs/en}/guide/task/pigeon.md | 0
.../docs/en}/guide/task/python.md | 0
.../user_doc => 2.0.3/docs/en}/guide/task/shell.md | 0
.../user_doc => 2.0.3/docs/en}/guide/task/spark.md | 0
.../user_doc => 2.0.3/docs/en}/guide/task/sql.md | 0
.../docs/en}/guide/task/stored-procedure.md | 0
.../docs/en}/guide/task/sub-process.md | 0
.../docs/en}/guide/task/switch.md | 0
.../user_doc => 2.0.3/docs/en}/guide/upgrade.md | 0
.../About_DolphinScheduler.md | 0
.../docs/zh}/architecture/cache.md | 0
.../docs/zh}/architecture/configuration.md | 0
.../docs/zh}/architecture/design.md | 0
.../docs/zh}/architecture/designplus.md | 0
.../docs/zh/architecture}/load-balance.md | 0
.../docs/zh}/architecture/metadata.md | 0
.../docs/zh/architecture}/task-structure.md | 0
.../zh}/guide/alert/alert_plugin_user_guide.md | 0
.../docs/zh}/guide/alert/enterprise-wechat.md | 0
.../docs/zh}/guide/datasource/hive.md | 0
.../docs/zh}/guide/datasource/introduction.md | 0
.../docs/zh}/guide/datasource/mysql.md | 0
.../docs/zh}/guide/datasource/postgresql.md | 0
.../docs/zh}/guide/datasource/spark.md | 0
.../docs/zh}/guide/expansion-reduction.md | 0
.../user_doc => 2.0.3/docs/zh/guide}/flink-call.md | 0
.../user_doc => 2.0.3/docs/zh}/guide/homepage.md | 0
.../docs/zh}/guide/installation/cluster.md | 0
.../docs/zh}/guide/installation/docker.md | 0
.../docs/zh}/guide/installation/hardware.md | 0
.../docs/zh}/guide/installation/kubernetes.md | 0
.../docs/zh}/guide/installation/pseudo-cluster.md | 0
.../docs/zh}/guide/installation/standalone.md | 0
.../docs/zh}/guide/introduction.md | 0
.../user_doc => 2.0.3/docs/zh}/guide/monitor.md | 0
.../zh}/guide/observability/skywalking-agent.md | 0
.../user_doc => 2.0.3/docs/zh/guide}/open-api.md | 0
.../docs/zh}/guide/parameter/built-in.md | 0
.../docs/zh}/guide/parameter/context.md | 0
.../docs/zh}/guide/parameter/global.md | 0
.../docs/zh}/guide/parameter/local.md | 0
.../docs/zh}/guide/parameter/priority.md | 0
.../docs/zh}/guide/project/project-list.md | 0
.../docs/zh}/guide/project/task-instance.md | 0
.../docs/zh}/guide/project/workflow-definition.md | 0
.../docs/zh}/guide/project/workflow-instance.md | 0
.../docs/zh}/guide/quick-start.md | 0
.../user_doc => 2.0.3/docs/zh}/guide/resource.md | 0
.../user_doc => 2.0.3/docs/zh}/guide/security.md | 0
.../docs/zh}/guide/task/conditions.md | 0
.../user_doc => 2.0.3/docs/zh}/guide/task/datax.md | 0
.../docs/zh}/guide/task/dependent.md | 0
.../user_doc => 2.0.3/docs/zh}/guide/task/flink.md | 0
.../user_doc => 2.0.3/docs/zh}/guide/task/http.md | 0
.../docs/zh}/guide/task/map-reduce.md | 0
.../docs/zh}/guide/task/pigeon.md | 0
.../docs/zh}/guide/task/python.md | 0
.../user_doc => 2.0.3/docs/zh}/guide/task/shell.md | 0
.../user_doc => 2.0.3/docs/zh}/guide/task/spark.md | 0
.../user_doc => 2.0.3/docs/zh}/guide/task/sql.md | 0
.../docs/zh}/guide/task/stored-procedure.md | 0
.../docs/zh}/guide/task/sub-process.md | 0
.../docs/zh}/guide/task/switch.md | 0
.../user_doc => 2.0.3/docs/zh}/guide/upgrade.md | 0
{site_config => docs/2.0.5/configs}/docs2-0-5.js | 0
.../About_DolphinScheduler.md | 0
.../docs/en}/architecture/cache.md | 0
.../docs/en}/architecture/configuration.md | 0
.../docs/en}/architecture/design.md | 0
.../docs/en}/architecture/designplus.md | 0
.../docs/en/architecture}/load-balance.md | 0
.../docs/en}/architecture/metadata.md | 0
.../docs/en/architecture}/task-structure.md | 0
.../en}/guide/alert/alert_plugin_user_guide.md | 0
.../docs/en}/guide/alert/dingtalk.md | 0
.../docs/en}/guide/alert/enterprise-wechat.md | 0
.../docs/en}/guide/datasource/hive.md | 0
.../docs/en}/guide/datasource/introduction.md | 0
.../docs/en}/guide/datasource/mysql.md | 0
.../docs/en}/guide/datasource/postgresql.md | 0
.../docs/en}/guide/datasource/spark.md | 0
.../docs/en}/guide/expansion-reduction.md | 0
.../user_doc => 2.0.5/docs/en/guide}/flink-call.md | 0
.../user_doc => 2.0.5/docs/en}/guide/homepage.md | 0
.../docs/en}/guide/installation/cluster.md | 0
.../docs/en}/guide/installation/docker.md | 0
.../docs/en}/guide/installation/hardware.md | 0
.../docs/en}/guide/installation/kubernetes.md | 0
.../docs/en}/guide/installation/pseudo-cluster.md | 0
.../docs/en}/guide/installation/standalone.md | 0
.../docs/en}/guide/introduction.md | 0
.../user_doc => 2.0.5/docs/en}/guide/monitor.md | 0
.../en}/guide/observability/skywalking-agent.md | 0
.../user_doc => 2.0.5/docs/en/guide}/open-api.md | 0
.../docs/en}/guide/parameter/built-in.md | 0
.../docs/en}/guide/parameter/context.md | 0
.../docs/en}/guide/parameter/global.md | 0
.../docs/en}/guide/parameter/local.md | 0
.../docs/en}/guide/parameter/priority.md | 0
.../docs/en}/guide/project/project-list.md | 0
.../docs/en}/guide/project/task-instance.md | 0
.../docs/en}/guide/project/workflow-definition.md | 0
.../docs/en}/guide/project/workflow-instance.md | 0
.../docs/en}/guide/quick-start.md | 0
.../user_doc => 2.0.5/docs/en}/guide/resource.md | 0
.../user_doc => 2.0.5/docs/en}/guide/security.md | 0
.../docs/en}/guide/task/conditions.md | 0
.../user_doc => 2.0.5/docs/en}/guide/task/datax.md | 0
.../docs/en}/guide/task/dependent.md | 0
.../user_doc => 2.0.5/docs/en}/guide/task/flink.md | 0
.../user_doc => 2.0.5/docs/en}/guide/task/http.md | 0
.../docs/en}/guide/task/map-reduce.md | 0
.../docs/en}/guide/task/pigeon.md | 0
.../docs/en}/guide/task/python.md | 0
.../user_doc => 2.0.5/docs/en}/guide/task/shell.md | 0
.../user_doc => 2.0.5/docs/en}/guide/task/spark.md | 0
.../user_doc => 2.0.5/docs/en}/guide/task/sql.md | 0
.../docs/en}/guide/task/stored-procedure.md | 0
.../docs/en}/guide/task/sub-process.md | 0
.../docs/en}/guide/task/switch.md | 0
.../user_doc => 2.0.5/docs/en}/guide/upgrade.md | 0
.../About_DolphinScheduler.md | 0
.../docs/zh}/architecture/cache.md | 0
.../docs/zh}/architecture/configuration.md | 0
.../docs/zh}/architecture/design.md | 0
.../docs/zh}/architecture/designplus.md | 0
.../docs/zh/architecture}/load-balance.md | 0
.../docs/zh}/architecture/metadata.md | 0
.../docs/zh/architecture}/task-structure.md | 0
.../zh}/guide/alert/alert_plugin_user_guide.md | 0
.../docs/zh}/guide/alert/dingtalk.md | 0
.../docs/zh}/guide/alert/enterprise-wechat.md | 0
.../docs/zh}/guide/datasource/hive.md | 0
.../docs/zh}/guide/datasource/introduction.md | 0
.../docs/zh}/guide/datasource/mysql.md | 0
.../docs/zh}/guide/datasource/postgresql.md | 0
.../docs/zh}/guide/datasource/spark.md | 0
.../docs/zh}/guide/expansion-reduction.md | 0
.../user_doc => 2.0.5/docs/zh/guide}/flink-call.md | 0
.../user_doc => 2.0.5/docs/zh}/guide/homepage.md | 0
.../docs/zh}/guide/installation/cluster.md | 0
.../docs/zh}/guide/installation/docker.md | 0
.../docs/zh}/guide/installation/hardware.md | 0
.../docs/zh}/guide/installation/kubernetes.md | 0
.../docs/zh}/guide/installation/pseudo-cluster.md | 0
.../docs/zh}/guide/installation/standalone.md | 0
.../docs/zh}/guide/introduction.md | 0
.../user_doc => 2.0.5/docs/zh}/guide/monitor.md | 0
.../zh}/guide/observability/skywalking-agent.md | 0
.../user_doc => 2.0.5/docs/zh/guide}/open-api.md | 0
.../docs/zh}/guide/parameter/built-in.md | 0
.../docs/zh}/guide/parameter/context.md | 0
.../docs/zh}/guide/parameter/global.md | 0
.../docs/zh}/guide/parameter/local.md | 0
.../docs/zh}/guide/parameter/priority.md | 0
.../docs/zh}/guide/project/project-list.md | 0
.../docs/zh}/guide/project/task-instance.md | 0
.../docs/zh}/guide/project/workflow-definition.md | 0
.../docs/zh}/guide/project/workflow-instance.md | 0
.../docs/zh}/guide/quick-start.md | 0
.../user_doc => 2.0.5/docs/zh}/guide/resource.md | 0
.../user_doc => 2.0.5/docs/zh}/guide/security.md | 0
.../docs/zh}/guide/task/conditions.md | 0
.../user_doc => 2.0.5/docs/zh}/guide/task/datax.md | 0
.../docs/zh}/guide/task/dependent.md | 0
.../user_doc => 2.0.5/docs/zh}/guide/task/flink.md | 0
.../user_doc => 2.0.5/docs/zh}/guide/task/http.md | 0
.../docs/zh}/guide/task/map-reduce.md | 0
.../docs/zh}/guide/task/pigeon.md | 0
.../docs/zh}/guide/task/python.md | 0
.../user_doc => 2.0.5/docs/zh}/guide/task/shell.md | 0
.../user_doc => 2.0.5/docs/zh}/guide/task/spark.md | 0
.../user_doc => 2.0.5/docs/zh}/guide/task/sql.md | 0
.../docs/zh}/guide/task/stored-procedure.md | 0
.../docs/zh}/guide/task/sub-process.md | 0
.../docs/zh}/guide/task/switch.md | 0
.../user_doc => 2.0.5/docs/zh}/guide/upgrade.md | 0
docs/en-us/1.3.5/user_doc/architecture-design.md | 332 ---
docs/en-us/1.3.6/user_doc/architecture-design.md | 332 ---
docs/en-us/1.3.6/user_doc/open-api.md | 64 -
.../1.3.8/user_doc/parameters-introduction.md | 80 -
docs/en-us/dev/user_doc/about/glossary.md | 79 -
docs/en-us/dev/user_doc/about/hardware.md | 48 -
docs/en-us/dev/user_doc/about/introduction.md | 19 -
docs/en-us/dev/user_doc/architecture/cache.md | 42 -
.../dev/user_doc/architecture/configuration.md | 424 ---
docs/en-us/dev/user_doc/architecture/design.md | 282 --
.../dev/user_doc/architecture/load-balance.md | 59 -
docs/en-us/dev/user_doc/architecture/metadata.md | 193 --
.../dev/user_doc/architecture/task-structure.md | 1114 -------
.../guide/alert/alert_plugin_user_guide.md | 14 -
docs/en-us/dev/user_doc/guide/alert/dingtalk.md | 27 -
.../user_doc/guide/alert/enterprise-webexteams.md | 64 -
.../dev/user_doc/guide/alert/enterprise-wechat.md | 14 -
docs/en-us/dev/user_doc/guide/alert/telegram.md | 42 -
docs/en-us/dev/user_doc/guide/datasource/hive.md | 39 -
.../dev/user_doc/guide/datasource/introduction.md | 6 -
docs/en-us/dev/user_doc/guide/datasource/mysql.md | 14 -
.../dev/user_doc/guide/datasource/postgresql.md | 13 -
docs/en-us/dev/user_doc/guide/datasource/spark.md | 13 -
.../dev/user_doc/guide/expansion-reduction.md | 248 --
docs/en-us/dev/user_doc/guide/flink-call.md | 123 -
docs/en-us/dev/user_doc/guide/homepage.md | 5 -
.../dev/user_doc/guide/installation/cluster.md | 39 -
.../dev/user_doc/guide/installation/docker.md | 1025 -------
.../dev/user_doc/guide/installation/kubernetes.md | 754 -----
.../user_doc/guide/installation/pseudo-cluster.md | 201 --
.../guide/installation/skywalking-agent.md | 74 -
.../dev/user_doc/guide/installation/standalone.md | 42 -
docs/en-us/dev/user_doc/guide/monitor.md | 32 -
docs/en-us/dev/user_doc/guide/open-api.md | 69 -
.../en-us/dev/user_doc/guide/parameter/built-in.md | 48 -
docs/en-us/dev/user_doc/guide/parameter/context.md | 66 -
docs/en-us/dev/user_doc/guide/parameter/global.md | 19 -
docs/en-us/dev/user_doc/guide/parameter/local.md | 19 -
.../en-us/dev/user_doc/guide/parameter/priority.md | 40 -
.../dev/user_doc/guide/project/project-list.md | 18 -
.../dev/user_doc/guide/project/task-instance.md | 11 -
.../user_doc/guide/project/workflow-definition.md | 114 -
.../user_doc/guide/project/workflow-instance.md | 62 -
docs/en-us/dev/user_doc/guide/resource.md | 165 -
docs/en-us/dev/user_doc/guide/security.md | 151 -
docs/en-us/dev/user_doc/guide/start/quick-start.md | 62 -
docs/en-us/dev/user_doc/guide/task/conditions.md | 36 -
docs/en-us/dev/user_doc/guide/task/datax.md | 63 -
docs/en-us/dev/user_doc/guide/task/dependent.md | 27 -
docs/en-us/dev/user_doc/guide/task/emr.md | 60 -
docs/en-us/dev/user_doc/guide/task/flink.md | 69 -
docs/en-us/dev/user_doc/guide/task/http.md | 47 -
docs/en-us/dev/user_doc/guide/task/map-reduce.md | 73 -
docs/en-us/dev/user_doc/guide/task/pigeon.md | 19 -
docs/en-us/dev/user_doc/guide/task/python.md | 15 -
docs/en-us/dev/user_doc/guide/task/shell.md | 43 -
docs/en-us/dev/user_doc/guide/task/spark.md | 68 -
docs/en-us/dev/user_doc/guide/task/sql.md | 43 -
.../dev/user_doc/guide/task/stored-procedure.md | 13 -
docs/en-us/dev/user_doc/guide/task/sub-process.md | 46 -
docs/en-us/dev/user_doc/guide/task/switch.md | 39 -
docs/en-us/dev/user_doc/guide/upgrade.md | 84 -
docs/en-us/release/faq.md | 708 -----
docs/en-us/release/history-versions.md | 71 -
docs/zh-cn/1.3.4/user_doc/task-structure.md | 1134 -------
docs/zh-cn/1.3.6/user_doc/flink-call.md | 150 -
docs/zh-cn/1.3.6/user_doc/load-balance.md | 58 -
docs/zh-cn/1.3.6/user_doc/open-api.md | 65 -
.../guide/alert/alert_plugin_user_guide.md | 12 -
.../user_doc/guide/datasource/introduction.md | 6 -
.../2.0.0/user_doc/guide/installation/cluster.md | 35 -
.../2.0.0/user_doc/guide/installation/hardware.md | 47 -
.../user_doc/guide/installation/standalone.md | 42 -
.../zh-cn/2.0.0/user_doc/guide/parameter/global.md | 19 -
docs/zh-cn/2.0.0/user_doc/guide/parameter/local.md | 19 -
.../2.0.0/user_doc/guide/project/task-instance.md | 11 -
.../user_doc/guide/project/workflow-instance.md | 61 -
docs/zh-cn/2.0.0/user_doc/guide/task/conditions.md | 36 -
docs/zh-cn/2.0.0/user_doc/guide/task/dependent.md | 27 -
.../2.0.0/user_doc/guide/task/stored-procedure.md | 12 -
docs/zh-cn/2.0.1/user_doc/guide/task/shell.md | 48 -
docs/zh-cn/2.0.3/user_doc/architecture/cache.md | 42 -
docs/zh-cn/2.0.5/user_doc/guide/alert/dingtalk.md | 26 -
docs/zh-cn/dev/user_doc/about/glossary.md | 58 -
docs/zh-cn/dev/user_doc/about/introduction.md | 12 -
.../dev/user_doc/architecture/configuration.md | 406 ---
docs/zh-cn/dev/user_doc/architecture/design.md | 287 --
docs/zh-cn/dev/user_doc/architecture/metadata.md | 185 --
.../user_doc/guide/alert/enterprise-webexteams.md | 66 -
.../dev/user_doc/guide/alert/enterprise-wechat.md | 13 -
docs/zh-cn/dev/user_doc/guide/alert/telegram.md | 41 -
docs/zh-cn/dev/user_doc/guide/datasource/hive.md | 40 -
docs/zh-cn/dev/user_doc/guide/datasource/mysql.md | 13 -
.../dev/user_doc/guide/datasource/postgresql.md | 13 -
docs/zh-cn/dev/user_doc/guide/datasource/spark.md | 19 -
.../dev/user_doc/guide/expansion-reduction.md | 245 --
docs/zh-cn/dev/user_doc/guide/homepage.md | 5 -
.../dev/user_doc/guide/installation/kubernetes.md | 755 -----
.../user_doc/guide/installation/pseudo-cluster.md | 200 --
.../guide/installation/skywalking-agent.md | 74 -
docs/zh-cn/dev/user_doc/guide/monitor.md | 32 -
.../zh-cn/dev/user_doc/guide/parameter/built-in.md | 49 -
docs/zh-cn/dev/user_doc/guide/parameter/context.md | 69 -
.../zh-cn/dev/user_doc/guide/parameter/priority.md | 40 -
.../dev/user_doc/guide/project/project-list.md | 17 -
.../user_doc/guide/project/workflow-definition.md | 109 -
docs/zh-cn/dev/user_doc/guide/resource.md | 168 --
docs/zh-cn/dev/user_doc/guide/security.md | 150 -
docs/zh-cn/dev/user_doc/guide/start/docker.md | 1024 -------
docs/zh-cn/dev/user_doc/guide/start/quick-start.md | 60 -
docs/zh-cn/dev/user_doc/guide/task/datax.md | 59 -
docs/zh-cn/dev/user_doc/guide/task/emr.md | 58 -
docs/zh-cn/dev/user_doc/guide/task/flink.md | 69 -
docs/zh-cn/dev/user_doc/guide/task/http.md | 48 -
docs/zh-cn/dev/user_doc/guide/task/map-reduce.md | 73 -
docs/zh-cn/dev/user_doc/guide/task/pigeon.md | 19 -
docs/zh-cn/dev/user_doc/guide/task/python.md | 15 -
docs/zh-cn/dev/user_doc/guide/task/spark.md | 69 -
docs/zh-cn/dev/user_doc/guide/task/sql.md | 43 -
docs/zh-cn/dev/user_doc/guide/task/sub-process.md | 47 -
docs/zh-cn/dev/user_doc/guide/task/switch.md | 37 -
docs/zh-cn/dev/user_doc/guide/upgrade.md | 82 -
docs/zh-cn/release/faq.md | 689 -----
docs/zh-cn/release/history-versions.md | 70 -
docsite.config.yml | 35 -
download/en-us/download.md | 73 -
download/en-us/download_ppt.md | 24 -
download/zh-cn/download.md | 73 -
download/zh-cn/download_ppt.md | 24 -
file/2019-10-26/DolphinScheduler_dailidong.pptx | Bin 4115300 -> 0 bytes
file/2019-10-26/DolphinScheduler_guowei.pptx | Bin 3418856 -> 0 bytes
file/2019-10-26/DolphinScheduler_qiaozhanwei.pptx | Bin 2400975 -> 0 bytes
file/2019-10-26/DolphinScheduler_zhangzongyao.pptx | Bin 15215166 -> 0 bytes
file/2019-10-26/Dolphinescheduler_baoqiwu.pptx | Bin 13195423 -> 0 bytes
file/2019-12-08/DolphinScheduler_liuxiaochun.pptx | Bin 1594353 -> 0 bytes
file/2019-12-08/DolphinScheduler_yangshuang.pptx | Bin 10712603 -> 0 bytes
file/2019-12-08/ShardingSphere_panjuan.pptx | Bin 1431633 -> 0 bytes
file/2019-12-08/ShardingSphere_zhangyonglun.pptx | Bin 3125688 -> 0 bytes
.../DolphinScheduler_Feature_Roadmap.pdf | Bin 9271719 -> 0 bytes
file/2020-07-25/DolphinScheduler_lijie.pptx | Bin 4740451 -> 0 bytes
file/2020-07-25/DolphinScheduler_qiaozhanwei.pptx | Bin 21892500 -> 0 bytes
file/2020-09-05/DolphinScheduler_baoliang.pptx | Bin 15383130 -> 0 bytes
file/2020-09-05/DolphinScheduler_liuhuijuan.pdf | Bin 3143900 -> 0 bytes
file/2020-10-24/DolphinScheduler&K8s_liwenhe.pptx | Bin 2604754 -> 0 bytes
.../Hudi&DolphinScheduler_yanghua&zhaoyuwei.pptx | Bin 8767843 -> 0 bytes
... - DolphinScheduler Introduction & Roadmap.pptx | Bin 10791288 -> 0 bytes
...hitecture breakthrough of DolphinScheduler.pptx | Bin 20701110 -> 0 bytes
...er architecture evolution - eBay ruanwenjun.key | Bin 21135612 -> 0 bytes
...er architecture evolution - eBay ruanwenjun.ppt | Bin 3794432 -> 0 bytes
file/DolphinScheduler-Introduction.pptx | Bin 16138535 -> 0 bytes
file/logo-template.pptx | Bin 47558 -> 0 bytes
googled0df7b96f277a143.html | 1 -
gulpfile.js | 123 -
package.json | 67 -
redirect.ejs | 40 -
site_config/blog.js | 385 ---
site_config/community.jsx | 450 ---
site_config/development.js | 133 -
site_config/docsdev.js | 623 ----
site_config/download.js | 39 -
site_config/home.jsx | 599 ----
site_config/site.js | 376 ---
sitemap.xml | 3148 --------------------
src/components/bar/index.jsx | 30 -
src/components/bar/index.scss | 59 -
src/components/button/index.jsx | 35 -
src/components/button/index.scss | 22 -
src/components/footer/index.jsx | 78 -
src/components/footer/index.scss | 210 --
src/components/header/index.jsx | 253 --
src/components/header/index.scss | 438 ---
src/components/language/index.jsx | 36 -
src/components/md2html/index.jsx | 96 -
src/components/md2html/index.scss | 35 -
src/components/mobileMenu/index.jsx | 109 -
src/components/pageSlider/index.jsx | 139 -
src/components/pageSlider/index.scss | 34 -
src/components/sidemenu/index.jsx | 57 -
src/components/sidemenu/index.scss | 148 -
src/components/sidemenu/item.jsx | 93 -
src/components/slider/index.jsx | 160 -
src/components/slider/index.scss | 30 -
src/markdown.scss | 1561 ----------
src/pages/blog/blogItem.jsx | 57 -
src/pages/blog/blogItem.scss | 57 -
src/pages/blog/index.jsx | 55 -
src/pages/blog/index.md.jsx | 35 -
src/pages/blog/index.md.scss | 20 -
src/pages/blog/index.scss | 55 -
src/pages/community/contactItem.jsx | 44 -
src/pages/community/contributorItem.jsx | 16 -
src/pages/community/ecoItem.jsx | 30 -
src/pages/community/eventCard.jsx | 25 -
src/pages/community/index.jsx | 48 -
src/pages/community/index.md.jsx | 40 -
src/pages/community/index.scss | 221 --
src/pages/development/index.md.jsx | 41 -
src/pages/docs/index.md.jsx | 107 -
src/pages/download/index.md.jsx | 41 -
src/pages/home/featureItem.jsx | 17 -
src/pages/home/index.jsx | 226 --
src/pages/home/index.scss | 628 ----
src/pages/user/index.jsx | 108 -
src/pages/user/user.scss | 167 --
src/reset.scss | 10 -
src/variables.scss | 29 -
template.ejs | 47 -
utils/index.js | 38 -
webpack.config.js | 67 -
1179 files changed, 6826 insertions(+), 51261 deletions(-)
diff --git a/.asf.yaml b/.asf.yaml
deleted file mode 100644
index eeccd05..0000000
--- a/.asf.yaml
+++ /dev/null
@@ -1,45 +0,0 @@
-# Licensed to the Apache Software Foundation (ASF) under one or more
-# contributor license agreements. See the NOTICE file distributed with
-# this work for additional information regarding copyright ownership.
-# The ASF licenses this file to You under the Apache License, Version 2.0
-# (the "License"); you may not use this file except in compliance with
-# the License. You may obtain a copy of the License at
-#
-# http://www.apache.org/licenses/LICENSE-2.0
-#
-# Unless required by applicable law or agreed to in writing, software
-# distributed under the License is distributed on an "AS IS" BASIS,
-# WITHOUT WARRANTIES OR CONDITIONS OF ANY KIND, either express or implied.
-# See the License for the specific language governing permissions and
-# limitations under the License.
-#
-
-# Web Site Deployment Service for Git Repositories
-staging:
- profile: ~
- whoami: master
- foo: trigger
-
-publish:
- whoami: asf-site
-
-# GitHub setting
-github:
- description: Apache DolphinScheduler website
- homepage: https://dolphinscheduler.apache.org/
- labels:
- - dolphinscheduler
- - website
- - apache
- enabled_merge_buttons:
- squash: true
- merge: false
- rebase: false
- protected_branches:
- master:
- required_status_checks:
- contexts:
- - CheckDeadLinks
- required_pull_request_reviews:
- dismiss_stale_reviews: true
- required_approving_review_count: 1
diff --git a/.babelrc b/.babelrc
deleted file mode 100644
index 42d28fa..0000000
--- a/.babelrc
+++ /dev/null
@@ -1,22 +0,0 @@
-{
- "plugins": [
- "transform-decorators-legacy",
- "transform-class-properties",
- "transform-object-rest-spread",
- "transform-object-assign",
- [
- "transform-runtime",
- {
- "helpers": false,
- "polyfill": false,
- "regenerator": true,
- "moduleName": "babel-runtime"
- }
- ]
- ],
- "presets": [
- "react",
- "stage-0",
- "es2015"
- ]
-}
diff --git a/.dlc.json b/.dlc.json
deleted file mode 100644
index 306ccd6..0000000
--- a/.dlc.json
+++ /dev/null
@@ -1,36 +0,0 @@
-{
- "ignorePatterns": [
- {
- "pattern": "^http://localhost"
- },
- {
- "pattern": "^https://hive.apache.org"
- },
- {
- "pattern": "^http://192"
- }
- ],
- "replacementPatterns": [
- {
- "pattern": "^/en-us/download/download.html$",
- "replacement": "https://dolphinscheduler.apache.org/en-us/download/download.html"
- },
- {
- "pattern": "^/zh-cn/download/download.html$",
- "replacement": "https://dolphinscheduler.apache.org/zh-cn/download/download.html"
- },
- {
- "pattern": "^/",
- "replacement": "{{BASEURL}}/"
- }
- ],
- "timeout": "10s",
- "retryOn429": true,
- "retryCount": 10,
- "fallbackRetryDelay": "1000s",
- "aliveStatusCodes": [
- 200,
- 401,
- 0
- ]
-}
diff --git a/.docsite b/.docsite
deleted file mode 100644
index e69de29..0000000
diff --git a/.eslintrc b/.eslintrc
deleted file mode 100644
index 0ed78ab..0000000
--- a/.eslintrc
+++ /dev/null
@@ -1,37 +0,0 @@
-{
- "extends": "eslint-config-ali/react",
- "parser": "babel-eslint",
- "env": {
- "browser": true,
- "node": true,
- "mocha": true
- },
- "globals": {
- "AK_GLOBAL": true,
- "dd": {},
- "_czc": {},
- "dplus": {},
- "salt": {},
- "_": true,
- "homePageData": {},
- "Lang": {}
- },
- "rules": {
- "linebreak-style": [0, "error", "windows"],
- "max-len": 0,
- "new-cap": 0,
- "no-param-reassign": 0,
- "no-prototype-builtins": 0,
- "prefer-const": 0,
- "prefer-destructuring": 0,
- "radix": ["error", "as-needed"],
- "react/jsx-no-bind": 0,
- "react/jsx-indent": 0,
- "react/jsx-indent-props": 0,
- "react/no-array-index-key": 0,
- "react/no-danger": 0,
- "react/no-did-mount-set-state": 0,
- "react/no-unescaped-entities": 0,
- "react/prop-types": 0
- }
-}
\ No newline at end of file
diff --git a/.gitignore b/.gitignore
index b463047..48e3d96 100644
--- a/.gitignore
+++ b/.gitignore
@@ -40,3 +40,33 @@ config.gypi
/dist/
/en-us/
/zh-cn/
+
+# Only for branch history-docs
+asset/
+blog/
+community/
+development/
+download/
+file/
+site_config/
+src/
+utils/
+site_config/
+.asf.yaml
+.babelrc
+.dlc.json
+.docsite
+.eslintrc
+.htaccess
+.nojekyll
+404.html
+docsite.config.yml
+googled0df7b96f277a143.html
+gulpfile.js
+index.html
+package.json
+package-lock.json
+redirect.ejs
+sitemap.xml
+template.ejs
+webpack.config.js
diff --git a/.htaccess b/.htaccess
deleted file mode 100644
index 3d90747..0000000
--- a/.htaccess
+++ /dev/null
@@ -1,23 +0,0 @@
-RedirectMatch 404 /\.htaccess
-
-<FilesMatch "\.html$">
- FileETag None
- Header unset ETag
- Header unset Pragma
- Header unset Cache-Control
- Header set Cache-Control "no-cache, no-store, must-revalidate"
- Header set Pragma "no-cache"
- Header set Expires "Thu, 1 Jan 1970 00:00:00 GMT"
-</FilesMatch>
-
-<IfModule mod_expires.c>
- ExpiresActive on
- ExpiresByType text/html "access plus 0 seconds"
- ExpiresByType image/png "access plus 1 day"
- ExpiresByType image/jpg "access plus 1 day"
- ExpiresByType image/jpeg "access plus 1 day"
- ExpiresByType text/css "access plus 1 day"
- ExpiresByType application/javascript "access plus 1 day"
- ExpiresByType application/json "access plus 1 day"
- ExpiresDefault "access plus 10 days"
-</IfModule>
\ No newline at end of file
diff --git a/.nojekyll b/.nojekyll
deleted file mode 100644
index e69de29..0000000
diff --git a/README.md b/README.md
index 7b3ad30..4343277 100644
--- a/README.md
+++ b/README.md
@@ -1,79 +1,4 @@
# Apache DolphinScheduler Official Website
-This project keeps all sources used for building up DolphinScheduler official website which is served at https://dolphinscheduler.apache.org/.
-
-## Prerequisite
-
-DolphinScheduler website is powered by [docsite](https://github.com/chengshiwen/docsite-ext).
-
-Please also make sure your node version is 10+, version lower than 10.x is not supported yet.
-
-## Build instruction
-
-1. Run `npm install` in the root directory to install the dependencies.
-2. Run `npm run start` in the root directory to start a local server, you will see the website in 'http://localhost:8080'.
-```
-Note: if you clone the code in Windows, not Mac. Please read the details below.
-If you execute the commands like the two steps above, you will get the exception "UnhandledPromiseRejectionWarning: Error: EPERM: operation not permitted, symlink '2.0.3' -> 'latest'".
-When meeting this problem. You can run two steps in the cmd.exe as an ADMINISTRATOR MEMBER.
-```
-3. Run `npm run build` to build source code into dist directory.
-4. Verify your change locally: `python -m SimpleHTTPServer 8000`, when your python version is 3 use :`python3 -m http.server 8000` instead.
-
-If you have higher version of node installed, you may consider `nvm` to allow different versions of `node` coexisting on your machine.
-
-1. Follow the [instructions](http://nvm.sh) to install nvm
-2. Run `nvm install v10.23.1` to install node v10
-3. Run `nvm use v10.23.1` to switch the working environment to node v10
-
-Then you are all set to run and build the website. Follow the build instruction above for the details.
-
-
-## How to send a PR
-
-1. Do not use `git add .` to commit all the changes.
-2. Just push your changed files, such as:
- * `*.md`
- * blog.js or docs.js or site.js
-3. Send a PR to **master** branch.
-
-## SEO
-
-Make sure each .md starts with the following texts:
-
-```
----
-title: title
-keywords: keywords1,keywords2, keywords3
-description: some description
----
-```
-
-
-## Guide for adding a new document
-
-### Add a new blog
-
-1. Add new .md file under blog/en-us or blog/zh-cn.
-2. Update site_config/blog.js, add a new entry to the blog in either en-us or zh-cn.
-3. Run docsite start locally to verify the blog can be displayed correctly.
-4. Send the pull request contains the .md and blog.js only.
-
-
-### Add a new article for development
-
-1. Add new .md file under docs/en-us/development or docs/zh-cn/development.
-2. Update site_config/development.js, add a new entry in either en-us or zh-cn.
-3. Run docsite start locally to verify the article can be displayed correctly.
-4. Send the pull request contains the *.md and development.js only.
-
-
-### Add a new article for docs
-
-1. Add new .md file under docs/en-us or docs/zh-cn.
-2. Update site_config/docs.js, add a new entry in either en-us or zh-cn.
-3. Run docsite start locally to verify the article can be displayed correctly.
-4. Send the pull request contains the *.md and docs.js only.
-
-Best Regards.
- Thanks for reading :)
+This branch is holding Apache DolphinScheduler history-docs, which include the version
+from 1.2.0 to 2.0.5
diff --git a/asset/dolphinscheduler-netutils.jar b/asset/dolphinscheduler-netutils.jar
deleted file mode 100644
index cc2fbfa..0000000
Binary files a/asset/dolphinscheduler-netutils.jar and /dev/null differ
diff --git a/blog/en-us/Apache-DolphinScheduler-2.0.1.md b/blog/en-us/Apache-DolphinScheduler-2.0.1.md
deleted file mode 100644
index 3cabb61..0000000
--- a/blog/en-us/Apache-DolphinScheduler-2.0.1.md
+++ /dev/null
@@ -1,274 +0,0 @@
----
-title: Apache DolphinScheduler 2.0.1 is here, and the highly anticipated one-click upgrade and plug-in finally come!
-keywords: Apache,DolphinScheduler,scheduler,big data,ETL,airflow,hadoop,orchestration, dataops,2.0.1
-description: Good news! Apache DolphinScheduler 2.0.1 version is officially released today!
----
-## Apache DolphinScheduler 2.0.1 is here, and the highly anticipated one-click upgrade and plug-in finally come!
-
-Good news! Apache DolphinScheduler 2.0.1 version is officially released today!
-
-In this version, DolphinScheduler has undergone a microkernel + plug-in architecture improvement, 70% of the code has
-been refactored, and the long-awaited plug-in function has also been emphatically optimized. In addition, there are many
-highlights in this upgrade, such as a one-click upgrade to the latest version, "de-ZK" in the registration center, and
-new task parameter transfer functions, etc..
-
-Download Apache DolphinScheduler 2.0.1:https://dolphinscheduler.apache.org/zh-cn/download/download.html
-
-The workflow execution process activities of Apache DolphinScheduler 2.0.1 are shown in the following figure:
-
-<div align=center>
-<img src="https://s1.imgpp.com/2021/12/20/master-process-2.0-en.png"/>
-</div>
-
-Start process activity diagram
-
-Version 2.0.1 enhanced the system's processing capabilities by optimizing the kernel, thereby greatly improving
-performance. The new UI interface also greatly improved the user experience. More importantly, there are two major
-changes in version 2.0.1: plug-in and refactoring.
-https://dolphinscheduler.apache.org/en-us/docs/latest/user_doc/guide/upgrade.html
-## 01 Plug-in
-
-Previously, some users had feedback that they hoped that Apache DolphinScheduler could be optimized for plug-inization.
-In respond, Apache DolphinScheduler 2.0.1 has optimized plug-in function, adding alarm plug-ins, registry plug-ins, and
-task plug-in management functions. With plug-in, users can meet their own functional needs more flexibly, customize
-development task components based on interfaces more simply, and seamlessly migrate user task components to a higher
-version of DolphinScheduler. DolphinScheduler is in the process of microkernel + plug-in architecture improvement. All
-core capabilities such as tasks, alarm components, data sources, resource storage, registry, etc. will be designed as
-extension points. We hope to improve the flexibility and friendliness of Apache DolphinScheduler itself through SPI. The
-related code can refer to the dolphinscheduler-spi module, and the extended interfaces of related plug-ins are also
-under this module. When users need to deploy the plug-in of related functions, it is recommended to read the code of
-this module first. Of course, it is also recommended that you read the document to save time. We have adopted an
-excellent front-end module form-create, which supports the generation of front-end UI components based on json. If
-plug-in development involves the front-end, we will use json to generate related front-end UI modules. The plug-in
-parameters are encapsulated in org.apache.dolphinscheduler.spi.params, which converts all relevant parameters into
-corresponding json. This means that you can completely draw front-end modules (mainly refers to forms) by Java.
-
-### 1 Alarm plug-in
-
-Taking the alert plug-in as an example, Apache DolphinScheduler 2.0.1 enables the loading of related plug-ins when the
-alert-server starts. Alert provides a variety of plug-in configuration methods and currently has built-in alert plug-ins
-such as Email, DingTalk, EnterpriseWeChat, and Script. When the plug-in module development work is completed, it can be
-enabled through a simple configuration.
-
-### 2 Multi-registry modules
-
-In Apache DolphinScheduler 1.X, the Zookeeper module plays a very important role , including monitoring and discovery of
-master/worker services, disconnection alarms, fault tolerance notification and so on. In version 2.0.1, we gradually "
-de-ZK" in the registry, weakening the role of Zookeeper, and adding plug-in management functions. In plug-in management,
-users can increase the support of registry centers such as ETCD, making Apache Dolphinscheduler more flexible and
-adaptable to more complex user needs.
-
-### 3 Task module plugin
-
-The new version also adds the task plug-in function, which enhances the isolation function of different task components.
-When a user develops a custom plug-in, he only needs to implement the plug-in interface. It mainly includes creating
-tasks (task initialization, task running, etc.) and task cancellation.
-
-If it is a Yarn task, you need to implement AbstractYarnTask. At present, developers need to use Vue to develop and
-deploy the front end of the task plug-in. In subsequent versions, we will implement the automatic drawing of front-end
-modules by Java.
-
-## 02 Refactor
-
-So far, Apache DolphinScheduler has refactored about 70% of the code and achieved a comprehensive upgrade.
-
-### 1 Master core optimization
-
-In the upgrade, we refactor the execution process of the Master, changing the previous state polling monitoring to an
-event notification mechanism, which greatly reduces the polling pressure of the database; removing the global lock,
-adding the fragmentation processing mechanism of the Master, and changing the sequence Read and write commands to
-parallel processing, which enhances the horizontal scalability of the Master; optimizes the workflow processing flow
-reduces the use of the thread pool and greatly increases the number of workflows processed by a single Master; adds the
-cache mechanism, optimizes the database connection method, and simplifies the processing process, reducing unnecessary
-time-consuming operations, etc.
-
-### 2 Workflow and task decoupling
-
-In Apache DolphinScheduler 1.x version, tasks and task relationships are saved in the workflow definition table in the
-form of large json. If a workflow is very large, (for example reaches 100 to 1000 tasks), the json will be too big to be
-parsed when in use. This process is more performance-consuming, and tasks cannot be reused; on the other hand, there is
-no good implementation solution in workflow version and task version for big json.
-
-Therefore, in the new version, we have decoupled the workflow and tasks, added a correlation chart between tasks and
-workflow, and added a log table to save the historical version of workflow definitions and task definition, which
-Improves the efficiency of workflow operation.
-
-The operation flow chart of the workflow and tasks under the API module are shown as below:
-
-<div align=center>
-<img src="https://s1.imgpp.com/2021/12/20/3.png"/>
-</div>
-
-## 03 Automatic Version Upgrade Function
-
-Automatic version upgrade finally comes true in version 2.0.1. The users can automatically upgrade Apache
-DolphinScheduler from version 1. x to version 2.0.1 by one line usage script, and you can use the new version to run the
-previous workflow without perception:
-
-```
-sh ./script/create-dolphinscheduler.sh
-```
-
-For specific upgrade documentation, please refer to:
-
-https://dolphinscheduler.apache.org/en-us/docs/latest/user_doc/guide/upgrade.html
-
-In addition, future versions of Apache DolphinScheduler can be automatically upgraded, saving the trouble of manual
-upgrades.
-
-## 04 List of New Features
-
-Details of the new features of Apache DolphinScheduler 2.0.1 are as follows:
-
-### 1 New Standalone service
-
-StandAlone Server is a service created to allow users to quickly experience the product. The registry and database
-H2-DataBase and Zk-TestServer are built-in. After modification, you can start StandAloneServer with one key to
-debugging.
-
-If you want a quick experience, after decompressing the installation package, you only need to configure the JDK
-environment to start the Apache DolphinScheduler system with one click, thereby reducing configuration costs and
-improving R&D efficiency.
-
-For detailed usage documentation, please refer to:
-
-https://dolphinscheduler.apache.org/en-us/docs/latest/user_doc/guide/installation/standalone.html
-
-Or use Docker to deploy all services with one
-click: https://dolphinscheduler.apache.org/en-us/docs/latest/user_doc/guide/installation/docker.html
-
-### 2 Task parameter transfer function
-
-Currently, the transfer between shell tasks and sql tasks is supported. Passing parameters between shell tasks:
-Set an out variable "trans" in the previous "create_parameter" task: echo'${setValue(trans=hello trans)}'
-
-<div align=center>
-<img src="https://s1.imgpp.com/2021/12/17/323f6a18d8a1d2f2d8fdcb5687c264b5.png"/>
-</div>
-Once Keyword: "${setValue(key=value)}" is detected in the task log of the current task, the system will automatically parse the variable transfer value, in the post-task, you can directly use the "trans" variable:
-
-<div align=center>
-<img src="https://s1.imgpp.com/2021/12/17/8be29339b73b594dc05a6b832d9330ec.png"/>
-</div>
-
-The parameter passing of the SQL task:
-The name of the custom variable prop of the SQL task needs to be consistent with the field name, and the variable will
-select the value corresponding to the column with the same variable name in the column name in the SQL query result. The
-output of user number:
-
-<div align=center>
-<img src="https://s1.imgpp.com/2021/12/17/85bc5216c01ca958cdf11d4bd555c8a6.png"/>
-</div>
-
-Use the variable "cnt" in downstream tasks:
-
-<div align=center>
-<img src="https://s1.imgpp.com/2021/12/17/4278d0b7f833b64f24fc3d6122287454.png"/>
-</div>
-
-2.0.1 adds switch task and pigeon task components:
-
-- switch task
-
-Setting the judgment condition in the switch task can realize the effect of running different conditional branches
-according to different conditional judgment results. For example, there are three tasks, the dependency is A -> B
--> [C, D], where task_a is the shell task and task_b is the switch task.
-
-In task A, a global variable named id is defined through a global variable, and the declaration method
-is `echo'${setValue(id=1)}' `.
-
-Task B adds conditions and uses the global variables declared upstream to achieve conditional judgment (global variables
-that exist when the Switch is running are just fine, which means that they can be global variables that are not directly
-generated upstream). Next, we set id as 1, run task C, and others run task D.
-
-Configure task C to run when the global variable id=1. Then edit ${id} == 1 in the condition of task B, and select C for
-branch circulation. For other tasks, select D in the branch circulation.
-
-
-<div align=center>
-<img src="https://s1.imgpp.com/2021/12/17/636f53ddc809f028ffdfc18fd08b5828.md.jpg"/>
-</div>
-
-
-
--pigeon task
-
-The pigeon task is a task component that can be docked with third-party systems. It can trigger task execution, cancel
-task execution, obtain task status, and obtain task logs. The pigeon task needs to configure the API address of the
-above task operation and the corresponding interface parameters in the configuration file. Enter a target task name in
-the task component to connect to the third-party system and can operate the task of the third-party system in Apache
-DolphinScheduler.
-
-### 3 Adds environmental management function
-
-The default environment configuration is dolphinscheduler_env.sh.
-
-Configure the worker running environment online. A worker can specify multiple environments, and each environment is
-equivalent to the dolphinscheduler_env.sh file.
-
-<div align=center>
-<img src="https://s1.imgpp.com/2021/12/17/ef8b444c6dbebe397daaaa3bbadf743f.png"/>
-</div>
-
-
-When creating a task, select the worker group and the corresponding environment variables. When the task is executed,
-the worker will execute the task in the corresponding execution environment.
-
-## 05 Optimization item
-
-### 1 Optimize the RestApi
-
-We have updated the new RestApi specification and re-optimized the API part by the specification, making it easier for
-users to use the API.
-
-### 2 Optimize the workflow version management
-
-We optimized the workflow version management function and increased the historical version of the workflow and tasks.
-
-### 3 Optimize worker group management function
-
-In version 2.0, the worker group management function is completed. Users can modify the group information of the worker
-through the page configuration, saving the troubåle to modify the configuration file on the server and restart the
-worker.
-
-After the optimization, each worker node will belong to its worker group, and be grouped to default by default. When the
-task is executed, the task can be assigned to the designated worker group, and finally run by the worker node in the
-group.
-
-There are two ways to modify the worker group:
-
-Open the "conf/worker.properties" configuration file on the worker node to be grouped, and modify the worker. groups
-parameter. The worker group to which the worker belongs can be modified during operation. If the modification is
-successful, the worker will use this newly created group, ignoring the configuration in worker. properties. Modify step
-by step: Security Center -> Worker Group Management -> Click'New Worker Group' -> Enter'Group Name' -> Select Existing
-Worker -> Click'Submit'.
-
-Other optimization issues:
-
-When starting the workflow, you can modify the startup parameters; Added workflow state automatically-launching when
-saving the workflow; Optimized the results returned by the API, and speeded up the page loading speed when creating a
-workflow; Speeded up the loading of workflow instance pages; Optimized the display information of the workflow
-relationship page; Optimized the import and export function, supporting cross-system import and export workflow;
-Optimized some API operations, such as adding several interface methods, task deletion check, etc.
-
-## 06 Changelogs
-
-In addition, Apache DolphinScheduler 2.0.1 also fixes some bugs, including:
-
-Fixed the problem that netty client would create multiple pipes; Fixed the problem of importing workflow definition
-errors; Fixed the problem that the task code would be obtained repeatedly; Fix the problem that the Hive data source
-connection fails when Kerberos is used; Fix the problem that the Standalone service fails to start; Fix the problem that
-the alarm group display failure; Fix the problem of abnormal file upload; Fix the problem that the Switch task fails to
-run; Fix the problem of invalid workflow timeout strategy; Fix the problem that the SQL task cannot send mail.
-
-## 07 Acknowledgements
-
-Thanks to the 289 community contributors who participated in the optimization and improvement of version 2.0.1 (in no
-particular order)!
-
-<div align=center>
-<img src="https://s1.imgpp.com/2021/12/17/2020b4f57e33734414a11149704ded92.png"/>
-</div>
-<div align=center>
-<img src="https://s1.imgpp.com/2021/12/17/1825b6945d5845233b7389479ba6c074.png"/>
-</div>
diff --git a/blog/en-us/Apache_dolphinScheduler_2.0.2.md b/blog/en-us/Apache_dolphinScheduler_2.0.2.md
deleted file mode 100644
index 71b1947..0000000
--- a/blog/en-us/Apache_dolphinScheduler_2.0.2.md
+++ /dev/null
@@ -1,122 +0,0 @@
----
-title: Apache DolphinScheduler 2.0.2 Release Announcement:WorkflowAsCode is Launched!
-keywords: Apache,DolphinScheduler,scheduler,big data,ETL,airflow,hadoop,orchestration,dataops,2.0.2
-description:In the long-awaited, WorkflowAsCode function is finally launched in version 2.0.2 as promised, bringing good news to users who need to dynamically create and update workflows in batches.
----
-# Apache DolphinScheduler 2.0.2 Release Announcement:WorkflowAsCode is Launched!
-
-<div align=center>
-<img src="/img/2022-1-13/1_3XcwBeN5HkBzZ76zXDcigw.jpeg"/>
-</div>
-
-In the long-awaited, WorkflowAsCode function is finally launched in version 2.0.2 as promised, bringing good news to users who need to dynamically create and update workflows in batches.
-
-In addition, the new version also adds the WeCom alarm group chat message push, simplifies the metadata initialization process, and fixes issues that existed in the former version, such as failure of service restart after forced termination, and the failure to add a Hive data source.
-
-## New Function
-
-### WorkflowAsCode
-
-First of all, in terms of new functions, version 2.0.2 released PythonGatewayServer, which is a Workflow-as-code server started in the same way as apiServer and other services.
-
-When PythonGatewayServer is enabled, all Python API requests are sent to PythonGatewayServer. Workflow-as-code lets users create workflows through the Python API, which is great news for users who need to create and update workflows dynamically and in batches. Workflows created with Workflow-as-code can be viewed in the web UI just like other workflows.
-
-The following is a Workflow-as-code test case:
-
-```py
-# Define workflow properties, including name, scheduling period, start time, tenant, etc.
-
-with ProcessDefinition(
- name="tutorial",
- schedule="0 0 0 * * ? *",
- start_time="2021-01-01",
- tenant="tenant_exists",
-) as pd:
- # Define 4 tasks, which are all shell tasks, the required parameters of shell tasks are task name, command information, here are all the shell commands of echo
-
- task_parent = Shell(name="task_parent", command="echo hello pydolphinscheduler")
- task_child_one = Shell(name="task_child_one", command="echo 'child one'")
- task_child_two = Shell(name="task_child_two", command="echo 'child two'")
- task_union = Shell(name="task_union", command="echo union")
-
- # Define dependencies between tasks
- # Here, task_child_one and task_child_two are first declared as a task group through python's list
- task_group = [task_child_one, task_child_two]
- # Use the set_downstream method to declare the task group task_group as the downstream of task_parent, and declare the upstream through set_upstream
- task_parent.set_downstream(task_group)
-
- # Use the bit operator << to declare the task_union as the downstream of the task_group, and support declaration through the bit operator >>
- task_union << task_group
-
-```
-When the above code runs, you can see workflow in the web UI as follows:
-
-``` --> task_child_one
- / \
-task_parent --> --> task_union
- \ /
- --> task_child_two
-```
-
-
-### 2 Wecom alarm mode supports group chat message push
-
-In the previous version, the WeChat alarm only supported the message notification; in version 2.0.2, when the user uses the Wecom alarm, it supports pushing the group chat message in the app to the user.
-
-## 02 Optimization
-
-### 1 Simplified metadata initialization process
-
-When Apache DolphinScheduler is first installed, running create-dolphinscheduler.sh requires a step by step upgrade from the oldest version to the current version. In order to initialize the metadata process more conveniently and quickly, version 2.0.2 allows users to directly install the current version of the database script, which improves the installation speed.
-
-### 2 Remove "+1" (days) in complement dates
-
-Removed the "+1" day in the complement date to avoid user confusion when the UI date always displays +1 when the complement is added.
-
-## 03 Bug Fixes
-
-[#7661] fix logger memory leak in worker
-[#7750] Compatible with historical version data source connection information
-[#7705] Memory constraints cause errors when upgrading from 1.3.5 to 2.0.2
-[#7786] Service restart fails after a forced termination
-[#7660] Process definition version create time is wrong
-[#7607] Failed to execute PROCEDURE node
-[#7639] Add default configuration of quartz and zookeeper in common configuration items
-[#7654] In the dependency node, an error is reported when there is an option that does not belong to the current project
-[#7658] Workflow replication error
-[#7609] Workflow is always running when worker sendResult succeeds but the master does not receive error report
-[#7554] H2 in Standalone Server will automatically restart after a few minutes, resulting in abnormal data loss
-[#7434] Error reported when executing MySQL table creation statement
-[#7537] Dependent node retry delay does not work
-[#7392] Failed to add a Hive data source
-
-Download: https://dolphinscheduler.apache.org/zh-cn/download/download.html
-Release Note: https://github.com/apache/dolphinscheduler/releases/tag/2.0.2
-
-
-## 04 Thanks
-
-
-As always, we would like to thank all the contributors (in no particular order) who have worked to polish Apache DolphinScheduler 2.0.2 as a better platform. It is your wisdom and efforts to make it more in line with the needs of users.
-
-<div align=center>
-<img src="/img/2022-1-13/1_IFBxUh2I0LFWF3Jkwz1e5g.png"/>
-</div>
-
-## The Way to Join US
-
-There are many ways to participate and contribute to the DolphinScheduler community, including:
-Documents, translation, Q&A, tests, codes, articles, keynote speeches, etc.
-
-We assume the first PR (document, code) to contribute to be simple and should be used to familiarize yourself with the submission process and community collaboration style.
-
-So the community has compiled the following list of issues suitable for novices: https://github.com/apache/dolphinscheduler/issues/5689
-List of non-newbie issues: https://github.com/apache/dolphinscheduler/issues?q=is%3Aopen+is%3Aissue+label%3A%22volunteer+wanted%22
-How to participate in the contribution: https://dolphinscheduler.apache.org/en-us/community/development/contribute.html
-
-Community Official Website:
-https://dolphinscheduler.apache.org/
-GitHub Code repository:
-https://github.com/apache/dolphinscheduler
-Your Star for the project is important, don’t hesitate to lighten a Star for Apache DolphinScheduler ❤️
-
diff --git a/blog/en-us/Apache_dolphinScheduler_2.0.3.md b/blog/en-us/Apache_dolphinScheduler_2.0.3.md
deleted file mode 100644
index 1b99b5a..0000000
--- a/blog/en-us/Apache_dolphinScheduler_2.0.3.md
+++ /dev/null
@@ -1,146 +0,0 @@
----
-title:Apache DolphinScheduler 2.0.3 Release Announcement: DingTalk alert plugin adds signature verification, and supports data sources to obtain links from multiple sessions
-keywords: Apache,DolphinScheduler,scheduler,big data,ETL,airflow,hadoop,orchestration,dataops,2.0.3
-description:Today, Apache DolphinScheduler announced the official release of version 2.0.3. In this version,
----
-# Apache DolphinScheduler 2.0.3 Release Announcement: DingTalk alert plugin adds signature verification, and supports data sources to obtain links from multiple sessions
-
-<div align=center>
-<img src="/img/2.0.3/2022-1-2701/1.png"/>
-</div>
-
-> Today, Apache DolphinScheduler announced the official release of version 2.0.3. In this version, DingTalk alert plugin adds signature verification and enables data sources to get links from multiple sessions. In addition, 2.0.3 also optimizes cache management, complement time, data source password display in logs, etc., and fixes several Bug.
-
-
-
-
-## Function Enhancement
-
-
-### DingTalk alert plugin adds signature verification
-
-
-2.0.3 Supports DingTalk robot alarm function through signature verification.
-
-<div align=center>
-<img src="/img/2.0.3/2022-1-2701/2.png"/>
-</div>
-
-**DingTalk parameter configuration**
-
-- Webhooks
-
-The format is as follows: https://oapi.dingtalk.com/robot/send?access_token=XXXXXX
-
-- Keyword
-
-Custom keywords for security settings
-
-- Secret
-
-Signature of security settings
-
-
-When a custom bot sends a message, you can specify the "@person list" by your mobile phone number. When a person in the "@people list" receives the message, there will be an @message reminder. Even set to Do Not Disturb mode, there will still be notification reminders for conversations, and the prompt "Someone @ you" will appear on the first screen.
-
-- @Mobiles
-
-The phone number of the person being @
-
-- @UserIds
-
-User userid of @person
-
-- @All
-
-@everyone
-
-
-
-
-For details, please refer to: https://open.dingtalk.com/document/robots/customize-robot-security-settings
-
-
-
-
-### Supports data source to get connection from multi sessions
-
-
-
-
-Previously, we use the JdbcDataSourceProvider.createOneSessionJdbcDataSource() method to create a connection pool in hive/impala set MaximumPoolSize=1, while in scheduling tasks, if hive/impala multitasking runs at the same time, getConnection=null will occur, and the SqlTask.prepareStatementAndBind() method will throw a null pointer exception.
-
-2.0.3 is optimized to support data sources getting links from multiple sessions.
-
-
-## Improvements
-
-
-
-
-### Introduces cache manager, reduce DB query in Master scheduling
-
-
-
-
-Since numerous database read operations, such as tenant, user, processDefinition, etc., will occur during the scheduling process of the master server, it will bring enormous pressure on the DB on the one hand, and will slow down the entire core scheduling process on the other hand.
-
-Considering that this part of business data involves more reading than writing, 2.0.3 introduces a cache module, which mainly acts on the Master node to cache business data such as tenants and workflow definitions, thus reduces database query pressure, and speeds up the core scheduling process. Please check the official website documentation for details: https://dolphinscheduler.apache.org/en-us/docs/latest/user_doc/architecture/cache.html
-
-
-
-
-### Optimize complement task's date, the complement time interval from '[left, right)' to '[left, right]'
-
-
-
-
-Previously, the complement time was "left closed and right open" (startDate <= N < endDate), which is actually not conducive to user understanding. After optimization, the deployment time interval is changed to "closed left and closed right".
-
-Complement case: https://dolphinscheduler.apache.org/en-us/docs/latest/user_doc/guide/project/workflow-definition.html
-
-
-### Encrypted data source password in the logs
-
-
-
-The password in the data source is encrypted to enhance privacy protection.
-
-## Bug Fixes
-
-
-* zkRoot in conf/config/install_ config does not take effect
-* Problems caused by modifying the administrator user information.
-* Add delete workflow instance when delete process definition
-* udf sub resource manage edit modal cant close
-* process is always running: netty writeAndFlush without retry when failed, leads to worker response to master failed
-* After deleting the running workflow, the master keeps brushing the error log
-* Edit the bug of worker grouping in environment management.
-* Dependent node ui dislocation
-* Error in querying historical version information of workflow
-* Solve the problem that task log output affects performance under high concurrency
-* The global parameters of the sub_process node are not passed to the associated workflow task
-* Query log can not show contents when task log in master on k8s
-* Duplicate processes in the process definition list
-* Process instance is always running: task is failure when process instance FailureStrategy.END
-* Field ‘is_directory’ in t_ds_resources table has error type in PostgreSQL database
-* Repair JDBC connection of Oracle
-* When there is a forbidden node in dag, the execution flow is abnormal
-* QuerySimpleList return wrong projectCode
-
-
-
-
-**Release Note:** https://github.com/apache/dolphinscheduler/releases/tag/2.0.3
-
-**Download:** https://dolphinscheduler.apache.org/en-us/download/download.html
-
-
-## Thanks to contributors
-
-Thanks to the community contributors for their active contributions to this release! This is the list of Contributors, in no particular order:
-<div align=center>
-<img src="/img/2.0.3/2022-1-2701/3.png"/>
-</div>
-
-
diff --git a/blog/en-us/Apache_dolphinScheduler_2.0.5.md b/blog/en-us/Apache_dolphinScheduler_2.0.5.md
deleted file mode 100644
index 0d3e0e5..0000000
--- a/blog/en-us/Apache_dolphinScheduler_2.0.5.md
+++ /dev/null
@@ -1,70 +0,0 @@
----
-title:Release News! Apache DolphinScheduler 2_0_5 optimizes The Fault Tolerance Process of Worker
-keywords: Apache,DolphinScheduler,scheduler,big data,ETL,airflow,hadoop,orchestration,dataops,Meetup
-description: Today, Apache DolphinScheduler announced the official release of version 2.0.5.
----
-<div align=center>
-<img src="/img/2022-3-7/1.png"/>
-</div>
-
-Today, Apache DolphinScheduler announced the official release of version 2.0.5. This version has carried out some functional optimizations, such as optimizing the fault tolerance process of Worker, adding the function of re-uploading files in the resource center, and making several bug fixes.
-
-## Optimization
-
-### Worker fault tolerance process
-
-Version 2.0.5 optimizes the worker's fault tolerance process so that when the server is interrupted due to excessive pressure, it can normally transfer tasks to other workers to continue execution to avoid task interruption.
-
-### Forbid to run task page sign optimization
-
-Optimized the display of flags on pages where tasks are prohibited from running, which distinguishes from the display of tasks that are normally executed, to prevent users from confusing work status.
-
-<div align=center>
-<img src="/img/2022-3-7/2.png"/>
-</div>
-
-### Added prompts to the task box
-In version 2.0.5, a prompt is added to the task box to display all the long task names, which is convenient for users.
-
-<div align=center>
-<img src="/img/2022-3-7/3.png"/>
-</div>
-
-
-### Added the re-uploading files function in the resource center
-
-The function of re-uploading files has been added to the resource center. When the user needs to modify the execution, automatical updating of the execution script can be realized without the requirement to reconfigure the task parameters.
-
-### Jump to the list page directly when modifying the workflow
-
-Changed the status that the page remained on the DAG page after modifying the workflow. After optimization, it can jump to the list page, which is convenient for users to follow-up operations.
-
-### Markdown information type added to DingTalk alert plugin
-
-Adds the Markdown information type to the alarm content of the DingTalk alarm plugin to enrich the information type support.
-
-## Bug Fix
-
-[[#8213](https://github.com/apache/dolphinscheduler/issues/8213)] The task run incorrectly when the worker group contains uppercase letters.
-
-[[#8347](https://github.com/apache/dolphinscheduler/pull/8347)] Fixed the problem of workflow cannot be stopped when the task fails and retries
-
-[[#8135](https://github.com/apache/dolphinscheduler/issues/8135)] JDBC connection parameter cannot input '@'
-
-[[#8367](https://github.com/apache/dolphinscheduler/issues/8367)] Fixed complement may not end normally
-
-[[#8170](https://github.com/apache/dolphinscheduler/issues/8170)] Fix the problem of failing to enter the sub-workflow from the page
-
-2.0.5 Download address:
-
-[https://dolphinscheduler.apache.org/en-us/download/download.html](https://dolphinscheduler.apache.org/en-us/download/download.html)
-
-Release Note: [https://github.com/apache/dolphinscheduler/releases/tag/2.0.5](https://github.com/apache/dolphinscheduler/releases/tag/2.0.5)
-
-## Thanks to Contributors
-
-Thanks to the contributors of Apache DolphinScheduler 2.0.5 version, the list of contributor GitHub IDs is as follows (in no particular order):
-
-<div align=center>
-<img src="/img/2022-3-7/4.png"/>
-</div>
diff --git a/blog/en-us/Awarded_most_popular_project_in_2021.md b/blog/en-us/Awarded_most_popular_project_in_2021.md
deleted file mode 100644
index 033fae7..0000000
--- a/blog/en-us/Awarded_most_popular_project_in_2021.md
+++ /dev/null
@@ -1,77 +0,0 @@
----
-title: Apache DolphinScheduler Won the「2021 OSC Most Popular Projects」award, and Whaleops Open Source Technology Received the honor of「Outstanding Chinese Open Source Original Startups」!
-keywords: Apache,DolphinScheduler,scheduler,big data,ETL,airflow,hadoop,orchestration,dataops,2021
-description: Recently, the "2021 OSC Best China Open Source Projects Poll」initiated by OSCHINA announced the selection results.
----
-
-# Apache DolphinScheduler Won the「2021 OSC Most Popular Projects」award, and Whaleops Open Source Technology Received the honor of「Outstanding Chinese Open Source Original Startups」!
-
-<div align=center>
-<img src="https://s1.imgpp.com/2022/01/07/_1ca0eca926145ffc5f05f15b6b612a2b_64635.jpg"/>
-</div>
-
-Recently, the "2021 OSC Best China Open Source Projects Poll」initiated by OSCHINA announced the selection results.
-
-With the love and support of the users and the open-source community, the cloud-native distributed big data scheduler Apache DolphinScheduler was awarded the 「OSCHINA Popularity Index Top 50 Open Source Projects」and 「Most Popular Projects」in 2021. And Its commercial operating company Whaleops Open Source Technology also was awarded the "Outstanding Chinese Open Source Native Startup" for its outstanding open-source operation performance.
-
-## Won the Honor of「Most Popular Projects」
-
-This year, the 「2021 OSC Best China Open Source Projects Poll" set up two rounds of voting. The first round of voting selected the 「OSCHINA Popularity Index TOP 50 Open Source Projects」based on the number of votes. The second round of voting was conducted based on the TOP 50 projects in the first round, and 30 "Most Popular Projects" were selected.
-
-In the first round of voting, OSCHINA selected TOP 5 of 7 major categories of projects (basic Software, Cloud-Native, Front-end, DevOps, Development Frameworks and Tools, AI & Big Data, IoT & 5G) based on the number of votes. Apache DolphinScheduler stood out in the "cloud-native" category and was on the list for its excellent cloud-native capabilities.
-
-After the fierce competition in the second round of voting, Apache DolphinScheduler won again and was rewarded the "Most Popular Project".
-
-## Whaleops Open Source Technology Won the 「Outstanding Chinese Open Source Native Startups」 Award
-
-"OSC China Open Source Project Poll" organized by Open Source China (OSCHINA, OSC Open Source Community) is the most authoritative and grandest open source project campaign in China. It aims to showcase the status quo of domestic open-source, explore domestic open source trends, and inspire domestic open-source talents, sequentially to promote the improvement of the domestic open source ecosystem.
-
-China's open-source software ecosystem is booming. In recent years, numerous outstanding open source software startups have emerged. They have beared original aspirations in mind to contribute to China's open-source software development, deeply cultivated in open-source to gave back to the community, and became an important force that cannot be ignored in the global open-source software ecosystem.
-
-To appreciate these open source startups for their outstanding contributions to the open-source community, and to let more developers know and understand these high-quality open-source startup teams that have set a good example for Chinese open-source entrepreneurs, OSCHINA specially set up the "Excellent Chinese Open Source Native Startups" award in this year’s poll.
-
-"Open Source Native Startups" refer to companies established based on open source projects and operate around the following business models:
-
-The team provides commercial services around self-built open source projects, or
-The team provides commercial services based on upstream open source projects
-
-OSCHINA has evaluated open-source native commercial companies in their entrepreneurial stage from the perspective of the company's related open source project communities status, the technical field development prospects of the company's related open source projects, and the company's development status. After professional evaluation, Whaleops Open Source Technology was rewaded the 「Excellent Chinese Open Source Native Startups」award.
-
-
-## About Apache DolphinScheduler
-
-
-Apache DolphinScheduler is a top-level project incubated by the Apache Software Foundation. As a new generation of distributed big data workflow task scheduler, it is committed to "solving the intricate dependencies between big data tasks and making the entire data process intuitive and visible". DolphinScheduler assembles Tasks in the form of DAG (Directed Acyclic Graph), which can monitor the running status of tasks in real-time. At the same time, it supports operations such as retry, [...]
-
-
-
-
-So far, the Apache DolphinScheduler community consists of 280+ experienced code contributors and 110+ non-code contributors, including PMC or Committer from other top Apache projects. Since its creation, the Apache DolphinScheduler open source community has continued to grow, and the WeChat user base has reached 6000+ people. As of January 2022, 600+ companies and institutions have adopted Apache DolphinScheduler in their production environments.
-
-Finally, we would like to thank OSCHINA for recognizing Apache DolphinScheduler and its commercial operating company Whaleops Open Source Technology, especially to every participant that is inseparable in the community build-up.
-
-
-
-
-In the future, we believe the community will scale new heights with this valuable honor, as well as the joint force of every contributor!
-
-
-## The Way to Join US
-
-There are many ways to participate and contribute to the DolphinScheduler community, including:
-Documents, translation, Q&A, tests, codes, articles, keynote speeches, etc.
-
-We assume the first PR (document, code) to contribute to be simple and should be used to familiarize yourself with the submission process and community collaboration style.
-
-- So the community has compiled the following list of issues suitable for novices: https://github.com/apache/dolphinscheduler/issues/5689
-
-- List of non-newbie issues: https://github.com/apache/dolphinscheduler/issues?q=is%3Aopen+is%3Aissue+label%3A%22volunteer+wanted%22
-- How to participate in the contribution: https://dolphinscheduler.apache.org/en-us/community/development/contribute.html
-
-- Community Official Website
-https://dolphinscheduler.apache.org/
-- GitHub Code repository: https://github.com/apache/dolphinscheduler
-
-Your Star for the project is important, don’t hesitate to lighten a Star for [Apache DolphinScheduler](https://github.com/apache/dolphinscheduler) ❤️
-
-
diff --git a/blog/en-us/Board_of_Directors_Report.md b/blog/en-us/Board_of_Directors_Report.md
deleted file mode 100644
index f0c05ef..0000000
--- a/blog/en-us/Board_of_Directors_Report.md
+++ /dev/null
@@ -1,99 +0,0 @@
----
-title:Apache DolphinScheduler Board Report: Community Runs Well, Commit Number Grows over 123%
-keywords: Apache,DolphinScheduler,scheduler,big data,ETL,airflow,hadoop,orchestration,dataops,Board Report
-description:ince graduating from the Apache Incubator on March 17, 2021
----
-# Apache DolphinScheduler Board Report: Community Runs Well, Commit Number Grows over 123%
-
-<div align=center>
-<img src="/img/2022-1-13/640.png"/>
-</div>
-
-Since graduating from the Apache Incubator on March 17, 2021, Apache DolphinScheduler has grown with the community for ten months. With the joint participation of the community, Apache DolphinScheduler has grown into a mature scheduling system product that has been tested in the production environment of hundreds of enterprises after several iterations.
-
-What progress has Apache DolphinScheduler made in nearly a year? Today we're going to review the changes that have taken place in the Apache DolphinScheduler and its community with this Apache report.
-
-
-## Base Data:
-
-Founded: 2021-03-17 (10 months ago)
-Chair: Lidong Dai
-Reporting schedule: January, April, July, October
-Next report date: Wed Jan 19 2022
-Community Health Score (Chi): 7.55 (Healthy)
-
-
-## Project Composition:
-* There are currently 39 committers and 16 PMC members in this project.
-* The Committer-to-PMC ratio is roughly 5:2.
-
-## Community changes, past quarter:
-* No new PMC members. Last addition was Calvin Kirs on 2021-05-07.
-* ShunFeng Cai was added as committer on 2021-12-18
-* Zhenxu Ke was added as committer on 2021-12-12
-* Wang Xingjie was added as committer on 2021-11-24
-* Yizhi Wang was added as committer on 2021-12-15
-* Jiajie Zhong was added as committer on 2021-12-12
-
-
-## Community Health Metrics:
-* Notable mailing list trends
-* Commit activity
-* GitHub PR activity
-* GitHub issues
-* Busiest GitHub issues/PRs
-## Notable mailing list trends:
-dev@dolphinscheduler.apache.org had a 64% increase in traffic in the past quarter (297 emails compared to 181):
-
-<div align=center>
-<img src="/img/2022-1-13/640-1.png"/>
-</div>
-
-## Commit activity:
-* 972 commits in the past quarter (123% increase)
-* 88 code contributors in the past quarter (25% increase)
-<div align=center>
-<img src="/img/2022-1-13/640-2.png"/>
-</div>
-
-## GitHub PR activity:
-* 824 PRs opened on GitHub, past quarter (89% increase)
-* 818 PRs closed on GitHub, past quarter (100% increase)
-<div align=center>
-<img src="/img/2022-1-13/640-3.png"/>
-</div>
-
-## GitHub issues:
-* 593 issues opened on GitHub, past quarter (90% increase)
-* 608 issues closed on GitHub, past quarter (155% increase)
-
-<div align=center>
-<img src="/img/2022-1-13/640-4.png"/>
-</div>
-
-## Busiest GitHub issues/PRs:
-* dolphinscheduler/pull/6894[Improvement][Logger]Logger server integrate into worker server(15 comments)
-* dolphinscheduler/pull/6744[Bug][SnowFlakeUtils] fix snowFlake bug(15 comments)
-* dolphinscheduler/pull/6674[Feature][unittest] Recover UT in AlertPluginManagerTest.java [closes: #6619](15 comments)
-* dolphinscheduler/issues/7039[Bug] [Task Plugin] hive sql execute failed(14 comments)
-* dolphinscheduler/pull/6782[improvement] improve install.sh if then statement(13 comments)
-* dolphinscheduler/issues/7485[Bug] [dolphinscheduler-datasource-api] Failed to create hive datasource using ZooKeeper way in 2.0.1(13 comments)
-* dolphinscheduler/pull/7214[DS-7016][feat] Auto create workflow while import sql script with specific hint(12 comments)
-* dolphinscheduler/pull/6708[FIX-#6505][Dao] upgrade the MySQL driver package for building MySQL jdbcUrl(12 comments)
-* dolphinscheduler/pull/7515[6696/1880][UI] replace node-sass with dart-sass(12 comments)
-* dolphinscheduler/pull/6913Use docker.scarf.sh to track docker user info(12 comments)
-
-## The Way to Join US
-
-There are many ways to participate and contribute to the DolphinScheduler community, including:
-Documents, translation, Q&A, tests, codes, articles, keynote speeches, etc.
-
-We assume the first PR (document, code) to contribute to be simple and should be used to familiarize yourself with the submission process and community collaboration style.
-
-So the community has compiled the following list of issues suitable for novices: https://github.com/apache/dolphinscheduler/issues/5689
-List of non-newbie issues: https://github.com/apache/dolphinscheduler/issues?q=is%3Aopen+is%3Aissue+label%3A%22volunteer+wanted%22
-How to participate in the contribution: https://dolphinscheduler.apache.org/en-us/community/development/contribute.html
-Community Official Website
-https://dolphinscheduler.apache.org/
-GitHub Code repository: https://github.com/apache/dolphinscheduler
-Your Star for the project is important, don’t hesitate to lighten a Star for Apache DolphinScheduler ❤️
\ No newline at end of file
diff --git a/blog/en-us/DAG.md b/blog/en-us/DAG.md
deleted file mode 100644
index 3a98168..0000000
--- a/blog/en-us/DAG.md
+++ /dev/null
@@ -1,166 +0,0 @@
-## Big Data Workflow Task Scheduling - Directed Acyclic Graph (DAG) for Topological Sorting
-
-### Reviewing the basics:
-
-#### Graph traversal:
-
-A graph traversal is a visit to all the vertices in a graph once and only once, starting from a vertex in the graph and following some search method along the edges of the graph.
-
-Note : the tree is a special kind of graph, so tree traversal can actually be seen as a special kind of graph traversal.
-
-#### There are two main algorithms for graph traversal
-
-##### Breadth First Search (BFS)
-
-The basic idea: first visit the starting vertex v, then from v, visit each of v's unvisited adjacent vertices w1, w2 , ... ,wi, then visit all the unvisited adjacent vertices of w1, w2, ... , wi in turn; from these visited vertices, visit all their unvisited adjacent vertices until all vertices in the graph have been visited. and from these visited vertices, visit all their unvisited adjacent vertices, until all vertices in the graph have been visited. If there are still vertices in the [...]
-
-##### Depth First Search (DFS)
-
-The basic idea: first visit a starting vertex v in the graph, then from v, visit any vertex w~1~ that is adjacent to v and has not been visited, then visit any vertex w~2~ that is adjacent to w~1~ and has not been visited ...... Repeat the above process. When it is no longer possible to go down the graph, go back to the most recently visited vertex, and if it has adjacent vertices that have not been visited, continue the search process from that point until all vertices in the graph have [...]
-
-#### Example
-
-In the diagram below, if the breadth first search (BFS) is used, the traversal is as follows: `1 2 5 3 4 6 7`. If the depth first search (DFS) is used, the traversal is as follows `1 2 3 4 5 6 7`.
-
-
-
-### Topological Sorting
-
-The definition of topological ordering on Wikipedia is :
-
-For any Directed Acyclic Graph (DAG), the topological sorting is a linear sorting of all its nodes (there may be multiple such node sorts in the same directed graph). This sorting satisfies the condition that for any two nodes U and V in the graph, if there is a directed edge pointing from U to V, then U must appear ahead of V in the topological sorting.
-
-**In layman's terms: Topological sorting is a linear sequence of all vertices of a directed acyclic graph (DAG), which must satisfy two conditions :**
-
-- Each vertex appears and only appears once.
-- If there is a path from vertex A to vertex B, then vertex A appears before vertex B in the sequence.
-
-**How to find out its topological sort? Here is a more commonly used method :**
-
-Before introducing this method, it is necessary to add the concepts of indegree and outdegree of a directed graph node.
-
-Assuming that there is no directed edge whose starting point and ending point are the same node in a directed graph, then:
-
-In-degree: Assume that there is a node V in the graph, and the in-degree is the number of edges that start from other nodes and end at V. That is, the number of all directed edges pointing to V.
-
-Out-degree: Assuming that there is a node V in the directed graph, the out-degree is the number of edges that currently have a starting point of V and point to other nodes. That is, the number of edges issued by V.
-
-1. Select a vertex with an in-degree of 0 from the DAG graph and output it.
-2. Delete the vertex and all directed edges starting with it from the graph.
-3. Repeat 1 and 2 until the current DAG graph is empty or there are no vertices of degree 0 in the current graph. The latter case indicates that a ring must exist in the directed graph.
-
-**For example, the following DAG graph :**
-
-
-
-
-|Node|in degree|out degree|count of in degree|count of out degree|
-|----|----|----|----|----|
-|Node 1|0|Node 2,Node 4|0|2|
-|Node 2|Node 1|Node 3,Node 4|1|2|
-|Node 3|Node 2,Node 4|Node 5|2|1|
-|Node 4|Node 1,Node 2|Node 3,Node 5|2|2|
-|Node 5|Node 3,Node 4|0|2|0|
-
-**Its topological sorting process is:**
-
-
-
-Therefore, the result of topological sorting is: {1,2,4,3,5}.
-
-If there is no node 2 —> the arrow of node 4, then it is as follows:
-
-
-
-We can get its topological sort as: {1,2,4,3,5} or {1,4,2,3,5}, that is, for the same DAG graph, there may be multiple topological sort results .
-
-Topological sorting is mainly used to solve the dependency problem in directed graphs.
-
-**When talking about the implementation, it is necessary to insert the following : **
-
-From this we can further derive an improved depth first search or breadth first search algorithm to accomplish topological sorting. Taking the breadth first search as an example, the only difference between this improved algorithm and the ordinary breadth first search is that we should save the degree of entry corresponding to each node and select the node with a degree of entry of 0 at each level of the traversal to start the traversal (whereas the ordinary breadth first search has no s [...]
-
-1. Initialize a Map or similar data structure to save the in-degree of each node.
-2. For the child nodes of each node in the graph, add 1 to the in-degree of its child nodes.
-3. Select any node with an in-degree of 0 to start traversal, and add this node to the output.
-4. For each node traversed, update the in-degree of its child node: subtract 1 from the in-degree of the child node.
-5. Repeat step 3 until all nodes have been traversed.
-6. If it is impossible to traverse all the nodes, it means that the current graph is not a directed acyclic graph. There is no topological sort.
-
-**The core Java code for breadth first search topological sorting is as follows :**
-
-```java
-public class TopologicalSort {
- /**
- * Determine whether there is a ring and the result of topological sorting
- *
- * Only directed acyclic graph (DAG) has topological sorting
- * The main methods of breadth first search:
- * 1、Iterate over all the vertices in the graph, and put the vertices whose in-degree is 0 into the queue.
- * 2、A vertex is polled from the queue, and the in-degree of the adjacent point of the vertex is updated (minus 1). If the in-degree of the adjacent point is reduced by 1 and then equals to 0, the adjacent point is entered into the queue.
- * 3、Keep executing step 2 until the queue is empty.
- * If it is impossible to traverse all the vertics, it means that the current graph is not a directed acyclic graph. There is no topological sort.
- *
- *
- * @return key returns the state, true if successful (no-loop), value if failed (loop), value is the result of topological sorting (could be one of these)
- */
- private Map.Entry<Boolean, List<Vertex>> topologicalSort() {
- // Node queue with an in-degree of 0
- Queue<Vertex> zeroIndegreeVertexQueue = new LinkedList<>();
- // Save the results
- List<Vertex> topoResultList = new ArrayList<>();
- // Save the nodes whose in-degree is not 0
- Map<Vertex, Integer> notZeroIndegreeVertexMap = new HashMap<>();
-
- // Scan all nodes and queue vertices with in-degree 0
- for (Map.Entry<Vertex, VertexInfo> vertices : verticesMap.entrySet()) {
- Vertex vertex = vertices.getKey();
- int inDegree = getIndegree(vertex);
-
- if (inDegree == 0) {
- zeroIndegreeVertexQueue.add(vertex);
- topoResultList.add(vertex);
- } else {
- notZeroIndegreeVertexMap.put(vertex, inDegree);
- }
- }
-
- // After scanning, there is no node with an in-degree of 0, indicating that there is a loop, and return directly
- if(zeroIndegreeVertexQueue.isEmpty()){
- return new AbstractMap.SimpleEntry(false, topoResultList);
- }
-
- // Using the topology algorithm, delete the node with an in-degree of 0 and its associated edges
- while (!zeroIndegreeVertexQueue.isEmpty()) {
- Vertex v = zeroIndegreeVertexQueue.poll();
- // Get the adjacent nodes
- Set<Vertex> subsequentNodes = getSubsequentNodes(v);
-
- for (Vertex subsequentVertex : subsequentNodes) {
-
- Integer degree = notZeroIndegreeVertexMap.get(subsequentVertex);
-
- if(--degree == 0){
- topoResultList.add(subsequentVertex);
- zeroIndegreeVertexQueue.add(subsequentVertex);
- notZeroIndegreeVertexMap.remove(subsequentVertex);
- }else{
- notZeroIndegreeVertexMap.put(subsequentVertex, degree);
- }
-
- }
- }
-
- //notZeroIndegreeVertexMap If it is empty, it means there is no ring
- AbstractMap.SimpleEntry resultMap = new AbstractMap.SimpleEntry(notZeroIndegreeVertexMap.size() == 0 , topoResultList);
- return resultMap;
-
- }
-}
-```
-
-*Notice: the output result is one of the topological sorting sequences of the graph.*
-
-Every time a vertex is taken from a set with an in-degree of 0, there is no special rule for taking out. The order of taking the vertices will result in a different topological sorting sequence (if the graph has multiple sorting sequences).
-
-Since each vertex is outputted with the edges starting from it removed. If the graph has V vertices and E edges, the time complexity of the algorithm is typically O(V+E). The final key of the algorithm as implemented here returns the state, true if it succeeds (no rings), with rings if it fails, and value if there are no rings as the result of topological sorting (which may be one of these). Note that the output is one of the topologically sorted sequences of the graph.
diff --git a/blog/en-us/DS-2.0-alpha-release.md b/blog/en-us/DS-2.0-alpha-release.md
deleted file mode 100644
index 4f34d61..0000000
--- a/blog/en-us/DS-2.0-alpha-release.md
+++ /dev/null
@@ -1,80 +0,0 @@
-# Refactoring, Plug-in, Performance Improves By 20 times, Apache DolphinScheduler 2.0 alpha Release Highlights Check!
-
-<div align='center'><img src="https://s1.imgpp.com/2021/11/16/a920be6733a3d99af38d1cdebfcbb3ff.md.png"></div>
-
-Hello community, good news! After nearly 10 months of joint efforts by more than 100 community contributors, we are happy to announce the release of Apache DolphinScheduler 2.0 alpha. This is the first major version of DolphinScheduler since it entered Apache. It has undergone a number of key updates and optimizations, which means a milestone in the development of DolphinScheduler.
-DolphinScheduler 2.0 alpha mainly refactors the implementation of Master, greatly optimizes the metadata structure and processing flow, adds SPI plug-in capabilities, and improves performance by 20 times. At the same time, the new version has designed a brand new UI interface to bring a better user experience. In addition, 2.0 alpha has newly added and optimized some features that are eagerly demanded in the community, such as parameter transfer, version control, import and export functions.
-Note: The current alpha version does not support automatic upgrades, and we will support this feature in the next version.
-
-2.0 alpha download link: https://dolphinscheduler.apache.org/en-us/download/download.html
-
-## Optimize the Kernel and Increase Performance By 20 Times
-
-Compared with DolphinScheduler 1.3.8, under the same hardware configuration (3 sets of 8-core 16G), 2.0 alpha throughput performance is increased by 20 times, which is mainly due to the reconstruction of the Master, the optimization of master execution process and the workflow processing process, etc. ,including:
-
-- Refactor the execution process of the Master, change the previous status polling monitoring to an event notification mechanism, which greatly reduces the pressure of the database polling;
-Remove the global lock, increase the fragmentation processing mechanism of the Master, change the sequential read and write commands to parallel processing, and enhance the horizontal expansion ability of the Master;
-
-- Optimize the workflow processing process, reduce the use of thread pool, and greatly increase the number of workflows processed by a single Master;
-Increase the caching mechanism to greatly reduce the number of database operations;
-
-- Optimize the database connection mode, which immensely reduces the time-consuming of database operation;
-
-- Simplify the processing flow and reduce unnecessary time-consuming operations during the processing.
-
-## UI Components Optimization Brings Brand New UI Interface
-
-<div align='center'><img src="https://s1.imgpp.com/2021/11/16/4e4024cbddbe3113f730c5e67f083c4f.md.png"></div>
-
-<div align='center'><img src="https://s1.imgpp.com/2021/11/16/75e002b21d827aee9aeaa3922c20c13f.md.png"></div>
-
-UI interface comparison: 1.3.9 (top) VS. 2.0 alpha (bottom)
-
-
->
-
-2.0 UI mainly optimized by:
-
-- Optimize the display of components: the interface is more concise, and the workflow process display is clearer;
-
-- Highlight the key content: click the task box can display task details;
-
-- Enhanced recognizability: The tool bar on the left is marked with names to make the tools easier to identify and easy to operate;
-
-- Adjust the order of the components: adjust the order of the components to be more in line with user habits.
-
-In addition to changes in performance and UI, DolphinScheduler has also undergone more than 20 new features and bug fixes.
-
-## List of New Features
-
-- Task result transfer function
-- Added Switch task and Pigeon task components
-- Added environmental management function
-- Added batch import , export and batch move functions
-- New registration center plug-in function
-- New task plugin function
-
-## Optimizations
-- Optimize the alarm group function
-- Optimize RestApi
-- Optimize workflow version management
-- Optimize import and export
-- Optimize worker group management function
-- Optimize the install.sh installation script to simplify the configuration process
-## Bug fix
-- [#6550]The list of environments in the DAG task pop-up window is not updated
-- [#6506]Fix install.sh for DS 2.0 and add comment to install_config.conf
-- [#6497]Shell task can not use user defined environment correctly
-- [#6478]Missing history data in complement data mode
-- [#6352]override the old process definition when I use the copy workflow feature
-- [#6342]Task instance page date backfill bug
-- [#5701]When deleting a user, the accessToken associated with the user should also be deleted
-- [#4809]cannot get application status when kerberos authentication is enabled
-- [#4450]Hive/Spark data sources do not support multi-tenancy when Kerberos authentication is enabled bug
-## Thanks to Contributors
-The release of DolphinScheduler 2.0 alpha embodies the wisdom and strength of the community contributors. Their active participation and great enthusiasm open the DolphinScheduler 2.0 era!
-Thanks so much for the participation of 100+ contributors (GitHub ID), and we are looking forward to more and more open sourcing enthusiasts joining the DolphinScheduler community co-construction, to contribute yourself to building a more usable big data workflow scheduling platform!
-
-<div align='center'><img src="https://s1.imgpp.com/2021/11/16/8926d45ead1f735e8cfca0e8142b315f.md.png"></div>
-
-2.0 List of alpha contributors
diff --git a/blog/en-us/DS_run_in_windows.md b/blog/en-us/DS_run_in_windows.md
deleted file mode 100644
index 23edb0d..0000000
--- a/blog/en-us/DS_run_in_windows.md
+++ /dev/null
@@ -1,102 +0,0 @@
-# Set-up DolphinScheduler Development Environment and Run Source Code on Windows OS
-
-1. ## Download Source Code
-
- Official Website: https://dolphinscheduler.apache.org/en-us/index.html
-
- GitHub Repository: https://github.com/apache/dolphinscheduler.git
-
- Here we use 1.3.6-release tag.
-
-2. ## Install Zookeeper on Windows
-
- 1. Download zookeeper https://www.apache.org/dyn/closer.lua/zookeeper/zookeeper-3.6.3/apache-zookeeper-3.6.3-bin.tar.gz.
-
- 2. Unzip 'apache-zookeeper-3.6.3-bin.tar.gz'.
-
- 3. Create 'data' and 'log' folder under zookeeper directory.
-
- 4. Copy the 'zoo_sample.cfg' file in the 'conf' directory, rename it to 'zoo.cfg' and modify the data and log directory settings:
-
- ~~~properties
- dataDir=I:\\setup\\apache-zookeeper-3.6.3-bin\\data
- dataLogDir=I:\\setup\\apache-zookeeper-3.6.3-bin\\log
- ~~~
-
- 5. Run the 'zkServer.cmd' in the bin directory, then run 'zkCli.cmd' to check the running status. Check zookeeper node information under root directory which shows success installation.
-
-3. ## Set-up Backend Environment
-
- 1. Create MYSQL database for debugging, named as 'dolphinschedulerKou'.
-
- 2. Import Maven project to IDEA, find 'pom.xml' under the root directory and modify the scope of 'mysql-connector-java' to compile.
-
- 3. Modify the 'datasource.properties' of module 'dolphinscheduler-dao'.
-
- ~~~properties
- # mysql
- spring.datasource.driver-class-name=com.mysql.jdbc.Driver
- spring.datasource.url=jdbc:mysql://localhost:3306/dolphinschedulerTest?useUnicode=true&characterEncoding=UTF-8
- spring.datasource.username=root
- spring.datasource.password=rootroot
- ~~~
-
- 4. Refresh dao module and run the main method of 'org.apache.dolphinscheduler.dao.upgrade.shell.CreateDolphinScheduler', the method will create project needed tables and data automatically.
-
- 5. Modify the 'zookeeper.properties' of module 'dolphinscheduler-service'.
-
- ~~~properties
- zookeeper.quorum=localhost:2181
- ~~~
-
- 6. Add standard output in the 'logback-worker.xml', 'logback-master.xml' and 'logback-api.xml'.
-
- ~~~xml
- <root level="INFO">
- <appender-ref ref="STDOUT"/> <!-- Add Standard Output -->
- <appender-ref ref="APILOGFILE"/>
- <appender-ref ref="SKYWALKING-LOG"/>
- </root>
- ~~~
-
-
-7. Start the MasterServer by execute the main method of 'org.apache.dolphinscheduler.server.master.MasterServer' and VM Options need to be set are:
-
- ~~~
- -Dlogging.config=classpath:logback-master.xml -Ddruid.mysql.usePingMethod=false
- ~~~
-
-8. Start the WorkerServer by execute the main method of 'org.apache.dolphinscheduler.server.worker.WorkerServer' and VM Options need to be set are:
-
- ~~~
- -Dlogging.config=classpath:logback-worker.xml -Ddruid.mysql.usePingMethod=false
- ~~~
-
-9. Start the ApiApplicationServer by execute the main method of 'org.apache.dolphinscheduler.api.ApiApplicationServer' and VM Options need to be set are:
-
- ~~~
- -Dlogging.config=classpath:logback-api.xml -Dspring.profiles.active=api
- ~~~
-
-10. If you need to use the log function, execute the main method of 'org.apache.dolphinscheduler.server.log.LoggerServer'.
-
-11. Backend swagger url: http://localhost:12345/dolphinscheduler/doc.html?language=en_us&lang=en.
-
-4. ## Set-up Frontend Environment
-
- 1. Install node (no more details).
-
- 2. Enter the folder 'dolphinscheduler-ui' and run.
-
- ~~~shell
- npm install
- npm run start
- ~~~
-
- 3. Visit [http://localhost:8888](http://localhost:8888/).
-
- 4. Sign in with admin account.
-
- > Username: admin
- >
- > Password: dolphinscheduler123
diff --git a/blog/en-us/DolphinScheduler-Vulnerability-Explanation.md b/blog/en-us/DolphinScheduler-Vulnerability-Explanation.md
deleted file mode 100644
index a31c641..0000000
--- a/blog/en-us/DolphinScheduler-Vulnerability-Explanation.md
+++ /dev/null
@@ -1,62 +0,0 @@
-[Security Notice] [Low:impact] DolphinScheduler Vulnerability Explanation
-
-
-The Apache DolphinScheduler community mailing list recently reported a vulnerability. Considering that many users have not subscribed to this mailing list, we hereby explain the situation:
-
-
-CVE-2021-27644
-
-
-Importance: Low
-
-
-Scope of impact: The exposed service is on the external network and the internal account is leaked. If none of the above, the user can decide whether to upgrade according to the actual demand.
-
-Affected version: <1.3.6
-
-Vulnerability description:
-
-
-This problem is caused by a vulnerability in mysql connectorj. Logged-in users of DolphinScheduler (users who are not logged in cannot perform this operation. It is recommended that companies conduct account security specifications) can fill in malicious parameters that cause security risks on the data source management page-Mysql data source. (Not affected if Mysql data source is not used)
-
-
-Repair suggestion: upgrade to version >=1.3.6
-
-
-Special thanks to
-
-
-Special thanks to the reporter of the vulnerability: Jin Chen from the Ant Security FG Lab, who restored the process of the vulnerability and provided the corresponding solution. The whole process showed the skills and expertise of professional security personnel, thanks for their contributions to the security guard of open source projects.
-
-
-Suggest
-
-
-Thanks to users for choosing Apache DolphinScheduler as the big data task scheduling system in enterprises, but it must be reminded that the scheduling system belongs to the core infrastructure of big data construction, please do not expose it to the external network. In addition, security measures should be taken for the account of internal personnel in the enterprise to reduce the risk of account leakage.
-
-
-Contribute
-
-
-So far, the Apache DolphinScheduler community has nearly 200+ code contributors and 70+ non-code contributors. Among them, there are also PMC or Committer of other top Apache projects. We embrace more partners to participate in the development of the open source community, working together to build a more stable, safe and reliable big data task scheduling system, and also contributing yourself to the rise of China's open source!
-
-
-WebSite: https://dolphinscheduler.apache.org/
-
-
-MailList: dev@dolphinscheduler@apache.org
-
-
-Twitter: @DolphinSchedule
-
-
-YouTube: https://www.youtube.com/channel/UCmrPmeE7dVqo8DYhSLHa0vA
-
-
-Slack: https://s.apache.org/dolphinscheduler-slack
-
-
-Contributor Guide: https://dolphinscheduler.apache.org/en-us/community/index.html
-
-
-If you have any questions about the vulnerability, welcome to participate in the discussion and we will wholeheartedly resolve your problems.
\ No newline at end of file
diff --git a/blog/en-us/DolphinScheduler_Kubernetes_Technology_in_action.md b/blog/en-us/DolphinScheduler_Kubernetes_Technology_in_action.md
deleted file mode 100644
index a65510a..0000000
--- a/blog/en-us/DolphinScheduler_Kubernetes_Technology_in_action.md
+++ /dev/null
@@ -1,458 +0,0 @@
----
-title:Technical Practice of Apache DolphinScheduler in Kubernetes System
-keywords: Apache,DolphinScheduler,scheduler,big data,ETL,airflow,hadoop,orchestration,dataops,Kubernetes
-description:Kubernetes is a cluster system based on container technology
----
-# Technical Practice of Apache DolphinScheduler in Kubernetes System
-
-<div align=center>
-<img src="/img/2022-02-24/1.jpeg"/>
-</div>
-
-Author | Yang Dian, Data and Algorithm Platform Architect | Shenzhen Transportation Center
-
-Editor | warrior_
-
-> Editor's note:
-
-> Kubernetes is a cluster system based on container technology, implements container orchestration, provides microservices and buses, and involves a large number of knowledge systems.
-
-> Starting from the author's actual work experience, this article shows us the use and technology sharing of DolphinScheduler in practice scenarios, hoping it can inspire those who have the same experience.
-
-## Why do we use DolphinSchedule? What value does it bring to us? And what problems did we encounter?
-
-Apache DolphinScheduler is an excellent distributed and easily scalable visual workflow task scheduling platform.
-
-In the field I'm working in, the application of DolphinScheduler can quickly solve the top ten pain points of data development for enterprises:
-
-- Multi-source data connection and access, most common data sources in the technical field can be accessed, and adding new data sources does not require too many changes;
-- Diversified +specialized + massive data task management, which really revolves problems focusing on big data (Hadoop family, Flink, etc.) task scheduling, and is significantly different from traditional schedulers;
-- Graphical task arrangement, super convenient user experience, competitive ability with commercial products, especially to most foreign open source products that cannot directly generate data tasks by dragging and dropping;
-- Task details, rich viewing of tasks, log, and time-running axis display, which well meet developers' requirement of refined data tasks management, quickly locating slow SQL and performance bottlenecks;
-- Support for a variety of distributed file systems, enrich users' choices for unstructured data;
-- Native multi-tenant management to meet the data task management and isolation requirements of large organizations;
-- Fully automatic distributed scheduling algorithm to balance all scheduling tasks;
-- The native function of cluster monitoring, which can monitor CPU, memory, number of connections, Zookeeper status is suitable for one-stop operation and maintenance for SME;
-- The native task alarm function, which minimizes the risk of task operation;
-- The strong community-based operation, listening to the real voice of customers, constantly adding new functions, and continuously optimizing the customer experience.
-
-I also encountered many new challenges in the projects launching various types of Apache DolphinScheduler:
-- How to deploy Apache DolphinScheduler with less human resources, and can a fully automatic cluster installation and deployment mode be realized?
-- How to standardize technical component implementation specifications?
-- Can unmanned supervision and system self-healing be achieved?
-- How to install and update the air-gap mode under the network security control?
-- Can it automatically expand without disturbing?
-- How to build and integrate the monitoring system?
-
-To solve the above challenges, we repeatedly integrated Apache DolphinScheduler into the existing Kubernetes cloud-native system to tackle problems and make Apache DolphinScheduler technology more powerful.
-
-## Kubernetes Technical System Bring New Technical Features to Apache DolphinScheduler
-
-
-After using Kubernetes to manage Apache DolphinScheduler, the overall technical solutions are quickly enriched and efficient technical features added, by which the above practical challenges tackled quickly:
-- The development environment and production environment was rapidly established in various independent deployment projects, and all can be implemented by one-key deployment and one-key upgrade;
-- Overall supports offline installation, and the installation speed is faster;
-- Unify the installation configuration information as much as possible to reduce the abnormal configuration of multiple projects. All configuration items can be managed through the internal git of the enterprise based on different projects;
-- Combine with object storage technology to unify unstructured data technology;
-- The convenient monitoring system is integrated with the existing prometheus monitoring system;
-- Mixed-use of multiple schedulers;
-- Fully automatic resource scheduling capability;
-- Fast self-healing ability, automatic abnormal restart, and restart mode based on probe mode.
-
-The cases in this article are based on Apache DolphinScheduler version 1.3.9.
-
-## Automated and Efficient Deployment Based on Helm tools
-
-First, let's introduce the installation method based on the Helm provided by the official website. Helm is the best way to find, share and use software to build Kubernetes, which is one of the graduate projects of cloud-native CNCF.
-
-<div align=center>
-<img src="/img/2022-02-24/2.png"/>
-</div>
-
-There are very detailed configuration files and cases on Apache DolphinScheduler official website and GitHub. Here I‘ll highlight some of the FAQs in the community.
-
-Official website document address
-https://dolphinscheduler.apache.org/en-us/docs/1.3.9/user_doc/kubernetes-deployment.html
-
-GitHub folder address
-https://github.com/apache/dolphinscheduler/tree/1.3.9-release/docker/kubernetes/dolphinscheduler
-
-Modify the image in the value.yaml file for offline installation (air-gap install);
-
-```
-image:
-repository: "apache/dolphinscheduler"
-tag: "1.3.9"
-pullPolicy: "IfNotPresent"
-```
-
-Pull, tag, and push for harbors installed in the company or private warehouses of other public clouds. Here we assume that the private warehouse address is harbor.abc.com, the host where the image is built has been docker login harbor.abc.com, and the new apache project under the private warehouse has been established and authorized.
-
-execute shell commands
-
-```
-docker pull apache/dolphinscheduler:1.3.9
-dock tag apache/dolphinscheduler:1.3.9
-harbor.abc.com/apache/dolphinscheduler:1.3.9
-docker push apache/dolphinscheduler:1.3.9
-```
-
-
-Then replace the image information in the value file. Here we recommend using the Always method to pull the image. In the production environment, try to check whether it is the latest image content every time to ensure the correctness of the software product. In addition, many coders are used to writing the tag as latest, and making the image without adding the tag information, which is very dangerous in the production environment. Because the image of the latest will be changed once any [...]
-
-```
-image:
-repository: "harbor.abc.com/apache/dolphinscheduler"
-tag: "1.3.9"
-pullPolicy: "Always"
-```
-
-Copy the entire directory of https://github.com/apache/dolphinscheduler/tree/1.3.9-release/docker/kubernetes/dolphinscheduler to a host that can execute the Helm command, and then execute
-```
-kubectl create ns ds139
-helm install dolphinscheduler . -n ds139 following the official website instruction to install offline.
-```
-to install offline.
-
-- To integrate DataX, MySQL, Oracle client components, first download the following components:
-https://repo1.maven.org/maven2/mysql/mysql-connector-java/5.1.49/mysql-connector-java-5.1.49.jar
-https://repo1.maven.org/maven2/com/oracle/database/jdbc/ojdbc8/
-https://github.com/alibaba/DataX/blob/master/userGuid.md Compile
-
-build and compile according to the prompt, and the file package is located in {DataX_source_code_home}/target/datax/datax/
-
-
-Create a new dockerfile based on the above plugin components, and the image that has been pushed to the private warehouse can be applied to the basic image.
-
-```
-FROM harbor.abc.com/apache/dolphinscheduler:1.3.9
-COPY *.jar /opt/dolphinscheduler/lib/
-RUN mkdir -p /opt/soft/datax
-COPY datax /opt/soft/datax
-```
-
-Save the dockerfile and execute the shell command
-
-
-```
-docker build -t harbor.abc.com/apache/dolphinscheduler:1.3.9-mysql-oracle-datax . #Don't forget the last point
-docker push harbor.abc.com/apache/dolphinscheduler:1.3.9-mysql-oracle-datax
-```
-
-Modify the value file
-
-```
-image:
-repository: "harbor.abc.com/apache/dolphinscheduler"
-tag: "1.3.9-mysql-oracle-datax"
-pullPolicy: "Always"
-```
-
-Execute helm install dolphinscheduler . -n ds139
-or helm upgrade dolphinscheduler -n ds139, or firstly helm uninstall dolphinscheduler -n ds139, and then execute helm install dolphinscheduler . -n ds139.
-
-- Generally, it is recommended to use an independent external PostgreSQL as the management database in the production environment, and use the independently installed Zookeeper environment (I used the Zookeeper operator https://github.com/pravega/zookeeper-operator in this case, which is scheduled in the same Kubernetes cluster as Apache DolphinScheduler ). We found that after using the external database, completely deleting and redeploying the Apache DolphinScheduler in Kubernetes, th [...]
-
-```
-## If not exists external database, by default, Dolphinscheduler's database will use it.
-postgresql:
-enabled: false
-postgresqlUsername: "root"
-postgresqlPassword: "root"
-postgresqlDatabase: "dolphinscheduler"
-persistence:
- enabled: false
- size: "20Gi"
- storageClass: "-"
-
-## If exists external database, and set postgresql.enable value to false.
-## external database will be used, otherwise Dolphinscheduler's database will be used.
-externalDatabase:
-type: "postgresql"
-driver: "org.postgresql.Driver"
-host: "192.168.1.100"
-port: "5432"
-username: "admin"
-password: "password"
-database: "dolphinscheduler"
-params: "characterEncoding=utf8"
-
-## If not exists external zookeeper, by default, Dolphinscheduler's zookeeper will use it.
-zookeeper:
-enabled: false
-fourlwCommandsWhitelist: "srvr,ruok,wchs,cons"
-persistence:
- enabled: false
- size: "20Gi"
- storageClass: "storage-nfs"
-zookeeperRoot: "/dolphinscheduler"
-
-## If exists external zookeeper, and set zookeeper.enable value to false.
-## If zookeeper.enable is false, Dolphinscheduler's zookeeper will use it.
-externalZookeeper:
-zookeeperQuorum: "zookeeper-0.zookeeper-headless.zookeeper.svc.cluster.local:2181,zookeeper-1.zookeeper-headless.zookeeper.svc.cluster.local:2181,zookeeper-2.zookeeper-headless.zookeeper.svc.cluster.local:2181"
-zookeeperRoot: "/dolphinscheduler"
-```
-
-## How to deploy GitOps based on Argo CD
-
-Argo CD is a declarative GitOps continuous delivery tool based on Kubernetes. GitOps is an incubation project of CNCF and a best practice tool for GitOps. For more details of GitOps, please refer to https://about.gitlab.com/topics/gitops/
-
-<div align=center>
-<img src="/img/2022-02-24/3.png"/>
-</div>
-
-GitOps can bring the following advantages to the implementation of Apache DolphinScheduler.
-- Graphical & one-click installation of clustered software;
-- Git records the full release process, one-click rollback;
-- Convenient DolphinScheduler tool log viewing.
-
-Implementation installation steps using Argo CD:
-- Download the Apache DolphinScheduler source code from GitHub, modify the value file, and refer to the content that needs to be modified in the helm installation in the previous chapter;
-- Create a new git project in the modified source code directory, and push it to the company's internal GitLab. The directory name of the GitHub source code is docker/kubernetes/dolphinscheduler;
-- Configure GitLab information in Argo CD, we use https mode here;
-
-<div align=center>
-<img src="/img/2022-02-24/4.png"/>
-</div>
-
-- Argo CD Create a new deployment project and fill in the relevant information
-
-<div align=center>
-<img src="/img/2022-02-24/5.png"/>
-</div>
-
-<div align=center>
-<img src="/img/2022-02-24/6.png"/>
-</div>
-
-Refresh and pull the deployment information in git to complete the final deployment work. You can see that pod, configmap, secret, service, ingress and other resources are automatically pulled up, and Argo CD displays the commit information and submitter username used by git push before, which completely records all release event information. At the same time, you can also roll back to the historical version with one click.
-
-<div align=center>
-<img src="/img/2022-02-24/7.png"/>
-</div>
-<div align=center>
-<img src="/img/2022-02-24/8.png"/>
-</div>
-
-- Relevant resource information can be seen through the kubectl command;
-```
-[root@tpk8s-master01 ~]# kubectl get po -n ds139
-NAME READY STATUS RESTARTS AGE
-dolphinscheduler-alert-96c74dc84-72cc9 1/1 Running 0 22m
-dolphinscheduler-api-78db664b7b-gsltq 1/1 Running 0 22m
-dolphinscheduler-master-0 1/1 Running 0 22m
-dolphinscheduler-master-1 1/1 Running 0 22m
-dolphinscheduler-master-2 1/1 Running 0 22m
-dolphinscheduler-worker-0 1/1 Running 0 22m
-dolphinscheduler-worker-1 1/1 Running 0 22m
-dolphinscheduler-worker-2 1/1 Running 0 22m
-
-[root@tpk8s-master01 ~]# kubectl get statefulset -n ds139
-NAME READY AGE
-dolphinscheduler-master 3/3 22m
-dolphinscheduler-worker 3/3 22m
-
-[root@tpk8s-master01 ~]# kubectl get cm -n ds139
-NAME DATA AGE
-dolphinscheduler-alert 15 23m
-dolphinscheduler-api 1 23m
-dolphinscheduler-common 29 23m
-dolphinscheduler-master 10 23m
-dolphinscheduler-worker 7 23m
-
-[root@tpk8s-master01 ~]# kubectl get service -n ds139
-NAME TYPE CLUSTER-IP EXTERNAL-IP PORT(S) AGE
-dolphinscheduler-api ClusterIP 10.43.238.5 <none> 12345/TCP 23m
-dolphinscheduler-master-headless ClusterIP None <none> 5678/TCP 23m
-dolphinscheduler-worker-headless ClusterIP None <none> 1234/TCP,50051/TCP 23m
-
-[root@tpk8s-master01 ~]# kubectl get ingress -n ds139
-NAME CLASS HOSTS ADDRESS
-dolphinscheduler <none> ds139.abc.com
-```
-
-- You can see that all pods are scattered on different hosts in the Kubernetes cluster, for example, workers 1 and 2 are on different nodes.
-
-<div align=center>
-<img src="/img/2022-02-24/9.png"/>
-</div>
-<div align=center>
-<img src="/img/2022-02-24/10.png"/>
-</div>
-
-We have configured ingress, and the company can easily use the domain name for access by configuring the pan-domain name within the company;
-<div align=center>
-<img src="/img/2022-02-24/11.png"/>
-</div>
-
-You can log in to the domain name for access:
-
-http:ds.139.abc.com/dolphinscheduler/ui/#/home
-
-
-- The specific configuration can modify the content in the value file:
-```
-ingress:
-enabled: true
-host: "ds139.abc.com"
-path: "/dolphinscheduler"
-tls:
- enabled: false
- secretName: "dolphinscheduler-tls"
-
-```
-- It is convenient to view the internal logs of each component of Apache DolphinScheduler:
-
-<div align=center>
-<img src="/img/2022-02-24/13.png"/>
-</div>
-
-- Check the deployed system, 3 masters, 3 workers, and Zookeeper are all configured normally;
-
-<div align=center>
-<img src="/img/2022-02-24/14.png"/>
-</div>
-<div align=center>
-<img src="/img/2022-02-24/15.png"/>
-</div>
-
-- Using Argo CD, it is very convenient to modify the number of replicas of components such as master, worker, api, alert, etc. Apache DolphinScheduler's helm configuration also reserves the setting information of CPU and memory. Here we modify the copy value in value. After modification, git push it to the company's internal GitLab.
-```
-master:
-
- ## PodManagementPolicy controls how pods are created during initial scale up, when replacing pods on nodes, or when scaling down.
-podManagementPolicy: "Parallel"
- ## Replicas is the desired number of replicas of the given Template.
-replicas: "5"
-
-worker:
- ## PodManagementPolicy controls how pods are created during initial scale up, when replacing pods on nodes, or when scaling down.
-podManagementPolicy: "Parallel"
- ## Replicas is the desired number of replicas of the given Template.
-replicas: "5"
-
-
-alert:
- ## Number of desired pods. This is a pointer to distinguish between explicit zero and not specified. Defaults to 1.
-replicas: "3"
-
-api:
- ## Number of desired pods. This is a pointer to distinguish between explicit zero and not specified. Defaults to 1.
-replicas: "3"
-```
-
-- Just click sync on Argo CD to synchronize, and the corresponding pods will be added as required
-
-<div align=center>
-<img src="/img/2022-02-24/17.png"/>
-</div>
-<div align=center>
-<img src="/img/2022-02-24/18.png"/>
-</div>
-
-```
-[root@tpk8s-master01 ~]# kubectl get po -n ds139
-NAME READY STATUS RESTARTS AGE
-dolphinscheduler-alert-96c74dc84-72cc9 1/1 Running 0 43m
-dolphinscheduler-alert-96c74dc84-j6zdh 1/1 Running 0 2m27s
-dolphinscheduler-alert-96c74dc84-rn9wb 1/1 Running 0 2m27s
-dolphinscheduler-api-78db664b7b-6j8rj 1/1 Running 0 2m27s
-dolphinscheduler-api-78db664b7b-bsdgv 1/1 Running 0 2m27s
-dolphinscheduler-api-78db664b7b-gsltq 1/1 Running 0 43m
-dolphinscheduler-master-0 1/1 Running 0 43m
-dolphinscheduler-master-1 1/1 Running 0 43m
-dolphinscheduler-master-2 1/1 Running 0 43m
-dolphinscheduler-master-3 1/1 Running 0 2m27s
-dolphinscheduler-master-4 1/1 Running 0 2m27s
-dolphinscheduler-worker-0 1/1 Running 0 43m
-dolphinscheduler-worker-1 1/1 Running 0 43m
-dolphinscheduler-worker-2 1/1 Running 0 43m
-dolphinscheduler-worker-3 1/1 Running 0 2m27s
-dolphinscheduler-worker-4 1/1 Running 0 2m27s
-
-```
-
-## Apache DolphinScheduler integrates with S3 Object Storage Technology
-
-How to configure the integration of s3 minio is one of the FAQs in the community. Here is the method of helm configuration based on Kubernetes.
-
-- Modify the s3 part of value, it is recommended to use ip+port to point to the minio server.
-```
-common:
- ##Configmap
-configmap:
- DOLPHINSCHEDULER_OPTS: ""
- DATA_BASEDIR_PATH: "/tmp/dolphinscheduler"
- RESOURCE_STORAGE_TYPE: "S3"
- RESOURCE_UPLOAD_PATH: "/dolphinscheduler"
- FS_DEFAULT_FS: "s3a://dfs"
- FS_S3A_ENDPOINT: "http://192.168.1.100:9000"
- FS_S3A_ACCESS_KEY: "admin"
- FS_S3A_SECRET_KEY: "password"
-```
-
-- The name of the bucket that stores dolphin files in minio is dolphinscheduler. I create new folders and files for testing here. The directory of the minio is under the tenant of the upload operation.
-
-<div align=center>
-<img src="/img/2022-02-24/19.png"/>
-</div>
-
-## Apache DolphinScheduler Integrates with Kube-Prometheus Technology
-
-
-
-- We use the Kube-prometheus operator technology in Kubernetes to automatically monitor the resources of each component of Apache DolphinScheduler after deploying.
-
-- Please pay attention to that the version of kube-prometheus needs to correspond to the major version of Kubernetes. https://github.com/prometheus-operator/kube-prometheus
-
-<div align=center>
-<img src="/img/2022-02-24/20.png"/>
-</div>
-<div align=center>
-<img src="/img/2022-02-24/21.png"/>
-</div>
-<div align=center>
-<img src="/img/2022-02-24/22.png"/>
-</div>
-
-## Technical Integration of Apache DolphinScheduler and Service Mesh
-
-- Through the service mesh technology, the observability analysis of API external service calls and internal calls of Apache DolphinScheduler can be realized to optimize the Apache DolphinScheduler product services.
-
-We use linkerd as a service mesh product for integration, which is also one of CNCF's excellent graduate projects.
-
-<div align=center>
-<img src="/img/2022-02-24/23.png"/>
-</div>
-
-Just modify the annotations in the value file of the Apache DolphinScheduler helm and redeploy, you can quickly inject the mesh proxy sidecar, as well as master, worker, API, alert and other components.
-
-```
-annotations: #{}
- linkerd.io/inject: enabled
-```
-You can observe the quality of service communication between components, the number of requests per second, etc.
-
-<div align=center>
-<img src="/img/2022-02-24/24.png"/>
-</div>
-<div align=center>
-<img src="/img/2022-02-24/25.png"/>
-</div>
-
-## Prospects on Apache DolphinScheduler Based on cloud-native Technology
-
-As a new-generation Cloud-Native big data tool, Apache DolphinScheduler is expected to integrate more excellent tools and features in the Kubernetes ecosystem in the future to meet more requirements of diversified user groups and scenarios.
-
-- Integration with Argo-workflow, users can call Argo-workflow single job, dag job, and periodic job in Apache DolphinScheduler through api, cli, etc.;
-- Use HPA to automatically expand and shrink workers to achieve unattended horizontal expansion;
-- Integrate the Spark operator and Flink operator tools of Kubernetes to achieve comprehensive cloud-native;
-- Implement multi-cloud and multi-cluster distributed job scheduling, and strengthen the architectural attributes of serverless+faas classes;
-- Use sidecar to periodically delete worker job logs to realize carefree operation and maintenance;
-
-Finally, I strongly recommend you to use Slack to communicate with the Apache DolphinScheduler community, which is officially recommended!
-
-<div align=center>
-<img src="/img/2022-02-24/26.png"/>
-</div>
\ No newline at end of file
diff --git a/blog/en-us/Eavy_Info.md b/blog/en-us/Eavy_Info.md
deleted file mode 100644
index 5825887..0000000
--- a/blog/en-us/Eavy_Info.md
+++ /dev/null
@@ -1,96 +0,0 @@
----
-title: Eavy Info Builds Data Asset Management Platform Services Based on Apache DolphinScheduler to Construct Government Information Ecology
-keywords: Apache,DolphinScheduler,scheduler,big data,ETL,airflow,hadoop,orchestration, dataops,2.0.1
-description: Based on the Apache DolphinScheduler, the cloud computing and big data provider Eavy Info
----
-# Eavy Info Builds Data Asset Management Platform Services Based on Apache DolphinScheduler to Construct Government Information Ecology | Use Case
-
-<div align=center>
-<img src="https://s1.imgpp.com/2021/12/29/1640759432737.png"/>
-</div>
-
-Based on the Apache DolphinScheduler, the cloud computing and big data provider Eavy Info has been serving the business operations in the company for more than a year.
-
-Combining with the government affairs informatization ecological construction business, Shandong Eavy Info built the data service module of its self-develop Asset Data Management and Control Platform based on Apache DolphinScheduler. How do they use Apache DolphinScheduler? Sun Hao, the R&D engineer of Evay Information, shared their experiences on their business practice.
-
-## R&D Background
-
-The prime operating of Eavy Info is focusing on ToG business, and data collection & sharing take a large proportion of their work. However, Traditional ETL tools, such as kettle, are not simple and easy enough to get started and employed for on-site project operation and maintenance by the front-line implementers. Therefore, creating a set of data acquisition (synchronization)-data processing-data management platform is particularly important.
-
-Out of this consideration, we have developed a Data Asset Management Platform, of which the core is a data service module based on Apache DolphinSchduler (referred to as DS below).
-
-Apache DolphinScheduler is a distributed, decentralized, easy-to-expand visual DAG scheduling system that supports multiple types of tasks including Shell, Python, Spark, Flink, etc., and has good scalability. Its overall structure is shown in the figure below:
-
-<div align=center>
-<img src="https://s1.imgpp.com/2021/12/28/1.png"/>
-</div>
-
-This is a typical master-slave architecture with strong horizontal scalability. The scheduling engine Quartz is a Java open source project of Spring Boot, it is easier to integrate and use for those familiar with Spring Boot development.
-
-As a scheduling system, DS supports the following functions:
-
-**Scheduling mode: ** The system supports timing scheduling and manual scheduling based on cron expressions. And it supports command types like workflow starting, execution starting from the current node, the fault-tolerant workflow resume, the paused process resume, execution starting from the failed node, complement, timing, rerun, pause, stop, and resume joinable threads. Among them, restoring the fault-tolerant workflow and restoring the joinable threads are two command types that ar [...]
-
-**Timing schedule:** The system uses quartz distributed scheduler and supports the visual generation of cron expressions.
-
-**Dependency: ** The system not only supports the dependency between the simple predecessor and successor nodes of the DAG but also provides task-dependent nodes to support custom task dependencies between processes.
-
-**Priority:** Support the priority of the process instance and task instance. If the priority of the process instance and task instance is not set, the default is first-in-first-out.
-
-**Email alert: ** Support SQL task query result email sending, process instance running result email alert, and fault tolerance alert notification.
-
-**Failure strategy:** For tasks that run in parallel, if there are tasks that fail, two failure strategy processing methods are provided. **Continue** refers to regardless of the status of the parallel running tasks until the end of the process failure. **End** means that once a failed task is found, the running parallel task will be killed at the same time, and the failed process will end.
-
-**Complement:** Complement historical data, support interval parallel, and serial complement methods.
-
-Based on Apache DolphinScheduler, we carry out the following practices.
-
-## Building A Data Synchronization Tool Based on DS
-
-In our business scenario, there are many types of business needs for data synchronization, but the amount of data is not particularly large and is real-time-undemanding. So at the beginning of the architecture selection, we chose the combination of Datax+Apache DolphinScheduler and implemented the transformation of the corresponding business. Now it is integrated into various projects as a service product to provide offline synchronization services.
-
-<div align=center>
-<img src="https://s1.imgpp.com/2021/12/29/1-1.png"/>
-</div>
-
-Synchronization tasks are divided into periodic tasks and one-time tasks. After the configuration tasks of the input and output sources, the corn expression needs to be configured for periodic tasks, and then the save interface is called to send the synchronization tasks to the DS scheduling platform.
-
-<div align=center>
-<img src="https://s1.imgpp.com/2021/12/29/2-1.png"/>
-</div>
-
-Synchronization tasks are divided into **periodic tasks** and **one-time tasks**. After the configuration tasks of the input and output sources are configured, the corn expression needs to be configured for periodic tasks, and then the **save interface** is called to send the synchronization tasks to the DS scheduling platform.
-
-We gave up the previous UI front-end of DS after comprehensive consideration and reused the DS back-end interfaces to carry the online procedure, start and stopping, deleting, and log viewing.
-
-The design of the entire synchronization module is aimed to reuse the diversity of input and output plugins of the Datax component and integrate with the optimization of DS to achieve an offline synchronization task. This is a component diagram of our current synchronization.
-
-
-<div align=center>
-
-<img src="https://s1.imgpp.com/2021/12/30/ffd0c839647bcce4c208ee0cf5b7622b.png"/>
-</div>
-
-## Self Development Practices Based on DS
-
-Anyone familiar with Datax knows that it is essentially an ETL tool, which provides a transformer module that supports Groovy syntax, and at the same time further enrich the tool classes used in the transformer in the Datax source code, such as replacing, regular matching, screening, desensitization, statistics, and other functions. That shows its property of Transform. Since the tasks are implemented with DAG diagrams in Apache DolphinScheduler, we wonder that is it possible to abstract [...]
-
-<div align=center>
-<img src="https://s1.imgpp.com/2021/12/30/ffd0c839647bcce4c208ee0cf5b7622b.png"/>
-</div>
-
-Each component is regarded as a module, and the dependency between the functions of each module is dealt with the dependency of DS. The corresponding component and the component transfer data are stored at the front-end, which means the front-end performs the transfer and logical judgments between most of the components after introducing input (input component) , since each component can be seen as an output/output of Datax. Once all parameters are set, the final output is determined. Th [...]
-
-PS: Because our business scenarios may involve cross-database queries (MySQL combined query of different instances), our SQL component uses Presto to implement a unified SQL layer, so that you can also use Presto to do combined retrieval even when data sources are under various IP instances (business-related).
-
-## Other Attempts
-
-People dabble in the governance process know that a simple governance process can lead to a quality report. We write part of the government records into ES, and then use the aggregation capabilities of ES to obtain a quality report.
-
-<div align=center>
-<img src="https://s1.imgpp.com/2021/12/29/4da40632c21dbea51d2951d98ee18f1b.png"/>
-</div>
-
-The above are some practices that we have made based on DS and middlewares like Datax, combining with businesses to meet our own needs.
-
-From EasyScheduler to the current Apache DolphinScheduler 2.0, we are more often a spectator or follower, but today we shared our practical experience to build data service modules of Data Asset Management and Control Platform based on Apache DolphinScheduler. Currently, we have served the on-site operation of multiple project departments of the company based on the Apache DolphinScheduler scheduling platform for more than a year. With the release of Apache DolphinScheduler 2.0, we have [...]
\ No newline at end of file
diff --git a/blog/en-us/Exploration_and_practice_of_Tujia_Big_Data_Platform_Based.md b/blog/en-us/Exploration_and_practice_of_Tujia_Big_Data_Platform_Based.md
deleted file mode 100644
index 970618e..0000000
--- a/blog/en-us/Exploration_and_practice_of_Tujia_Big_Data_Platform_Based.md
+++ /dev/null
@@ -1,169 +0,0 @@
----
-title: Exploration and practice of Tujia Big Data Platform Based on Apache DolphinScheduler
-keywords: Apache,DolphinScheduler,scheduler,big data,ETL,airflow,hadoop,orchestration,dataops,Meetup,Tujia
-description: Tujia introduced Apache DolphinScheduler in 2019. At the recent Apache DolphinScheduler Meetup in February
----
-# Exploration and practice of Tujia Big Data Platform Based on Apache DolphinScheduler
-
-<div align=center>
-
-<img src="/img/2022-3-9/Eng/1.jpeg"/>
-
-</div>
-
-Tujia introduced Apache DolphinScheduler in 2019. At the recent Apache DolphinScheduler Meetup in February, Tujia Big Data Engineer Xuchao Zan introduced the process of Tujia's access to Apache DolphinScheduler and the functional improvements in detail.
-
-<div align=center>
-
-<img style="width: 25%;" src="/img/2022-3-9/Eng/2.png"/>
-</div>
-
-Xuchao Zan, Big Data Engineer, Data Development engineer from Tujia, is mainly responsible for the development, maintenance, and tuning of the big data platform.
-
-**Watch the record here:**[https://www.bilibili.com/video/BV1Ki4y117WV?spm_id_from=333.999.0.0](https://www.bilibili.com/video/BV1Ki4y117WV?spm_id_from=333.999.0.0)
-
-This speech mainly consists of 4 parts. The first part is the current status of Tujia's platform, introducing the process of Tujia's data flow, how Tujia provides data services, and the role of Apache DolphinScheduler in the platform. The second part will introduce the scheduler selection process of Tujia, which mainly refers to some features of the scheduler and the process of access. The third part is mainly about some improvements and functional expansions of the system, including sup [...]
-
-## Status of Tujia Big Data Platform
-
-### 01 Big Data Platform schema
-
-First, let's introduce the schema of Tujia Big Data Platform and the role of Apache DolphinScheduler in the platform.
-
-<div align=center>
-
-<img src="/img/2022-3-9/Eng/3.png"/>
-
-</div>
-
-The architecture of Tujia Big Data Platform
-
-The picture above shows the architecture of our data platform, which mainly includes **data source, data collection, data storage, data management, and finally service provision.**
-
-**The main source of the data** includes three parts: data synchronization of the business from library MySQL API, involving the Dubbo interface, the http interface, and the embedded point data of the web page.
-
-**Data collection** adopts real-time and offline synchronization, business data is incremental synchronized based on Canal, logs are collected by Flume, and Kafka is collected in real-time and falls on HDFS.
-
-**The data storage process** mainly involves some data synchronization services. After the data falls into HDFS, it is cleaned, processed, and then pushed online to provide services.
-
-**At the data management level**, the data dictionary records the metadata information of the business, the definition of the model, and the mapping relationship between various levels, which is convenient for users to find the data they care about; the log records the operation log of the task, and the alarm configures the fault information, etc. The dispatching system, as a command center of big data, rationally allocates dispatching resources and can better serve the business. Metrics [...]
-
-The last part is the**data service**, which mainly includes ad hoc data query, report preview, data download and upload analysis, online business data support release, etc.
-
-### 02 The role of Apache DolphinScheduler in the platform
-
-The following focuses on the role of the scheduler in the platform. The data tunnel is synchronized, and incremental data is pulled regularly every morning. After the data is cleaned and processed, it is pushed online to provide services. It also charges the processing of the data model and the interface configuration greatly improves Productivity. The service of the timed report, push email, support the display of attachments, text table, and line chart. The report push function allows [...]
-
-## Introduces Apache DolphinScheduler
-
-The second part is about the work we have done by introducing Apache DolphinScheduler.
-
-
-Apache DolphinScheduler is advanced in many aspects. As a command center of big data, it's undoubtedly reliable. The decentralized design of Apache DolphinScheduler avoids the single point of failure problem, and once a problem occurs with one node, the task will be automatically restarted on other nodes, which greatly improves the system's reliability.
-
-In addition, Apache DolphinScheduler is simple and practical, which reduces learning costs and improves work efficiency. Now many staff in the company are using it, including analysts, product operational staff, and developers.
-
-The scalability of scheduling is also very important because as the amount of tasks increases, the cluster can add resources in time to provide services. A wide range of applications is also a key reason for us to choose Apache DolphinScheduler. It supports a variety of task types: Shell, MR, Spark, SQL (MySQL, PostgreSQL, Hive, SparkSQL), Python, Sub_Process, Procedure, etc. and enables workflow timing scheduling and dependency scheduling, manual scheduling, manual pause/stop/resume, as [...]
-
-Next is the upgrade of our time scheduling.
-
-Before adopting Apache DolphinScheduler, our scheduling was quite confusing. Some people deployed their own local Crontab, some used Oozie for scheduling, and some used the system for time schedules. The management is chaotic, the timeliness& accuracy cannot be guaranteed, and tasks cannot be found from time to time due to lacking one unified scheduling platform. In addition, the self-built scheduler is not stable enough due to lacking configuration dependency and data output guarantee, [...]
-
-<div align=center>
-
-<img src="/img/2022-3-9/Eng/4.png"/>
-
-</div>
-
-In 2019, we introduced Apache DolphinScheduler, and it has been running stably for nearly 3 years.
-
-Below is some data of our system transfer.
-
-
-We have built an Apache DolphinScheduler cluster with a total of 4 physical machines. Currently, a single machine supports 100 task scheduling concurrently.
-
-Algorithms are also configured with special machines and be isolated.
-
-
-Most of our tasks are processed by Oozie, which are mainly Spark and Hive tasks. There are also some scripts on Crontab, some mailing tasks, and scheduled tasks of the reporting system.
-
-## The Scheduling System Re-built Based on Apache DolphinScheduler
-
-Before reconstruction, we have optimized the system, such as supporting table-level dependencies, extending the mail function, etc. After introducing Apache DolphinScheduler, we re-built a scheduling system based on it to provide better services.
-
-**Firstly, the synchronization of table dependencies was supported.**At that time, considering the task migration may be parallel, the tasks could not be synchronized all at once and needed to be marked as the table tasks run successfully. Therefore, we developed the function to solve the dependency problem in task migration. However, the different naming style of users makes it difficult to locate the task of the table when configuring dependencies, and we cannot identify which tables a [...]
-
-<div align=center>
-
-<img src="/img/2022-3-9/Eng/5.png"/>
-
-</div>
-
-**Secondly, Mail tasks support multiple tables.**Scheduling has its self-installed mail push function, but only supports a single table. With more and more business requirements, we need to configure multiple tables and multiple sheets, and the number of text and attachments required to be displayed differently, which needs to be configured. In addition, it is necessary to support the function of line charts to enrich the text pages. Furthermore, users also want to be able to add notes t [...]
-
-<div align=center>
-
-<img src="/img/2022-3-9/Eng/6.png"/>
-
-</div>
-
-<div align=center>
-
-<img src="/img/2022-3-9/Eng/7.png"/>
-
-</div>
-
-**Thirdly, it supports rich data source synchronization.** Due to some data transmission issues, we needed to modify configuration codes greatly, compile, package and upload data in the previous migration process, which is complex and error-prone, and data and online data cannot be separated due to data source disunity; in terms of development efficiency, there is a lot of repetition in the code, and the lack of a unified configuration tool and the unreasonable parameter configuration le [...]
-
-<div align=center>
-
-<img src="/img/2022-3-9/Eng/8.png"/>
-
-</div>
-
-<div align=center>
-
-<img src="/img/2022-3-9/Eng/9.png"/>
-
-</div>
-
-We simplified the data development process, made MySQL support the high availability of pxc/mha, and improved the efficiency of data synchronization.
-
-
-The input data sources support relational databases and FTP synchronization. As the computing engine, the output data sources of Spark support various relational databases, as well as message middleware Kafka, MQ, and Redis.
-
-Next, let's get through the process of our implementation.
-
-
-We have extended the data source of Apache DolphinScheduler to support the expansion of Kafka mq and namespace. Before MySQL synchronization, we first calculate an increment locally and synchronize the incremental data to MySQL. Spark also supports the HA of MySQL pxc/qmha. In addition, there is a qps limit when pushing MQ and Redis, we control the number of partitions and concurrency of Spark by the amount of data.
-
-## Improvements
-
-The fourth part is mainly about the added functions to the system, including:
-
-1. Spark supports publishing system
-2. Data quality open up
-3. Display of Data lineage
-### 01 Spark task supports publishing system
-
-More than 80% of our usual schedules are Spark jar package tasks, but the task release process and the code modification are not normalized. This leads to code inconsistencies from time to time, and even causes online problems in severe cases.
-
-This requires us to refine the release process for tasks. We mainly use the publishing system, the Jenkens packaging function, compile and package to generate btag, and then publish and generate rtag after the test is completed, and the code is merged into master. This avoids the problem of code inconsistency and reduces the steps for jar package upload. After compiling and generating the jar package, the system will automatically push the jar package to the resource center of Apache Dol [...]
-
-### 02 Data quality connection
-
-Data quality is the basis for ensuring the validity and accuracy of analytical conclusions. We need a complete data monitoring output process to make the data more convincing. The quality platform ensures data accuracy, integrity, consistency, and timeliness from four aspects, and supports multiple alarm methods such as telephone, WeCom, and email to inform users.
-
-
-Next, we will introduce how to connect the data quality and scheduling system. After the scheduling task is completed, the message record is sent, the data quality platform receives the message, and triggers the rule of the data quality monitoring. Abide by the monitoring rule, the downstream operation is blocked or an alarm message is sent.
-
-### 03 Data linage display
-
-Data lineage is key to metadata management, data governance, and data quality. It can track the source, processing, and provenance of data, provide a basis for data value assessment, and describe the flow of source data among processes, tables, reports, and ad hoc queries, the dependencies between tables, tables & offline ETL tasks, as well as scheduling platforms & computing engines. The data warehouse is built on Hive, and the raw data of Hive often comes from the production DB, and th [...]
-
-
-* **Data trace:** When data is abnormal, it helps to trace the cause of the abnormality; impact analysis, tracing the source of the data and data processing process.
-* **Data e****valuat****ion**: Provide a basis for evaluating data value in terms of data target, update importance, and update frequency.
-* **Life cycle:** Intuitively obtain the entire life cycle of data, providing a basis for data governance.
-The collection process of data linage is mainly about: Spark monitors the SQL and inserted tables by monitoring the Spark API, obtains and parses the Spark execution plan.
-
diff --git a/blog/en-us/FAQ.md b/blog/en-us/FAQ.md
deleted file mode 100644
index 84bb506..0000000
--- a/blog/en-us/FAQ.md
+++ /dev/null
@@ -1,13 +0,0 @@
-#### Q: What is Apache DolphinScheduler?
-A: DolphinScheduler is a distributed and extensible workflow scheduler platform with a powerful DAG visual interface. It is dedicated to solving complex task dependencies in data pipelines, making multi types of tasks available out of the box
-
-#### Q: Is DolphinScheduler an ETL tool?
-A: Apache DolphinScheduler is an open-source visual workflow automation tool used for setting up and maintaining data pipelines.
-
-DolphinScheduler isn't an ETL tool. but it can helps you manage ETL pipelines and it also integrates some ETL tools like sqoop and datax.
-
-#### Q:What is DolphinScheduler used for?
-A: Apache DolphinScheduler is a workflow automation and scheduling platform that can be used to assemble and manage data pipelines. Similar systems such as oozie and Azkaban etc
-
-#### Q: Who is using Apache DolphinScheduler?
-A: more than 400+ companys are using DolphinScheduler as their scheduler platform, some user cases like IBM、Tencent、meituan、Lenovo、Nokia、SHEIN、Inspur、pingan、ICBC and so on
\ No newline at end of file
diff --git a/blog/en-us/Hangzhou_cisco.md b/blog/en-us/Hangzhou_cisco.md
deleted file mode 100644
index da6fac3..0000000
--- a/blog/en-us/Hangzhou_cisco.md
+++ /dev/null
@@ -1,138 +0,0 @@
-# Cisco Hangzhou's Travel Through Apache DolphinScheduler Alert Module Refactor
-
-<div align=center>
-
-<img src="/img/3-16/Eng/1.png"/>
-
-</div>
-
->Cisco Hangzhou has introduced Apache DolphinScheduler into the company's self-built big data platform. At present, the team of **Qingwang Li, Big Data Engineer from Cisco Hangzhou**has basically completed the Alert Module reform, which aims to build a more complete Alert module to meet the needs of complex alerts in business scenarios.
-<div align=center>
-
-<img src="/img/3-16/Eng/2.png"/>
-
-</div>
-
-Li Qingwang
-
-Big Data Engineer, Cisco Hangzhou, is responsible for big data development, such as Spark and the scheduling systems.
-We encountered many problems in using the original scheduling platform to process big data tasks. For example, for a task of processing and aggregated analysis of data, multiple pre-Spark tasks are used to process and analyze data from different data sources firstly, and the final Spark task aggregates and analyzes the results processed during this period to get the final data we want. Unfortunately, the scheduling platform could not execute multiple tasks serially, and we had to estimat [...]
-To our surprise, the core function of Apache DolphinScheduler - **workflow definition can connect tasks in series**, perfectly fits our needs. So, we introduced Apache DolphinScheduler into our big data platform, and I was mainly responsible for the Alert module reform. At present, other colleagues are promoting the integration of K8s, hoping that future tasks will be executed in K8s.
-Today, I will share the reform journey of the Alert module.
-
-## 01 **Alert Module Design**
-
-<div align=center>
-<img src="/img/3-16/Eng/3.png"/>
-</div>
-
-Design of the DolphinScheduler Alert module
-The Alert mode of Apache DolphinScheduler version 1.0 uses configuring alert.properties to send alerts by configuring emails, SMS, etc., but this method is no longer suitable for the current scenario. The official has also refactored the alarm module. For details of the design ideas, please refer to the official documents:
-
-[https://github.com/apache/dolphinscheduler/issues/3049](https://github.com/apache/dolphinscheduler/issues/3049)
-
-[https://dolphinscheduler.apache.org/en-us/development/backend/spi/alert.html](https://dolphinscheduler.apache.org/en-us/development/backend/spi/alert.html)
-
-The Apache DolphinScheduler alert module is an independently started service, and one of the cores is the AlertPluginManager class. The alarm module integrates many plug-ins, such as DingTalk, WeChat, Feishu, mail, etc., which are written in the source code in an independent form. When the service is started, the plug-in will be parsed and the configured parameters will be formatted into JSON, by which the front-end page will automatically be rendered. AlertPluginManager caches plugins i [...]
-
-When the workflow is configured with a notification policy, the Worker executes the workflow, and the execution result matches the notification policy successfully. After inserting the alarm data into the DB, the thread pool scans the DB and calls the send method of the AlertSender class to transfer the alarm data. Alarm data is bound to an alarm group, which corresponds to multiple alarm instances. The AlertSender class traverses the alert instance, obtains the plug-in instance through [...]
-
-It should be noted that the RPC service is also started when the Alert server is started. This is an alarm method designed for special types of tasks, such as SQL query reports. It allows workers to directly access the Alert server through RPC, and use the Alert module to complete the alarm, while the data is not written to DB. But on the whole, the alarm mode of Apache DolphinScheduler is still based on the way of writing DB and asynchronous interaction.
-
-<div align=center>
-
-<img src="/img/3-16/Eng/4.png"/>
-
-</div>
-
-After defining the workflow, you can set the notification policy and bind the alarm group before starting.
-
-<div align=center>
-
-<img src="/img/3-16/Eng/5.png"/>
-
-</div>
-
-In the task dimension, you can configure a timeout alarm to be triggered when the task times out. There is no alarm group configuration here, tasks and workflows share the same alarm group. When the task times out, it will be pushed to the alarm group set by the workflow.
-
-<div align=center>
-
-<img src="/img/3-16/Eng/6.png"/>
-
-</div>
-
-The above figure is a flowchart of system alarm configuration. It shows that a workflow can be configured with multiple task instances, tasks can be configured to timeout to trigger alarms, and workflow success or failure can trigger alarms. An alarm group can be bound to multiple alarm instances. But this configuration mode is not reasonable. We hope that the alarm instance can also match the status of the workflow/task instance, that is, the success and failure of the workflow call the [...]
-
-<div align=center>
-
-<img src="/img/3-16/Eng/7.png"/>
-
-</div>
-
-Create an alarm group that can be bound to multiple alarm instances.
-
-## 02 **Big data task alarm scenario**
-
-The following are some common big data task alarm scenarios in our daily work.
-
-<div align=center>
-
-<img src="/img/3-16/Eng/8.png"/>
-
-</div>
-
-For scheduled tasks, notifications are sent before starting execution, when the task goes online, goes offline, or modifies parameters, whether the task execution succeeds or fails. While for different results of the same task, we want to trigger different notifications, such as SMS, DingTalk, or WeChat group notification for successful tasks, and if the task fails, we need to notify the corresponding R&D personnel as soon as possible to get a faster response, and at this time, @correspo [...]
-
-Although the architecture of Apache DolphinScheduler meets the requirements of the actual scenario, the problem is that the page configuration of the alarm module can only choose to trigger the notification for successful or fail tasks, and it is bound to the same alarm group, that is, the way of alarming is the same regardless of success or failure, which does not satisfy our need for different results to be notified in different ways in a real production environment. Therefore, we made [...]
-
-## 03 **Alert module modification**
-
-<div align=center>
-
-<img src="/img/3-16/Eng/9.png"/>
-
-</div>
-
-The first refactor points to alert instance. Previously, when an alarm instance was added, triggering an alarm would trigger the send method of the instance. We hope that when defining an alarm instance, an alarm policy can be bound. There are three options: send if the task succeeds, send on failure, and send on both success and failure.
-
-In the task definition dimension, there is a timeout alarm function, which actually corresponds to the failed strategy.
-
-<div align=center>
-
-<img src="/img/3-16/Eng/10.png"/>
-
-</div>
-
-The above picture shows the completed configuration page. On the Create Alarm Instance page, we added an alarm type field, choosing to call the plugin on success, failure, or whether it succeeds or fails.
-
-<div align=center>
-
-<img src="/img/3-16/Eng/11.png"/>
-
-</div>
-
-The above picture shows the architecture of the Apache DolphinScheduler alarm module after the refactor. We have made two changes to it.
-
-First, when the workflow or task is executed, if an alarm is triggered, when writing to the DB, the execution result of the workflow or task will be saved, whether it succeeds or fails.
-
-Second, adds a logical judgment to the alarm instance calling send method, which matches the alarm instance with the task status, executes the alarm instance sending logic if it matches, and filters if it does not match.
-
-The alarm module refactored supports the following scenarios:
-
-<div align=center>
-
-<img src="/img/3-16/Eng/12.png"/>
-
-</div>
-
-For detailed design, please refer to the issue: [https://github.com/apache/dolphinscheduler/issues/7992](https://github.com/apache/dolphinscheduler/issues/7992)
-
-See the code for details: [https://github.com/apache/dolphinscheduler/pull/8636](https://github.com/apache/dolphinscheduler/pull/8636)
-
-In addition, we also put forward some proposals to the community for the alarm module of Apache DolphinScheduler. Welcome anyone who is interested in this issue to follow up the work together:
-
-* When the workflow starts or goes online or offline, or when parameters are modified, a notification can be triggered;
-* The alarming scenario is for worker monitoring. If the worker hangs up or disconnects from ZK and loses its heartbeat, it will consider the worker is down, trigger an alarm, and match the alarm group with ID 1 by default. This setting is explained in the source code, which is easy to be ignored, and you won't likely to set the alarm group with ID 1, thus fails you to get the notification of worker downtime instantly;
-* The alarm module currently supports Feishu, DingTalk, WeChat, Email, and other plug-ins, which are commonly used by domestic users. While users abroad are more used to plug-ins like Webex Teams, or PagerDuty, a commonly used alarm plug-in abroad. We re-developed these and plug-ins and contributed them to the community. For now, there are some more commonly used plug-ins abroad, such as Microsoft Teams, etc., anyone who is interested in it is recommended to submit a PR to the community.
-The last but not least, big data practitioners probably are not skilled with the front-end stuff and may quit by the front-end page development when developing and alarm plug-ins. But I'd like to point out that you do not need to write front-end code at all when developing the Apache DolphinScheduler alarm plug-in. You only need to configure the parameters to be entered on the page or the buttons to be selected in the Java code when creating a new alarm instance plug-in (see org.apache.d [...]
-
diff --git a/blog/en-us/How_Does_360_DIGITECH_process_10_000+_workflow_instances_per_day.md b/blog/en-us/How_Does_360_DIGITECH_process_10_000+_workflow_instances_per_day.md
deleted file mode 100644
index 43c634c..0000000
--- a/blog/en-us/How_Does_360_DIGITECH_process_10_000+_workflow_instances_per_day.md
+++ /dev/null
@@ -1,175 +0,0 @@
----
-title: How Does 360 DIGITECH process 10,000+ workflow instances per day by Apache DolphinScheduler
-keywords: Apache,DolphinScheduler,scheduler,big data,ETL,airflow,hadoop,orchestration,dataops,Meetup,Tujia
-description: ince 2020, 360 DIGITECH has fully migrated its scheduling system from Azkaban to Apache DolphinScheduler
----
-# How Does 360 DIGITECH process 10,000+ workflow instances per day by Apache DolphinScheduler
-
-<div align=center>
-
-<img src="/img/2022-3-11/Eng/1.jpeg"/>
-
-</div>
-
-Since 2020, 360 DIGITECH has fully migrated its scheduling system from Azkaban to Apache DolphinScheduler. As a senior user of DolphinScheduler, 360 DIGITECH now uses DolphinScheduler to process 10,000+ workflow instances per day.
-
-To meet the practical needs of big data platform and algorithm model business, 360 DIGITECH has made many modifications on DolphinScheduler such as alarm monitoring expansion, worker maintenance mode adding, multi-server room renovation, etc. to make it more convenient for operation and maintenance.
-
-How did they carry out the re-development? Jianmin Liu, a big data engineer at 360 DIGITECH, shared this topic in detail at the Apache DolphinScheduler February Meetup.
-
-<div align=center>
-
-<img style="width: 25%;" src="/img/2022-3-11/Eng/2.png"/>
-
-</div>
-
-Jianmin Liu, a big data engineer from 360 DIGITECH, mainly engaged in the research of ETL task and scheduling framework, and the development of big data platforms and real-time computing platforms.
-
-## Migrate from Azkaban to Apache DolphinScheduler
-
-By 2019, 360 DIGITECH used Azkaban for big data processing.
-
-Azkaban is a batch workflow task scheduler open-sourced by Linkedin. It is easy to install, users can create tasks and upload zip packages for workflow scheduling just by installing the web and executor server.
-
-Azkaban's web-executor architecture is shown as below:
-
-<div align=center>
-
-<img style="width: 40%;" src="/img/2022-3-11/Eng/3.png"/>
-
-</div>
-
-### Disadvantages of Azkaban
-
-Azkaban is suitable for simple scheduling scenarios, and after three years of use, we found three fatal flaws in it.
-
-1. Poor experience
-
-It has no visual task creation feature, and you need to upload a zip package to create and modify tasks, which is not convenient; in addition, Azkaban has no function to manage resource files.
-
-2. Not powerful enough
-
-Azkaban lacks some indispensable features for production environments, such as complement and cross-task dependencies; user and permission control are too weak, and scheduling configuration does not support per month, so we need to make up for it in many other ways in production.
-
-3. Not good enough stability
-
-The most fatal flaw of Azkaban is that it is not stable enough, and tasks are often backlogged when the executor is overloaded; tasks at the hourly or minute level are prone to miss scheduling; there are no timeout alerts, and although we have developed our own limited SMS alerts, we are still prone to production accidents.
-
-We had a retrofit in 2018 to address these shortcomings, but the Azkaban source code was complex and the retrofit was painful, so we decided to re-choose the scheduler. At that time, we tested Airflow, DolphinScheduler, and XXL-job, but the Airflow Python technology stack didn't match ours, and the XXL-job functionality was too simple, so it was clear that DolphinScheduler was the better choice.
-
-In 2019, we Folked the code of EasyScheduler 1.0, migrated parts of it with scheduling tasks in 2020, and went it live until now.
-
-### Selection Research
-
-We chose DolphinScheduler because of its four advantages:
-
-1. Decentralized structure with multiple masters and multiple workers.
-
-2. Scheduling framework is powerful and supports multiple task types with cross-project dependencies and complement capabilities.
-
-3. Good user experience, visual DAG workflows editing, and ease of operation.
-
-4.Java stack with good scalability.
-
-The conversion process was very smooth and we migrated the scheduling system to DolphinScheduler without any problems.
-
-## Use of DolphinScheduler
-
-At 360 DIGITECH, DolphinScheduler is not only used by the Big Data department, but also by the Algorithms department for some of its features. To make it easier for the Algorithms department to use DolphinScheduler's features, we integrated it into our own Yushu Big Data Platform.
-
-### Yushu Big Data Platform
-
-<div align=center>
-
-<img src="/img/2022-3-11/Eng/4.png"/>
-
-</div>
-
-Yushu is a big data platform composed of basic components, Yushu platform, monitoring&operation, and business service layer, which can do a query, data real-time calculation, message queue, real-time data warehouse, data synchronization, data governance, and others. Among them, offline scheduling uses DolphinScheduler to schedule the ETL task of scheduling data sources to Hive data warehouse, as well as supports TiDB real-time monitoring, real-time data reports, and other functions.
-
-### DolphinScheduler Nested to Yushu
-
-To support the company's algorithm modeling needs, we extracted some common nodes and nested a layer of UI with API calls.
-
-The algorithm department mostly uses Python scripts and SQL nodes, timed with box-table logic, then configured with machine learning algorithms for training, and then called the model in Python to generate model scores after assembling data. We wrapped some Kafka nodes to read Hive data and push it to Kafka via Spark.
-
-### Task Type
-
-<div align=center>
-
-<img src="/img/2022-3-11/Eng/5.png"/>
-
-</div>
-
-DolphinScheduler supports Shell, SQL, Python, and Jar task types. Shell supports Sqoop DataX mr synchronization task and Hive-SQL, Spark-SQL; SQL node mainly supports TiDB SQL (handling upstream sub-base and sub-table monitoring) and Hive SQL. Python task types support offline calls to model scripts, etc.; and Jar packages mainly support Spark Jar offline management.
-
-### Task Scenario
-
-<div align=center>
-
-<img src="/img/2022-3-11/Eng/6.png"/>
-
-</div>
-
-The task scenario of DolphinScheduler is mainly about synchronizing various data sources such as MySQL, Hbase, etc. to Hive, and then generating DW directly through ETL workflow, assembling or calling through Python scripts, generating models and rule results, and then pushing them to Kafka, which will offer risk control system quota, approval, and analysis, and feed the results to the business system. This shows a complete workflow example of the DolphinScheduler scheduling process.
-
-### Operations and Maintenance of DolphinScheduler
-
-Currently, DolphinScheduler is processing 10000+ workflows per day. Considering the fact that many offline reports depend on DolphinScheduler, operation and maintenance is very important.
-
-At 360 DIGITECH, the operation and maintenance of DolphinScheduler are divided into three parts:
-
-* DS Dependent Components Operations and Maintenance
-
-The DolphinScheduler dependent component of DolphinScheduler is mainly for MySQL monitoring and Zookeeper monitoring.
-
-Because workflow definition meta information, workflow instances&task instances, Quartz scheduling, and Commands all rely on MySQL, MySQL monitoring is critical. There was a time when our network in the server room went down causing many workflow instances to miss schedule, and the problems are troubleshoot by the follow-up MySQL monitoring.
-
-The importance of Zookeeper monitoring also goes without saying. The master-worker state and task queue both rely on Zookeeper, fortunately, Zookeeper has been stable and no problems have occurred yet.
-
-* Master and Worker Status Monitoring
-As we all know, the Master is responsible for task slicing, which actually does not have a great impact on the business, so we just use emails to monitor it; however, a hung worker will cause task delays and increase the pressure on the cluster. In addition, if Yarn tasks are killed unsuccessfully, task tolerance may lead to repeated Yarn tasks, so we use phone alerts to monitor worker status.
-
-### Grafana Big Board Monitoring Workflow Instance Status
-
-For this, we have created a Grafana monitoring dashboard for Ops, which can monitor the status of workflow instances in real-time, including the number of workflow instances, the running status of workflow instances by project, and timeout warning settings.
-
-## DolphinScheduler Retrofit
-
-### Experience optimization
-
-1. Authorize individuals to resource files and projects, and resource files distinguish between edit and readable to facilitate authorization.
-
-2. Extend global variables (process definition id, task id, etc. included into global variables), allow tasks submitted to yarn to be tracked to the scheduled tasks, facilitate cluster management, lock resources by counting workflow instances to facilitate maintenance.
-
-3. Carry workflow replication, task instance query interface optimization to speed up query speed, and optimize UI.
-
-### Add SMS alert
-
-To make up for the weakness of the original email alert, we added an SMS alert to the UI to save the workflow definition to ensure the key tasks be monitored properly. In addition, we also changed the alert receivers to user names and extended the AlertType to SMS, email, and other alert methods to associate with user information, such as cell phone numbers, to ensure that we can receive alerts in time when important tasks fail.
-
-### Add maintenance mode to Worker
-
-When a worker machine needs maintenance, it's necessary to ensure no new tasks are submitted to the worker. For this, we have added a maintenance mode to the worker, which consists of four main points.
-
-1. The UI sets the worker into maintenance mode, calling the API to write to the zk path specified by the worker.
-
-2.WorkerServer performs scheduled polling for maintenance mode or not.
-
-3.WorkerServer in maintenance mode, FetchTaskThread not fetching new tasks.
-
-4. The worker task finishes running and can be restarted.
-
-After the above reform, the pressure on our O&M is greatly reduced.
-
-### Multi-server room renovation
-
-Finally, we also performed a multi-server room renovation. Since we had 2 clusters in different server rooms, our goal was to set up multiple server rooms in scheduling, so that when one server room fails, we can switch to others with one click, and use the same scheduling framework in multiple server rooms.
-
-The pointcut for the renovation is to set up multi-server rooms in the scheduling to ensure that a certain task can be started in multiple server rooms. The renovation process went like this:
-
-1. ZK is deployed in each server room, and the Master and Worker are registered to the corresponding server room, the Master is responsible for task slicing, and the Worker is responsible for task processing.
-
-2. Attach schedule and command with datacenter information.
-
-3. To ensure dual-server room task switching, resource files are uploaded for upper room tasks, while changing task interfaces, task dependencies, and Master fault tolerance all need to be modified to match the corresponding room.
diff --git a/blog/en-us/Introducing-Apache-DolphinScheduler-1.3.9.md b/blog/en-us/Introducing-Apache-DolphinScheduler-1.3.9.md
deleted file mode 100644
index 4d0c166..0000000
--- a/blog/en-us/Introducing-Apache-DolphinScheduler-1.3.9.md
+++ /dev/null
@@ -1,83 +0,0 @@
-# Introducing Apache DolphinScheduler 1.3.9, StandaloneServer is Available!
-
-[](https://imgpp.com/image/OQFd4)
-
-On October 22, 2021, we are excited to announce the release of Apache DolphinScheduler 1.3.9. After a month and a half,Apache DolphinScheduler 1.3.9 brings StandaloneServer to users with the joint efforts of the community. StandaloneServer is a major update of this version, which means a huge leap in ease of use, and the details will be introduced below. In addition, this upgrade also fixes two critical bugs in 1.3.8.
-
-## 1.3.9 Download:[1.3.9 Download Link](https://dolphinscheduler.apache.org/en-us/download/download.html)
-
-In 1.3.9, the main updates include:
-
-
-## New Features
-
-
-**[Feature#6480] Add StandaloneServer to make development and operation easier**
-
-StandaloneServer is a service created to allow users to quickly experience the product. The registry and database H2-DataBase and Zk-TestServer are built-in. Users can start StandaloneServer for debugging with only one line of command.
-
-The way to start StandaloneServer with one-line command: switch to a user with sudo permission, and run the script
-
-```plain
-sh ./bin/dolphinscheduler-daemon.sh start standalone-server
-```
-
-It shows that 1.3.9 reduces the configuration cost through built-in components. You only need to configure the jdk environment to start the DolphinScheduler system with one click, thereby improving the efficiency of research and development. For detailed usage documents, please refer to: link to be added.
-
- <img src="/img/login_en.png" width="60%" />
-
-Access the front page address, interface IP (self-modified) [http://localhost:12345/dolphinscheduler](http://localhost:12345/dolphinscheduler), with the default name and password:admin/dolphinscheduler123.
-
-The detailed user docs for Standalone, please refer to:[1.3.9 standalone-server](https://dolphinscheduler.apache.org/en-us/docs/1.3.9/user_doc/standalone-server.html)
-
-## Optimization and Fix
-
-**☆[Fix #6337][Task] Sql limit param no default value**
-
-When the SqlTask is executed, if the limit parameter is not set, the displayed result is empty. Based on this, the default parameters have been added in 1.3.9, and relevant instructions have been made on the log to allow users to track the problem more clearly.
-
-**☆[Bug#6429] [ui] sub_process node open sub_task show empty page #6429**
-
-The problem that the sub_task node is displayed as empty has been fixed.
-
-## Contributor
-
-Thanks to PMC Ke Zhenxu from the SkyWalking community for his contribution to StandaloneServer, Apache DolphinScheduler will persistently optimize the function design and enhance the user experience with the participation of more contributors, so stay tuned.
-
-### 1 DolphinScheduler introduction
-
-Apache DolphinScheduler is a distributed and extensible workflow scheduler platform with powerful DAG visual interfaces, dedicated to solving complex job dependencies in the data pipeline and providing various types of jobs available out of box.
-
-DolphinScheduler assembles Tasks in the form of DAG (Directed Acyclic Graph), which can monitor the running status of tasks in real time. At the same time, it supports operations such as retry, recovery from designated nodes, suspend and Kill tasks, and focuses on the following 6 capabilities :
-
-<img src="https://s1.imgpp.com/2021/10/25/WechatIMG89.md.jpg" width="60%" />
-
-
-## 2 Partial User Cases
-
-According to incomplete statistics, as of October 2020, 600+ companies and institutions have adopted DolphinScheduler in production environments. Partial cases are shown as below (in no particular order).
-
-[](https://imgpp.com/image/OQylI)
-
-## 3 Participate in Contribution
-
-With the prosperity of open source in China, the Apache DolphinScheduler community ushered in vigorous development. In order to make better and easy-to-use scheduling system, we sincerely welcome partners who love open source to join Apache DolphinScheduler Community.
-
-There are many ways to participate in and contribute to the Apache DolphinScheduler Community, including:
-
-* Contribute to the first PR (document, code). We hope it to be simple and a try to get yourself familiar with the submission process and community collaboration.
-* We have compiled a list of issues suitable for novices: [Good First Issues](https://github.com/apache/dolphinscheduler/issues/5689)
-* And a list of issues for non-newbie: [Volunteer Wanted](https://github.com/apache/dolphinscheduler/issues?q=is%3Aopen+is%3Aissue+label%3A%22volunteer+wanted%22)
-* How to participate in the contribution: [Participate in Contributing](https://dolphinscheduler.apache.org/en-us/community/development/contribute.html)
-
-Apache DolphinScheduler Community needs you! Even if a small piece of tile will make a big differnce.
-
-If you are interested in contributing code we created [Good First Issues ](https://github.com/apache/dolphinscheduler/issues/5689) to get you started. If you have any questions about [code](https://github.com/apache/dolphinscheduler), [installation](https://dolphinscheduler.apache.org/en-us/download/download.html), and [docs](https://dolphinscheduler.apache.org/en-us/docs/1.3.9/user_doc/quick-start.html) please do not hesitate to reach out to us on [slack](https://app.slack.com/client/T0 [...]
-
-**Community Official Website**
-
-[https://dolphinscheduler.apache.org/](/)
-
-**Code Warehouse Address**
-
-[https://github.com/apache/dolphinscheduler](https://github.com/apache/dolphinscheduler)
\ No newline at end of file
diff --git a/blog/en-us/Json_Split.md b/blog/en-us/Json_Split.md
deleted file mode 100644
index 9e9c4c4..0000000
--- a/blog/en-us/Json_Split.md
+++ /dev/null
@@ -1,103 +0,0 @@
-## Why did we split the big json that holds the tasks and relationships in the DolphinScheduler workflow definition?
-
-### The Background
-
-Currently DolphinScheduler saves tasks and relationships in process as big json to the process_definition_json field in the process_definiton table in the database. If a process is large, for example, with 1000 tasks, the json field becomes very large and needs to be parsed when using the json, which is very performance intensive and the tasks cannot be reused, so the community plans to start a json splitting project. Encouragingly, we have now completed most of this work, so a summary i [...]
-
-### Summarization
-
-The json split project was started on 2021-01-12 and the main development was initially completed by 2021-04-25. The code has been merged into the dev branch. Thanks to lenboo, JinyLeeChina, simon824 and wen-hemin for coding.
-
-The main changes, as well as the contributions, are as follows:
-
-- Code changes 12793 lines
-- 168 files modified/added
-- 145 Commits in total
-- There were 85 PRs
-
-### Review of the split programme
-
-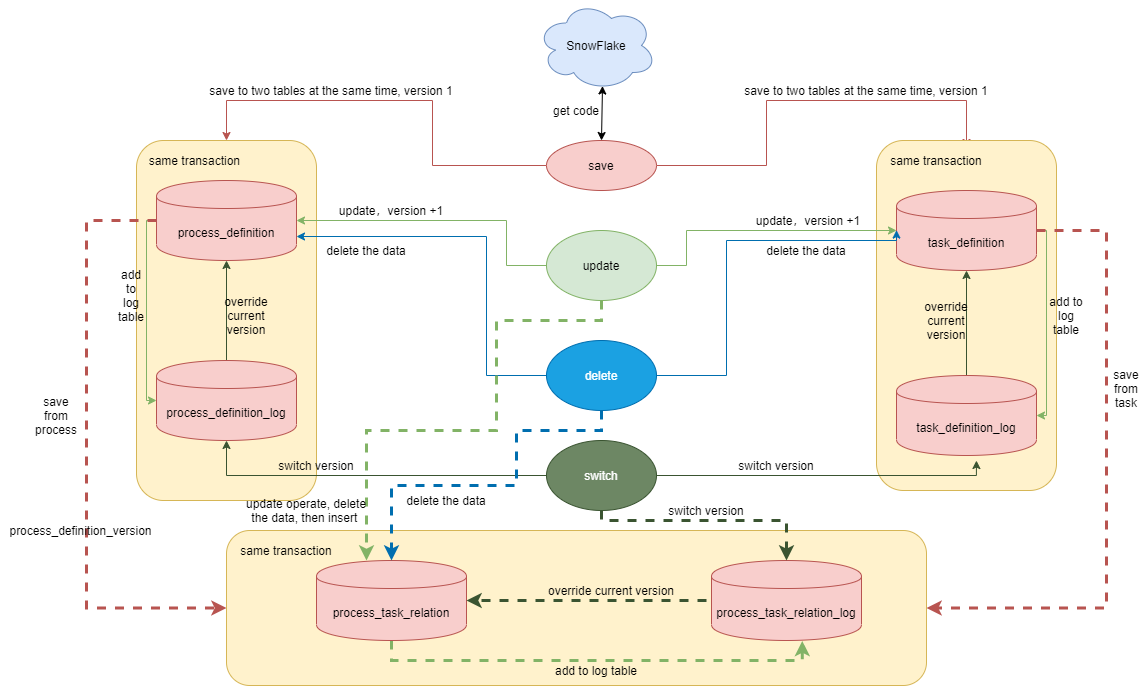
-
-- [ ] When the api module performs a save operation
-
-1. The process definition is saved to process_definition (main table) and process_definition_log (log table), both tables hold the same data and the process definition version is 1
-2. The task definition table is saved to task_definition (main table) and task_definition_log (log table), also saving the same data, with task definition version 1
-3. process task relationships are stored in the process_task_relation (main table) and process_task_relation_log (log table), which holds the code and version of the process, as tasks are organised through the process and the dag is drawn in terms of the process. The current node of the dag is also known by its post_task_code and post_task_version, the predecessor dependency of this node is identified by pre_task_code and pre_task_version, if there is no dependency, the pre_task_code and [...]
-
-- [ ] When the api module performs an update operation, the process definition and task definition update the main table data directly, and the updated data is inserted into the log table. The main table is deleted and then inserted into the new relationship, and the log table is inserted directly into the new relationship.
-- [ ] When the api module performs a delete operation, the process definition, task definition and relationship table are deleted directly from the master table, leaving the log table data unchanged.
-- [ ] When the api module performs a switch operation, the corresponding version data in the log table is overwritten directly into the main table.
-
-### Json Access Solutions
-
-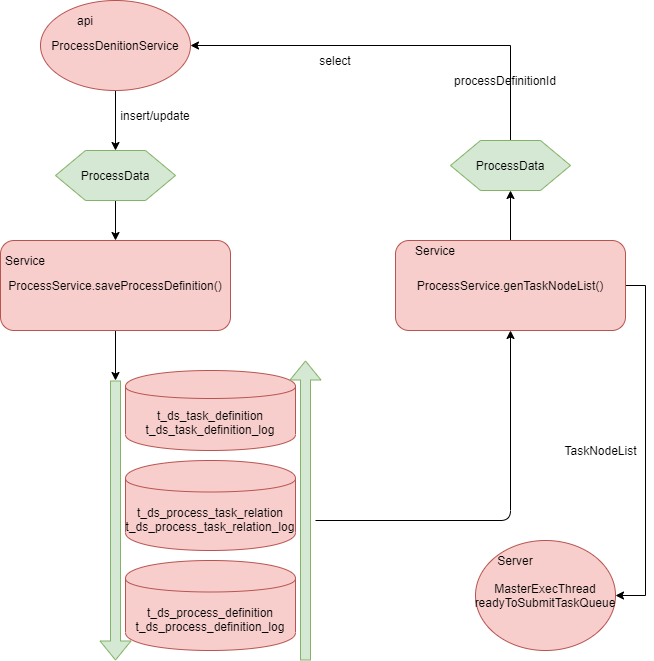
-
-- [ ] In the current phase of the splitting scheme, the api module controller layer remains unchanged and the incoming big json is still mapped to ProcessData objects in the service layer. insert or update operations are done in the public Service module through the ProcessService. saveProcessDefiniton() entry in the public Service module, which saves the database operations in the order of task_definition, process_task_relation, process_definition. When saving, the task is changed if it [...]
-
-- [ ] The data is assembled in the public Service module through the ProcessService.genTaskNodeList() entry, or assembled into a ProcessData object, which in turn generates a json to return
-- [ ] The Server module (Master) also gets the TaskNodeList through the public Service module ProcessService.genTaskNodeList() to generate the dispatch dag, which puts all the information about the current task into the MasterExecThread. readyToSubmitTaskQueue queue in order to generate taskInstance, dispatch to worker
-
-
-
-## Phase 2 Planning
-
-### API / UI module transformation
-
-- [ ] The processDefinition interface requests a back-end replacement for processDefinitonCode via processDefinitionId
-- [ ] Support for separate definition of task, the current task is inserted and modified through the workflow, Phase 2 needs to support separate definition
-- [ ] Frontend and backend controller layer json splitting, Phase 1 has completed the api module service layer to dao json splitting, Phase 2 needs to complete the front-end and controller layer json splitting
-
-### server module retrofit
-
-- [ ] Replace process_definition_id with process_definition_code in t_ds_command and t_ds_error_command、t_ds_schedules
-- [ ] Generating a taskInstance process transformation
-
-The current process_instance is generated from the process_definition and schedules and command tables, while the taskInstance is generated from the MasterExecThread. readyToSubmitTaskQueue queue, and the data in the queue comes from the dag object. At this point, the queue and dag hold all the information about the taskInstance, which is very memory intensive. It can be modified to the following data flow, where the readyToSubmitTaskQueue queue and dag hold the task code and version inf [...]
-
-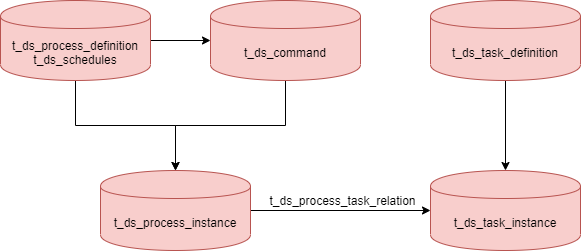
-
----
-
-**Appendix: The snowflake algorithm**
-
-**snowflake:** is an algorithm for generating distributed, drama-wide unique IDs called **snowflake**, which was created by Twitter and used for tweeting IDs.
-
-A Snowflake ID has 64 bits. the first 41 bits are timestamps, representing the number of milliseconds since the selected period. The next 10 bits represent the computer ID to prevent conflicts. The remaining 12 bits represent the serial number of the generated ID on each machine, which allows multiple Snowflake IDs to be created in the same millisecond. snowflakeIDs are generated based on time and can therefore be ordered by time. In addition, the generation time of an ID can be inferred [...]
-
-1. **Structure of the snowflake algorithm:**
-
- 
-
- It is divided into 5 main parts.
-
- 1. is 1 bit: 0, this is meaningless.
- 2. is 41 bits: this represents the timestamp
- 3. is 10 bits: the room id, 0000000000, as 0 is passed in at this point.
- 4. is 12 bits: the serial number, which is the serial number of the ids generated at the same time during the millisecond on a machine in a certain room, 0000 0000 0000.
-
- Next we will explain the four parts:
-
-**1 bit, which is meaningless:**
-
-Because the first bit in binary is a negative number if it is 1, but the ids we generate are all positive, so the first bit is always 0.
-
-**41 bit: This is a timestamp in milliseconds.**
-
-41 bit can represent as many numbers as 2^41 - 1, i.e. it can identify 2 ^ 41 - 1 milliseconds, which translates into 69 years of time.
-
-**10 bit: Record the work machine ID, which represents this service up to 2 ^ 10 machines, which is 1024 machines.**
-
-But in 10 bits 5 bits represent the machine room id and 5 bits represent the machine id, which means up to 2 ^ 5 machine rooms (32 machine rooms), each of which can represent 2 ^ 5 machines (32 machines), which can be split up as you wish, for example by taking out 4 bits to identify the service number and the other 6 bits as the machine number. This can be combined in any way you like.
-
-**12 bit: This is used to record the different ids generated in the same millisecond.**
-
-12 bit can represent the maximum integer of 2 ^ 12 - 1 = 4096, that is, can be distinguished from 4096 different IDs in the same milliseconds with the numbers of the 12 BIT representative. That is, the maximum number of IDs generated by the same machine in the same milliseconds is 4096
-
-In simple terms, if you have a service that wants to generate a globally unique id, you can send a request to a system that has deployed the SnowFlake algorithm to generate the unique id. The SnowFlake algorithm then receives the request and first generates a 64 bit long id using binary bit manipulation, the first bit of the 64 bits being meaningless. This is followed by 41 bits of the current timestamp (in milliseconds), then 10 bits to set the machine id, and finally the last 12 bits [...]
-
-The characteristics of SnowFlake are:
-
-1. the number of milliseconds is at the high end, the self-incrementing sequence is at the low end, and the entire ID is trended incrementally.
-2. it does not rely on third-party systems such as databases, and is deployed as a service for greater stability and performance in generating IDs.
-3. the bit can be allocated according to your business characteristics, very flexible.
diff --git a/blog/en-us/K8s_Cisco_Hangzhou.md b/blog/en-us/K8s_Cisco_Hangzhou.md
deleted file mode 100644
index 64f9ba6..0000000
--- a/blog/en-us/K8s_Cisco_Hangzhou.md
+++ /dev/null
@@ -1,176 +0,0 @@
----
-title:Fully Embracing K8s, Cisco Hangzhou Seeks to Support K8s Tasks Based on ApacheDolphinScheduler
-keywords: Apache,DolphinScheduler,scheduler,big data,ETL,airflow,hadoop,orchestration,dataops,K8s
-description: K8s is the future of the cloud and is the only infrastructure platform that
----
-# Fully Embracing K8s, Cisco Hangzhou Seeks to Support K8s Tasks Based on ApacheDolphinScheduler
-
-<div align=center>
-
-<img src="/img/2022-03-21/1.png"/>
-
-</div>
-
-K8s is the future of the cloud and is the only infrastructure platform that connects public and private clouds, making it the choice of more enterprises to modernize their IT.
-
-
-
-Based on Apache DolphinScheduler, Cisco Hangzhou is also exploring K8s support, and some of the features are already running successfully. Recently, Qian Li, a big data engineer from Cisco Hangzhou, will share their results with us.
-
-<div align=center>
-
-<img src="/img/2022-03-21/2.png"/>
-
-</div>
-
-
-Qian Li
-
-
-
-Big Data Engineer from Cisco Hangzhou, with years of experience in Big Data solutions, Spark, Flink, scheduling system, ETL, and other projects.
-
-
-
-My presentation will be related to these parts: Namespace management, K8s tasks running continuously, workflow scheduling of K8s tasks, and our future planning.
-
-## 01 Namespace Management
-
-### Resource Management
-
-
-
-In the first part, I will first introduce resource management. The purpose of introducing resource management is to use K8s clusters to run tasks that are not part of the Apache DolphinScheduler concept of scheduling, such as Namespace, which are more akin to a data solution that limits resources in a queue if the CPU has limited memory, and enabling some resource isolation.
-
-
-
-In the future, we may merge some of the resource management functionality onto Apache DolphinScheduler.
-
-### Adding, deleting, and maintaining management
-
-We can add some Type, i.e. the type of tag, e.g. some Namespace only allows certain types of jobs to be run. We can count the number of jobs under the Namespace, the number of pods, the number of resources requested, requests, etc. to see the resource usage of the queue, and the interface is only available to the administrator by default.
-
-<div align=center>
-
-<img src="/img/2022-03-21/3.png"/>
-
-</div>
-
-### Multiple K8s clusters
-
-K8s supports multiple clusters, we can connect to multiple K8s clusters via the Apache DolphinScheduler client, batch, PROD, etc to build multiple sets of K8s clusters and support multiple K8s clusters via Namespace.
-
-
-
-We can edit the developed clusters and modify all the properties such as memory.
-
-
-
-In the new version, user permissions are set in user master, authorizing a user to submit tasks to a Namespace and edit resources.
-
-## 02 K8s tasks running continuously
-
-The second section is about the types of tasks we currently support.
-
-### Mirrors that are started without exiting, such as ETL
-
-ETL, for example, is a task that must be done manually before it will exit after being committed. Once a task like this is committed, it sinks the data and theoretically never stops as long as no upgrades.
-
-<div align=center>
-
-<img src="/img/2022-03-21/4.png"/>
-
-</div>
-
-This kind of task may not actually be used for scheduling, as it only has two states, start and stop. So we put it in a live list and made a set of monitors. The POD runs in real-time, interacting mainly through a Fabris operator, and can be dynamically scaled to improve resource utilization.
-
-### Flink tasks
-
-We can manage the CPU down to 0.01%, making full use of the K8s virtual CPU.
-
-<div align=center>
-
-<img src="/img/2022-03-21/5.png"/>
-
-</div>
-
-<div align=center>
-
-<img src="/img/2022-03-21/6.png"/>
-
-</div>
-
-<div align=center>
-
-<img src="/img/2022-03-21/7.png"/>
-
-</div>
-
-We also use Flink Tasks, an ETL-based extension that includes an interface for editing, viewing status, going online, going offline, deleting, execution history, and monitoring. We designed the Flink UI using a proxy model and developed permission controls to prevent external parties from modifying it.
-
-Flink starts by default based on a checkpoint, or can be created at a specified time, or submitted and started based on the last checkpoint.
-
-Flink tasks support multiple mirror versions, as K8s is inherently mirror-running, you can specify mirrors directly to choose a package, or via the file to submit the task.
-
-Also, Batch type tasks may be run once and finished or may be scheduled on a cycle basis and exit automatically after execution, which is not quite the same as Flink, so for this type of task, we would deal it based on Apache DolphinScheduler.
-
-## 03 Running K8s tasks
-
-### Workflow scheduling for K8s tasks
-
-We added some Flink batch and Spark batch tasks at the bottom and added some configurations such as the resources used, the namespace to be run, and so on. The image information can be started with some custom parameters, and when wrapped up it is equivalent to the plugin model, which is perfectly extended by Apache DolphinScheduler.
-
-<div align=center>
-
-<img src="/img/2022-03-21/8.png"/>
-
-</div>
-
-### Spark Tasks
-
-Under Spark tasks, you can view information such as CPU, files upload supports Spark Jar packages, or can be configured separately.
-
-<div align=center>
-
-<img src="/img/2022-03-21/9.png"/>
-
-</div>
-
-This multi-threaded upper layer can dramatically increase processing speed.
-
-## 04 Others and Delineation
-
-### Watch Status
-
-<div align=center>
-
-<img src="/img/2022-03-21/10.jpeg"/>
-
-</div>
-
-In addition to the above changes, we have also optimized the task run state.
-
-
-
-When a task is submitted, the runtime may fail and even the parallelism of the task may change based on certain policies in the real run state. In this case, we need to watch and fetch the task status in real-time and synchronize it with the Apache DolphinScheduler system to ensure that the status seen in the interface is always accurate.
-
-
-
-For batch, we can treat it with or without the watch, as it is not a standalone task that requires fully watch and the namespace resource usage is based on watch mode so that the state is always accurate.
-
-### Multiple environments
-
-Multi-environment means that the same task can be pushed to different K8s clusters, such as a Flink task.
-
-
-
-In terms of code, there are two ways to do watch, one is to put some pods separately, for example when using the K8s module, define information about multiple K8s clusters, create some watch pods on each cluster to watch the status of tasks in the cluster and do some proxy functions. Another option is to follow the API or a separate service and start a watch service to track all K8s clusters. However, this does not allow you to do proxying outside of the K8s internal and external networks.
-
-
-
-There are several options to watch Batch, one of them is by synchronization based on Apache DolphinScheduler, which is more compatible with the latter. We may submit a PR on this work in the future soon. Spark uses the same model, providing a number of pods to interact with, and the internal code we use is the Fabric K8s client.
-
-
-
-Going forward, we will be working with Apache DolphinScheduler to support the features discussed here and share more information about our progress. Thank you all!
-
diff --git a/blog/en-us/Lizhi-case-study.md b/blog/en-us/Lizhi-case-study.md
deleted file mode 100644
index 0ede05b..0000000
--- a/blog/en-us/Lizhi-case-study.md
+++ /dev/null
@@ -1,112 +0,0 @@
-# A Formidable Combination of Lizhi Machine Learning Platform& DolphinScheduler Creates New Paradigm for Data Process in the Future
-
->Editor's word: The online audio industry is a blue ocean market in China nowadays. According to CIC data, the market size of China’s online audio industry has grown from 1.6 billion yuan in 2016 to 13.1 billion yuan in 2020, with a compound annual growth rate of 69.4%. With the popularity of the Internet of Things, audio has permeated into various terminals mobiles, vehicles, smart hardware, home equipment, and other various scenarios, sequentially to maximize the accompanying advantage [...]
-
->In recent years, domestic audio communities have successfully been listed one after another. Among them, Lizhi was listed on NASDAQ in 2020 as the first “online audio” stock, which has more than 200 million users at present. In the information age, the audio industry also faces the generation of massive UGC data on the platform. On one hand, the users and consumers of audio content expect high-efficiency information transmission; on the other hand, the internet audio platforms hope that [...]
-
-## Background
-
-<div align=center>
-<img src="https://s1.imgpp.com/2021/11/23/radio-g360707f44_1920.md.jpg"/>
-</div>
-
-Lizhi is a fast-growing UGC audio community company that attaches great importance to AI and data analysis technology development. AI can find the right voice for each user among the massively fragmented audios, and build it into a sustainable ecological closed loop. And data analysis can guide the company’s fast-growing business. Both of the two fields need to process massive amounts of data and require a big data scheduling system.
-
-Before the beginning of 2020, Lizhi used the Azkaban scheduling system. Although the big data scheduling SQL/shell/python scripts and other big data-related modules can complete the entire AI process, it is not easy and reusable enough. The machine learning platform is a set of systems built specifically for AI, which abstracts the AI development paradigm, i.e. data acquisition, data preprocessing, model training, model prediction, model evaluation, and model release into modules. Each m [...]
-## Challenges During Machine Learning Platform Development
-During the machine learning platform development, Lizhi has some clear requirements for the scheduling system:
-
-1. It should be able to store and calculate massive data, such as screening samples, generating portraits, feature preprocessing, distributed model training, etc.;
-
-2. The DAG execution engine is required, and the processes such as data acquisition -> data preprocessing -> model training -> model prediction -> model evaluation -> model release should be executed in series with DAG.
-
-In the development by Azkaban, the team encountered some challenges:
-
-Challenge 1: The development model is cumbersome, which requires the user to package scripts and build DAG dependencies, and there is no implementation of DAG drag and drop;
-
-Challenge 2: The modules are not rich enough, and the scripted AI modules are not universal enough, thus the team need to develop modules repeatedly, which is unnecessary and error-prone;
-
-Challenge 3: Stand-alone deployment, the system is unstable and prone to failures. Besides, Task jams can easily cause downstream tasks to fail.
-
-## Turn to DolphinScheduler
-
-After stepping on numerous pits in the old system, the Lizhi machine learning team, including recommendation system engineers Haibin Yu, Yanbin Lin, Huanjie Xie, and Yifei Guo, decided to adopt DolphinScheduler.
-
-Currently, 1,600+ processes and 12,000+ tasks (IDC) are running smoothly on DolphinScheduler every day.
-
-Haibin Yu said that the majority of the users of the scheduling system are recommendation algorithms engineers> data analysts> risk control algorithms engineers> business developers (importance decreased in order). Not all of them are masters of data management operations, and DolphinScheduler perfectly meets their needs for a simple, easy-to-use, drag-and-drop scheduling system:
-
-1. Distributed decentralized architecture and fault tolerance mechanism to ensure the high availability of the system;
-
-2. Visual DAG drag-and-drop UI, easy to use, and iterates quickly;
-
-3. It supports various modules, and can simply develop and integrate its modules;
-
-4. The community is active, hence there are no worries about the project supports;
-
-5. It is very close to the operating mode of the machine learning platform, using DAG to drag and drop UI programming.
-
-## Use Case
-
-After selecting the DolphinScheduler, the Lizhi machine learning platform carries out re-development based on it and applies the achievements to actual business scenarios, which are mainly about recommendation and risk control. Recommendation scenarios cover recommendation of voice, anchor, live broadcast, podcast, friend, etc., and risk control scenarios cover risk control in payment, advertising, and comment, etc.
-At the technical level of the platform, Lizhi optimizes the extended modules for the five paradigms of machine learning, i.e. obtaining training samples, data preprocessing, model training, model evaluation, and model release.
-
-A simple xgboost case:
-
-<div align=center>
-<img src="https://s1.imgpp.com/2021/11/23/1.png"/>
-</div>
-
-### 1. Obtaining training samples
-
-At present, Lizhi does not directly select data from Hive, and joins the union, splitting the sample afterward, but directly processes the sample by shell nodes.
-
-<div align=center>
-<img src="https://s1.imgpp.com/2021/11/23/2.png"/>
-</div>
-
-### 2. Data preprocessing
-
-Transformer& custom preprocessing configuration file, use the same configuration for online training, and feature preprocessing is performed after the feature is obtained. It contains the itemType and its feature set to be predicted, the user’s userType and its feature set, as well as the associated and crossed itemType and its feature set. Define the transformer function for each feature preprocessing, supports custom transformer and hot update, xgboost, and tf model feature preprocessi [...]
-
-<div align=center>
-<img src="https://s1.imgpp.com/2021/11/23/2.png"/>
-</div>
-
-
-### 3. Xgboost training
-
-It supports w2v, xgboost, tf model training modules. The training modules are first packaged with TensorFlow or PyTorch and then packaged into DolphinScheduler modules.
-For example, in the xgboost training process, use Python to package the xgboost training script into the xgboost training node of DolphinScheduler, and show the parameters required for training on the interface. The file exported by “training set data preprocessing” is input to the training node through HDFS.
-
-<div align=center>
-<img src="https://s1.imgpp.com/2021/11/23/3.png"/>
-</div>
-
-### 4. Model release
-
-
-The release model will send the model and preprocessing configuration files to HDFS and insert records into the model release table. The model service will automatically identify the new model, update the model, and provide online prediction services to the external.
-
-<div align=center>
-<img src="https://s1.imgpp.com/2021/11/23/4.png"/>
-</div>
-
-
-Haibin Yu said that due to historical and technical limitations, Lizhi has not yet built a machine learning platform like Ali PAI, but the practice has proved that similar platform functions can be achieved based on DolphinScheduler.
-
-In addition, Lizhi has also carried out many re-developments based on DolphinScheduler to make the scheduling system more in line with actual business needs, such as:
-
-1. Pop-up the window of whether to set timing when defining the workflow
-
-2. Add display pages for all workflow definitions to facilitate searching
-
- a) Add the workflow definition filter and jump to the workflow instance page, and use a line chart to show the change of its running time
- b) The workflow instance continues to dive to the task instance
-
-3. Enter parameters during runtime to configure the disabled task nodes
-
-## Machine Learning Platform based on Scheduling System May Lead the Future Trend
-
-Deep learning is a leading trend in the future. Lizhi has developed new modules for deep learning models. The entire tf process has been completed yet, and LR and GBDT model-related modules are also in the plan. The latter two deep learning models are relatively more simple, easier to get started, faster to iterate, and can be used in generally recommended scenarios. After implementation, the Lizhi machine learning platform can be more complete.
-Lizhi believes that if the scheduling system can be improved in terms of kernel stability, drag-and-drop UI support, convenient modules' expansion, task plug-in, and task parameter transfer, building the machine learning platform based on the scheduling system may become a common practice in the industry.
diff --git a/blog/en-us/Meetup_2022_02_26.md b/blog/en-us/Meetup_2022_02_26.md
deleted file mode 100644
index 787213d..0000000
--- a/blog/en-us/Meetup_2022_02_26.md
+++ /dev/null
@@ -1,77 +0,0 @@
----
-title: # Sign Up to Apache DolphinScheduler Meetup Online | We Are Waiting For You to Join the Grand Gathering on 2.26 2022!
-keywords: Apache,DolphinScheduler,scheduler,big data,ETL,airflow,hadoop,orchestration,dataops,Meetup
-description: Hello the community! After having adjusted ourselves from the pleasure of Spring Festival and re-devote to the busywork,
----
-# Sign Up to Apache DolphinScheduler Meetup Online | We Are Waiting For You to Join the Grand Gathering on 2.26 2022!
-
-<div align=center>
-<img src="/img/2022-02-26/08.png"/>
-</div>
-
-Hello the community! After having adjusted ourselves from the pleasure of Spring Festival and re-devote to the busywork, study, and life, Apache DolphinScheduler is here to wish everyone a prosperous and successful journey in the Lunar year of the Tiger.
-
-In this early spring, Apache DolphinScheduler's first Meetup in 2022 is also coming soon. I believe that the community has already been looking forward to it!
-
-In this Meetup, four guests from our user companies will share their experience when using Apache DolphinScheduler. Whether you are a user of Apache DolphinScheduler or a spectator, you can definitely learn a lot from it.
-
-We are looking forward to more friends joining us in the future, and more and more users will share practical experiences with each other.
-
-The first show of Apache DolphinScheduler in February 2022 will be broadcast on time at 14:00 pm on February 26th.
-
-You can also click https://www.slidestalk.com/m/679 to go directly to the live broadcast reservation interface!
-
-
-
-## 1. Introduction to the Event
-
-Topic: Apache DolphinScheduler user practice sharing
-
-Time: 2022-2-26 14:00-17:00(Beijing Time)
-
-Format: Live online
-
-[Click https://www.slidestalk.com/m/679](https://www.slidestalk.com/m/679) **to register.**
-
-**Click** [https://asf-dolphinscheduler.slack.com/](https://asf-dolphinscheduler.slack.com/) **to join the community on Slack**
-
-## 2. Event Highlights
-
-In this meetup, we invite four big data engineers from 360 Digital Technology, CISCO (China) and Tujia to share their development experiences based on Apache DolphinScheduler, which is highly representative and typical. The topics they shared with us refer to the exploration and application on the K8S cluster and the transformation of the Alert module, etc., which are of great significance to solve the difficulties you may encounter in scenario application. We hope these technical exchan [...]
-
-## 3. Event Agenda
-
-<div align=center>
-<img src="/img/2022-02-26/Schedule.png"/>
-</div>
-
-
-## 4. Topic introduction
-
-Jianmin Liu/ 360 Digital Technology/ Big Data Engineer
-
-**Speech Topic: Practice on Apache DolphinScheduler in 360 Digital Technology**
-
-**Speech Outline:** The evolution of big data scheduling from Azkaban to DS, and the use and transformation of Apache DolphinScheduler.
-
-Qingwang Li/CISCO (China) /Big Data Engineer
-
-**Speech Topic: Retrofit of Apache DolphinScheduler Alert module**
-
-**Speech outline:** The exploration of switching to Apache DolphinScheduler, and modifying the Alert module of Apache DolphinScheduler for different alarm scenarios.
-
-
-Xuchao Zan/Tujia/Big Data Engineer
-
-**Speech topic: Exploration and Application on Apache DolphinScheduler**
-
-**Speech outline:** Tujia introduced Apache DolphinScheduler, built Tujia data scheduling system, and completed the access of data services, including offline tables, emails, and data synchronization. The function development on the scheduling system will be introduced in detail.
-
-Qian Liu/Cisco(China)/Big Data Engineer
-
-**Speech Topic: Exploration and Application of Apache DolphinScheduler on K8S Cluster**
-
-**Speech outline:** The exploration of switching to Apache DolphinScheduler, and submitting tasks to k8s based on DS secondary development support. Currently, tasks such as mirroring, Spark, Flink, etc. can be run on our scheduling system, and the exploration of log monitoring and alarming will also be introduced.
-
-We will see you at 14:00 on February 26th!
-
diff --git a/blog/en-us/Twos.md b/blog/en-us/Twos.md
deleted file mode 100644
index ce03129..0000000
--- a/blog/en-us/Twos.md
+++ /dev/null
@@ -1,34 +0,0 @@
----
-title: Congratulations! Apache DolphinScheduler Has Been Approved As A TWOS Candidate Member
-keywords: Apache,DolphinScheduler,scheduler,big data,ETL,airflow,hadoop,orchestration,dataops,TWOS
-description: Recently, TWOS officially announced the approval of 6 full members and 3 candidate members,
----
-## Congratulations! Apache DolphinScheduler Has Been Approved As A TWOS Candidate Member
-
-<div align=center>
-<img src="https://s1.imgpp.com/2022/01/10/1641804549068.png"/>
-</div>
-
-Recently, TWOS officially announced the approval of 6 full members and 3 candidate members, Apache DolphinScheduler, a cloud-native distributed big data scheduler, was listed by TWOS.
-
-Apache DolphinScheduler is a new-generation workflow scheduling platform that is distributed and easy to expand. It is committed to "solving the intricate dependencies among big data tasks and visualizing the entire data processing". Its powerful visual DAG interface greatly improves the user experience and can configure workflow without complex code.
-
-Since it was officially open-sourced in April 2019, Apache DolphinScheduler (formerly known as EasyScheduler) has undergone several architectural evolutions. So far, the relevant open-source codes have accumulated 7100+ Stars, with 280+ experienced code contributors, 110+ non-code contributors participating in the project, which includes PMCs and Committers of other Apache top-level projects. The Apache DolphinScheduler open source community continues to grow, and the WeChat user group h [...]
-
-## TWOS
-
-At the "2021 OSCAR Open Source Industry Conference", China Academy of Telecommunication Research of MIIT (CAICT) officially established TWOS. TWOS is composed of open-source projects and open-source communities, which aims to guide the establishment of a healthy, credible, sustainable open source community, and build a communication platform providing a complete set of open source risk monitoring and ecological monitoring services.
-
-To help enterprises reduce the risk of using open source software and promote the establishment of a credible open source ecosystem, CAICT has created a credible open-source standard system, which carries authoritative evaluation on enterprise open source governance capabilities, open-source project compliance, open-source community maturity, open-source tool detection capabilities, Open- source risk management capabilities of commercial products.
-
-After being screened by TWOS evaluation criteria, Apache DolphinScheduler was approved to be a candidate member, which shows its recognition of Apache DolphinScheduler's way of open-source operation, maturity, and contribution, and encourages the community to keep active.
-
-On September 17, 2021, the first batch of members joined TWOS, including 25 full members such as openEuler, openGauss, MindSpore, openLookeng, etc., and 27 candidate members like Apache RocketMQ, Dcloud, Fluid, FastReID, etc., with a total of 52 members:
-
-<div align=center>
-<img src="https://s1.imgpp.com/2022/01/10/1.png"/>
-</div>
-
-Only two communities were selected for the second batch of candidate members—Apache DolphinScheduler and PolarDB, an open-source cloud-native ecological distributed database contributed by Alibaba Cloud.
-
-The Apache DolphinScheduler community is very honored to be selected as a candidate member of TWOS, which is an affirmation and incentive for the entire industry to build the community a better place. The community will make persistent efforts and strive to become a full member as soon as possible., and provide more value for China's open-source ecological construction together, with all the TWOS members!
\ No newline at end of file
diff --git a/blog/en-us/YouZan-case-study.md b/blog/en-us/YouZan-case-study.md
deleted file mode 100644
index dcc118f..0000000
--- a/blog/en-us/YouZan-case-study.md
+++ /dev/null
@@ -1,387 +0,0 @@
-# From Airflow to Apache DolphinScheduler, the Roadmap of Scheduling System On Youzan Big Data Development Platform
-
-<div align=center>
-<img src="https://s1.imgpp.com/2021/12/16/1639383815755.png"/>
-</div>
-
-At the recent Apache DolphinScheduler Meetup 2021, Zheqi Song, the Director of Youzan Big Data Development Platform
-shared the design scheme and production environment practice of its scheduling system migration from Airflow to Apache
-DolphinScheduler.
-
-This post-90s young man from Hangzhou, Zhejiang Province joined Youzan in September 2019, where he is engaged in the
-research and development of data development platforms, scheduling systems, and data synchronization modules. When he
-first joined, Youzan used Airflow, which is also an Apache open source project, but after research and production
-environment testing, Youzan decided to switch to DolphinScheduler.
-
-How does the Youzan big data development platform use the scheduling system? Why did Youzan decide to switch to Apache
-DolphinScheduler? The message below will uncover the truth.
-
-## Youzan Big Data Development Platform(DP)
-
-As a retail technology SaaS service provider, Youzan is aimed to help online merchants open stores, build data products
-and digital solutions through social marketing and expand the omnichannel retail business, and provide better SaaS
-capabilities for driving merchants' digital growth.
-
-At present, Youzan has established a relatively complete digital product matrix with the support of the data center:
-
-<div align=center>
-<img src="https://s1.imgpp.com/2021/12/16/1_Jjgx5qQfjo559_oaJP-DAQ.png"/>
-</div>
-
-Youzan has established a big data development platform (hereinafter referred to as DP platform) to support the
-increasing demand for data processing services. This is a big data offline development platform that provides users with
-the environment, tools, and data needed for the big data tasks development.
-
-<div align=center>
-<img src="https://s1.imgpp.com/2021/12/16/1_G9znZGQ1XBhJva0tjWa6Bg.png"/>
-</div>
-
-Youzan Big Data Development Platform Architecture
-
-Youzan Big Data Development Platform is mainly composed of five modules: basic component layer, task component layer,
-scheduling layer, service layer, and monitoring layer. Among them, the service layer is mainly responsible for the job
-life cycle management, and the basic component layer and the task component layer mainly include the basic environment
-such as middleware and big data components that the big data development platform depends on. The service deployment of
-the DP platform mainly adopts the master-slave mode, and the master node supports HA. The scheduling layer is
-re-developed based on Airflow, and the monitoring layer performs comprehensive monitoring and early warning of the
-scheduling cluster.
-
-### 1 Scheduling layer architecture design
-
-<div align=center>
-<img src="https://s1.imgpp.com/2021/12/16/1_UDNCmMrZtcswj62aqNXA1g.png"/>
-</div>
-
-Youzan Big Data Development Platform Scheduling Layer Architecture Design
-
-In 2017, our team investigated the mainstream scheduling systems, and finally adopted Airflow (1.7) as the task
-scheduling module of DP. In the design of architecture, we adopted the deployment plan of Airflow + Celery + Redis +
-MySQL based on actual business scenario demand, with Redis as the dispatch queue, and implemented distributed deployment
-of any number of workers through Celery.
-
-In the HA design of the scheduling node, it is well known that Airflow has a single point problem on the scheduled node.
-To achieve high availability of scheduling, the DP platform uses the Airflow Scheduler Failover Controller, an
-open-source component, and adds a Standby node that will periodically monitor the health of the Active node. Once the
-Active node is found to be unavailable, Standby is switched to Active to ensure the high availability of the schedule.
-
-### 2 Worker nodes load balancing strategy
-
-In addition, to use resources more effectively, the DP platform distinguishes task types based on CPU-intensive
-degree/memory-intensive degree and configures different slots for different celery queues to ensure that each machine's
-CPU/memory usage rate is maintained within a reasonable range.
-
-## Scheduling System Upgrade and Selection
-
-Since the official launch of the Youzan Big Data Platform 1.0 in 2017, we have completed 100% of the data warehouse
-migration plan in 2018. In 2019, the daily scheduling task volume has reached 30,000+ and has grown to 60,000+ by 2021.
-the platform’s daily scheduling task volume will be reached. With the rapid increase in the number of tasks, DP's
-scheduling system also faces many challenges and problems.
-
-### 1 Pain points of Airflow
-
-1. In-depth re-development is difficult, the commercial version is separated from the community, and costs relatively
- high to upgrade ;
-2. Based on the Python technology stack, the maintenance and iteration cost higher;
-3. Performance issues:
-
-<div align=center>
-<img src="https://s1.imgpp.com/2021/12/16/1_U33OWzzfw2Dqn3ryCNbSvw.png"/>
-</div>
-
-Airflow's schedule loop, as shown in the figure above, is essentially the loading and analysis of DAG and generates DAG
-round instances to perform task scheduling. Before Airflow 2.0, the DAG was scanned and parsed into the database by a
-single point. It leads to a large delay (over the scanning frequency, even to 60s-70s) for the scheduler loop to scan
-the Dag folder once the number of Dags was largely due to business growth. This seriously reduces the scheduling
-performance.
-
-4. Stability issues:
-
-The Airflow Scheduler Failover Controller is essentially run by a master-slave mode. The standby node judges whether to
-switch by monitoring whether the active process is alive or not. If it encounters a deadlock blocking the process
-before, it will be ignored, which will lead to scheduling failure. After similar problems occurred in the production
-environment, we found the problem after troubleshooting. Although Airflow version 1.10 has fixed this problem, this
-problem will exist in the master-slave mode, and cannot be ignored in the production environment.
-
-Taking into account the above pain points, we decided to re-select the scheduling system for the DP platform.
-
-In the process of research and comparison, Apache DolphinScheduler entered our field of vision. Also to be Apache's top
-open-source scheduling component project, we have made a comprehensive comparison between the original scheduling system
-and DolphinScheduler from the perspectives of performance, deployment, functionality, stability, and availability, and
-community ecology.
-
-This is the comparative analysis result below:
-
-<div align=center>
-<img src="https://s1.imgpp.com/2021/12/16/1_Rbr05klPmQIc7WPFNeEH-w.png"/>
-</div>
-
-<div align=center>
-<img src="https://s1.imgpp.com/2021/12/16/1_Ity1QoRL_Yu5aDVClY9AgA.png"/>
-</div>
-
-Airflow VS DolphinScheduler
-
-### 1 DolphinScheduler valuation
-
-<div align=center>
-<img src="https://s1.imgpp.com/2021/12/16/1_o8c1Y1TFAOis3KozzJnvfA.png"/>
-</div>
-
-As shown in the figure above, after evaluating, we found that the throughput performance of DolphinScheduler is twice
-that of the original scheduling system under the same conditions. And we have heard that the performance of
-DolphinScheduler will greatly be improved after version 2.0, this news greatly excites us.
-
-In addition, at the deployment level, the Java technology stack adopted by DolphinScheduler is conducive to the
-standardized deployment process of ops, simplifies the release process, liberates operation and maintenance manpower,
-and supports Kubernetes and Docker deployment with stronger scalability.
-
-In terms of new features, DolphinScheduler has a more flexible task-dependent configuration, to which we attach much
-importance, and the granularity of time configuration is refined to the hour, day, week, and month. In addition,
-DolphinScheduler's scheduling management interface is easier to use and supports worker group isolation. As a
-distributed scheduling, the overall scheduling capability of DolphinScheduler grows linearly with the scale of the
-cluster, and with the release of new feature task plug-ins, the task-type customization is also going to be attractive
-character.
-
-From the perspective of stability and availability, DolphinScheduler achieves high reliability and high scalability, the
-decentralized multi-Master multi-Worker design architecture supports dynamic online and offline services and has
-stronger self-fault tolerance and adjustment capabilities.
-
-
-And also importantly, after months of communication, we found that the DolphinScheduler community is highly active, with
-frequent technical exchanges, detailed technical documents outputs, and fast version iteration.
-
-
-In summary, we decided to switch to DolphinScheduler.
-
-## DolphinScheduler Migration Scheme Design
-
-After deciding to migrate to DolphinScheduler, we sorted out the platform's requirements for the transformation of the
-new scheduling system.
-
-In conclusion, the key requirements are as below:
-
-1. Users are not aware of migration. There are 700-800 users on the platform, we hope that the user switching cost can
- be reduced;
-2. The scheduling system can be dynamically switched because the production environment requires stability above all
- else. The online grayscale test will be performed during the online period, we hope that the scheduling system can be
- dynamically switched based on the granularity of the workflow;
-3. The workflow configuration for testing and publishing needs to be isolated. Currently, we have two sets of
- configuration files for task testing and publishing that are maintained through GitHub. Online scheduling task
- configuration needs to ensure the accuracy and stability of the data, so two sets of environments are required for
- isolation.
-
-In response to the above three points, we have redesigned the architecture.
-
-
-### 1 Architecture design
-
-1. Keep the existing front-end interface and DP API;
-2. Refactoring the scheduling management interface, which was originally embedded in the Airflow interface, and will be
- rebuilt based on DolphinScheduler in the future;
-3. Task lifecycle management/scheduling management and other operations interact through the DolphinScheduler API;
-4. Use the Project mechanism to redundantly configure the workflow to achieve configuration isolation for testing and
- release.
-
-<div align=center>
-<img src="https://s1.imgpp.com/2021/12/16/1_eusVhW4QAJ2uO-J96bqiFg.png"/>
-</div>
-
-Refactoring Design
-
-
-We entered the transformation phase after the architecture design is completed. We have transformed DolphinScheduler's
-workflow definition, task execution process, and workflow release process, and have made some key functions to
-complement it.
-
-
-- Workflow definition status combing
-
-<div align=center>
-<img src="https://s1.imgpp.com/2021/12/16/-1.png"/>
-</div>
-
-We first combed the definition status of the DolphinScheduler workflow. The definition and timing management of
-DolphinScheduler work will be divided into online and offline status, while the status of the two on the DP platform is
-unified, so in the task test and workflow release process, the process series from DP to DolphinScheduler needs to be
-modified accordingly.
-
-- Task execution process transformation
-
-Firstly, we have changed the task test process. After switching to DolphinScheduler, all interactions are based on the
-DolphinScheduler API. When the task test is started on DP, the corresponding workflow definition configuration will be
-generated on the DolphinScheduler. After going online, the task will be run and the DolphinScheduler log will be called
-to view the results and obtain log running information in real-time.
-
-<div align=center>
-<img src="https://s1.imgpp.com/2021/12/16/-1.png"/>
-</div>
-<div align=center>
-<img src="https://s1.imgpp.com/2021/12/16/-3.png"/>
-</div>
-- Workflow release process transformation
-
-Secondly, for the workflow online process, after switching to DolphinScheduler, the main change is to synchronize the
-workflow definition configuration and timing configuration, as well as the online status.
-
-<div align=center>
-<img src="https://s1.imgpp.com/2021/12/16/1_4-ikFp_jJ44-YWJcGNioOg.png"/>
-</div>
-<div align=center>
-<img src="https://s1.imgpp.com/2021/12/16/-5.png"/>
-</div>
-The original data maintenance and configuration synchronization of the workflow is managed based on the DP master, and
-only when the task is online and running will it interact with the scheduling system. Based on these two core changes,
-the DP platform can dynamically switch systems under the workflow, and greatly facilitate the subsequent online
-grayscale test.
-
-### 2 Function completion
-
-
-In addition, the DP platform has also complemented some functions. The first is the adaptation of task types.
-
-
-- Task type adaptation
-
-Currently, the task types supported by the DolphinScheduler platform mainly include data synchronization and data
-calculation tasks, such as Hive SQL tasks, DataX tasks, and Spark tasks. Because the original data information of the
-task is maintained on the DP, the docking scheme of the DP platform is to build a task configuration mapping module in
-the DP master, map the task information maintained by the DP to the task on DP, and then use the API call of
-DolphinScheduler to transfer task configuration information.
-
-<div align=center>
-<img src="https://s1.imgpp.com/2021/12/16/1_A76iOa5LKyPiu-NoopmYrA.png"/>
-</div>
-
-Because some of the task types are already supported by DolphinScheduler, it is only necessary to customize the
-corresponding task modules of DolphinScheduler to meet the actual usage scenario needs of the DP platform. For the task
-types not supported by DolphinScheduler, such as Kylin tasks, algorithm training tasks, DataY tasks, etc., the DP
-platform also plans to complete it with the plug-in capabilities of DolphinScheduler 2.0.
-
-### 3 Transformation schedule
-
-Because SQL tasks and synchronization tasks on the DP platform account for about 80% of the total tasks, the
-transformation focuses on these task types. At present, the adaptation and transformation of Hive SQL tasks, DataX
-tasks, and script tasks adaptation have been completed.
-
-<div align=center>
-<img src="https://s1.imgpp.com/2021/12/16/1_y7HUfYyLs9NxnTzENKGSCA.png"/>
-</div>
-### 4 Function complement
-
-- Catchup mechanism realizes automatic replenishment
-
-DP also needs a core capability in the actual production environment, that is, Catchup-based automatic replenishment and
-global replenishment capabilities.
-
-The catchup mechanism will play a role when the scheduling system is abnormal or resources is insufficient, causing some
-tasks to miss the currently scheduled trigger time. When the scheduling is resumed, Catchup will automatically fill in
-the untriggered scheduling execution plan.
-
-The following three pictures show the instance of an hour-level workflow scheduling execution.
-
-In Figure 1, the workflow is called up on time at 6 o'clock and tuned up once an hour. You can see that the task is
-called up on time at 6 o'clock and the task execution is completed. The current state is also normal.
-
-<div align=center>
-<img src="https://s1.imgpp.com/2021/12/16/1_MvQGZ-FKKLMvKrlWihXHgg.png"/>
-</div>
-
-figure 1
-
-Figure 2 shows that the scheduling system was abnormal at 8 o'clock, causing the workflow not to be activated at 7
-o'clock and 8 o'clock.
-
-<div align=center>
-<img src="https://s1.imgpp.com/2021/12/16/1_1WxLOtd1Oh2YERmtGcRb0Q.png"/>
-</div>
-figure 2
-
-Figure 3 shows that when the scheduling is resumed at 9 o'clock, thanks to the Catchup mechanism, the scheduling system
-can automatically replenish the previously lost execution plan to realize the automatic replenishment of the scheduling.
-
-<div align=center>
-<img src="https://s1.imgpp.com/2021/12/16/126ec1039f7aa614c.png"/>
-</div>
-
-Figure 3
-
-This mechanism is particularly effective when the amount of tasks is large. When the scheduled node is abnormal or the
-core task accumulation causes the workflow to miss the scheduled trigger time, due to the system's fault-tolerant
-mechanism can support automatic replenishment of scheduled tasks, there is no need to replenish and re-run manually.
-
-At the same time, this mechanism is also applied to DP's global complement.
-
-- Global Complement across Dags
-
-<div align=center>
-<img src="https://s1.imgpp.com/2021/12/16/1_eVyyABTQCLeSGzbbuizfDA.png"/>
-</div>
-
-DP platform cross-Dag global complement process
-
-The main use scenario of global complements in Youzan is when there is an abnormality in the output of the core upstream
-table, which results in abnormal data display in downstream businesses. In this case, the system generally needs to
-quickly rerun all task instances under the entire data link.
-
-Based on the function of Clear, the DP platform is currently able to obtain certain nodes and all downstream instances
-under the current scheduling cycle through analysis of the original data, and then to filter some instances that do not
-need to be rerun through the rule pruning strategy. After obtaining these lists, start the clear downstream clear task
-instance function, and then use Catchup to automatically fill up.
-
-This process realizes the global rerun of the upstream core through Clear, which can liberate manual operations.
-
-Because the cross-Dag global complement capability is important in a production environment, we plan to complement it in
-DolphinScheduler.
-
-## Current Status & Planning & Outlook
-
-### 1 DolphinScheduler migration status
-
-
-The DP platform has deployed part of the DolphinScheduler service in the test environment and migrated part of the
-workflow.
-
-After docking with the DolphinScheduler API system, the DP platform uniformly uses the admin user at the user level.
-Because its user system is directly maintained on the DP master, all workflow information will be divided into the test
-environment and the formal environment.
-
-
-<div align=center>
-<img src="https://s1.imgpp.com/2021/12/16/1_bXwtKI2HJzQuHCMW5y3hgg.png"/>
-</div>
-
-DolphinScheduler 2.0 workflow task node display
-
-The overall UI interaction of DolphinScheduler 2.0 looks more concise and more visualized and we plan to directly
-upgrade to version 2.0.
-
-### 2 Access planning
-
-
-At present, the DP platform is still in the grayscale test of DolphinScheduler migration., and is planned to perform a
-full migration of the workflow in December this year. At the same time, a phased full-scale test of performance and
-stress will be carried out in the test environment. If no problems occur, we will conduct a grayscale test of the
-production environment in January 2022, and plan to complete the full migration in March.
-
-<div align=center>
-<img src="https://s1.imgpp.com/2021/12/16/1_jv3ScivmLop7GYjKIECaiw.png"/>
-</div>
-
-### 3 Expectations for DolphinScheduler
-
-In the future, we strongly looking forward to the plug-in tasks feature in DolphinScheduler, and have implemented
-plug-in alarm components based on DolphinScheduler 2.0, by which the Form information can be defined on the backend and
-displayed adaptively on the frontend.
-
-<div align=center>
-<img src="https://s1.imgpp.com/2021/12/16/1_3jP2KQDtFy71ciDoUyW3eg.png"/>
-</div>
-
-"
-
-I hope that DolphinScheduler's optimization pace of plug-in feature can be faster, to better quickly adapt to our
-customized task types.
-
-——Zheqi Song, Head of Youzan Big Data Development Platform
-
-"
\ No newline at end of file
diff --git a/blog/en-us/architecture-design.md b/blog/en-us/architecture-design.md
deleted file mode 100644
index c4e0284..0000000
--- a/blog/en-us/architecture-design.md
+++ /dev/null
@@ -1,319 +0,0 @@
----
-title: Architecture Design
-keywords: Apache,DolphinScheduler,scheduler,big data,ETL,airflow,hadoop,orchestration, dataops
-description: Before explaining the architecture of the schedule system, let us first understand the common nouns of the schedule system.
----
-## Architecture Design
-Before explaining the architecture of the schedule system, let us first understand the common nouns of the schedule system.
-
-### 1.Noun Interpretation
-
-**DAG:** Full name Directed Acyclic Graph,referred to as DAG。Tasks in the workflow are assembled in the form of directed acyclic graphs, which are topologically traversed from nodes with zero indegrees of ingress until there are no successor nodes. For example, the following picture:
-<p align="center">
- <img src="/img/dag_examples_cn.jpg" alt="dag" width="60%" />
- <p align="center">
- <em>dag example</em>
- </p>
-</p>
-**Process definition**: Visualization **DAG** by dragging task nodes and establishing associations of task nodes
-
-**Process instance**: A process instance is an instantiation of a process definition, which can be generated by manual startup or scheduling. The process definition runs once, a new process instance is generated
-
-**Task instance**: A task instance is the instantiation of a specific task node when a process instance runs, which indicates the specific task execution status
-
-**Task type**: Currently supports SHELL, SQL, SUB_PROCESS (sub-process), PROCEDURE, MR, SPARK, PYTHON, DEPENDENT (dependency), and plans to support dynamic plug-in extension, note: the sub-**SUB_PROCESS** is also A separate process definition that can be launched separately
-
-**Schedule mode** : The system supports timing schedule and manual schedule based on cron expressions. Command type support: start workflow, start execution from current node, resume fault-tolerant workflow, resume pause process, start execution from failed node, complement, timer, rerun, pause, stop, resume waiting thread. Where **recovers the fault-tolerant workflow** and **restores the waiting thread** The two command types are used by the scheduling internal control and cannot be ca [...]
-
-**Timed schedule**: The system uses **quartz** distributed scheduler and supports the generation of cron expression visualization
-
-**Dependency**: The system does not only support **DAG** Simple dependencies between predecessors and successor nodes, but also provides **task dependencies** nodes, support for custom task dependencies between processes**
-
-**Priority**: Supports the priority of process instances and task instances. If the process instance and task instance priority are not set, the default is first in, first out.
-
-**Mail Alert**: Support **SQL Task** Query Result Email Send, Process Instance Run Result Email Alert and Fault Tolerant Alert Notification
-
-**Failure policy**: For tasks running in parallel, if there are tasks that fail, two failure policy processing methods are provided. **Continue** means that the status of the task is run in parallel until the end of the process failure. **End** means that once a failed task is found, Kill also drops the running parallel task and the process ends.
-
-**Complement**: Complement historical data, support ** interval parallel and serial ** two complement methods
-
-
-
-### 2.System architecture
-
-#### 2.1 System Architecture Diagram
-<p align="center">
- <img src="/img/architecture.jpg" alt="System Architecture Diagram" />
- <p align="center">
- <em>System Architecture Diagram</em>
- </p>
-</p>
-
-
-
-#### 2.2 Architectural description
-
-* **MasterServer**
-
- MasterServer adopts the distributed non-central design concept. MasterServer is mainly responsible for DAG task split, task submission monitoring, and monitoring the health status of other MasterServer and WorkerServer.
- When the MasterServer service starts, it registers a temporary node with Zookeeper, and listens to the Zookeeper temporary node state change for fault tolerance processing.
-
-
-
- ##### The service mainly contains:
-
- - **Distributed Quartz** distributed scheduling component, mainly responsible for the start and stop operation of the scheduled task. When the quartz picks up the task, the master internally has a thread pool to be responsible for the subsequent operations of the task.
-
- - **MasterSchedulerThread** is a scan thread that periodically scans the **command** table in the database for different business operations based on different ** command types**
-
- - **MasterExecThread** is mainly responsible for DAG task segmentation, task submission monitoring, logic processing of various command types
-
- - **MasterTaskExecThread** is mainly responsible for task persistence
-
-
-
-* **WorkerServer**
-
- - WorkerServer also adopts a distributed, non-central design concept. WorkerServer is mainly responsible for task execution and providing log services. When the WorkerServer service starts, it registers the temporary node with Zookeeper and maintains the heartbeat.
-
- ##### This service contains:
-
- - **FetchTaskThread** is mainly responsible for continuously receiving tasks from **Task Queue** and calling **TaskScheduleThread** corresponding executors according to different task types.
- - **LoggerServer** is an RPC service that provides functions such as log fragment viewing, refresh and download.
-
- - **ZooKeeper**
-
- The ZooKeeper service, the MasterServer and the WorkerServer nodes in the system all use the ZooKeeper for cluster management and fault tolerance. In addition, the system also performs event monitoring and distributed locking based on ZooKeeper.
- We have also implemented queues based on Redis, but we hope that EasyScheduler relies on as few components as possible, so we finally removed the Redis implementation.
-
- - **Task Queue**
-
- The task queue operation is provided. Currently, the queue is also implemented based on Zookeeper. Since there is less information stored in the queue, there is no need to worry about too much data in the queue. In fact, we have over-measured a million-level data storage queue, which has no effect on system stability and performance.
-
- - **Alert**
-
- Provides alarm-related interfaces. The interfaces mainly include **Alarms**. The storage, query, and notification functions of the two types of alarm data. The notification function has two types: **mail notification** and **SNMP (not yet implemented)**.
-
- - **API**
-
- The API interface layer is mainly responsible for processing requests from the front-end UI layer. The service provides a RESTful api to provide request services externally.
- Interfaces include workflow creation, definition, query, modification, release, offline, manual start, stop, pause, resume, start execution from this node, and more.
-
- - **UI**
-
- The front-end page of the system provides various visual operation interfaces of the system. For details, see the **[System User Manual] (System User Manual.md)** section.
-
-
-
-#### 2.3 Architectural Design Ideas
-
-##### I. Decentralized vs centralization
-
-###### Centralization Thought
-
-The centralized design concept is relatively simple. The nodes in the distributed cluster are divided into two roles according to their roles:
-
-<p align="center">
- <img src="https://analysys.github.io/easyscheduler_docs_cn/images/master_slave.png" alt="master-slave role" width="50%" />
- </p>
-
-- The role of Master is mainly responsible for task distribution and supervising the health status of Slave. It can dynamically balance the task to Slave, so that the Slave node will not be "busy" or "free".
-- The role of the Worker is mainly responsible for the execution of the task and maintains the heartbeat with the Master so that the Master can assign tasks to the Slave.
-
-Problems in the design of centralized :
-
-- Once the Master has a problem, the group has no leader and the entire cluster will crash. In order to solve this problem, most Master/Slave architecture modes adopt the design scheme of the master and backup masters, which can be hot standby or cold standby, automatic switching or manual switching, and more and more new systems are available. Automatically elects the ability to switch masters to improve system availability.
-- Another problem is that if the Scheduler is on the Master, although it can support different tasks in one DAG running on different machines, it will generate overload of the Master. If the Scheduler is on the Slave, all tasks in a DAG can only be submitted on one machine. If there are more parallel tasks, the pressure on the Slave may be larger.
-
-###### Decentralization
-
- <p align="center">
- <img src="https://analysys.github.io/easyscheduler_docs_cn/images/decentralization.png" alt="decentralized" width="50%" />
- </p>
-
-- In the decentralized design, there is usually no Master/Slave concept, all roles are the same, the status is equal, the global Internet is a typical decentralized distributed system, networked arbitrary node equipment down machine , all will only affect a small range of features.
-- The core design of decentralized design is that there is no "manager" that is different from other nodes in the entire distributed system, so there is no single point of failure problem. However, since there is no "manager" node, each node needs to communicate with other nodes to get the necessary machine information, and the unreliable line of distributed system communication greatly increases the difficulty of implementing the above functions.
-- In fact, truly decentralized distributed systems are rare. Instead, dynamic centralized distributed systems are constantly emerging. Under this architecture, the managers in the cluster are dynamically selected, rather than preset, and when the cluster fails, the nodes of the cluster will spontaneously hold "meetings" to elect new "managers". Go to preside over the work. The most typical case is the Etcd implemented in ZooKeeper and Go.
-
-- Decentralization of EasyScheduler is the registration of Master/Worker to ZooKeeper. The Master Cluster and the Worker Cluster are not centered, and the Zookeeper distributed lock is used to elect one Master or Worker as the “manager” to perform the task.
-
-##### 二、Distributed lock practice
-
-EasyScheduler uses ZooKeeper distributed locks to implement only one Master to execute the Scheduler at the same time, or only one Worker to perform task submission.
-
-1. The core process algorithm for obtaining distributed locks is as follows
-
- <p align="center">
- <img src="https://analysys.github.io/easyscheduler_docs_cn/images/distributed_lock.png" alt="Get Distributed Lock Process" width="50%" />
- </p>
-
-2. Scheduler thread distributed lock implementation flow chart in EasyScheduler:
-
- <p align="center">
- <img src="https://analysys.github.io/easyscheduler_docs_cn/images/distributed_lock_procss.png" alt="Get Distributed Lock Process" width="50%" />
- </p>
-
-##### Third, the thread is insufficient loop waiting problem
-
-- If there is no subprocess in a DAG, if the number of data in the Command is greater than the threshold set by the thread pool, the direct process waits or fails.
-- If a large number of sub-processes are nested in a large DAG, the following figure will result in a "dead" state:
-
- <p align="center">
- <img src="https://analysys.github.io/easyscheduler_docs_cn/images/lack_thread.png" alt="Thread is not enough to wait for loop" width="50%" />
- </p>
-
-In the above figure, MainFlowThread waits for SubFlowThread1 to end, SubFlowThread1 waits for SubFlowThread2 to end, SubFlowThread2 waits for SubFlowThread3 to end, and SubFlowThread3 waits for a new thread in the thread pool, then the entire DAG process cannot end, and thus the thread cannot be released. This forms the state of the child parent process loop waiting. At this point, the scheduling cluster will no longer be available unless a new Master is started to add threads to break s [...]
-
-It seems a bit unsatisfactory to start a new Master to break the deadlock, so we proposed the following three options to reduce this risk:
-
-1. Calculate the sum of the threads of all Masters, and then calculate the number of threads required for each DAG, that is, pre-calculate before the DAG process is executed. Because it is a multi-master thread pool, the total number of threads is unlikely to be obtained in real time.
-2. Judge the single master thread pool. If the thread pool is full, let the thread fail directly.
-3. Add a Command type with insufficient resources. If the thread pool is insufficient, the main process will be suspended. This way, the thread pool has a new thread, which can make the process with insufficient resources hang up and wake up again.
-
-Note: The Master Scheduler thread is FIFO-enabled when it gets the Command.
-
-So we chose the third way to solve the problem of insufficient threads.
-
-##### IV. Fault Tolerant Design
-
-Fault tolerance is divided into service fault tolerance and task retry. Service fault tolerance is divided into two types: Master Fault Tolerance and Worker Fault Tolerance.
-
-###### 1. Downtime fault tolerance
-
-Service fault tolerance design relies on ZooKeeper's Watcher mechanism. The implementation principle is as follows:
-
- <p align="center">
- <img src="https://analysys.github.io/easyscheduler_docs_cn/images/fault-tolerant.png" alt="EasyScheduler Fault Tolerant Design" width="40%" />
- </p>
-
-The Master monitors the directories of other Masters and Workers. If the remove event is detected, the process instance is fault-tolerant or the task instance is fault-tolerant according to the specific business logic.
-
-
-
-- Master fault tolerance flow chart:
-
- <p align="center">
- <img src="https://analysys.github.io/easyscheduler_docs_cn/images/fault-tolerant_master.png" alt="Master Fault Tolerance Flowchart" width="40%" />
- </p>
-
-After the ZooKeeper Master is fault-tolerant, it is rescheduled by the Scheduler thread in EasyScheduler. It traverses the DAG to find the "Running" and "Submit Successful" tasks, and monitors the status of its task instance for the "Running" task. You need to determine whether the Task Queue already exists. If it exists, monitor the status of the task instance. If it does not exist, resubmit the task instance.
-
-
-
-- Worker fault tolerance flow chart:
-
- <p align="center">
- <img src="https://analysys.github.io/easyscheduler_docs_cn/images/fault-tolerant_worker.png" alt="Worker Fault Tolerance Flowchart" width="40%" />
- </p>
-
-Once the Master Scheduler thread finds the task instance as "need to be fault tolerant", it takes over the task and resubmits.
-
- Note: Because the "network jitter" may cause the node to lose the heartbeat of ZooKeeper in a short time, the node's remove event occurs. In this case, we use the easiest way, that is, once the node has timeout connection with ZooKeeper, it will directly stop the Master or Worker service.
-
-###### 2. Task failure retry
-
-Here we must first distinguish between the concept of task failure retry, process failure recovery, and process failure rerun:
-
-- Task failure Retry is task level, which is automatically performed by the scheduling system. For example, if a shell task sets the number of retries to 3 times, then the shell task will try to run up to 3 times after failing to run.
-- Process failure recovery is process level, is done manually, recovery can only be performed from the failed node ** or ** from the current node **
-- Process failure rerun is also process level, is done manually, rerun is from the start node
-
-
-
-Next, let's talk about the topic, we divided the task nodes in the workflow into two types.
-
-- One is a business node, which corresponds to an actual script or processing statement, such as a Shell node, an MR node, a Spark node, a dependent node, and so on.
-- There is also a logical node, which does not do the actual script or statement processing, but the logical processing of the entire process flow, such as sub-flow sections.
-
-Each ** service node** can configure the number of failed retries. When the task node fails, it will automatically retry until it succeeds or exceeds the configured number of retries. **Logical node** does not support failed retry. But the tasks in the logical nodes support retry.
-
-If there is a task failure in the workflow that reaches the maximum number of retries, the workflow will fail to stop, and the failed workflow can be manually rerun or process resumed.
-
-
-
-##### V. Task priority design
-
-In the early scheduling design, if there is no priority design and fair scheduling design, it will encounter the situation that the task submitted first may be completed simultaneously with the task submitted subsequently, but the priority of the process or task cannot be set. We have redesigned this, and we are currently designing it as follows:
-
-- According to ** different process instance priority ** prioritizes ** same process instance priority ** prioritizes ** task priority within the same process ** takes precedence over ** same process ** commit order from high Go to low for task processing.
-
- - The specific implementation is to resolve the priority according to the json of the task instance, and then save the ** process instance priority _ process instance id_task priority _ task id** information in the ZooKeeper task queue, when obtained from the task queue, Through string comparison, you can get the task that needs to be executed first.
-
- - The priority of the process definition is that some processes need to be processed before other processes. This can be configured at the start of the process or at the time of scheduled start. There are 5 levels, followed by HIGHEST, HIGH, MEDIUM, LOW, and LOWEST. As shown below
-
- <p align="center">
- <img src="https://analysys.github.io/easyscheduler_docs_cn/images/process_priority.png" alt="Process Priority Configuration" width="40%" />
- </p>
-
- - The priority of the task is also divided into 5 levels, followed by HIGHEST, HIGH, MEDIUM, LOW, and LOWEST. As shown below
-
- <p align="center">
- <img src="https://analysys.github.io/easyscheduler_docs_cn/images/task_priority.png" alt="task priority configuration" width="35%" />
- </p>
-
-##### VI. Logback and gRPC implement log access
-
-- Since the Web (UI) and Worker are not necessarily on the same machine, viewing the log is not as it is for querying local files. There are two options:
- - Put the logs on the ES search engine
- - Obtain remote log information through gRPC communication
-- Considering the lightweightness of EasyScheduler as much as possible, gRPC was chosen to implement remote access log information.
-
- <p align="center">
- <img src="https://analysys.github.io/easyscheduler_docs_cn/images/grpc.png" alt="grpc remote access" width="50%" />
- </p>
-
-- We use a custom Logback FileAppender and Filter function to generate a log file for each task instance.
-- The main implementation of FileAppender is as follows:
-
-```java
- /**
- * task log appender
- */
- Public class TaskLogAppender extends FileAppender<ILoggingEvent> {
-
- ...
-
- @Override
- Protected void append(ILoggingEvent event) {
-
- If (currentlyActiveFile == null){
- currentlyActiveFile = getFile();
- }
- String activeFile = currentlyActiveFile;
- // thread name: taskThreadName-processDefineId_processInstanceId_taskInstanceId
- String threadName = event.getThreadName();
- String[] threadNameArr = threadName.split("-");
- // logId = processDefineId_processInstanceId_taskInstanceId
- String logId = threadNameArr[1];
- ...
- super.subAppend(event);
- }
-}
-```
-
-Generate a log in the form of /process definition id/process instance id/task instance id.log
-
-- Filter matches the thread name starting with TaskLogInfo:
-- TaskLogFilter is implemented as follows:
-
-```java
- /**
- * task log filter
- */
-Public class TaskLogFilter extends Filter<ILoggingEvent> {
-
- @Override
- Public FilterReply decide(ILoggingEvent event) {
- If (event.getThreadName().startsWith("TaskLogInfo-")){
- Return FilterReply.ACCEPT;
- }
- Return FilterReply.DENY;
- }
-}
-```
-
-
-
-### summary
-
-Starting from the scheduling, this paper introduces the architecture principle and implementation ideas of the big data distributed workflow scheduling system-EasyScheduler. To be continued
diff --git a/blog/en-us/meetup_2019_10_26.md b/blog/en-us/meetup_2019_10_26.md
deleted file mode 100644
index 943ac2f..0000000
--- a/blog/en-us/meetup_2019_10_26.md
+++ /dev/null
@@ -1,15 +0,0 @@
-
-Apache Dolphin Scheduler(Incubating) Meetup has been held successfully in Shanghai 2019.10.26.
-
-Address: Shanghai Changning Yuyuan Road 1107 Chuangyi space (Hongji) 3r20
-
-The meetup was begin at 2:00 pm, and close at about 5:00 pm.
-
-The Agenda is as following:
-
-* Introduction/overview of DolphinScheduler (William-GuoWei 14:00-14:20). [PPT](https://github.com/apache/dolphinscheduler-website/tree/master/file/2019-10-26/DolphinScheduler_guowei.pptx)
-* DolphinScheduler internals, fairly technical: how DolphinScheduler works and so on (Zhanwei Qiao 14:20-15:00) [PPT](https://github.com/apache/dolphinscheduler-website/tree/master/file/2019-10-26/DolphinScheduler_qiaozhanwei.pptx)
-* From open source users to PPMC --- Talking about Me and DolphinScheduler(Baoqi Wu from guandata 15:10-15:50)[PPT](https://github.com/apache/dolphinscheduler-website/tree/master/file/2019-10-26/Dolphinescheduler_baoqiwu.pptx)
-* Application and Practice of Dolphin Scheduler in cathay-ins (Zongyao Zhang from cathay-ins 15:50-16:30)[PPT](https://github.com/apache/dolphinscheduler-website/tree/master/file/2019-10-26/DolphinScheduler_zhangzongyao.pptx)
-* Recently released features and Roadmap (Lidong Dai 16:30-17:00) [PPT](https://github.com/apache/dolphinscheduler-website/tree/master/file/2019-10-26/DolphinScheduler_dailidong.pptx)
-* Free discussion
diff --git a/blog/en-us/meetup_2019_12_08.md b/blog/en-us/meetup_2019_12_08.md
deleted file mode 100644
index 0b72f8a..0000000
--- a/blog/en-us/meetup_2019_12_08.md
+++ /dev/null
@@ -1,63 +0,0 @@
-
-
-
-
-Apache ShardingSphere & DolphinScheduler joint Meetup
-
-Address: 7th Floor, Haizhi Venture Capital Building, No. 34 Beijing Haidian Street
-
-Meeting time: December 8, 2019 14:00 ~ 17: 30
-
-
-
-**Meetup description**
-
-Today, open source is blooming in China, and the open source trend is unstoppable. The Apache community already has more than 10 open source projects from our native China. This time, users and technology enthusiasts who joined the two Apache community projects gathered together to share open source technologies together for China's native open source contribution!
-
-Apache ShardingSphere (Incubator) is a set of open source distributed database middleware. It aims to fully and reasonably utilize the computing and storage capabilities of relational databases in distributed scenarios, and provides standardized data sharding, distributed transactions, and database governance. Functions can be applied to various diverse application scenarios such as Java isomorphism, heterogeneous languages, and cloud native.
-
-Apache DolphinScheduler (Incubator) is a distributed decentralized, easily scalable visual DAG workflow task scheduling system. Committed to solving the intricate dependencies in the data processing process, it has features such as high reliability (HA), easy expansion, support for most task scenarios, and ease of use (visual drag), and has been used by dozens of companies.
-
-The two communities are invited to use the theory and practice of partners and Committer to share experience and communicate on the spot. At the end of the event, there will be wonderful discussions on how to join the Apache community and become a Committer or PPMC. Contribute to open source together!
-
-
-
-**The agenda is as follows**
-
-* 14:00 - 14:40 The integration of DolphinScheduler and containerization (Xiaochun Liu) [PPT](/file/2019-12-08/DolphinScheduler_liuxiaochun.pptx)
-* 14:40 - 15:20 Analyzing of Sharding-Proxy principle (Yonglun Zhang [PPT](/file/2019-12-08/ShardingSphere_zhangyonglun.pptx)
-* 15:20 - 16:00 Migration and application of DolphinScheduler in Baiwang (Shuang Yang)[PPT](/file/2019-12-08/DolphinScheduler_yangshuang.pptx)
-* 16:10 - 16:40 The Architecture of ShardingSphere and Roadmap (Juan Pan) [PPT](/file/2019-12-08/ShardingSphere_panjuan.pptx)
-* 16:40 - 17:20 Roundtable Discussion - How to join the Apache community and to be a committer
-* Free discussion
-
-
-
-**Wonderful moment**
-
-
-
-
-
-
-
-
-
-
-
-
-
-
-
-
-
-
-
-
-
-
-
-
-
-
-
diff --git a/blog/zh-cn/Apache-DolphinScheduler-2.0.1.md b/blog/zh-cn/Apache-DolphinScheduler-2.0.1.md
deleted file mode 100644
index d56dad8..0000000
--- a/blog/zh-cn/Apache-DolphinScheduler-2.0.1.md
+++ /dev/null
@@ -1,265 +0,0 @@
-# Apache DolphinScheduler 2.0.1 来了,备受期待的一键升级、插件化终于实现!
-
-<div align=center>
-<img src="https://s1.imgpp.com/2021/12/17/1639647220322.png"/>
-</div>
-
-> 编者按:好消息!Apache DolphinScheduler 2.0.1 版本今日正式发布!
-
-> 本版本中,DolphinScheduler 经历了一场微内核+插件化的架构改进,70% 的代码被重构,一直以来备受期待的插件化功能也得到重要优化。此外,本次升级还有不少亮点,如一键升级至最新版本、注册中心“去 ZK 化”、新增任务参数传递功能等。
-
-> Apache DolphinScheduler 2.0.1 下载地址:
-> https://dolphinscheduler.apache.org/zh-cn/download/download.html
-
-Apache DolphinScheduler 2.0.1 的工作流执行流程活动如下图所示:
-
-<div align=center>
-<img src="https://s1.imgpp.com/2021/12/17/82a493951882982a22823a08ab8718e7.png"/>
-</div>
-
-启动流程活动图
-
-2.0.1 版本通过优化内核增强了系统处理能力,从而在性能上得到较大提升,全新的 UI 界面也极大地提升了用户体验。更重要的是,2.0.1 版本还有两个重大变化:插件化和重构。
-
-
-## 01 插件化
-
-
-此前,有不少用户反馈希望 Apache DolphinScheduler 可以优化插件化,为响应用户需求,Apache DolphinScheduler 2.0.1 在插件化上更进了一步,**新增了告警插件、注册中心插件和任务插件管理功能。** 利用插件化,用户可以更加灵活地实现自己的功能需求,更加简单地根据接口自定义开发任务组件,也可以无缝迁移用户的任务组件至 DolphinScheduler 更高版本中。
-
-DolphinScheduler 正在处于**微内核 + 插件化的架构改进**之中,所有核心能力如任务、告警组件、数据源、资源存储、注册中心等都将被设计为扩展点,我们希望通过 SPI 来提高 Apache DolphinScheduler 本身的灵活性和友好性。
-
-相关代码可以参考 dolphinscheduler-spi 模块,相关插件的扩展接口也皆在该模块下。用户需要实现相关功能插件化时,建议先阅读此模块代码。当然,也建议大家阅读文档以节省时间。
-
-我们采用了一款优秀的前端组件 form-create,它支持基于 json 生成前端 UI 组件,如果插件开发涉及到前端,我们会通过 json 来生成相关前端 UI 组件。
-
-org.apache.dolphinscheduler.spi.params 里对插件的参数做了封装,它会将相关参数全部转化为对应的 json。这意味着,你完全可以通过 Java 代码的方式完成前端组件的绘制(这里主要是表单)。
-
-### 1 告警插件
-
-
-以告警插件为例,我们实现了在 alert-server 启动时加载相关插件。alert 提供了多种插件配置方法,目前已经内置了 Email、DingTalk、EnterpriseWeChat、Script 等告警插件。当插件模块开发工作完成后,通过简单的配置即可启用。
-
-### 2 多注册中心组件
-
-在 Apache DolphinScheduler 1.X 中,Zookeeper 组件有着非常重要的意义,包括 master/worker 服务的监控发现、失联告警、通知容错等功能。在 2.0.1 版本中,我们在注册中心逐渐“去 ZK 化”,弱化了 Zookeeper 的作用,新增了插件管理功能。
-
-在插件管理中,用户可以增加 ETCD 等注册中心的支持,使得 Apache Dolphinscheduler 的灵活性更高,能适应更复杂的用户需求。
-
-### 3 任务组件插件
-
-
-新版本还新增了任务插件功能,增强了不同的任务组件的隔离功能。用户开发自定义插件时,只需要实现插件的接口即可。主要包含创建任务(任务初始化、任务运行等方法)和任务取消。
-
-如果是 Yarn 任务,则需要实现 AbstractYarnTask。目前,任务插件的前端需要开发者自己使用 Vue 开发部署,在后续版本中,我们将实现由 Java 代码的方式完成前端组件的自动绘制。
-
-
-## 02 重构
-
-
-迄今为止,Apache DolphinScheduler 已经重构了约 70% 的代码,实现了全面的升级。
-
-### 1 Master 内核优化
-
-2.0.1 版本升级包括重构了 Master 的执行流程,将之前状态轮询监控改为事件通知机制,大幅减轻了数据库的轮询压力;去掉全局锁,增加了 Master 的分片处理机制,将顺序读写命令改为并行处理,增强了 Master 横向扩展能力;优化工作流处理流程,减少了线程池的使用,大幅提升单个 Master 处理的工作流数量;增加缓存机制,优化数据库连接方式,以及简化处理流程,减少处理过程中不必要的耗时操作等。
-
-
-### 2 工作流和任务解耦
-
-
-在 Apache DolphinScheduler 1.x 版本中,任务及任务关系保存是以大 json 的方式保存到工作流定义表中的,如果某个工作流很大,比如达到 100 至 1000 个任务规模,这个 json 字段会非常大,在使用时需要解析 json。这个过程比较耗费性能,且任务无法重用;另一方面,基于大 json,在工作流版本及任务版本上也没有很好的实现方案。
-
-因此,在新版本中,我们针对工作流和任务做了解耦,新增了任务和工作流的关系表,并新增了日志表,用来保存工作流定义和任务定义的历史版本,大幅提高工作流运行的效率。
-
-下图为 API 模块下工作流和任务的操作流程图:
-
-<div align=center>
-<img src="https://s1.imgpp.com/2021/12/17/27405914b6eced124394f2079676633c.png"/>
-</div>
-
-
-## 03 版本自动升级功能
-
-
-2.0.1 增加了版本自动升级功能,用户可以从 1.x 版本自动升级到 2.0.1 版本。只需要运行一个使用脚本,即可无感知地使用新版本运行以前的工作流:
-sh ./script/create-dolphinscheduler.sh
-
-**具体升级文档请参考:** https://dolphinscheduler.apache.org/zh-cn/docs/latest/user_doc/guide/upgrade.html
-
-另外,Apache DolphinScheduler 将来的版本均可实现自动升级,省去手动升级的麻烦。
-
-## 04 新功能列表
-
-
-Apache DolphinScheduler 2.0.1 新增功能详情如下:
-
-### 1 新增 Standalone 服务
-
-StandAloneServer 是为了让用户快速体验产品而创建的服务,其中内置了注册中心和数据库 H2-DataBase、Zk-TestServer,在修改后一键启动 StandAloneServer 即可进行调试。
-
-如果想快速体验,在解压安装包后,用户只需要配置 jdk 环境等即可一键启动 Apache DolphinScheduler 系统,从而减少配置成本,提高研发效率。
-
-详细的使用文档请参考:https://dolphinscheduler.apache.org/zh-cn/docs/latest/user_doc/guide/installation/standalone.html
-
-或者使用 Docker 一键部署所有的服务:https://dolphinscheduler.apache.org/zh-cn/docs/latest/user_doc/guide/installation/docker.html
-
-### 2 任务参数传递功能
-
-
-目前支持 shell 任务和 sql 任务之间的传递。
-
-- shell 任务之间的传参:
-
-
-在前一个"create_parameter"任务中设置一个out的变量”trans“: echo '${setValue(trans=hello trans)}'
-
-<div align=center>
-<img src="https://s1.imgpp.com/2021/12/17/323f6a18d8a1d2f2d8fdcb5687c264b5.png"/>
-</div>
-
-
-当前置任务中的任务日志中检测到关键字:”${setValue(key=value)}“, 系统会自动解析变量传递值,在后置任务中,可以直接使用”trans“变量:
-
-<div align=center>
-<img src="https://s1.imgpp.com/2021/12/17/8be29339b73b594dc05a6b832d9330ec.png"/>
-</div>
-
-- SQL 任务的参数传递:
-
-SQL 任务的自定义变量 prop 的名字需要和字段名称一致,变量会选择 SQL 查询结果中的列名中与该变量名称相同的列对应的值。输出用户数量:
-
-<div align=center>
-<img src="https://s1.imgpp.com/2021/12/17/85bc5216c01ca958cdf11d4bd555c8a6.png"/>
-</div>
-
-在下游任务中使用变量”cnt“:
-
-<div align=center>
-<img src="https://s1.imgpp.com/2021/12/17/4278d0b7f833b64f24fc3d6122287454.png"/>
-</div>
-
-新增 switch 任务和 pigeon 任务组件:
-
-- switch 任务
-
-在 switch 任务中设置判断条件,可以实现根据不同的条件判断结果运行不同的条件分支的效果。例如:有三个任务,其依赖关系是 A -> B -> [C, D] ,其中 task_a是 shell 任务,task_b 是 switch 任务。
-
-任务 A 中通过全局变量定义了名为 id 的全局变量,声明方式为`echo '${setValue(id=1)}' `。
-
-任务 B 增加条件,使用上游声明的全局变量实现条件判断(注意 Switch 运行时存在的全局变量就行,意味着可以是非直接上游产生的全局变量)。下面我们想要实现当 id 为 1 时,运行任务 C,其他运行任务 D。
-
-配置当全局变量 id=1 时,运行任务 C。则在任务 B 的条件中编辑 ${id} == 1,分支流转选择 C。对于其他任务,在分支流转中选择 D。
-
-<div align=center>
-<img src="https://s1.imgpp.com/2021/12/17/636f53ddc809f028ffdfc18fd08b5828.md.jpg"/>
-</div>
-
-
-- pigeon 任务
-
-pigeon 任务,是一个可以和第三方系统对接的一种任务组件,可以实现触发任务执行、取消任务执行、获取任务状态,以及获取任务日志等功能。pigeon 任务需要在配置文件中配置上述任务操作的 API 地址,以及对应的接口参数。在任务组件里输入一个目标任务名称,即可对接第三方系统,实现在 Apache DolphinScheduler 中操作第三方系统的任务。
-
-
-### 3 新增环境管理功能
-
-
-默认环境配置为 dolphinscheduler_env.sh。
-
-在线配置 Worker 运行环境,一个 Worker 可以指定多个环境,每个环境等价于 dolphinscheduler_env.sh 文件。
-
-<div align=center>
-<img src="https://s1.imgpp.com/2021/12/17/ef8b444c6dbebe397daaaa3bbadf743f.png"/>
-</div>
-
-在创建任务的时候,选择 worker 分组和对应的环境变量,任务在执行时,worker 会在对应的执行环境中执行任务。
-
-
-## 05 优化项
-
-
-### 1 优化 RestApi
-
-
-我们更新了新的 RestApi 规范,并且按照规范,重新优化了 API 部分,使得用户在使用 API 时更加简单。
-
-
-### 2 优化工作流版本管理
-
-
-优化了工作流版本管理功能,增加了工作流和任务的历史版本。
-
-
-### 3 优化 worker 分组管理功能
-
-在 2.0 版本中,我们新增了 worker 分组管理功能,用户可以通过页面配置来修改 worker 所属的分组信息,无需到服务器上修改配置文件并重启 worker,使用更加便捷。
-
-优化 worker 分组管理功能后,每个 worker 节点都会归属于自己的 Worker 分组,默认分组为 default。在任务执行时,可以将任务分配给指定 worker 分组,最终由该组中的 worker 节点执行该任务。
-
-修改 worker 分组有两种方法:
-
-1. 打开要设置分组的 worker 节点上的"conf/worker.properties"配置文件,修改 worker.groups 参数。
-2. 可以在运行中修改 worker 所属的 worker 分组,如果修改成功,worker 就会使用这个新建的分组,忽略 worker.properties 中的配置。修改步骤为"安全中心 -> worker 分组管理 -> 点击 '新建 worker 分组' -> 输入'组名称' -> 选择已有 worker -> 点击'提交'"
-
-其他优化事项:
-
-1. 增加了启动工作流的时候,可以修改启动参数;
-2. 新增了保存工作流时,自动上线工作流状态;
-3. 优化了 API 返回结果,加快了创建工作流时页面的加载速度;
-4. 加快工作流实例页面的加载速度;
-5. 优化工作流关系页面的显示信息;
-6. 优化了导入导出功能,支持跨系统导入导出工作流;
-7. 优化了一些 API 的操作,如增加了若干接口方法,增加任务删除检查等。
-
-
-## 06 变更日志
-
-
-另外Apache DolphinScheduler 2.0.1 也修复了一些 bug,主要包括:
-
-1. 修复了 netty 客户端会创建多个管道的问题;
-2. 修复了导入工作流定义错误的问题;
-3. 修复了任务编码会重复获取的问题;
-4. 修复使用 Kerberos 时,Hive 数据源连接失败的问题;
-5. 修复 Standalone 服务启动失败问题;
-6. 修复告警组显示故障的问题;
-7. 修复文件上传异常的问题;
-8. 修复 Switch 任务运行失败的问题;
-9. 修复工作流超时策略失效的问题;
-10. 修复 sql 任务不能发送邮件的问题。
-
-
-## 07 致谢
-
-
-感谢 289 位参与 2.0.1 版本优化和改进的社区贡献者(排名不分先后)!
-
-<div align=center>
-<img src="https://s1.imgpp.com/2021/12/17/2020b4f57e33734414a11149704ded92.png"/>
-</div>
-
-
-<div align=center>
-<img src="https://s1.imgpp.com/2021/12/17/1825b6945d5845233b7389479ba6c074.png"/>
-</div>
-
-## 8 加入社区
-
-随着国内开源的迅猛崛起,Apache DolphinScheduler 社区迎来蓬勃发展,为了做更好用、易用的调度,真诚欢迎热爱开源的伙伴加入到开源社区中来,为中国开源崛起献上一份自己的力量,让本土开源走向全球。
-
-参与 DolphinScheduler 社区有非常多的参与贡献的方式,包括:
-
-<div align=center>
-<img src="https://s1.imgpp.com/2021/12/17/bca55edc877ed6136703a6251e3a19f9.png"/>
-</div>
-
-贡献第一个PR(文档、代码) 我们也希望是简单的,第一个PR用于熟悉提交的流程和社区协作以及感受社区的友好度。
-
-* 社区汇总了以下适合新手的问题列表:https://github.com/apache/dolphinscheduler/issues/5689
-
-* 进阶问题列表:https://github.com/apache/dolphinscheduler/issues?q=is%3Aopen+is%3Aissue+label%3A%22volunteer+wanted%22
-
-* 如何参与贡献链接:https://dolphinscheduler.apache.org/zh-cn/community/development/contribute.html
-
-来吧,DolphinScheduler开源社区需要您的参与,为中国开源崛起添砖加瓦吧,哪怕只是小小的一块瓦,汇聚起来的力量也是巨大的。
-
-参与开源可以近距离与各路高手切磋,迅速提升自己的技能,如果您想参与贡献,我们有个贡献者种子孵化群,可以添加社区小助手微信(Leonard-ds) 手把手教会您( 贡献者不分水平高低,有问必答,关键是有一颗愿意贡献的心 )。
\ No newline at end of file
diff --git a/blog/zh-cn/Apache_dolphinScheduler_2.0.2.md b/blog/zh-cn/Apache_dolphinScheduler_2.0.2.md
deleted file mode 100644
index 66b4019..0000000
--- a/blog/zh-cn/Apache_dolphinScheduler_2.0.2.md
+++ /dev/null
@@ -1,131 +0,0 @@
-# WorkflowAsCode 来了,Apache DolphinScheduler 2.0.2 惊喜发布!
-
-<div align=center>
-<img src="/img/2022-1-13/1_3XcwBeN5HkBzZ76zXDcigw.jpeg"/>
-</div>
-
-千呼万唤中,WorkflowAsCode 功能终于在 2.0.2 版本中如约上线,为有动态、批量创建和更新工作流需求的用户带来福音。
-
-此外,新版本还新增企业微信告警群聊会话消息推送,简化了元数据初始化流程,并修复了旧版本中强制终止后服务重启失败,添加 Hive 数据源失败等问题。</font>
-
-## 01 新功能
-
-### 1 WorkflowAsCode
-
-
-首先在新功能上,2.0.2 版本重磅发布了 PythonGatewayServer, 这是一个 Workflow-as-code 的服务端,与 apiServer 等服务的启动方式相同。
-
-启用 PythonGatewayServer 后,所有 Python API 的请求都会发送到 PythonGatewayServer。Workflow-as-code 让用户可以通过 Python API 创建工作流,对于有动态、批量地创建和更新工作流的用户来说是一个好消息。通过 Workflow-as-code 创建的工作流与其他工作流一样,都可以在 web UI 查看。
-
-以下为一个 Workflow-as-code 测试用例:
-
-```py
-
-# 定义工作流属性,包括名称、调度周期、开始时间、使用租户等信息
-with ProcessDefinition(
- name="tutorial",
- schedule="0 0 0 * * ? *",
- start_time="2021-01-01",
- tenant="tenant_exists",
-) as pd:
- # 定义4个任务,4个都是 shell 任务,shell 任务的必填参数为任务名、命令信息,这里都是 echo 的 shell 命令
- task_parent = Shell(name="task_parent", command="echo hello pydolphinscheduler")
- task_child_one = Shell(name="task_child_one", command="echo 'child one'")
- task_child_two = Shell(name="task_child_two", command="echo 'child two'")
- task_union = Shell(name="task_union", command="echo union")
-
- # 定义任务间依赖关系
- # 这里将 task_child_one,task_child_two 先声明成一个任务组,通过 python 的 list 声明
- task_group = [task_child_one, task_child_two]
- # 使用 set_downstream 方法将任务组 task_group 声明成 task_parent 的下游,如果想要声明上游则使用 set_upstream
- task_parent.set_downstream(task_group)
-
- # 使用位操作符 << 将任务 task_union 声明成 task_group 的下游,同时支持通过位操作符 >> 声明
- task_union << task_group
-
-```
-
-上面的代码运行后,可以在 web UI 看到的工作流如下:
-
-``` --> task_child_one
- / \
-task_parent --> --> task_union
- \ /
- --> task_child_two
-```
-### 2 企业微信告警方式支持群聊消息推送
-
-在此前版本中,微信告警方式仅支持消息通知方式;在 2.0.2 版本中,用户在使用企业微信的告警时,支持进行应用内以群聊会话消息推送的方式推送给用户。
-
-## 02 优化
-
-### 1 简化元数据初始化流程
-
-首次安装 Apache DolphinScheduler 时,运行 create-dolphinscheduler.sh 需要从最早的版本逐步升级到当前版本。为了更方便快捷地初始化元数据流程,2.0.2 版本让用户可以直接安装当前版本的数据库脚本,提升安装速度。
-
-### 2 删除补数日期中的“+1”(天)
-
-删除了补数日期中的“+1”天,以避免补数时 UI 日期总显示 +1 给用户造成的困惑。
-## 03 Bug 修复
-
-[#7661] 修复 logger 在 worker 中的内存泄漏
-[#7750] 兼容历史版本数据源连接信息
-[#7705] 内存限制导致从 1.3.5 升级到 2.0.2 出现错误
-[#7786] 强制终止后服务重启失败
-[#7660] 流程定义版本创建时间错误
-[#7607] 执行 PROCEDURE 节点失败
-[#7639] 在通用配置项中添加 quartz 和 zookeeper 默认配置
-[#7654] 在依赖节点中,出现不属于当前项目的选项时报错
-[#7658] 工作流复制错误
-[#7609] worker sendResult 成功但 master 未收到错误时,工作流始终在运行
-[#7554] Standalone Server 中的 H2 会在数分钟后自动重启,导致数据异常丢失
-[#7434] 执行 MySQL 建表语句报错
-[#7537] 依赖节点重试延迟不起作用
-[#7392] 添加 Hive 数据源失败
-
-下载:https://dolphinscheduler.apache.org/zh-cn/download/download.html
-Release Note:https://github.com/apache/dolphinscheduler/releases/tag/2.0.2
-
-## 04 致谢
-
-一如既往地,感谢所有为 2.0.2版本建言献策并付诸行动的 Contributor(排名不分先后),是你们的智慧和付出让 Apache DolphinScheduler 更加符合用户的使用需求。
-
-<div align=center>
-<img src="/img/2022-1-13/1_IFBxUh2I0LFWF3Jkwz1e5g.png"/>
-</div>
-
-
-## 05 参与贡献
-
-
-随着国内开源的迅猛崛起,Apache DolphinScheduler 社区迎来蓬勃发展,为了做更好用、易用的调度,真诚欢迎热爱开源的伙伴加入到开源社区中来,为中国开源崛起献上一份自己的力量,让本土开源走向全球。
-
-参与 DolphinScheduler 社区有非常多的参与贡献的方式,包括:
-
-贡献第一个PR(文档、代码) 我们也希望是简单的,第一个PR用于熟悉提交的流程和社区协作以及感受社区的友好度。
-
-社区汇总了以下适合新手的问题列表:https://github.com/apache/dolphinscheduler/issues/5689
-
-非新手问题列表:https://github.com/apache/dolphinscheduler/issues?q=is%3Aopen+is%3Aissue+label%3A%22volunteer+wanted%22
-
-如何参与贡献链接:https://dolphinscheduler.apache.org/zh-cn/community/development/contribute.html
-
-来吧,DolphinScheduler开源社区需要您的参与,为中国开源崛起添砖加瓦吧,哪怕只是小小的一块瓦,汇聚起来的力量也是巨大的。
-
-
-社区官网
-https://dolphinscheduler.apache.org/
-
-代码仓地址
-https://github.com/apache/dolphinscheduler
-
-您的 Star,是 Apache DolphinScheduler 为爱发电的动力❤️ ~
-
-
-
-
-
-
-
-
-
diff --git a/blog/zh-cn/Apache_dolphinScheduler_2.0.3.md b/blog/zh-cn/Apache_dolphinScheduler_2.0.3.md
deleted file mode 100644
index 4ab649a..0000000
--- a/blog/zh-cn/Apache_dolphinScheduler_2.0.3.md
+++ /dev/null
@@ -1,121 +0,0 @@
-# Apache DolphinScheduler 2.0.3 发布,支持钉钉告警签名校验,数据源可从多个会话获取链接
-
-<div align=center>
-<img src="/img/2.0.3/2022-1-27/1.png"/>
-</div>
-
-
-> 今天,Apache DolphinScheduler 宣布 2.0.3 版本正式发布。本版本支持钉钉告警签名校验,以及数据源从多个会话获取链接。此外,2.0.3 还对缓存管理、补数时间、日志中的数据源密码显示等进行优化,并修复了若干关键Bug。
-
-## 新增功能
-### 钉钉告警支持加签名校验
-
-2.0.3 支持通过签名方式实现钉钉机器人报警的功能。
-
-<div align=center>
-<img src="/img/2.0.3/2022-1-27/2.png"/>
-</div>
-
-钉钉的参数配置
-
-- Webhook
-
-格式如下:https://oapi.dingtalk.com/robot/send?access_token=XXXXXX
-
-- Keyword
-
-
-安全设置的自定义关键词
-
-- Secret
-
-
-安全设置的加签
-
-自定义机器人发送消息时,可以通过手机号码指定“被@人列表”。在“被@人列表”中的人员收到该消息时,会有@消息提醒。设置为免打扰模式,会话仍然会有通知提醒,在首屏出现“有人@你”提示。
-
-- @Mobiles
-
-
-被@人的手机号
-
-- @UserIds
-
-
-被@人的用户userid
-
-- @All
-
-
-是否@所有人
-
-### 支持数据源从多个会话获取链接
-
-此前,使用 JdbcDataSourceProvider.createOneSessionJdbcDataSource() 方法hive/impala 创建连接池设置了 MaximumPoolSize=1,但是调度任务中,如果 hive/impala 多任务同时运行,会出现 getConnection=null 的情况,SqlTask.prepareStatementAndBind() 方法会抛出空指针异常。
-
-
-
-
-2.0.3 优化了这一点,支持数据源从多个会话获取链接。
-
-
-## 优化
-### 缓存管理优化,减少 Master 调度过程中的 DB 查询次数
-
-
-
-
-由于主服务器调度进程,中会出现大量的数据库读操作,如 tenant、user、processDefinition 等,这一方面会给 DB 带来巨大压力,另一方面会减慢整个核心调度过程。
-
-
-
-
-考虑到这部分业务数据是多读少写的场景,2.0.3 引入了缓存模块,主要作用于 Master 节点,将业务数据如租户、工作流定义等进行缓存,降低数据库查询压力,加快核心调度进程,详情可查看官网文档:https://dolphinscheduler.apache.org/en-us/docs/latest/user_doc/architecture/cache.html
-
-
-
-### 补数时间区间从 “左闭右开” 改为 “左闭右闭”
-
-此前,补数时间为“左闭右开”(startDate <= N < endDate),不利于用户理解。优化之后,部署时间区间改为“左闭右闭”。
-
-### 对日志中的数据源密码进行加密显示
-
-数据源中的密码进行加密,加强隐私保护。
-
-
-
-## Bug 修复
-
-* zkRoot 配置不起作用
-* 修复修改管理员账号的用户信息引起的错误
-* 增加删除工作流定义同时删除工作流实例
-* UDF 编辑文件夹对话框不能取消
-* 修复因为 netty 通讯没有失败重试,worker 和 master 通讯失败,导致工作流一直运行中的问题
-* 删除运行中的工作流,Master 会一直打印失败日志
-* 修复环境变量中选择 workerGroup 的问题
-* 修复依赖任务中告警设置不起作用的问题
-* 工作流历史版本查询信息出错
-* 解决高并发下任务日志输出影响性能的问题
-* sub_process 节点的全局参数未传递给关联的工作流任务
-* K8S 上 Master 任务登录时,查询日志无法显示内容
-* 进程定义列表中存在重复进程
-* 当流程实例 FailureStrategy.END 时任务失败,流程实例一直在运行
-* t_ds_resources 表中的“is_directory”字段在 PostgreSQL 数据库中出现类型错误
-* 修复 Oracle 的 JDBC 连接
-* Dag 中有禁止节点时,执行流程异常
-* querySimpleList 返回错误的项目代码
-
-
-
-
-**Release Note:** https://github.com/apache/dolphinscheduler/releases/tag/2.0.3
-
-**下载地址:** https://dolphinscheduler.apache.org/en-us/download/download.html
-
-## 感谢贡献者
-
-感谢社区 Contributor 对本版本的积极贡献,以下为 Contributor 名单,排名不分先后:
-
-<div align=center>
-<img src="/img/2.0.3/2022-1-27/3.png"/>
-</div>
diff --git a/blog/zh-cn/Apache_dolphinScheduler_2.0.5.md b/blog/zh-cn/Apache_dolphinScheduler_2.0.5.md
deleted file mode 100644
index 26dff5d..0000000
--- a/blog/zh-cn/Apache_dolphinScheduler_2.0.5.md
+++ /dev/null
@@ -1,75 +0,0 @@
-# Apache DolphinScheduler 2.0.5 发布,Worker 容错流程优化
-
-<div align=center>
-<img src="/img/2022-3-7/1.png"/>
-</div>
-
-今天,Apache DolphinScheduler 宣布 2.0.5 版本正式发布。此次版本进行了一些功能优化,如 Worker 的容错流程优化,在资源中心增加了重新上传文件的功能,并进行了若干 Bug 修复。
-
-
-## 优化
-
-### Worker 容错流程
-
-2.0.5 版本优化了 worker 的容错流程,使得服务器由于压力过大导致 worker 服务中断时,可以正常将任务转移至其他worker 上继续执行,避免任务中断。
-
-### 禁止运行任务页面标志
-
-优化禁止运行任务的页面显示标志,区别于正常执行的任务显示,以免用户混淆工作状态。
-
-<div align=center>
-
-<img src="/img/2022-3-7/2.png"/>
-
-</div>
-
-### 任务框增加提示语
-
-2.0.5 版本在任务框上增加了提示语,可以显示出全部的长任务名字,方便用户查看。
-
-<div align=center>
-
-<img src="/img/2022-3-7/3.png"/>
-
-</div>
-
-### 资源中心增加重新上传文件功能
-
-在资源中心增加了重新上传文件的功能,当用户需要修改执行脚本时,无需再重新配置任务参数,可实现自动更新执行脚本功能。
-
-### 修改工作流后跳转到列表页
-
-改变了此前修改工作流以后页面仍然留在 DAG 页面的现状,优化后可跳转到列表页,便于用户后续操作。
-
-### 钉钉告警插件新增 Markdown 信息类型
-
-在钉钉告警插件的告警内容中新增 Markdown 信息类型,丰富信息类型支持。
-
-## Bug 修复
-
-[[#8213](https://github.com/apache/dolphinscheduler/issues/8213)] 修复了当 worker 分组包含大写字母时,任务运行错误的问题;
-
-[[#8347](https://github.com/apache/dolphinscheduler/pull/8347)] 修复了当任务失败重试时,工作流不能被停止的问题;
-
-[[#8135](https://github.com/apache/dolphinscheduler/issues/8135)] 修复了 jdbc 连接参数不能输入‘@’的问题;
-
-[[#8367](https://github.com/apache/dolphinscheduler/issues/8367)] 修复了补数时可能不会正常结束的问题;
-
-[[#8170](https://github.com/apache/dolphinscheduler/issues/8170)] 修复了从页面上不能进入子工作流的问题。
-
-**2.0.5 下载地址**:
-
-[https://dolphinscheduler.apache.org/zh-cn/download/download.html](https://dolphinscheduler.apache.org/zh-cn/download/download.html)
-
-**Release Note:**[https://github.com/apache/dolphinscheduler/releases/tag/2.0.5](https://github.com/apache/dolphinscheduler/releases/tag/2.0.5)
-
-## 感谢贡献者
-
-感谢 Apache DolphinScheduler 2.0.5 版本的贡献者,贡献者 GitHub ID 列表如下(排名不分先后):
-
-<div align=center>
-
-<img src="/img/2022-3-7/4.png"/>
-
-</div>
-
diff --git a/blog/zh-cn/Awarded_most_popular_project_in_2021.md b/blog/zh-cn/Awarded_most_popular_project_in_2021.md
deleted file mode 100644
index 399768a..0000000
--- a/blog/zh-cn/Awarded_most_popular_project_in_2021.md
+++ /dev/null
@@ -1,56 +0,0 @@
-# Apache DolphinScheduler 获评 2021 年度「最受欢迎项目」!
-<div align=center>
-<img src="https://s1.imgpp.com/2022/01/07/_c449bb07189725ea562d5ba404504b8f_96119.md.jpg"/>
-</div>
-
-> 近日,由 OSCHINA 举办的「2021 OSC 中国开源项目」评选活动公布了评选结果。
->
-> 在广大用户和开源社区的喜爱和支持下,云原生分布式大数据调度系统 Apache DolphinScheduler 获评 2021 年度「OSCHINA 人气指数 Top 50 开源项目」和「最受欢迎项目」。
-
-## 获评「最受欢迎项目」
-<div align=center>
-<img src="https://s1.imgpp.com/2022/01/07/1.png"/>
-</div>
-
-<div align=center>
-<img src="https://s1.imgpp.com/2022/01/07/2.png"/>
-</div>
-
-
-
-今年,「2021 OSC 中国开源项目」活动设置了两轮投票环节,第一轮投票根据票数选出了「OSCHINA 人气指数 TOP 50 开源项目」。第二轮投票基于第一轮选出的 TOP 50 项目进行,并在此基础上通过投票选出了 30 个「最受欢迎项目」。
-在第一轮投票中,OSCHINA 根据票数选出「组织」项目 7 大分类(基础软件、云原生、大前端、DevOps、开发框架与工具、AI & 大数据、IoT & 5G)中每个分类的 TOP 5,Apache DolphinScheduler 在「云原生」大类中脱颖而出,凭借优秀的云原生能力入选。
-之后,经过第二轮投票的激烈角逐,Apache DolphinScheduler 再次胜出,获得「最受欢迎项目」奖项。
-
-<div align=center>
-<img src="https://s1.imgpp.com/2022/01/07/3-1.png"/>
-</div>
-
-中国开源软件生态蓬勃发展,近年来涌现出了一大批优秀的开源软件创企,他们不忘初心,深耕开源,回馈社区,为中国开源软件事业添砖加瓦,成为全球开源软件生态中不可忽视的重要力量。「OSC 中国开源项目评选」是开源中国(OSCHINA,OSC 开源社区)举办的国内最权威、最盛大的开源项目评选活动,旨在更好地展示国内开源现状,探讨国内开源趋势,激励国内开源人才,促进国内开源生态完善。
-
-
-## Apache DolphinScheduler:分布式工作流任务调度系统
-
-Apache DolphinScheduler 是 Apache 基金会孵化的顶级项目。作为新一代大数据任务调度系统,致力于“解决大数据任务之间错综复杂的依赖关系,使整个数据处理流程直观可见”。DolphinScheduler 以 DAG(有向无环图) 的方式将 Task 组装起来,可实时监控任务的运行状态,同时支持重试、从指定节点恢复失败、暂停及 Kill 任务等操作,并专注于可视化 DAG、调用高可用、丰富的任务类型、以来、任务日志/告警机制和补数 6 大能力。
-迄今为止,Apache DolphinScheduler 社区已经有 280+ 经验丰富的代码贡献者,110+ 非代码贡献者,其中也不乏其他 Apache 顶级项目的 PMC 或者 Committer。自创建以来,Apache DolphinScheduler 开源社区在不断发展壮大,微信用户群已达 6000+ 人。截止 2022 年 1 月,已经有 600 + 家公司及机构在生产环境中采用 Apache DolphinScheduler。
-最后,感谢 OSCHINA 对 Apache DolphinScheduler 以及其背后商业公司白鲸开源科技的认可,更感谢社区每一位参与者,开源之力滴水成河,Apache DolphinScheduler 社区的壮大离不开每一位贡献者的参与。社区将以此为鞭策,希望在更多小伙伴的助力之下,社区可以再上一层楼!
-
-## 参与贡献
-
-随着国内开源的迅猛崛起,Apache DolphinScheduler 社区迎来蓬勃发展,为了做更好用、易用的调度,真诚欢迎热爱开源的伙伴加入到开源社区中来,为中国开源崛起献上一份自己的力量,让本土开源走向全球。
-
-参与 DolphinScheduler 社区有非常多的参与贡献的方式,包括:
-
-**文档、翻译、答疑、测试、代码、实践文章、原理文章、会议分享等**
-
-贡献第一个PR(文档、代码) 我们也希望是简单的,第一个PR用于熟悉提交的流程和社区协作以及感受社区的友好度。
-
-- 社区汇总了以下适合新手的问题列表:https://github.com/apache/dolphinscheduler/issues/5689
-
-- 非新手问题列表:https://github.com/apache/dolphinscheduler/issues?q=is%3Aopen+is%3Aissue+label%3A%22volunteer+wanted%22
-
-- 如何参与贡献链接:https://dolphinscheduler.apache.org/zh-cn/community/development/contribute.html
-
-来吧,DolphinScheduler开源社区需要您的参与,为中国开源崛起添砖加瓦吧,哪怕只是小小的一块瓦,汇聚起来的力量也是巨大的。
-
-参与开源可以近距离与各路高手切磋,迅速提升自己的技能,如果您想参与贡献,我们有个贡献者种子孵化群,可以添加社区小助手微信(Leonard-ds) 手把手教会您( 贡献者不分水平高低,有问必答,关键是有一颗愿意贡献的心 )。添加小助手微信时请说明想参与贡献。
diff --git a/blog/zh-cn/Board_of_Directors_Report.md b/blog/zh-cn/Board_of_Directors_Report.md
deleted file mode 100644
index 9e9db9f..0000000
--- a/blog/zh-cn/Board_of_Directors_Report.md
+++ /dev/null
@@ -1,80 +0,0 @@
-# Apache DolphinScheduler 董事会报告:社区健康运行,Commit 增长 123%
-
-<div align=center>
-<img src="/img/2022-1-13/640.png"/>
-</div>
-
-
-> 自 2021 年 3 月 17 日从 Apache 孵化器毕业以来,Apache DolphinScheduler 不知不觉已经和社区一起经过了十个月的成长。在社区的共同参与下,Apache DolphinScheduler 在数次版本迭代后,蜕变为一个经过数百家企业生产环境检验的成熟调度系统产品。
-
-> 在将近一年的时间里,Apache DolphinScheduler 有了哪些进步?今天我们将通过这篇 Apache 报告,一起回顾这段时间发生在 Apache DolphinScheduler 及其社区中的变化。
-
-## 基本数据:
-
-成立: 2021 年 03 月 17 日(十个月前)
-Chair: 代立冬
-下次报告日期: 2022 年 1 月 19 日(星期三)
-社区健康评分( Chi ): 7.55(健康)
-
-## 项目组成:
-* 目前该项目中有 39 个 committer 和 16 个 PMC 成员。
-* committer 与 PMC 的比例大约是 5 : 2 。
-## 与上季度相比,社区的变化:
-* 无新的 PMC 成员加入。最新加入的 PMC 成员为 Calvin Kirs,加入时间 2021 - 05 - 07 。
-* ShunFeng Cai 于 2021 年 12 月 18 日新晋 committer。
-* Zhenxu Ke 于 2021 年 12 月 12 日新晋 committer。
-* Wang Xingjie 于 2021 年 11 月 24 日新晋 committer。
-* Yi zhi Wang 于 2021 年 12 月 15 日新晋 committer。
-* Jiajie Zhong于 2021 年 12 月 12 日新晋 committer。
-
-## 社区健康指标:
-
-* 邮件列表趋势
-* commit 数量
-* GitHub PR 数量
-* GitHub issue
-* 最活跃的 GitHub issues/ PR
-## 邮件列表趋势:
-Dev@DolphinScheder.apache.org 在过去的一个季度,流量增长 64%( 297 封电子邮件,上季度为 181 封):
-
-<div align=center>
-<img src="/img/2022-1-13/640-1.png"/>
-</div>
-
-
-
-## commit 数量:
-* 上季度共 972 个 commit(增长 123 %)
-* 上季度共新增 88 个代码贡献者(增长 25 %)
-<div align=center>
-<img src="/img/2022-1-13/640-2.png"/>
-</div>
-
-
-## GitHub PR 数量:
-* GitHub 上新开 824 个 PR ,较上季度(增长 89 %)
-* GitHub 上关闭 818 个 PR ,较上季度(增长 100 %)
-<div align=center>
-<img src="/img/2022-1-13/640-3.png"/>
-</div>
-
-## GitHub issues:
-GitHub 上新开 593 个 issues, 较上季度(增长 90 %)
-GitHub 上关闭 608 个 issue,较上季度(增长 155 %)
-
-<div align=center>
-<img src="/img/2022-1-13/640-4.png"/>
-</div>
-
-## 讨论最热烈的 GitHub issues/ PR :
-
-* dolphinscheduler/pull/6894[Improvement][Logger]Logger server integrate into worker server(15 comments)
-* dolphinscheduler/pull/6744[Bug][SnowFlakeUtils] fix snowFlake bug(15 comments)
-* dolphinscheduler/pull/6674[Feature][unittest] Recover UT in AlertPluginManagerTest.java [closes: #6619](15 comments)
-* dolphinscheduler/issues/7039[Bug] [Task Plugin] hive sql execute failed(14 comments)
-* dolphinscheduler/pull/6782[improvement] improve install.sh if then statement(13 comments)
-* dolphinscheduler/issues/7485[Bug] [dolphinscheduler-datasource-api] Failed to create hive datasource using ZooKeeper way in 2.0.1(13 comments)
-* dolphinscheduler/pull/7214[DS-7016][feat] Auto create workflow while import sql script with specific hint(12 comments)
-* dolphinscheduler/pull/6708[FIX-#6505][Dao] upgrade the MySQL driver package for building MySQL jdbcUrl(12 comments)
-* dolphinscheduler/pull/7515[6696/1880][UI] replace node-sass with dart-sass(12 comments)
-* dolphinscheduler/pull/6913Use docker.scarf.sh to track docker user info(12 comments)
\ No newline at end of file
diff --git a/blog/zh-cn/DAG.md b/blog/zh-cn/DAG.md
deleted file mode 100644
index d798b59..0000000
--- a/blog/zh-cn/DAG.md
+++ /dev/null
@@ -1,167 +0,0 @@
-## 大数据工作流任务调度--有向无环图(DAG)之拓扑排序
-
-### 回顾基础知识:
-
-- 图的遍历
-
- 图的遍历是指从图中的某一个顶点出发,按照某种搜索方法沿着图中的边对图中的所有顶点访问一次且仅访问一次。
-
- **注意树是一种特殊的图,所以树的遍历实际上也可以看作是一种特殊的图的遍历**
-
-- 图的遍历主要有两种算法
-
- - 广度优先搜索(Breadth First Search,BFS)
-
- 基本思想:首先访问起始顶点 v,接着由 v 出发,依次访问 v 的各个未访问过的邻接顶点 w1, w2 , … ,wi ,然后依次访问 w1, w2 , … , w~i~ 的所有未被访问过的邻接顶点;再从这些访问过的顶点出发,访问它们所有未被访问过的邻接顶点,直至图中所有顶点都被访问过为止。若此时图中尚有顶点未被访问,则另选图中一个未曾被访问过的顶点作为起始点,重复上述过程,直至图中所有的顶点都被访问到为止。
-
-
-
- - 深度优先搜索(Depth First Search,DFS)
-
- 基本思想:首先访问图中某一起始顶点 v,然后由 v 出发,访问与 v 邻接且未被访问过的任一顶点 w1,再访问与 w1 邻接且未被访问的任一顶点 w2 …… 重复上述过程。当不能再继续向下访问时,依次退回到最近被访问的顶点,若它还有邻接顶点未被访问过,则从该点开始继续上述搜索过程,直至图中所有顶点均被访问过为止。
-
-- 举例说明
-
- 如下图,如果采用 广度优先搜索(BFS)遍历如下 `1 2 5 3 4 6 7`,如果采用深度优先搜索(DFS)遍历如下 `1 2 3 4 5 6 7`。
-
- 
-
-### 拓扑排序(Topological Sorting)
-
-**维基百科上拓扑排序的定义为**
-
-对于任何有向无环图 (Directed Acyclic Graph,DAG) 而言,其拓扑排序为其所有结点的一个线性排序(同一个有向图可能存在多个这样的结点排序)。该排序满足这样的条件——对于图中的任意两个结点 U 和 V,若存在一条有向边从 U 指向 V,则在拓扑排序中 U 一定出现在 V 前面。
-
-**通俗来讲:拓扑排序是一个有向无环图 (DAG) 的所有顶点的线性序列, 该序列必须满足两个条件**
-
-- 每个顶点出现且只出现一次。
-- 若存在一条从顶点 A 到顶点 B 的路径,那么在序列中顶点 A 出现在顶点 B 的前面。
-
-**如何找出它的拓扑排序呢?这里说一种比较常用的方法:**
-
-在介绍这个方法之前有必要补充下有向图结点的入度 (indegree) 和出度 (outdegree) 的概念。假设有向图中不存在起点和终点为同一结点的有向边,则:
-
-入度:设有向图中有一结点 V,其入度即为当前所有从其他结点出发,终点为 V 的的边的数目。也就是所有指向V的有向边的数目。
-
-出度:设有向图中有一结点 V,其出度即为当前所有起点为 V,指向其他结点的边的数目。也就是所有由 V 发出的边的数目。
-
-1. 从 DAG 图中选择一个入度为 0 的顶点并输出。
-2. 从图中删除该顶点和所有以它为起点的有向边。
-3. 重复 1 和 2 直到当前的 DAG 图为空或当前图中不存在入度为 0 的顶点为止。后一种情况说明有向图中必然存在环。
-
-**例如下面这个 DAG 图:**
-
-
-
-
-|结点|入度结点|出度结点|入度结点个数|出度结点个数|
-|----|----|----|----|----|
-|结点1|0|结点2,结点4|0个|2个|
-|结点2|结点1|结点3,结点4|1个|2个|
-|结点3|结点2,结点4|结点5|2个|1个|
-|结点4|结点1,结点2|结点3,结点5|2个|2个|
-|结点5|结点3,结点4|0|2个|0个|
-
-
-
-
-它的拓扑排序流程为:
-
-于是,得到拓扑排序后的结果是: {1,2,4,3,5} 。
-
-如果没有结点 2 —> 结点 4 的这个箭头,那么如下:
-
-
-
-我们可以得到它的拓扑排序为:{1,2,4,3,5} 或者 {1,4,2,3,5} ,即对同一 DAG 图来说,它的拓扑排序结果可能存在多个。
-
-拓扑排序主要用来解决有向图中的依赖问题。
-
-**在讲到实现的时候,有必要插以下内容:**
-
-由此我们可以进一步得出一个改进的深度优先遍历或广度优先遍历算法来完成拓扑排序。以广度优先遍历为例,这一改进后的算法与普通的广度优先遍历唯一的区别在于我们应当保存每一个结点对应的入度,并在遍历的每一层选取入度为 0 的结点开始遍历(而普通的广度优先遍历则无此限制,可以从该吃呢个任意一个结点开始遍历)。这个算法描述如下:
-
-1. 初始化一个 Map 或者类似数据结构来保存每一个结点的入度。
-2. 对于图中的每一个结点的子结点,将其子结点的入度加 1。
-3. 选取入度为 0 的任意一个结点开始遍历,并将该节点加入输出。
-4. 对于遍历过的每个结点,更新其子结点的入度:将子结点的入度减 1。
-5. 重复步骤 3,直到遍历完所有的结点。
-6. 如果无法遍历完所有的结点,则意味着当前的图不是有向无环图。不存在拓扑排序。
-
-**广度优先遍历拓扑排序的核心 Java 代码如下:**
-
-```java
-public class TopologicalSort {
- /**
- * 判断是否有环及拓扑排序结果
- *
- * 有向无环图(DAG)才有拓扑(topological)排序
- * 广度优先遍历的主要做法:
- * 1、遍历图中所有的顶点,将入度为0的顶点入队列。
- * 2、从队列中poll出一个顶点,更新该顶点的邻接点的入度(减1),如果邻接点的入度减1之后等于0,则将该邻接点入队列。
- * 3、一直执行第2步,直到队列为空。
- * 如果无法遍历完所有的结点,则意味着当前的图不是有向无环图。不存在拓扑排序。
- *
- *
- * @return key返回的是状态, 如果成功(无环)为true, 失败则有环, value为拓扑排序结果(可能是其中一种)
- */
- private Map.Entry<Boolean, List<Vertex>> topologicalSort() {
- //入度为0的结点队列
- Queue<Vertex> zeroIndegreeVertexQueue = new LinkedList<>();
- //保存结果
- List<Vertex> topoResultList = new ArrayList<>();
- //保存入度不为0的结点
- Map<Vertex, Integer> notZeroIndegreeVertexMap = new HashMap<>();
-
- //扫描所有的顶点,将入度为0的顶点入队列
- for (Map.Entry<Vertex, VertexInfo> vertices : verticesMap.entrySet()) {
- Vertex vertex = vertices.getKey();
- int inDegree = getIndegree(vertex);
-
- if (inDegree == 0) {
- zeroIndegreeVertexQueue.add(vertex);
- topoResultList.add(vertex);
- } else {
- notZeroIndegreeVertexMap.put(vertex, inDegree);
- }
- }
-
- //扫描完后,没有入度为0的结点,说明有环,直接返回
- if(zeroIndegreeVertexQueue.isEmpty()){
- return new AbstractMap.SimpleEntry(false, topoResultList);
- }
-
- //采用topology算法, 删除入度为0的结点和它的关联边
- while (!zeroIndegreeVertexQueue.isEmpty()) {
- Vertex v = zeroIndegreeVertexQueue.poll();
- //得到相邻结点
- Set<Vertex> subsequentNodes = getSubsequentNodes(v);
-
- for (Vertex subsequentVertex : subsequentNodes) {
-
- Integer degree = notZeroIndegreeVertexMap.get(subsequentVertex);
-
- if(--degree == 0){
- topoResultList.add(subsequentVertex);
- zeroIndegreeVertexQueue.add(subsequentVertex);
- notZeroIndegreeVertexMap.remove(subsequentVertex);
- }else{
- notZeroIndegreeVertexMap.put(subsequentVertex, degree);
- }
-
- }
- }
-
- //notZeroIndegreeVertexMap如果为空, 表示没有环
- AbstractMap.SimpleEntry resultMap = new AbstractMap.SimpleEntry(notZeroIndegreeVertexMap.size() == 0 , topoResultList);
- return resultMap;
-
- }
-}
-```
-
-*注意输出结果是该图的拓扑排序序列之一。*
-
-每次在入度为 0 的集合中取顶点,并没有特殊的取出规则,取顶点的顺序不同会得到不同的拓扑排序序列(如果该图有多种排序序列)。
-
-由于输出每个顶点的同时还要删除以它为起点的边。如果图有 V 个顶点,E 条边,则一般该算法的时间复杂度为 O(V+E)。这里实现的算法最终 key 返回的是状态, 如果成功(无环)为 true, 失败则有环, 无环时 value 为拓扑排序结果(可能是其中一种)。注意输出结果是该图的拓扑排序序列之一。每次在入度为 0 的集合中取顶点,并没有特殊的取出规则,取顶点的顺序不同会得到不同的拓扑排序序列(如果该图有多种排序序列)。
diff --git a/blog/zh-cn/DS-2.0-alpha-release.md b/blog/zh-cn/DS-2.0-alpha-release.md
deleted file mode 100644
index 281a633..0000000
--- a/blog/zh-cn/DS-2.0-alpha-release.md
+++ /dev/null
@@ -1,99 +0,0 @@
-# 重构、插件化、性能提升 20 倍,Apache DolphinScheduler 2.0 alpha 发布亮点太多!
-
-<div align='center'><img src="https://s1.imgpp.com/2021/11/16/a920be6733a3d99af38d1cdebfcbb3ff.md.png"></div>
-
-
-社区的小伙伴们,好消息!经过 100 多位社区贡献者近 10 个月的共同努力,我们很高兴地宣布 Apache DolphinScheduler 2.0 alpha 发布。这是 DolphinScheduler 自进入 Apache 以来的首个大版本,进行了多项关键更新和优化,是 DolphinScheduler 发展中的里程碑。
-
-DolphinScheduler 2.0 alpha 主要重构了 Master 的实现,大幅优化了元数据结构和处理流程,增加了 SPI 插件化等能力,在性能上提升 20 倍。同时,新版本设计了全新的 UI 界面,带来更好的用户体验。另外,2.0 alpha 还新添加和优化了一些社区呼声极高的功能,如参数传递、版本控制、导入导出等功能。
-
-注意:当前 alpha 版本还未支持自动升级,我们将在下个版本中支持这一功能。
-
-
-
-**2.0 alpha 下载地址:https://dolphinscheduler.apache.org/en-us/download/download.html**
-
-
-## 优化内核,性能提升 20 倍
-
-相较于 DolphinScheduler 1.3.8,同等硬件配置下(3 台 8 核 16 G),2.0 alpha 吞吐性能提升 20 倍,这主要得益于 Master 的重构,Master 执行流程和优化了工作流处理流程等,包括:
-- 重构 Master 的执行流程,将之前状态轮询监控改为事件通知机制,大幅减轻了数据库的轮询压力;
-- 去掉全局锁,增加了 Master 的分片处理机制,将顺序读写命令改为并行处理,增强了 Master 横向扩展能力;
-- 优化工作流处理流程,减少了线程池的使用,大幅提升单个 Master 处理的工作流数量;
-- 增加缓存机制,大幅减少数据库的操作次数;
-- 优化数据库连接方式,极大地缩减数据库操作耗时;
-- 简化处理流程,减少处理过程中不必要的耗时操作。
-
-
-
-## 优化 UI 组件,全新的 UI 界面
-
-<div align='center'><img src="https://s1.imgpp.com/2021/11/16/4e4024cbddbe3113f730c5e67f083c4f.md.png"></div>
-
-<div align='center'><img src="https://s1.imgpp.com/2021/11/16/75e002b21d827aee9aeaa3922c20c13f.md.png"></div>
-
-
-<center>
- UI 界面对比:1.3.9(上) VS. 2.0 alpha(下)
-</center>
-
-
->
-2.0 UI 重要优化在以下几个方面:
-
-
-- 优化组件显示:界面更简洁,流程显示更清晰,一目了然;
-- 突出重点内容:鼠标点击任务框,显示任务详情信息;
-- 增强可识别性:左侧工具栏标注名称,使工具更易识别,便于操作;
-- 调整组件顺序:调整组件排列顺序,更符合用户习惯。
-
-
-除了性能与 UI 上的变化外,DolphinScheduler 也新增和优化了 20 多项功能
-及 BUG 修复。
-
-
-## 新功能列表
-<div align='center'><img src="https://s1.imgpp.com/2021/11/16/WX20211116-164031.md.png"></div>
-
-
-## 优化项
-
-<div align='center'><img src="https://s1.imgpp.com/2021/11/16/WX20211116-164042.md.png"></div>
-
-
-
-## Bug 修复
-<div align='center'><img src="https://s1.imgpp.com/2021/11/16/WX20211116-164059.md.png"></div>
-
-
-
-## 感谢贡献者
-
-
-DolphinScheduler 2.0 alpha 的发布凝聚了众多社区贡献者的智慧和力量,是他们的积极参与和极大的热情开启了 DolphinScheduler 2.0 时代!
-
-非常感谢 100+ 位(GitHub ID)社区小伙伴的贡献,期待更多人能够加入 DolphinScheduler 社
-区共建,为打造一个更好用的大数据工作流调度平台贡献自己的力量!
-
-<div align='center'><img src="https://s1.imgpp.com/2021/11/16/8926d45ead1f735e8cfca0e8142b315f.md.png"></div>
-
-
-<center>2.0 alpha 贡献者名单</center>
-
-## 加入我们
-
-随着国内开源的迅猛崛起,Apache DolphinScheduler 社区迎来蓬勃发展,为了做更好用、易用的调度,真诚欢迎热爱开源的伙伴加入到开源社区中来,为中国开源崛起献上一份自己的力量,让本土开源走向全球。
-
-贡献第一个PR(文档、代码) 我们也希望是简单的,第一个PR用于熟悉提交的流程和社区协作以及感受社区的友好度。
-
-社区汇总了以下适合新手的问题列表:https://github.com/apache/dolphinscheduler/issues/5689
-
-进阶问题列表:https://github.com/apache/dolphinscheduler/issues?q=is%3Aopen+is%3Aissue+label%3A%22volunteer+wanted%22
-
-如何参与贡献链接:https://dolphinscheduler.apache.org/zh-cn/community/development/contribute.html
-
-来吧,DolphinScheduler开源社区需要您的参与,为中国开源崛起添砖加瓦吧,哪怕只是小小的一块瓦,汇聚起来的力量也是巨大的。
-
-参与开源可以近距离与各路高手切磋,迅速提升自己的技能,如果您想参与贡献,我们有个贡献者种子孵化群,可以添加社区小助手微信(Leonard-ds) 手把手教会您( 贡献者不分水平高低,有问必答,关键是有一颗愿意贡献的心 )。添加小助手微信时请说明想参与贡献。
-
-来吧,开源社区非常期待您的参与。
diff --git a/blog/zh-cn/DS_architecture_evolution.md b/blog/zh-cn/DS_architecture_evolution.md
deleted file mode 100644
index 5966827..0000000
--- a/blog/zh-cn/DS_architecture_evolution.md
+++ /dev/null
@@ -1,135 +0,0 @@
-# Apache DolphinScheduler 架构演进及开源经验分享
-
-## 引言
-来自 eBay 的文俊同学在近期的上海开源大数据 Meetup 上做了十分精彩的 “Apache DolphinScheduler 的架构演进” 分享。本次分享有近 200 人参与,在线观看次数超过 2,500 次
-
-## 演讲者介绍
-
-阮文俊,eBay 开发工程师,DolphinScheduler 贡献者。
-
-视频分享参见
-[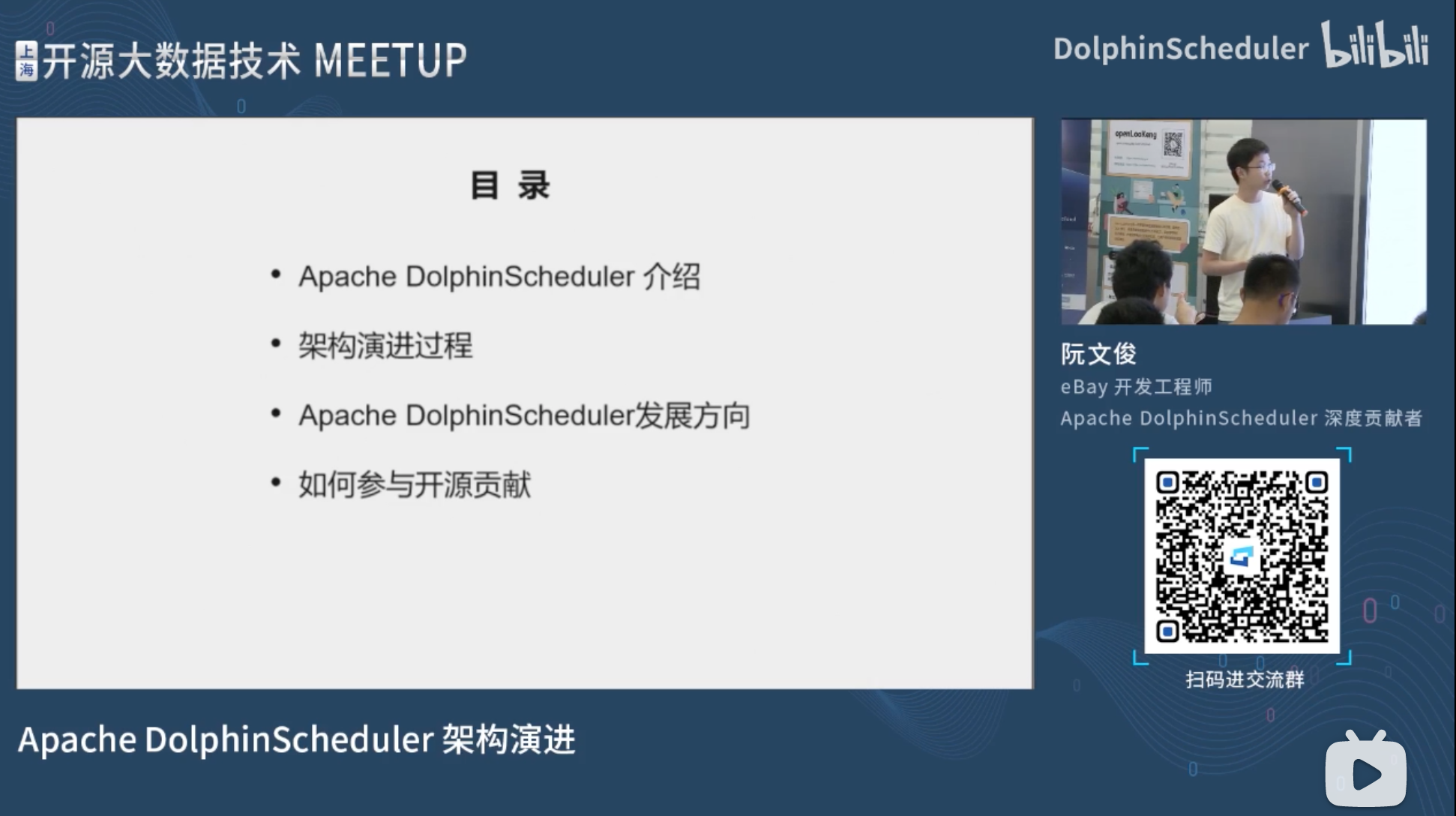](https://www.bilibili.com/video/BV11M4y1T7VT)
-
-
-
-## Apache DolphinScheduler介绍
-
-Apache DolphinScheduler是一个分布式去中心化,易扩展的可视化DAG工作流任务调度平台。致力于解决数据处理流程中错综复杂的依赖关系,使调度系统在数据处理流程中开箱即用。DolphinScheduler以有向无环图的方式将任务连接起来,可实时监控任务的运行状态,同时支持取消、暂停、恢复、从指定任务节点重跑等操作。
-
-DolphinScheduler具有以下几个优良功能特性:
-
-- **Cloud Native** — 支持多云/数据中心工作流管理,也支持 Kubernetes、Docker 部署和自定义任务类型,分布式调度,整体调度能力随集群规模线性增长
-
-- **高可靠与高可扩展性** — 去中心化的多 Master 多 Worker 设计架构,支持服务动态上下线,自我容错与调节能力
-
-- **支持多租户**
-
-- **丰富的使用场景** — 包括流、暂停、恢复操作,以及额外的任务类型,如 Spark、Hive、MR、Shell、Python、Flink 以及 DS 独有的子工作流、任务依赖设计,扩展点采用插件化的实现方式
-
-- **简单易用** — 所有流程定义操作可视化编排,定义关键信息一目了然,一键部署
-
-关于DolphinSheduler更多功能介绍和开发文档请查阅官网详细信息 https://dolphinscheduler.apache.org
-
-
-## 架构演进过程
-
-**1.2.x架构**
-
-DolphinScheduler最初进入Apache孵化器的版本是1.2,在这一版本中采用的架构由以下几个重要部分组成:
-
-- 去中心化的master节点,负责工作流调度、DAG任务切分、任务提交监控和监听其它节点健康状态等任务
-- 去中心化的worker节点,负责执行任务和维护任务的生命周期等
-- 数据库,存储工作流元数据,运行实例数据
-- Zookeeper,主要负责注册中心、分布式锁、任务队列等工作任务
-
-1.2版本基本实现了高可靠的工作流调度系统,但是也存在多个问题:
-
-- **重量级的worker**,worker节点需要负责多种任务
-- 异步派发任务会导致**任务执行延迟**
-- 由于masker和worker都需要依赖数据库,导致数据库压力大
-
-[]
-
-
-
-**1.3.x架构**
-
-针对1.2版本存在的问题,1.3架构进行了如下改进:
-
-- 去任务队列,保证master节点同步派发任务,降低任务执行延迟
-- 轻量级worker,worker节点只负责执行任务,单一化worker职责
-- 减小数据库压力,worker不再连接数据库
-- 采用多任务负载均衡策略,master根据worker节点资源使用情况分配任务,提高worker资源利用率
-
-这些改进有效改进了1.2版本的缺陷,但仍存在一些问题,例如:
-
-- master调度工作流时需要依赖**分布式锁**,导致工作流吞吐量难以提升
-- 因为需要创建大量线程池,多数线程处于轮询数据库,导致**master资源利用率低**
-- master轮询数据库,仍然导致**数据库压力大**
-- 各组件存在**耦合**情况
-
-
-
-
-
-**2.0架构**
-
-针对1.3版本的缺陷,2.0架构进一步做出改进:
-
-- **去分布式锁**,对master进行分区编号,实现错位查询数据库,避免多个节点同时访问同一个工作流造成的冲突问题
-- 重构master线程模型,对所有工作流使用**统一的线程池**
-- 重构数据库中**DAG元数据**模型
-- **彻底的插件化**,所有扩展点都采用插件化实现
-- **数据血缘关系分析**
-
-#### 1 去分布式锁
-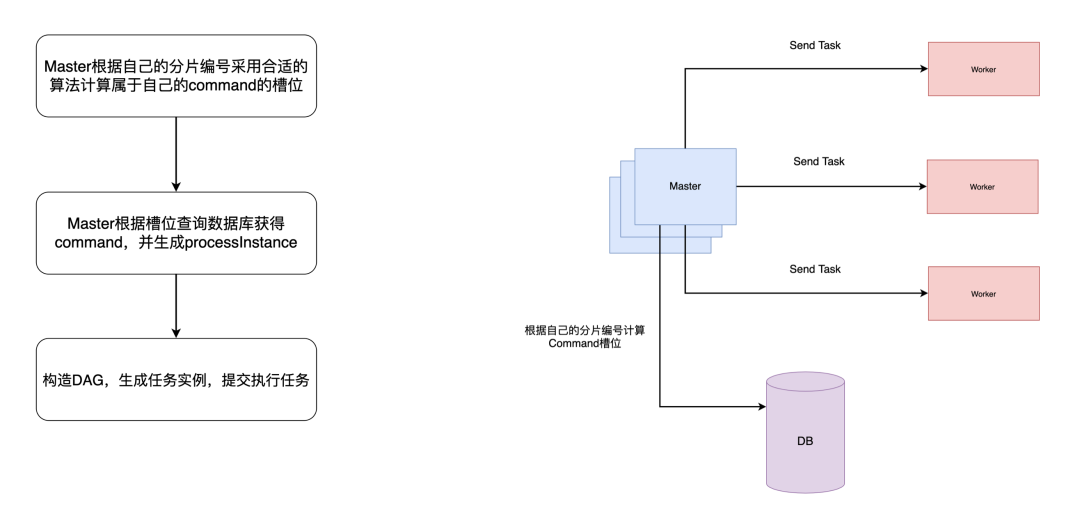
-
-#### 2 重构 master 中的线程模型
-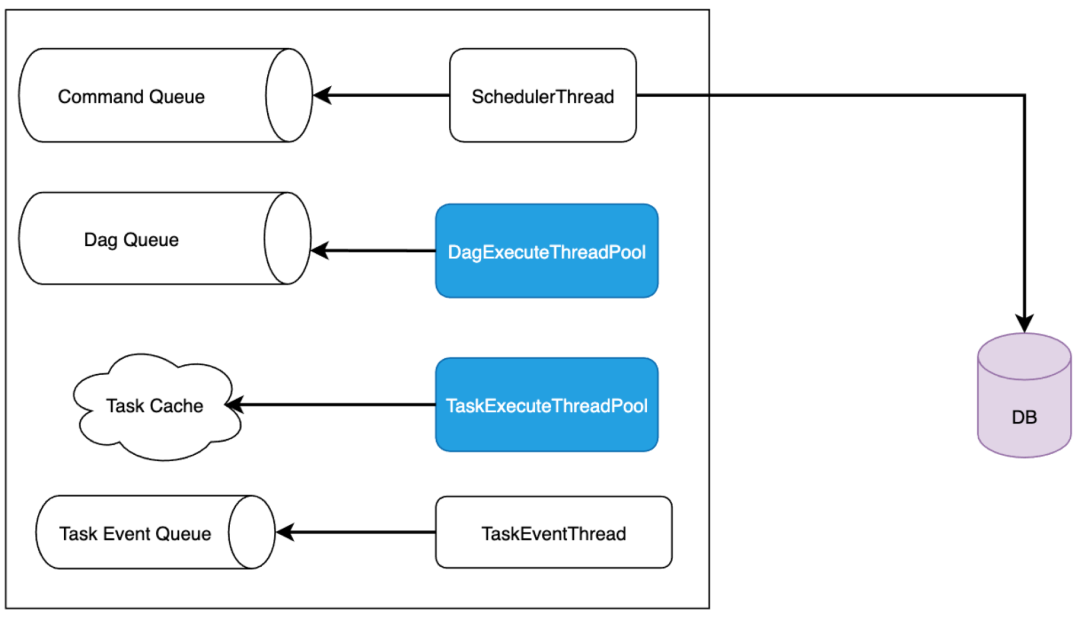
-SchedulerThread 负责从数据库中查询 Command 并提交到 Command Queue
-
-DagExecuteThreadPool 从 Command Queue 中取 command,并构造 DAG实例添加到 DAG 队列,进行处理,当前 DAG 没有未执行的任务,则当前 DAG 执行结束
-
-TaskExecuteThreadPool 提交任务给 Worker
-
-TaskEventThread 监听任务事件队列,修改任务状态
-
-#### 3 彻底的插件化
-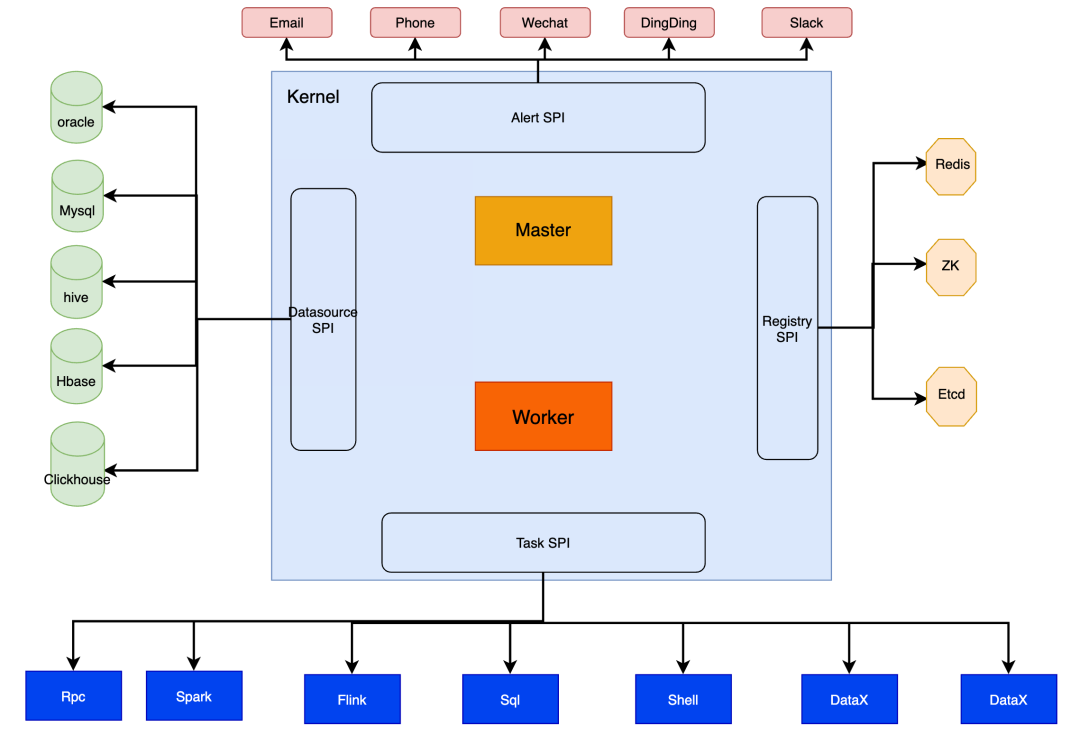
-
-所有扩展点都采用插件化实现
-
-告警SPI
-
-注册中心SPI
-
-资源存储SPI
-
-任务插件SPI
-
-数据源SPI
-
-……
-
-
-## Apache DolphinScheduler发展方向
-
-开发者阮文俊针对dolphinsheduler的未来发展方向,也分享了一些看法:
-
-- 系统更稳、速度更快(高吞吐、低延迟、智能化运维、高可用)
-- 支持更多的任务集成(深度学习任务、CI/CD等其它系统集成、存储过程和数据质量任务、容器调度任务、复杂调度场景等)
-- 轻量化dolphinscheduler内核,提供基础调度服务
-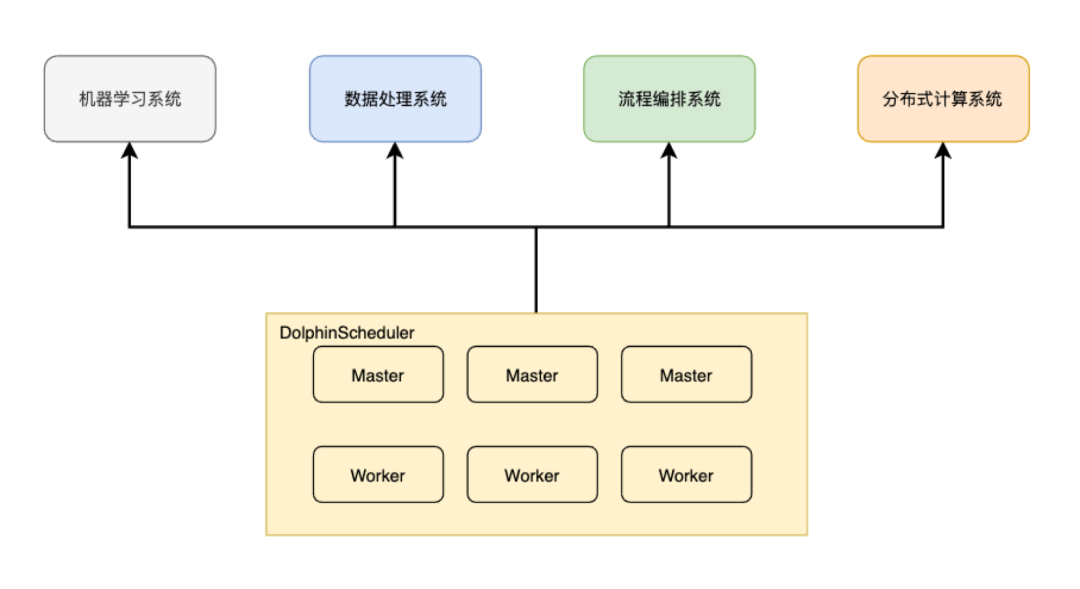
-
-
-## 如何参与开源贡献
-
-最后,开发者阮文俊针对入门新手如何参与开源贡献的问题,提出了宝贵的指导意见:
-
-- 从小事做起,积累开发经验
-- 关注社区动态,积极参与讨论,更好融入社区
-- 坚持开源精神,乐于帮助他人
-- 持之以恒
-
-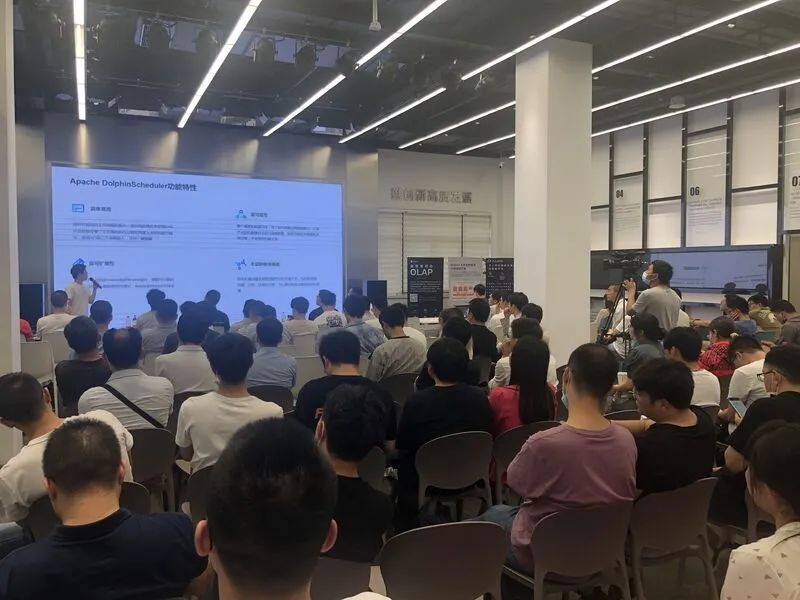
diff --git a/blog/zh-cn/DS_run_in_windows.md b/blog/zh-cn/DS_run_in_windows.md
deleted file mode 100644
index d2ec2de..0000000
--- a/blog/zh-cn/DS_run_in_windows.md
+++ /dev/null
@@ -1,107 +0,0 @@
-# DolphinScheduler 在 Windows 本地搭建开发环境,源码启动
-
-如果您对本地开发的视频教程感兴趣的话,也可以跟着视频来一步一步操作:
-[](https://www.bilibili.com/video/BV1hf4y1b7sX)
-
-1. ## 下载源码
-
- 官网 :https://dolphinscheduler.apache.org/zh-cn/index.html
-
- 地址 :https://github.com/apache/dolphinscheduler.git
-
- 这里选用 1.3.6-release 分支。
-
-2. ## windows安装zk
-
- 1. 下载zk https://www.apache.org/dyn/closer.lua/zookeeper/zookeeper-3.6.3/apache-zookeeper-3.6.3-bin.tar.gz
-
- 2. 解压apache-zookeeper-3.6.3-bin.tar.gz
-
- 3. 在zk的目录下新建data、log文件夹
-
- 4. 将conf目录下的zoo_sample.cfg文件,复制一份,重命名为zoo.cfg,修改其中数据和日志的配置,如:
-
- ~~~properties
- dataDir=D:\\code\\apache-zookeeper-3.6.3-bin\\data
- dataLogDir=D:\\code\\apache-zookeeper-3.6.3-bin\\log
- ~~~
-
- 5. 在bin中运行 zkServer.cmd,然后运行zkCli.cmd 查看zk运行状态,可以查看zk节点信息即代表安装成功。
-
-3. ## 搭建后端环境
-
- 1. 新建一个自我调试的mysql库,库名可为 :dolphinschedulerKou
-
- 2. 把代码导入idea,修改根项目中 pom.xml,将 mysql-connector-java 依赖的 scope 修改为 compile
-
- 3. 修改 dolphinscheduler-dao 模块的 datasource.properties
-
- ~~~properties
- # mysql
- spring.datasource.driver-class-name=com.mysql.jdbc.Driver
- spring.datasource.url=jdbc:mysql://192.168.2.227:3306/dolphinschedulerKou?useUnicode=true&characterEncoding=UTF-8
- spring.datasource.username=root
- spring.datasource.password=Dm!23456
- ~~~
-
- 4. 刷新 dao 模块,运行 org.apache.dolphinscheduler.dao.upgrade.shell.CreateDolphinScheduler 的 main 方法,自动插入项目所需的表和数据
-
- 5. 修改 dolphinscheduler-service 模块的 zookeeper.properties
-
- ~~~properties
- zookeeper.quorum=localhost:2181
- ~~~
-
- 6. 在logback-worker.xml、logback-master.xml、logback-api.xml中添加控制台输出
-
- ~~~xml
- <root level="INFO">
- <appender-ref ref="STDOUT"/> <!-- 添加控制台输出 -->
- <appender-ref ref="APILOGFILE"/>
- <appender-ref ref="SKYWALKING-LOG"/>
- </root>
- ~~~
-
-
-
- 7. 启动 MasterServer,执行 org.apache.dolphinscheduler.server.master.MasterServer 的 main 方法,需要设置 VM Options:
-
- ~~~
- -Dlogging.config=classpath:logback-master.xml -Ddruid.mysql.usePingMethod=false
- ~~~
-
- 8. 启动WorkerServer,执行org.apache.dolphinscheduler.server.worker.WorkerServer的 main方法,需要设置 VM Options:
-
- ~~~
- -Dlogging.config=classpath:logback-worker.xml -Ddruid.mysql.usePingMethod=false
- ~~~
-
- 9. 启动 ApiApplicationServer,执行 org.apache.dolphinscheduler.api.ApiApplicationServer 的 main 方法,需要设置 VM Options:
-
- ~~~
- -Dlogging.config=classpath:logback-api.xml -Dspring.profiles.active=api
- ~~~
-
- 10. 如果需要用到日志功能,执行 org.apache.dolphinscheduler.server.log.LoggerServer 的main 方法。
-
- 11. 后端swagger地址 :http://localhost:12345/dolphinscheduler/doc.html?language=zh_CN&lang=cn
-
-4. ## 搭建前端环境
-
- 1. 本机安装node(不再赘述)
-
- 2. 进入 dolphinscheduler-ui,运行
-
- ~~~shell
- npm install
- npm run start
- ~~~
-
- 3. 访问 [http://localhost:8888](http://localhost:8888/)
-
- 4. 登录管理员账号
-
- > 用户:admin
- >
- > 密码:dolphinscheduler123
-
diff --git a/blog/zh-cn/DolphinScheduler_Kubernetes_Technology_in_action.md b/blog/zh-cn/DolphinScheduler_Kubernetes_Technology_in_action.md
deleted file mode 100644
index ee4a904..0000000
--- a/blog/zh-cn/DolphinScheduler_Kubernetes_Technology_in_action.md
+++ /dev/null
@@ -1,457 +0,0 @@
-# Apache DolphinScheduler在Kubernetes体系中的技术实战
-
-作者 | 杨滇,深圳交通中心 数据和算法平台架构师
-
-## Kubernetes技术体系给Apache DolphinScheduler带来的技术新特性
-
-Apache DolphinScheduler是当前非常优秀的分布式易扩展的可视化工作流任务调度平台。
-
-基于笔者所在公司业务的特性,阐述我们使用 Kubernetes 作为Apache DolphinScheduler的技术底座的原因:
-
-+ 各类独立部署项目,需要快速建立开发环境和生产环境;
-+ 项目环境互联网访问受限,服务器只能使用离线的安装方式;
-+ 尽可能统一的安装配置的信息,减少多个项目配置的异常;
-+ 与对象存储技术的结合,统一非结构化数据的技术;
-+ 便捷的监控体系,与现有监控集成;
-+ 多种调度器的混合使用;
-+ 全自动的资源调整能力;
-+ 快速的自愈能力;
-
-本文的案例都是基于Apache DolphinScheduler1.3.9版本为基础。Hadoop
-
-## 基于helm工具的自动化高效部署方式
-
-首先,我们介绍基于官网提供的helm的安装方式。Helm 是查找、分享和使用软件构建 Kubernetes 的最优方式。也是云原生CNCF的毕业项目之一。
-
-<div align=center>
-<img src="/img/2022-02-22/2.png"/>
-</div>
-
-海豚的官网和GitHub上有非常详细的配置文件和案例。这里我们重点介绍一些社区中经常出现的咨询和问题。
-
-官网文档地址 https://dolphinscheduler.apache.org/zh-cn/docs/1.3.9/user_doc/kubernetes-deployment.html
-
-GitHub文件夹地址 https://github.com/apache/dolphinscheduler/tree/1.3.9-release/docker/kubernetes/dolphinscheduler/
-
-+ 在value.yaml文件中修改镜像,以实现离线安装(air-gap install);
- https://about.gitlab.com/topics/gitops/
- ~~~yaml
- image:
- repository: "apache/dolphinscheduler"
- tag: "1.3.9"
- pullPolicy: "IfNotPresent"
- ~~~
-
- 针对公司内部安装好的harbor,或者其他公有云的私仓,进行pull,tag,以及push。这里我们假定私仓地址是harbor.abc.com,你所在构建镜像的主机已经进行了docker login harbor.abc.com, 且已经建立和授权私仓下面新建apache项目。
-
- 执行shell命令
-
- ~~~shell
- docker pull apache/dolphinscheduler:1.3.9
- dock tag apache/dolphinscheduler:1.3.9 harbor.abc.com/apache/dolphinscheduler:1.3.9
- docker push apache/dolphinscheduler:1.3.9
- ~~~
-
- 再替换value文件中的镜像信息,这里我们推荐使用Always的方式拉取镜像,生产环境中尽量每次去检查是否是最新的镜像内容,保证软件制品的正确性。此外,很多同学会把tag写成latest(制作镜像不写tag信息,这样在生产环境非常危险,任何人push了镜像,就相当于改变了latest的tag的镜像,而且也无法判断latest是什么版本,所以建议要明确每次发版的tag,并且使用Always。
-
- ~~~yaml
- image:
- repository: "harbor.abc.com/apache/dolphinscheduler"
- tag: "1.3.9"
- pullPolicy: "Always"GitHub
- ~~~
-
- 把 https://github.com/apache/dolphinscheduler/tree/1.3.9-release/docker/kubernetes/dolphinscheduler/ 整个目录copy到可以执行helm命令的主机,然后按照官网执行
-
-
- ~~~shell
- kubectl create ns ds139Git
- MySQL install dolphinscheduler . -n ds139
- ~~~
-
- 即可实现离线安装。
-
-+ 集成DataX MySQL Oracle客户端组件,首先下载以下组件
-
- https://repo1.maven.org/maven2/MySQL/MySQL-connector-java/5.1.49/MySQL-connector-java-5.1.49.jar
-
- https://repo1.maven.org/maven2/com/Oracle/database/jdbc/ojdbc8/
-
- https://GitHub.com/alibaba/DataX/blob/master/userGuid.md 根据提示进行编译构建,文件包位于 {DataX_source_code_home}/target/DataX/DataX/
-
- 基于以上plugin组件新建dockerfile,基础镜像可以使用已经push到私仓的镜像。
-
- ~~~dock
- FROM harbor.abc.com/apache/dolphinscheduler:1.3.9
- COPY *.jar /opt/dolphinscheduler/lib/
- RUN mkdir -p /opt/soft/DataX
- COPY DataX /opt/soft/DataX
- ~~~
-
- 保存dockerfile,执行shell命令
-
- ~~~shell
- docker build -t harbor.abc.com/apache/dolphinscheduler:1.3.9-MySQL-Oracle-DataX . #不要忘记最后一个点
- docker push harbor.abc.com/apache/dolphinscheduler:1.3.9-MySQL-Oracle-DataX
- ~~~
-
- 修改value文件
-
- ~~~yaml
- image:
- repository: "harbor.abc.com/apache/dolphinscheduler"
- tag: "1.3.9-MySQL-Oracle-DataX"
- pullPolicy: "Always"
- ~~~
-
- 执行helm install dolphinscheduler . -n ds139,或者执行helm upgrade dolphinscheduler -n ds139,也可以先helm uninstall dolphinscheduler -n ds139,再执行helm install dolphinscheduler . -n ds139。
-
-+ 通常生产环境建议使用独立外置postgresql作为管理数据库,并且使用独立安装的zookeeper环境(本案例使用了zookeeper operator https://GitHub.com/pravega/zookeeper-operator ,与Apache DolphinScheduler在同一个Kubernetes集群中)。
-
- ~~~yaml
- ## If not exists external database, by default, Dolphinscheduler's database will use it.
- postgresql:
- enabled: false
- postgresqlUsername: "root"
- postgresqlPassword: "root"
- postgresqlDatabase: "dolphinscheduler"
- persistence:
- enabled: false
- size: "20Gi"
- storageClass: "-"
-
- ## If exists external database, and set postgresql.enable value to false.
- ## external database will be used, otherwise Dolphinscheduler's database will be used.
- externalDatabase:
- type: "postgresql"
- driver: "org.postgresql.Driver"
- host: "192.168.1.100"
- port: "5432"
- username: "admin"
- password: "password"
- database: "dolphinscheduler"
- params: "characterEncoding=utf8"
-
- ## If not exists external zookeeper, by default, Dolphinscheduler's zookeeper will use it.
- zookeeper:
- enabled: false
- fourlwCommandsWhitelist: "srvr,ruok,wchs,cons"
- persistence:
- enabled: false
- size: "20Gi"
- storageClass: "storage-nfs"
- zookeeperRoot: "/dolphinscheduler"
-
- ## If exists external zookeeper, and set zookeeper.enable value to false.
- ## If zookeeper.enable is false, Dolphinscheduler's zookeeper will use it.
- externalZookeeper:
- zookeeperQuorum: "zookeeper-0.zookeeper-headless.zookeeper.svc.cluster.local:2181,zookeeper-1.zookeeper-headless.zookeeper.svc.cluster.local:2181,zookeeper-2.zookeeper-headless.zookeeper.svc.cluster.local:2181"
- zookeeperRoot: "/dolphinscheduler"
- ~~~
-
-
-
-## 基于argo-cd的Gitops部署方式
-
-argo-cd是基于Kubernetes 的声明式Gitops持续交付工具。argo-cd是CNCF的孵化项目,Gitops的最佳实践工具。关于Gitops的解释可以参考https://about.gitlab.com/topics/gitops/
-
-<div align=center>
-<img src="/img/2022-02-22/3.png"/>
-</div>
-
-Gitops可以为Apache DolphinScheduler的实施带来以下优点。
-
-+ 图形化安装集群化的软件,一键安装;
-+ Git记录全发版流程,一键回滚;
-+ 便捷的海豚工具日志查看;
-
-使用argo-cd的实施安装步骤:
-
-+ 从GitHub上下载Apache DolphinScheduler源码,修改value文件,参考上个章节helm安装需要修改的内容;
-
-+ 把修改后的源码目录新建Git项目,并且push到公司内部的Gitlab中,GitHub源码的目录名为docker/Kubernetes/dolphinscheduler;
-
-+ 在argo-cd中配置Gitlab信息,我们使用https的模式;
-
-<div align=center>
-<img src="/img/2022-02-22/4.png"/>
-</div>
-
-+ argo-cd新建部署工程,填写相关信息
-
-<div align=center>
-<img src="/img/2022-02-22/5.png"/>
-</div>
-
-<div align=center>
-<img src="/img/2022-02-22/6.png"/>
-</div>
-
-+ 对Git中的部署信息进行刷新和拉取,实现最后的部署工作;可以看到pod,configmap,secret,service等等资源全自动拉起。
-
-<div align=center>
-<img src="/img/2022-02-22/7.png"/>
-</div>
-
-<div align=center>
-<img src="/img/2022-02-22/8.png"/>
-</div>
-
-+ 通过kubectl命令可以看到相关资源信息;
-
- ~~~shell
- [root@tpk8s-master01 ~]# kubectl get po -n ds139
- NAME READY STATUS RESTARTS AGE
- dolphinscheduler-alert-96c74dc84-72cc9 1/1 Running 0 22m
- dolphinscheduler-api-78db664b7b-gsltq 1/1 Running 0 22m
- dolphinscheduler-master-0 1/1 Running 0 22m
- dolphinscheduler-master-1 1/1 Running 0 22m
- dolphinscheduler-master-2 1/1 Running 0 22m
- dolphinscheduler-worker-0 1/1 Running 0 22m
- dolphinscheduler-worker-1 1/1 Running 0 22m
- dolphinscheduler-worker-2 1/1 Running 0 22m
-
- [root@tpk8s-master01 ~]# kubectl get statefulset -n ds139
- NAME READY AGE
- dolphinscheduler-master 3/3 22m
- dolphinscheduler-worker 3/3 22m
-
- [root@tpk8s-master01 ~]# kubectl get cm -n ds139
- NAME DATA AGE
- dolphinscheduler-alert 15 23m
- dolphinscheduler-api 1 23m
- dolphinscheduler-common 29 23m
- dolphinscheduler-master 10 23m
- dolphinscheduler-worker 7 23m
-
- [root@tpk8s-master01 ~]# kubectl get service -n ds139
- NAME TYPE CLUSTER-IP EXTERNAL-IP PORT(S) AGE
- dolphinscheduler-api ClusterIP 10.43.238.5 <none> 12345/TCP 23m
- dolphinscheduler-master-headless ClusterIP None <none> 5678/TCP 23m
- dolphinscheduler-worker-headless ClusterIP None <none> 1234/TCP,50051/TCP 23m
-
- [root@tpk8s-master01 ~]# kubectl get ingress -n ds139
- NAME CLASS HOSTS ADDRESS
- dolphinscheduler <none> ds139.abc.com
-
- ~~~
-
-
-
-+ 可以看到所有的pod都分撒在Kubernetes集群中不同的host上,例如worker1和2都在不同的节点上。
-
-<div align=center>
-<img src="/img/2022-02-22/9.png"/>
-</div>
-
-<div align=center>
-<img src="/img/2022-02-22/10.png"/>
-</div>
-
-+ 我们配置了ingress,公司内部配置了泛域名就可以方便的使用域名进行访问;
-
-<div align=center>
-<img src="/img/2022-02-22/11.png"/>
-</div>
-
- 可以登录域名进行访问。
-
-<div align=center>
-<img src="/img/2022-02-22/12.png"/>
-</div>
-
- 具体配置可以修改value文件中的内容:
-
- ~~~yaml
- ingress:
- enabled: true
- host: "ds139.abc.com"
- path: "/dolphinscheduler"
- tls:
- enabled: false
- secretName: "dolphinscheduler-tls"
- ~~~
-
-- 方便查看Apache DolphinScheduler各个组件的内部日志:
-
-<div align=center>
-<img src="/img/2022-02-22/13.png"/>
-</div>
-
-+ 对部署好的系统进行检查,3个master,3个worker,zookeeper都配置正常;
-
-<div align=center>
-<img src="/img/2022-02-22/14.png"/>
-</div>
-
-<div align=center>
-<img src="/img/2022-02-22/15.png"/>
-</div>
-
-<div align=center>
-<img src="/img/2022-02-22/16.png"/>
-</div>
-
-+ 使用argo-cd可以非常方便的进行修改master,worker,api,alert等组件的副本数量,海豚的helm配置也预留了cpu和内存的设置信息。这里我们修改value中的副本值。修改后,提交公司内部Gitlab。
-
- ~~~~yaml
- master:
- ## PodManagementPolicy controls how pods are created during initial scale up, when replacing pods on nodes, or when scaling down.
- podManagementPolicy: "Parallel"
- ## Replicas is the desired number of replicas of the given Template.
- replicas: "5"
-
- worker:
- ## PodManagementPolicy controls how pods are created during initial scale up, when replacing pods on nodes, or when scaling down.
- podManagementPolicy: "Parallel"
- ## Replicas is the desired number of replicas of the given Template.
- replicas: "5"
-
-
- alert:
- ## Number of desired pods. This is a pointer to distinguish between explicit zero and not specified. Defaults to 1.
- replicas: "3"
-
- api:
- ## Number of desired pods. This is a pointer to distinguish between explicit zero and not specified. Defaults to 1.
- replicas: "3"
- ~~~~
-
-- 只需要在argo-cd点击sync同步,对应的pods都按照需求进行了增加
-
-<div align=center>
-<img src="/img/2022-02-22/17.png"/>
-</div>
-
-<div align=center>
-<img src="/img/2022-02-22/18.png"/>
-</div>
-
- ~~~
- [root@tpk8s-master01 ~]# kubectl get po -n ds139
- NAME READY STATUS RESTARTS AGE
- dolphinscheduler-alert-96c74dc84-72cc9 1/1 Running 0 43m
- dolphinscheduler-alert-96c74dc84-j6zdh 1/1 Running 0 2m27s
- dolphinscheduler-alert-96c74dc84-rn9wb 1/1 Running 0 2m27s
- dolphinscheduler-api-78db664b7b-6j8rj 1/1 Running 0 2m27s
- dolphinscheduler-api-78db664b7b-bsdgv 1/1 Running 0 2m27s
- dolphinscheduler-api-78db664b7b-gsltq 1/1 Running 0 43m
- dolphinscheduler-master-0 1/1 Running 0 43m
- dolphinscheduler-master-1 1/1 Running 0 43m
- dolphinscheduler-master-2 1/1 Running 0 43m
- dolphinscheduler-master-3 1/1 Running 0 2m27s
- dolphinscheduler-master-4 1/1 Running 0 2m27s
- dolphinscheduler-worker-0 1/1 Running 0 43m
- dolphinscheduler-worker-1 1/1 Running 0 43m
- dolphinscheduler-worker-2 1/1 Running 0 43m
- dolphinscheduler-worker-3 1/1 Running 0 2m27s
- dolphinscheduler-worker-4 1/1 Running 0 2m27s
- ~~~
-
-
-
-## Apache DolphinScheduler与s3对象存储技术集成
-
-许多同学在海豚的社区中提问,如何配置s3 minio的集成。这里给出基于Kubernetes的helm配置。
-
-+ 修改value中s3的部分,建议使用ip+端口指向minio服务器。
-
- ~~~yaml
- common:
- ## Configmap
- configmap:
- DOLPHINSCHEDULER_OPTS: ""
- DATA_BASEDIR_PATH: "/tmp/dolphinscheduler"
- RESOURCE_STORAGE_TYPE: "S3"
- RESOURCE_UPLOAD_PATH: "/dolphinscheduler"
- FS_DEFAULT_FS: "s3a://dfs"
- FS_S3A_ENDPOINT: "http://192.168.1.100:9000"
- FS_S3A_ACCESS_KEY: "admin"
- FS_S3A_SECRET_KEY: "password"
- ~~~
-
-+ minio中存放海豚文件的bucket名字是dolphinscheduler,这里新建文件夹和文件进行测试。
-
-<div align=center>
-<img src="/img/2022-02-22/19.png"/>
-</div>
-
-<div align=center>
-<img src="/img/2022-02-22/19-1.png"/>
-</div>
-
-
-
-## Apache DolphinScheduler与 Kube-prometheus 的技术集成
-
-+ 我们在Kubernetes使用kube-prometheus operator技术,实现了在部署海豚后,自动实现了对海豚各个组件的资源监控。
-
-+ 请注意kube-prometheus的版本,需要对应Kubernetes主版本。https://GitHub.com/prometheus-operator/kube-prometheus
-
-<div align=center>
-<img src="/img/2022-02-22/20.png"/>
-</div>
-
-<div align=center>
-<img src="/img/2022-02-22/21.png"/>
-</div>
-
-<div align=center>
-<img src="/img/2022-02-22/18.png"/>
-</div>
-
-<div align=center>
-<img src="/img/2022-02-22/22.png"/>
-</div>
-
-
-## Apache DolphinScheduler与 Service Mesh 的技术集成
-
-+ 通过 Service Mesh 技术可以实现对海豚内部的服务调用,以及海豚api外部调用的可观测性分析,以实现Apache DolphinScheduler产品的自身服务优化。
-
-+ 我们使用linkerd作为Service Mesh的产品进行集成,linkerd也是CNCF优秀的毕业项目。
-
- <div align=center>
-<img src="/img/2022-02-22/23.png"/>
-</div>
-
-
-
-+ 只需要在海豚helm的value文件中修改annotations,重新部署,就可以快速实现mesh proxy sidecar的注入。可以对master,worker,api,alert等组件都注入。
-
- ~~~yaml
- annotations: #{}
- linkerd.io/inject: enabled
- ~~~
-
-可以观察组件之间的服务通信质量,每秒请求的次数等等。
-
-<div align=center>
-<img src="/img/2022-02-22/24.png"/>
-</div>
-
-<div align=center>
-<img src="/img/2022-02-22/25.png"/>
-</div>
-
-
-## 未来Apache DolphinScheduler基于云原生技术的展望
-
-Apache DolphinScheduler作为面向新一代云原生大数据工具,未来可以在Kubernetes生态集成更多的优秀工具和特性,满足更多的用户群体和场景。
-
-+ 和argo-workflow的集成,可以通过api,cli等方式在Apache DolphinScheduler中调用argo-workflow单个作业,dag作业,以及周期性作业;
-
-+ 使用hpa的方式,自动扩缩容worker,实现无人干预的水平扩展方式;
-
-+ 集成Kubernetes的spark operator和Hadoopoperator工具,全面的云原生化;
-
-+ 实现多云和多集群的分布式作业调度;
-
-+ 采用sidecar实现定期删除worker作业日志;
-
-<div align=center>
-<img src="/img/2022-02-22/26.png"/>
-</div>
-
-
-
diff --git "a/blog/zh-cn/DolphinScheduler\346\274\217\346\264\236\346\203\205\345\206\265\350\257\264\346\230\216.md" "b/blog/zh-cn/DolphinScheduler\346\274\217\346\264\236\346\203\205\345\206\265\350\257\264\346\230\216.md"
deleted file mode 100644
index de6504e..0000000
--- "a/blog/zh-cn/DolphinScheduler\346\274\217\346\264\236\346\203\205\345\206\265\350\257\264\346\230\216.md"
+++ /dev/null
@@ -1,43 +0,0 @@
-【安全通报】【影响程度:低】DolphinScheduler 漏洞情况说明
-
-Apache DolphinScheduler 社区邮件列表最近通告了 1个漏洞,考虑到有很多用户并未订阅此邮 件列表,我们特地在此进行情况说明:
-
-CVE-2021-27644
-
-重要程度: 低
-
-影响范围: 暴露服务在外网中、且内部账号泄露。如果无上述情况,用户可根据实际情况决定是否需要升级。
-
-影响版本: <1.3.6
-
-漏洞说明:
-
-此问题是由于mysql connectorj 漏洞引起的,DolphinScheduler登陆用户(未登录用户无法执行此操作,建议企业做好账号安全规范)可在数据源管理页面-Mysql数据源填写恶意参数,导致安全隐患。(未使用Mysql数据源的不影响)
-
-修复建议: 升级到>=1.3.6版本
-
-特别感谢
-
-特别感谢漏洞报告者:来自蚂蚁安全非攻实验室的锦辰同学,他提供了漏洞的还原过程以及对应的解决方案。整个过程呈现了专业安全人员的技能和高素质,感谢他们为开源项目的安全守护所作出的贡献。
-
-建议
-
-十分感谢广大用户选择 Apache DolphinScheduler 作为企业的大数据任务调度系统,但必须要提醒的是调度系统属于大数据建设中核心基础设施,请不要将其暴露在外网中。此外应该对企业内部人员账号做好安全措施,降低账号泄露的风险。
-
-贡献
-
-迄今为止,Apache DolphinScheduler 社区已经有近200+ 位代码贡献者,70+位非代码贡献者。其中也不乏其他Apache顶级项目的PMC或者Committer,非常欢迎更多伙伴也能参与到开源社区建设中来,为建造一个更加稳定安全可靠的大数据任务调度系统而努力,同时也为中国开源崛起献上自己的一份力量!
-
-WebSite :https://dolphinscheduler.apache.org/
-
-MailList :dev@dolphinscheduler@apache.org
-
-Twitter :@DolphinSchedule
-
-YouTube :https://www.youtube.com/channel/UCmrPmeE7dVqo8DYhSLHa0vA
-
-Slack :https://s.apache.org/dolphinscheduler-slack
-
-Contributor Guide:https://dolphinscheduler.apache.org/en-us/community/index.html
-
-如果对漏洞有任何疑问,欢迎参与讨论,竭诚解决大家的疑虑:
\ No newline at end of file
diff --git a/blog/zh-cn/Eavy_Info.md b/blog/zh-cn/Eavy_Info.md
deleted file mode 100644
index fdd645b..0000000
--- a/blog/zh-cn/Eavy_Info.md
+++ /dev/null
@@ -1,109 +0,0 @@
-# 亿云基于 DolphinScheduler 构建资产数据管理平台服务,助力政务信息化生态建设 | 最佳实践
-<div align=center>
-<img src="https://s1.imgpp.com/2021/12/30/1639640547411.png"/>
-</div>
-
-作者| 孙浩
-
-> 编 者 按:基于 Apache Dolphinscheduler 调度平台,云计算和大数据提供商亿云信息已经服务公司多个项目部的地市现场平稳运行一年之久。
-
-> 结合政务信息化生态建设业务,亿云信息基于 DolphinScheduler 构建了资产数据管控平台的数据服务模块。他们是如何进行探索和优化的?亿云信息研发工程师 孙浩 进行了详细的用户实践交流分享。
-
-## 01 研发背景
-
-亿云主要的业务主要是 ToG 的业务,而业务前置的主要工作,在于数据的采集和共享,传统 ETL 工具,例如 kettle 等工具对于一线的实施人员的来说上手难度还是有的,再就是类似 kettle 的工具本身做为独立的部分,本身就增加了现场项目运维的使用难度。因此,如何实现一套数据采集(同步)—数据处理—数据管理的平台,就显得尤为重要。
-出于这样的考虑,我们开发了数据资产管理平台,而管理平台的核心就是我们基于DolphinSchduler(简称 DS)实现的数据服务模块。
-DolphinScheduler 是一个分布式去中心化,易扩展的可视化 DAG 调度系统,支持包括 Shell、Python、Spark、Flink 等多种类型的 Task 任务,并具有很好的扩展性。其整体架构如下图所示:
-
-<div align=center>
-<img src="https://s1.imgpp.com/2021/12/28/1.png"/>
-</div>
-
-典型的 master-slave 架构,横向扩展能力强,调度引擎是 Quartz,本身作为 Spring Boot 的 java 开源项目,对于熟悉 Spring Boot 开发的人,集成使用更加的简单上手。
-
-DS 作为调度系统支持以下功能:
-
-调度方式:系统支持基于 cron 表达式的定时调度和手动调度。命令类型支持:启动工作流、从当前节点开始执行、恢复被容错的工作流、恢复暂停流程、从失败节点开始执行、补数、定时、重跑、暂停、停止、恢复等待线程。其中恢复被容错的工作流和恢复等待线程两种命令类型是由调度内部控制使用,外部无法调用。
-
-定时调度:系统采用 quartz 分布式调度器,并同时支持 cron 表达式可视化的生成。
-
-依赖:系统不单单支持 DAG 简单的前驱和后继节点之间的依赖,同时还提供任务依赖节点,支持流程间的自定义任务依赖。
-
-优先级 :支持流程实例和任务实例的优先级,如果流程实例和任务实例的优先级不设置,则默认是先进先出。
-
-邮件告警:支持 SQL任务 查询结果邮件发送,流程实例运行结果邮件告警及容错告警通知。
-
-失败策略:对于并行运行的任务,如果有任务失败,提供两种失败策略处理方式,继续是指不管并行运行任务的状态,直到流程失败结束。结束是指一旦发现失败任务,则同时Kill掉正在运行的并行任务,流程失败结束。
-
-补数:补历史数据,支持区间并行和串行两种补数方式。
-
-我们基于 Dolphinscheduler 与小伙伴一起进行如下的实践。
-
-## 02 基于DS构建数据同步工具
-
-回归业务本身,我们的业务场景,数据同步的业务需要是类型多,但数据量基本不会特别大,对实时要求并不高。所以在架构选型之初,我们就选择了 datax+ds 的组合,并进行对应业务的改造实现,现在作为服务产品融合在各个项目中,提供离线同步服务。
-
-
-
-<div align=center>
-<img src="https://s1.imgpp.com/2021/12/30/1.png"/>
-</div>
-
-
-同步任务分为了周期任务和一次性任务,在配置完成输入输出源的配置任务之后,周期任务的话,需要配置 corn 表达式,然后调用保存接口,将同步任务发送给DS 的调度平台。
-
-<div align=center>
-<img src="https://s1.imgpp.com/2021/12/30/2.png"/>
-</div>
-我们这里综合考虑放弃了之前 DS 的 UI 前端(第二部分在自助开发模块会给大家解释),复用 DS 后端的上线、启停、删除、日志查看等接口。
-
-<div align=center>
-
-<img src="https://s1.imgpp.com/2021/12/30/4.png"/>
-</div>
-
-<div align=center>
-<img src="https://s1.imgpp.com/2021/12/30/5.png"/>
-</div>
-
-整个同步模块的设计思路,就是重复利用 datax 组件的输入输出 plugin 多样性,配合 DS 的优化,来实现一个离线的同步任务,这个是当前我们的同步的一个组件图,实时同步这块不再赘述。
-
-<div align=center>
-<img src="https://s1.imgpp.com/2021/12/30/9.png"/>
-</div>
-
-## 03 基于DS的自助开发实践
-
-熟悉 datax的人都知道它本质上是一个 ETL 工具,而其 Transform 的属性体现在,它提供了一个支持 grovy 语法的 transformer 模块,同时可以在 datax 源码中进一步丰富 transformer 中用到工具类,例如替换、正则匹配、筛选、脱敏、统计等功能。而 Dolphinscheduler 的任务,是可以用 DAG 图来实现,那么我们想到,是否存在一种可能,针对一张表或者几张表,把每个 datax 或者 SQL 抽象成一个数据治理的小模块,每个模块按照 DAG 图去设计,并且在上下游之间可以实现数据的传递,最好还是和 DS 一样的可以拖拽式的实现。于是,我们基于前期对 datax 与 ds 的使用,实现了一个自助开发的模块。
-
-<div align=center>
-<img src="https://s1.imgpp.com/2021/12/30/6.png"/>
-</div>
-
-每个组件可能是一个模块,每个模块功能之间的依赖关系,我们利用 ds 的depend 来处理,而对应组件与组件传递数据,我们利用前端去存储,也就是我们在引入 input(输入组件)之后,让前端来进行大部分组件间的传递和逻辑判断,因为每个组件都可以看作一个 datax 的(输出/输出),所有参数在输入时,最终输出的全集基本就确定了,这也是我们放弃 DS 的 UI 前端的原因。之后,我们将这个 DAG 图组装成 DS 的定义的类型,同样交付给 ds 任务中心。
-
-PS:因为我们的业务场景可能存在跨数据库查询的情况(不同实例的 mysql 组合查询),我们的 SQL 组件底层使用 Presto 来实现一个统一 SQL 层,这样即使是不同 IP 实例下的数据源(业务上有关联意义),也可以通过 Presto 来支持组合检索。
-
-## 04 其他的一些简单尝试
-熟悉治理流程的人都知道,如果能够做到简单的治理流程化,那么必然可以产出一份质量报告。我们在自助开发的基础上进行优化,将部分治理的记录写入 ES 中,再利用 ES 的聚合能力来实现了一个质量报告。
-
-<div align=center>
-<img src="https://s1.imgpp.com/2021/12/30/7.png"/>
-</div>
-
-<div align=center>
-<img src="https://s1.imgpp.com/2021/12/30/8.png"/>
-</div>
-
-<div align=center>
-<img src="https://s1.imgpp.com/2021/12/30/10.png"/>
-</div>
-
-以上便是我们使用 DS 结合 datax 等中间件,并结合业务背景所做的一些符合自身需求的实践。
-
-## 05 感谢
-
-从 EasyScheduler 到现在的 DolphinScheduler 2.0,我们更多时候还是作为旁观者,或者是追随者,而这次更多地是从这一年来使用 Dolphinscheduler 构建我们数据资产管控平台数据服务模块的实践来进行交流分享。当前基于Dolphinscheduler 调度平台,我们已经服务了公司多个项目部的地市现场运行。随着 DolphinScheduler 2.0 发版,我们也和 DolphinScheduler 一起在不断进步的社区环境中共同成长。
-
-## 06 欢迎更多实践分享
-如果你也是 Apache DolphinScheduler 的用户或实践者,欢迎投稿或联系社区分享你们的实践经验,开源共享,互帮互助!
diff --git a/blog/zh-cn/Exploration_and_practice_of_Tujia_Big_Data_Platform_Based.md b/blog/zh-cn/Exploration_and_practice_of_Tujia_Big_Data_Platform_Based.md
deleted file mode 100644
index ba45f1f..0000000
--- a/blog/zh-cn/Exploration_and_practice_of_Tujia_Big_Data_Platform_Based.md
+++ /dev/null
@@ -1,145 +0,0 @@
-# 途家大数据平台基于 Apache DolphinScheduler 的探索与实践
-
-<div align=center>
-<img src="/img/2022-3-9/1.jpeg"/>
-</div>
-
->途家在 2019 年引入 Apache DolphinScheduler,在不久前的 Apache DolphinScheduler 2 月份的 Meetup上,**途家大数据工程师 昝绪超** 详细介绍了途家接入 Apache DolphinScheduler 的历程,以及进行的功能改进。
-<div align=center>
-<img style="width: 25%;" src="/img/2022-3-9/2.png"/>
-</div>
-
-途家大数据工程师数据开发工程师,主要负责大数据平台的开发,维护和调优。
-
-本次演讲主要包括4个部分,第一部分是途家的平台的现状,介绍途家的数据的流转过程,如何提供数据服务,以及Apache DolphinScheduler在平台中扮演的角色。第二部分,调度选型,主要介绍调度的一些特性,以及接入的过程。第三部分主要介绍我们对系统的的一些改进和功能扩展,包括功能表依赖的支持,邮件任务扩展,以及数据同步的功能,第四部分是根据业务需求新增的一些功能,如Spark jar包支持发布系统,调度与数据质量打通,以及表血缘展示。
-
-## 途家数据平台现状
-### 01 数据架构
-
-
-首先介绍一下途家数据平台的架构以及Apache DolphinScheduler在数据平台扮演的角色。
-
-<div align=center>
-<img src="/img/2022-3-9/3.png"/>
-</div>
-
-途家数据平台架构
-
-
-上图为我司数据平台的架构,主要包括**数据源,数据采集,数据存储,数据管理,最后提供服务**。
-
-**数据源**主要来源包括三个部分:业务库MySQL API数据同步,涉及到Dubbo接口、http 接口,以及web页面的埋点数据。
-
-**数据采集**采用实时和离线同步,业务数据是基于Canal的增量同步,日志是Flume,Kafka实时收集,落到HDFS上。
-
-**数据存储**过程,主要涉及到一些数据同步服务,数据落到HDFS 后经过清洗加工,推送到线上提供服务。
-
-**数据管理**层面,数据字典记录业务的元数据信息,模型的定义,各层级之间的映射关系,方便用户找到自己关心的数据;日志记录任务的运行日志,告警配置故障信息等。调度系统,作为大数据的一个指挥中枢,合理分配调度资源,可以更好地服务于业务。指标库记录了维度和属性,业务过程指标的规范定义,用于更好的管理和使用数据。Abtest记录不同指标和策略对产品功能的影响;数据质量是数据分析有效性和准确性的基础。
-
-最后是**数据服务**部分,主要包括数据的即席查询,报表预览,数据下载上传分析,线上业务数据支持发布等。
-
-### 02 Apache DolphinScheduler在平台的作用
-
-
-下面着重介绍调度系统在平台扮演的角色。数据隧道同步,每天凌晨定时拉去增量数据。数据清洗加工后推送到线上提供服务。数据的模型的加工,界面化的配置大大提高了开发的效率。定时报表的服务,推送邮件,支持附件,正文table 以及折线图的展示。报表推送功能,数据加工后,分析师会配置一些数据看板,每天DataX把计算好的数据推送到MySQL,做报表展示。
-
-## 接入DS
-
-第二部分介绍我们接入Apache DolphinScheduler做的一些工作。
-
-
-Apache DolphinScheduler具有很多优势,作为大数据的一个指挥中枢,系统的可靠性毋庸置疑,Apache DolphinScheduler 去中心化的设计避免了单点故障问题,以及节点出现问题,任务会自动在其他节点重启,大大提高了系统的可靠性。
-
-
-此外,调度系统简单实用,减少了学习成本,提高工作效率,现在公司很多人都在用我们的调度系统,包括分析师、产品运营,开发。
-
-调度的扩展性也很重要,随着任务量的增加,集群能及时增添资源,提供服务。应用广泛也是我们选择它的一个重要原因,它支持丰富的任务类型:Shell、MR、Spark、SQL(MySQL、PostgreSQL、Hive、SparkSQL),Python,Sub_Process,Procedure等,支持工作流定时调度、依赖调度、手动调度、手动暂停/停止/恢复,同时支持失败重试/告警、从指定节点恢复失败、Kill任务等操作等。它的优势很多,一时说不完,大家都用起来才知道。
-
-
-接下就是我们定时调度的升级。
-
-在采用 Apache DolphinScheduler之前,我们的调度比较混乱,有自己部署本地的Crontab,也有人用Oozie做调度,还有部分是在系统做定时调度。管理起来比较混乱,没有统一的管理调度平台,时效性,和准确性得不到保障,管理任务比较麻烦,找不到任务的情况时有发生。此外,自建调度稳定性不足,没有配置依赖,数据产出没有保障,而且产品功能单一,支持的任务调度有限。
-
-<div align=center>
-<img src="/img/2022-3-9/4.png"/>
-</div>
-2019年,我们引入Apache DolphinScheduler ,到现在已经接近三年时间,使用起来非常顺手。
-
-下面是我们迁移系统的一些数据。
-
-
-我们搭建了DS集群 ,共4台实体机 ,目前单机并发支持100个任务调度。
-
-
-算法也有专门的机器,并做了资源隔离。
-
-Oozie 任务居多 ,主要是一些Spark和Hive 任务 。还有Crontab 上的一些脚本,一些邮件任务以及报表系统的定时任务。
-
-## 基于DS的调度系统构建
-
-在此之前,我们也对系统做过优化,如支持表级别的依赖,邮件功能的扩展等。接入Apache DolphinScheduler后,我们在其基础之上进行了调度系统构建,以能更好地提供服务。
-
-
-**第一. 支持表依赖的同步,**当时考虑到任务迁移,会存在并行的情况,任务一时没法全部同步,需要表任务运行成功的标记,于是我们开发了一版功能,解决了任务迁移中的依赖问题。然而,每个人的命名风格不太一样,导致配置依赖的时候很难定位到表的任务,我们也无法识别任务里面包含哪些表,无法判断表所在的任务,这给我们使用造成不小的麻烦。
-
-<div align=center>
-<img src="/img/2022-3-9/5.png"/>
-</div>
-<div align=center>
-<img src="/img/2022-3-9/6.png"/>
-</div>
-
-**第二.邮件任务支持多table。** 调度里面自带邮件推送功能,但仅支持单个table ,随着业务要求越来越多,我们需要配置多个 table和多个sheet,要求正文和附件的展示的的条数不一样,需要配置,另外还需要支持折线图的功能,丰富正文页面。此外,用户还希望能在正文或者每个表格下面加注释,进行指标的说明等。我们使用Spark jar包实现了邮件推送功能,支持异常预警、表依赖缺失等。
-
-<div align=center>
-< img src="/img/2022-3-9/7.png"/>
-</div>
-<div align=center>
-< img src="/img/2022-3-9/8.png"/>
-</div>
-
-**第三.支持丰富的数据源同步。**由于在数据传输方面存在一些问题,在以前迁移的过程中,我们需要修改大量的配置代码,编译打包上传,过程繁琐,经常出现漏改,错该,导致线上故障,数据源不统一,测试数据和线上数据无法分开;在开发效率方面,代码有大量重复的地方,缺少统一的配置工具,参数配置不合理,导致MySQL压力大,存在宕机的风险;数据传输后,没有重复值校验,数据量较大的时候,全量更新,导致MySQL压力比较大。MySQL 传输存在单点故障问题,任务延迟影响线上服务。
-
-
-<div align=center>
-<img src="/img/2022-3-9/9.png"/>
-</div>
-<div align=center>
-<img src="/img/2022-3-9/10.png"/>
-</div>
-我们在此过程中简化了数据开发的流程,使得MySQL支持 pxc/mha的高可用,提升了数据同步的效率。
-
-我们支持输入的数据源支持关系型数据库,支持FTP同步,Spark作为计算引擎,输出的数据源支持各种关系型数据库,以及消息中间件Kafka、MQ和Redis。
-
-接下来讲一下我们实现的过程。
-
-我们对Apache DolphinScheduler 的数据源做了扩展,支持kafka mq和namespace 的扩展,MySQL 同步之前首先在本地计算一个增量,把增量数据同步到MySQL,Spark 也支持了MySQL pxc/qmha 的高可用。另外,在推送MQ和Redis时会有qps 的限制,我们根据数据量控制Spark的分区数和并发量。
-
-## 改进
-
-第四部分主要是对系统新增的一些功能,来完善系统。主要包含以下三点:
-
-1. Spark支持发布系统
-2. 数据质量打通
-3. 数据血缘的展示
-### **01**Spark 任务支持发布系统
-
-由于我们平时的调度80%以上都是Spark jar 包任务,但任务的发布流程缺少规范,代码修改随意,没有完整的流程规范,各自维护一套代码。这就导致代码不一致的情况时有发生,严重时还会造成线上问题。
-
-这要求我们完善任务的发布流程。我们主要使用发布系统,Jenkens 打包功能,编译打包后生成btag,在测试完成后再发布生成rtag ,代码合并到master 。这就避免了代码不一致的问题,也减少了jar包上传的步骤。在编译生成jar 包后,系统会自动把jar包推送到Apache DolphinScheduler的资源中心,用户只需配置参数,选择jar包做测试发布即可。在运行Spark任务时,不再需要把文件拉到本地,而是直接读取HDFS上的jar包。
-
-### 02 数据质量打通
-
-数据质量是保障分析结论的有效性和准确性的基础。我们需要要完整的数据监控产出流程才能让数据更有说服力。质量平台从四个方面来保证数据准确性,完整性一致性和及时性,并支持电话、企业微信和邮件等多种报警方式来告知用户。
-
-接下来将介绍如何将数据质量和调度系统打通。调度任务运行完成后,发送消息记录,数据质量平台消费消息,触发数据质量的规则监控 根据监控规则来阻断下游运行或者是发送告警消息等。
-
-### 03 数据血缘关系展示
-
-数据血缘是元数据管理、数据治理、数据质量的重要一环,其可以追踪数据的来源、处理、出处,为数据价值评估提供依据,描述源数据流程、表、报表、即席查询之间的流向关系,表与表的依赖关系、表与离线ETL任务,调度平台、计算引擎之间的依赖关系。数据仓库是构建在Hive之上,而Hive的原始数据往往来自生产DB,也会把计算结果导出到外部存储,异构数据源的表之间是有血缘关系的。
-
-
-* **追踪数据溯源**:当数据发生异常,帮助追踪到异常发生的原因;影响面分析,追踪数据的来源,追踪数据处理过程。
-* **评估数据价值**:从数据受众、更新量级、更新频次等几个方面给数据价值的评估提供依据。
-* **生命周期**:直观地得到数据整个生命周期,为数据治理提供依据。
-血缘的收集过程主要是 :Spark 通过监控Spark API来监听SQL和插入的表,获取Spark的执行计划 ,并解析Spark 执行计划。
diff --git a/blog/zh-cn/Hangzhou_cisco.md b/blog/zh-cn/Hangzhou_cisco.md
deleted file mode 100644
index cab6fba..0000000
--- a/blog/zh-cn/Hangzhou_cisco.md
+++ /dev/null
@@ -1,140 +0,0 @@
-# 杭州思科对 Apache DolphinScheduler Alert 模块的改造
-
-<div align=center>
-<img src="/img/3-16/1.png"/>
-</div>
-
-杭州思科已经将 Apache DolphinScheduler 引入公司自建的大数据平台。目前,**杭州思科大数据工程师 李庆旺** 负责 Alert 模块的改造已基本完成,以更完善的 Alert 模块适应实际业务中对复杂告警的需求。
-<div align=center>
-
-<img src="/img/3-16/2.png"/>
-
-</div>
-
-李庆旺
-
-杭州思科 大数据工程师,主要负责 Spark、调度系统等大数据方向开发。
-
-我们在使用原有的调度平台处理大数据任务时,在操作上多有不便。比如一个对数据进行处理聚合分析的任务,首先由多个前置 Spark 任务对不同数据源数据进行处理、分析。最后的 Spark 任务对这期间处理的结果进行再次聚合、分析,得到我们想要的最终数据。但遗憾的是当时的调度平台无法串行执行多个任务,需要估算任务处理时间来设置多个任务的开始执行时间。同时其中一个任务执行失败,需要手动停止后续任务。这种方式既不方便,也不优雅。
-
-而 Apache DolphinScheduler 的核心功能——**工作流定义可以将任务串联起来**,完美契合我们的需求。于是,我们将 Apache DolphinScheduler 引入自己的大数据平台,而我主要负责 Alert 模块改造。目前我们其他同事也在推进集成 K8s,希望未来任务在 K8s 中执行。
-
-今天分享的是 Alert 模块的改造。
-
-## 01 **Alert 模块的设计**
-
-<div align=center>
-
-<img src="/img/3-16/3.png"/>
-
-</div>
-
-DolphinScheduler Alert 模块的设计
-
-Apache DolphinScheduler 1.0 版本的 Alert 模式使用配置alert.properties的方式,通过配置邮箱、短信等实现告警,但这样的方式已经不适用于当前的场景了。官方也进行过告警模块重构,详情设计思路参考官方文档:
-
-[https://github.com/apache/dolphinscheduler/issues/3049](https://github.com/apache/dolphinscheduler/issues/3049)
-
-[https://dolphinscheduler.apache.org/zh-cn/development/backend/spi/alert.html](https://dolphinscheduler.apache.org/zh-cn/development/backend/spi/alert.html)
-
-
-Apache DolphinScheduler 告警模块是一个独立启动的服务,核心之一是 AlertPluginManager 类。告警模块集成了很多插件,如钉钉、微信、飞书、邮件等,以独立的形式写在源码中,启动服务时会解析插件并将配置的参数格式化成JSON形式,前端通过JSON自动渲染出页面。AlertPluginManager 在启动时会缓存插件到内存中。AlertServer类会启动线程池,定时扫描DB。
-
-当工作流配置了通知策略,同时Worker 执行工作流结束,执行结果匹配通知策略成功后,DB插入告警数据后,线程池扫描 DB,调用AlertSender 类的send方法传入告警数据。告警数据绑定的是告警组,一个告警组对应了多个告警实例。AlertSender类遍历告警实例,通过AlertPluginManager类获取插件实例,调用实例的发送方法,最后更新结果。这是 Apache DolphinScheduler 的整个告警流程。
-
-需要注意的是,Alert server 启动的同时也启动了 RPC 服务,这是一种针对特殊类型任务,如 SQL 查询报表而设计的告警方式,可以让 Worker 通过 RPC 直接访问 Alert Server,利用 Alert 模块完成告警,这个数据不写入 DB。但从整体上来说,Apache DolphinScheduler 的告警模式还是以写 DB,异步交互的方式为主。
-
-<div align=center>
-<img src="/img/3-16/4.png"/>
-</div>
-
-定义工作流之后,可以在启动前设置通知策略,绑定告警组。
-
-<div align=center>
-
-<img src="/img/3-16/5.png"/>
-
-</div>
-
-在任务维度,可以配置超时告警,当任务超时可以触发报警。这里没有告警组配置,任务和工作流共用一个告警组,当任务超时,会推送到工作流设置的告警组。
-
-<div align=center>
-
-<img src="/img/3-16/6.png"/>
-
-</div>
-
-上图为系统告警配置的流程图。可以看到,一个工作流可以配置多个任务实例,任务可以配置超时触发告警,工作流成功或者失败可以触发告警。一个告警组可以绑定多个告警实例。这样的配置不太合理,我们希望告警实例也可以匹配工作流/任务实例的状态,也就是工作流成功和失败调用同一个告警组,但是触发不同的告警实例。这样使用起来更符合真实场景。
-
-<div align=center>
-
-<img src="/img/3-16/7.png"/>
-
-</div>
-
-创建告警组,一个告警组可以绑定多个告警实例。
-
-## 02 **大数据任务告警场景**
-
-<div align=center>
-
-<img src="/img/3-16/8.png"/>
-
-</div>
-
-以下是我们日常工作中的一些 常见的大数据任务告警场景。
-
-对于定时任务,在开始执行前、任务上线、下线或修改参数,以及任务执行成功或失败时都发送通知。区别是,对于同一任务不同结果,我们希望触发不同的通知,比如成功发短信通知或者钉钉微信群通知即可,而任务失败了需要在第一时间通知对应的研发人员,以得到更快的响应,这时候钉钉微信群中@对应研发人员或者电话通知会更及时。目前,公司的任务调度平台是任务中调用API 进行通知,这种与代码强耦合的方式极其不方便,实际上可以抽象成一个更为通用的模块来实现。
-Apache DolphinScheduler 的架构虽然符合实际场景需求,但问题在于告警模块页面配置只能选择成功触发通知,或失败触发通知,绑定的是同一个告警组,即无论成功还是失败,告警的途径是相同的,这一点并不满足我们在实际生产环境中需要不同结果以不同方式通知的需求。因此,我们对 Alert 模块进行了一些改造。
-
-## 03 **Alert 模块的改造**
-
-<div align=center>
-
-<img src="/2022-3-15/3-15/9.png"/>
-
-</div>
-
-改造的第一步是告警实例。此前,新增一个告警实例,触发告警就会触发该实例的 send 方法,我们希望在定义告警实例时可以绑定一个告警策略,有三个选项,成功发、失败发,以及成功和失败都发。
-
-
-在任务定义维度,有一个超时告警的功能,实际上对应失败的策略。
-
-<div align=center>
-
-<img src="/img/3-16/10.png"/>
-
-</div>
-
-上图为改造完成的配置页面,在创建告警实例页面,我们添加了一个告警类型字段,选择是在成功、失败,或者无论成功或失败时调用插件。
-
-<div align=center>
-
-<img src="/img/3-16/11.png"/>
-
-</div>
-
-上图为改造后Apache DolphinScheduler 告警模块的架构,我们对其中进行了两点改造。
-
-
-其一,在执行完工作流或任务时,如果触发告警,在写入DB时,会保存本次工作流或者任务的执行结果,具体是成功还是失败。
-
-第二,调用告警实例发送方法添加了一个逻辑判断,将告警实例与任务状态进行匹配,匹配则执行该告警实例发送逻辑,不匹配则过滤。
-
-
-改造后告警模块支持场景如下:
-
-<div align=center>
-<img src="/img/3-16/12.png"/>
-</div>
-
-详细设计请参考 issue:[https://github.com/apache/dolphinscheduler/issues/7992](https://github.com/apache/dolphinscheduler/issues/7992)
-
-代码详见:[https://github.com/apache/dolphinscheduler/pull/8636](https://github.com/apache/dolphinscheduler/pull/8636)
-
-此外,我们还针对 Apache DolphinScheduler 的告警模块向社区提出几点优化的建议,感兴趣的小伙伴可以跟进 issue,一起来做后续的工作:
-
-* 工作流启动或上下线或参数修改时,可以触发通知;
-* 告警场景针对 worker 的监控,如果 worker 挂掉或和 ZK 断开失去心跳,会认为 worker 宕机,会触发告警,但会默认匹配 ID 为 1 的告警组。这样的设置是在源码中写明的,但不看源码不知道其中的逻辑,不会专门设置ID为1的告警组,无法第一时间得到worker宕机的通知;
-* 告警模块目前支持飞书、钉钉、微信、邮件等多种插件,这些插件适用于国内用户,但国外用户可能使用不同的插件,如思科使用的 Webex Teams,国外常用告警插件 PagerDuty,我们也都进行开发并贡献给了社区。同时还有一些比较常用的比如Microsoft Teams等,感兴趣的小伙伴也可以提个PR,贡献到社区。
-最后一点,可能大数据领域的小伙伴对于前端不太熟悉,想要开发并贡献告警插件,但是想到需要开发前端就不想进行下去了。开发 Apache DolphinScheduler 告警插件是不需要写前端代码的,只需要在新建告警实例插件时,在 Java 代码中配置好页面中需要输入的参数或者需要选择的按钮(源码详见org.apache.dolphinscheduler.spi.params),系统会自动格式化成 JSON 格式,前端通过form-create 可以通过 JSON 自动渲染成页面。因此,完全不用担心写前端的问题。
diff --git a/blog/zh-cn/Hangzhou_cisco.md.md b/blog/zh-cn/Hangzhou_cisco.md.md
deleted file mode 100644
index cab6fba..0000000
--- a/blog/zh-cn/Hangzhou_cisco.md.md
+++ /dev/null
@@ -1,140 +0,0 @@
-# 杭州思科对 Apache DolphinScheduler Alert 模块的改造
-
-<div align=center>
-<img src="/img/3-16/1.png"/>
-</div>
-
-杭州思科已经将 Apache DolphinScheduler 引入公司自建的大数据平台。目前,**杭州思科大数据工程师 李庆旺** 负责 Alert 模块的改造已基本完成,以更完善的 Alert 模块适应实际业务中对复杂告警的需求。
-<div align=center>
-
-<img src="/img/3-16/2.png"/>
-
-</div>
-
-李庆旺
-
-杭州思科 大数据工程师,主要负责 Spark、调度系统等大数据方向开发。
-
-我们在使用原有的调度平台处理大数据任务时,在操作上多有不便。比如一个对数据进行处理聚合分析的任务,首先由多个前置 Spark 任务对不同数据源数据进行处理、分析。最后的 Spark 任务对这期间处理的结果进行再次聚合、分析,得到我们想要的最终数据。但遗憾的是当时的调度平台无法串行执行多个任务,需要估算任务处理时间来设置多个任务的开始执行时间。同时其中一个任务执行失败,需要手动停止后续任务。这种方式既不方便,也不优雅。
-
-而 Apache DolphinScheduler 的核心功能——**工作流定义可以将任务串联起来**,完美契合我们的需求。于是,我们将 Apache DolphinScheduler 引入自己的大数据平台,而我主要负责 Alert 模块改造。目前我们其他同事也在推进集成 K8s,希望未来任务在 K8s 中执行。
-
-今天分享的是 Alert 模块的改造。
-
-## 01 **Alert 模块的设计**
-
-<div align=center>
-
-<img src="/img/3-16/3.png"/>
-
-</div>
-
-DolphinScheduler Alert 模块的设计
-
-Apache DolphinScheduler 1.0 版本的 Alert 模式使用配置alert.properties的方式,通过配置邮箱、短信等实现告警,但这样的方式已经不适用于当前的场景了。官方也进行过告警模块重构,详情设计思路参考官方文档:
-
-[https://github.com/apache/dolphinscheduler/issues/3049](https://github.com/apache/dolphinscheduler/issues/3049)
-
-[https://dolphinscheduler.apache.org/zh-cn/development/backend/spi/alert.html](https://dolphinscheduler.apache.org/zh-cn/development/backend/spi/alert.html)
-
-
-Apache DolphinScheduler 告警模块是一个独立启动的服务,核心之一是 AlertPluginManager 类。告警模块集成了很多插件,如钉钉、微信、飞书、邮件等,以独立的形式写在源码中,启动服务时会解析插件并将配置的参数格式化成JSON形式,前端通过JSON自动渲染出页面。AlertPluginManager 在启动时会缓存插件到内存中。AlertServer类会启动线程池,定时扫描DB。
-
-当工作流配置了通知策略,同时Worker 执行工作流结束,执行结果匹配通知策略成功后,DB插入告警数据后,线程池扫描 DB,调用AlertSender 类的send方法传入告警数据。告警数据绑定的是告警组,一个告警组对应了多个告警实例。AlertSender类遍历告警实例,通过AlertPluginManager类获取插件实例,调用实例的发送方法,最后更新结果。这是 Apache DolphinScheduler 的整个告警流程。
-
-需要注意的是,Alert server 启动的同时也启动了 RPC 服务,这是一种针对特殊类型任务,如 SQL 查询报表而设计的告警方式,可以让 Worker 通过 RPC 直接访问 Alert Server,利用 Alert 模块完成告警,这个数据不写入 DB。但从整体上来说,Apache DolphinScheduler 的告警模式还是以写 DB,异步交互的方式为主。
-
-<div align=center>
-<img src="/img/3-16/4.png"/>
-</div>
-
-定义工作流之后,可以在启动前设置通知策略,绑定告警组。
-
-<div align=center>
-
-<img src="/img/3-16/5.png"/>
-
-</div>
-
-在任务维度,可以配置超时告警,当任务超时可以触发报警。这里没有告警组配置,任务和工作流共用一个告警组,当任务超时,会推送到工作流设置的告警组。
-
-<div align=center>
-
-<img src="/img/3-16/6.png"/>
-
-</div>
-
-上图为系统告警配置的流程图。可以看到,一个工作流可以配置多个任务实例,任务可以配置超时触发告警,工作流成功或者失败可以触发告警。一个告警组可以绑定多个告警实例。这样的配置不太合理,我们希望告警实例也可以匹配工作流/任务实例的状态,也就是工作流成功和失败调用同一个告警组,但是触发不同的告警实例。这样使用起来更符合真实场景。
-
-<div align=center>
-
-<img src="/img/3-16/7.png"/>
-
-</div>
-
-创建告警组,一个告警组可以绑定多个告警实例。
-
-## 02 **大数据任务告警场景**
-
-<div align=center>
-
-<img src="/img/3-16/8.png"/>
-
-</div>
-
-以下是我们日常工作中的一些 常见的大数据任务告警场景。
-
-对于定时任务,在开始执行前、任务上线、下线或修改参数,以及任务执行成功或失败时都发送通知。区别是,对于同一任务不同结果,我们希望触发不同的通知,比如成功发短信通知或者钉钉微信群通知即可,而任务失败了需要在第一时间通知对应的研发人员,以得到更快的响应,这时候钉钉微信群中@对应研发人员或者电话通知会更及时。目前,公司的任务调度平台是任务中调用API 进行通知,这种与代码强耦合的方式极其不方便,实际上可以抽象成一个更为通用的模块来实现。
-Apache DolphinScheduler 的架构虽然符合实际场景需求,但问题在于告警模块页面配置只能选择成功触发通知,或失败触发通知,绑定的是同一个告警组,即无论成功还是失败,告警的途径是相同的,这一点并不满足我们在实际生产环境中需要不同结果以不同方式通知的需求。因此,我们对 Alert 模块进行了一些改造。
-
-## 03 **Alert 模块的改造**
-
-<div align=center>
-
-<img src="/2022-3-15/3-15/9.png"/>
-
-</div>
-
-改造的第一步是告警实例。此前,新增一个告警实例,触发告警就会触发该实例的 send 方法,我们希望在定义告警实例时可以绑定一个告警策略,有三个选项,成功发、失败发,以及成功和失败都发。
-
-
-在任务定义维度,有一个超时告警的功能,实际上对应失败的策略。
-
-<div align=center>
-
-<img src="/img/3-16/10.png"/>
-
-</div>
-
-上图为改造完成的配置页面,在创建告警实例页面,我们添加了一个告警类型字段,选择是在成功、失败,或者无论成功或失败时调用插件。
-
-<div align=center>
-
-<img src="/img/3-16/11.png"/>
-
-</div>
-
-上图为改造后Apache DolphinScheduler 告警模块的架构,我们对其中进行了两点改造。
-
-
-其一,在执行完工作流或任务时,如果触发告警,在写入DB时,会保存本次工作流或者任务的执行结果,具体是成功还是失败。
-
-第二,调用告警实例发送方法添加了一个逻辑判断,将告警实例与任务状态进行匹配,匹配则执行该告警实例发送逻辑,不匹配则过滤。
-
-
-改造后告警模块支持场景如下:
-
-<div align=center>
-<img src="/img/3-16/12.png"/>
-</div>
-
-详细设计请参考 issue:[https://github.com/apache/dolphinscheduler/issues/7992](https://github.com/apache/dolphinscheduler/issues/7992)
-
-代码详见:[https://github.com/apache/dolphinscheduler/pull/8636](https://github.com/apache/dolphinscheduler/pull/8636)
-
-此外,我们还针对 Apache DolphinScheduler 的告警模块向社区提出几点优化的建议,感兴趣的小伙伴可以跟进 issue,一起来做后续的工作:
-
-* 工作流启动或上下线或参数修改时,可以触发通知;
-* 告警场景针对 worker 的监控,如果 worker 挂掉或和 ZK 断开失去心跳,会认为 worker 宕机,会触发告警,但会默认匹配 ID 为 1 的告警组。这样的设置是在源码中写明的,但不看源码不知道其中的逻辑,不会专门设置ID为1的告警组,无法第一时间得到worker宕机的通知;
-* 告警模块目前支持飞书、钉钉、微信、邮件等多种插件,这些插件适用于国内用户,但国外用户可能使用不同的插件,如思科使用的 Webex Teams,国外常用告警插件 PagerDuty,我们也都进行开发并贡献给了社区。同时还有一些比较常用的比如Microsoft Teams等,感兴趣的小伙伴也可以提个PR,贡献到社区。
-最后一点,可能大数据领域的小伙伴对于前端不太熟悉,想要开发并贡献告警插件,但是想到需要开发前端就不想进行下去了。开发 Apache DolphinScheduler 告警插件是不需要写前端代码的,只需要在新建告警实例插件时,在 Java 代码中配置好页面中需要输入的参数或者需要选择的按钮(源码详见org.apache.dolphinscheduler.spi.params),系统会自动格式化成 JSON 格式,前端通过form-create 可以通过 JSON 自动渲染成页面。因此,完全不用担心写前端的问题。
diff --git a/blog/zh-cn/How_Does_360_DIGITECH_process_10_000+_workflow_instances_per_day.md b/blog/zh-cn/How_Does_360_DIGITECH_process_10_000+_workflow_instances_per_day.md
deleted file mode 100644
index a6a05c0..0000000
--- a/blog/zh-cn/How_Does_360_DIGITECH_process_10_000+_workflow_instances_per_day.md
+++ /dev/null
@@ -1,156 +0,0 @@
-# 日均处理 10000+ 工作流实例,Apache DolphinScheduler 在 360 数科的实践
-
-<div align=center>
-<img src="/img/2022-3-11/1.jpeg"/>
-</div>
-
->从 2020 年起,360 数科全面将调度系统从 Azkaban 迁移到 Apache DolphinScheduler。作为 DolphinScheduler 的资深用户,360 数科如今每天使用 DolphinScheduler 日均处理 10000+ 工作流实例。为了满足大数据平台和算法模型业务的实际需求,360 数科在 DolphinScheduler 上进行了告警监控扩展、worker增加维护模式、多机房等改造,以更加方便运维。他们具体是如何进行二次开发的呢?**360 数科大数据工程师 刘建敏** 在不久前的 Apache DolphinScheduler 2 月份的 Meetup 上进行了详细的分享。
-<div align=center>
-<img style="width: 25%;" src="/img/2022-3-11/2.png"/>
-</div>
-
-刘建敏
-
-360 数科 大数据工程师,主要从事 ETL 任务与调度框架的研究,大数据平台以及实时计算平台的开发。
-
-## 从 Azkaban 迁移到 DolphinScheduler
-
-2019 年之前,360 数科采用 Azkaban 调度进行大数据处理。
-
-Azkaban 是由 Linkedin 开源的批量工作流任务调度器。其安装简单,只需安装 web 与 executor server,就可以创建任务,并通过上传 zip 包实现工作流调度。
-
-Azkaban 的 web-executor 架构如下图所示:
-
-<div align=center>
-<img style="width: 40%;" src="/img/2022-3-11/3.png"/>
-</div>
-
-
-### Azkaban 的缺点
-
-Azkaban 适用于场景简单的调度,经过三年时间的使用,我们在发现它存在三个重要缺陷:
-
-1. 体验性差
-没有可视化创建任务功能,创建与修改任务都需要通过上传 zip 包来实现,这并不方便;另外,Azkaban 没有管理资源文件功能。
-
-2. 功能不够强大
-Azkaban 缺乏一些生产环境中不可或缺的功能,比如补数、跨任务依赖功能;用户与权限管理太弱,调度配置不支持按月,在生产上我们需要用很多其他方式进行弥补。
-
-3. 稳定性不够好
-最重要的一点是 Azkaban 稳定性不够,当 executor 负载过高时,任务经常会出现积压;小时级别或分钟级别的任务容易出现漏调度;没有超时告警,虽然我们自己开发了有限的短信告警,但还是容易出现生产事故。
-
-<div align=center>
-<img src="/img/2022-3-11/4.png"/>
-</div>
-
-
-针对这些缺陷,我们在 2018 曾进行过一次改造,但由于 Azkaban 源码复杂,改造的过程很是痛苦,因此我们决定重新选型。当时,我们测试了 Airflow、DolphinScheduler 和 XXL-job,但 Airflow Python 的技术栈与我们不符,而 XXL-job 功能又过于简单,显然,DolphinScheduler 是更好的选择。
-
-2019 年,我们 Folk 了 EasyScheduler 1.0 的代码,在 2020 年进行改造与调度任务的部分迁移,并上线运行至现在。
-
-### DolphinScheduler 选型调研
-
-为什么我们选择 DolphinScheduler?因为其有四点优势:
-
-1. 去中心化结构,多 Master 多 Worker;
-2. 调度框架功能强大,支持多种任务类型,具备跨项目依赖、补数功能;
-3. 用户体验性好,可视化编辑 DAG 工作流,操作方便;
-4. Java 技术栈,扩展性好。
-###
-改造过程非常流畅,我们顺利地将调度系统迁移到了 DolphinScheduler。
-
-## DolphinScheduler 的使用
-在 360 数科,DolphinScheduler 不仅用于大数据部门,算法部门也在使用其部分功能。为了让算法模型部们更方便地使用 DolphinScheduler 的功能,我们将其整合进了我们自己的毓数大数据平台。
-
-### 毓数大数据平台
-
-<div align=center>
-<img src="/img/2022-3-11/5.png"/>
-</div>
-毓数是一个由基础组件、毓数平台、监控运维和业务支撑层组成的大数据平台,可实现查询、数据实时计算、消息队列、实时数仓、数据同步、数据治理等功能。其中,离线调度部分便是通过 DolphinScheduler 调度数据源到 Hive 数仓的 ETL 任务,以及支持 TiDB 实时监控,以实时数据报表等功能。
-
-### DolphinScheduler 嵌套到毓数
-
-为了支持公司算法建模的需求,我们抽取了常用的一些节点,并嵌套了一层 UI,并调用 API。
-
-<div align=center>
-<img src="/img/2022-3-11/6.png"/>
-</div>
-算法部门多用 Python 脚本和 SQL 节点,用框表逻辑进行定时,再配置机器学习算法进行训练,组装数据后用 Python 调用模型生成模型分。我们封装了一些 Kafka 节点,通过 Spark 读取 Hive 数据并推送到 Kafka。
-
-### 任务类型
-
-<div align=center>
-<img src="/img/2022-3-11/7.png"/>
-</div>
-DolphinScheduler 支持的任务类型有 Shell、SQL、Python 和 Jar。其中,Shell 支持 Sqoop DataX mr 同步任务和 Hive-SQL、Spark-SQL;SQL 节点主要是支持 TiDB SQL(处理上游分库分表的监控) 和 Hive SQL;Python 任务类型支持离线调用模型脚本等;Jar 包主要支持 Spark Jar 离线管理。
-
-### 任务场景
-
-<div align=center>
-<img src="/img/2022-3-11/8.png"/>
-</div>
-DolphinScheduler 的任务场景主要是将各种数据源,如 MySQL、Hbase 等数据源调度同步至 Hive,再通过 ETL 工作流直接生成 DW。通过 Python 脚本组装或调用,生成模型和规则结果,再推送到 Kafka。Kafka 会给出风控系统调额、审批和分析,并将结果反馈给业务系统。这就是 DolphinScheduler 调度流程的一个完整工作流示例。
-
-## DolphinScheduler 的运维
-
-目前 ,DolphinScheduler 日均处理工作流已达到 10000+ 的规模,很多离线报表依赖于 DolphinScheduler,因此运维非常重要。
-
-###
-在 360 数科,对 DolphinScheduler 的运维主要分为三部分:
-
-* DS 依赖组件运维
-<div align=center>
-<img src="/img/2022-3-11/9.png"/>
-</div>
-
-DolphinScheduler 依赖组件的运维主要是针对 MySQL 监控和 Zookeeper 监控。
-
-因为工作流定义元信息、工作流实例和任务实例、Quartz 调度、Command 都依赖 MySQL,因此 MySQL 监控至关重要。曾经有一次机房网络中断,导致很多工作流实例出现漏调度,之后通过 MySQL 监控才得以排查。
-
-Zookeeper 监控的重要性也不言而喻,Master worker 状态和 task 队列都依赖 Zookeeper,但运行至今,Zookeeper 都比较稳定,还未有问题发生。
-
-* Master 与 Worker 状态监控
-我们都知道,Master 负责的是任务切分,实际上对业务的影响并不是很大,因此我们采用邮件监控即可;但 Worker 挂掉会导致任务延迟,增加集群压力。另外,由于之前 Yarn 运行任务不一定被成功地 kill,任务容错还可能导致 Yarn 任务重复运行,因此我们对 Worker 状态采用电话告警。
-
-### Grafana 大盘监控工作流实例状态
-
-<div align=center>
-<img src="/img/2022-3-11/10.png"/>
-</div>
-此外,我们还为运维创建了 Grafana 监控看板,可以实时监控工作流实例的状态,包括工作流实例的数量,各项目工作流实例运行状态,以及超时预警设置等。
-
-## DolphinScheduler 改造
-
-### 体验性改造
-
-1. 增加个人可以授权资源文件与项目,资源文件区分编辑与可读权限,方便授权;
-2. 扩展全局变量(流程定义id, 任务id等放到全局变量中),提交到yarn上任务可追踪到调度的任务,方便集群管理,统计工作流实例锁好资源,利于维护
-3. 工作流复制、任务实例查询接口优化,提高查询速度,以及 UI 优化。
-### 增加短信告警改造
-
-因为原有的邮箱告警不足以保证重要任务的监控,为此我们在 UI 上增加了 SMS 告警方式,保存工作流定义。另外,我们还把告警 receivers 改造成用户名,通过 AlertType 扩展成短信、邮箱等告警方式关联到用户信息,如手机号等,保证重要性任务失败后可以及时收到告警。
-
-### Worker 增加维护模式改造
-
-此外,当 worker 机器需要维护时,需要这台 worker 上不会有新的运行任务提交过来。为此,我们对 worker 增加了维护模式改造,主要包括 4 点:
-
-1. UI 设置 worker 进行维护模式,调用 API 写入worker 指定的 zk 路径;
-2. WorkerServer 进行定时调度轮询是否维护模式;
-3. WorkerServer 进行维护模式,FetchTaskThread 不获取新增任务;
-4. worker 任务运行结束,可进行重启。
-经过以上改造,我们运维的压力大大减轻。
-
-### 多机房改造
-
-最后,我们还进行了多机房改造。因为我们之前有 2 套集群,分布在不同的机房,我们的改造目标是在调度中设置多机房,这样当某一个机房出现故障后,可一键切换到其他机房使用,实现多机房使用同一套调度框架。
-
-<div align=center>
-<img src="/img/2022-3-11/11.png"/>
-</div>
-
-改造的切入点就是在调度中设置多机房,以保证某一任务可以在多机房启动。改造流程如下:
-
-1. 在各机房分别部署 ZK,Master 与 Worker 注册到对应的机房,Master 负责任务切分,Worker 负责任务处理,各自处理各机房的任务;
-2. schedule 与 command 带上 datacenter 信息
-3. 为保证双机房任务切换,资源文件进行上机房任务上传,同时改动任务接口,任务依赖、Master容错都需要改造过滤对应机房。
\ No newline at end of file
diff --git a/blog/zh-cn/K8s_Cisco_Hangzhou.md b/blog/zh-cn/K8s_Cisco_Hangzhou.md
deleted file mode 100644
index 19ed29d..0000000
--- a/blog/zh-cn/K8s_Cisco_Hangzhou.md
+++ /dev/null
@@ -1,116 +0,0 @@
-# 全面拥抱 K8s,ApacheDolphinScheduler 应用与支持 K8s 任务的探索
-
-<div align=center>
-<img src="/img/2022-03-21/1.png"/>
-</div>
-
->K8s 打通了主流公私云之间的壁垒,成为唯一连通公私云的基础架构平台。K8s 是未来云端的趋势,全面拥抱 K8s 成为更多企业推动 IT 现代化的选择。
->>杭州思科基于 Apache DolphinScheduler,也在进行支持 K8s 的相关探索,且部分功能已经成功上线运行。今天,来自杭州思科的大数据工程师 李千,将为我们分享他们的开发成果。
-
-<div align=center>
-<img src="/img/2022-03-21/2.png"/>
-</div>
-
-李千,杭州思科 大数据工程师,多年大数据解决方案经验,有 Spark,Flink,以及调度系统,ETL 等方面的项目经验。
-
-正文:
-
-本次我的分享主要分为这几部分,Namespace 管理,持续运行的 K8s 任务,K8s 任务的工作流调度,以及未来的规划。
-
-## Namespace 管理
-
-### 资源管理
-
-第一部分中,我首先介绍一下资源管理。我们引入资源管理目的,是为了利用 K8s 集群运行不属于 Apache DolphinScheduler 所属的调度概念上的任务,比如 Namespace,更类似于一个数据解决方案,如果 CPU 的 memory 有限,就可以限制队列中的资源,实现一定的资源隔离。
-
-以后我们可能会把一部分资源管理功能合并到 Apache DolphinScheduler 上。
-
-### 增删维护管理
-
-我们可以加一些 Type,即标记的类型,比如某些 Namespace 只允许跑一些特定类型的 job。我们可以统计Namespace 下面的任务数量、pod 数量、请求资源量、请求等,查看队列的资源使用情况,界面默认只有管理员才可以操作。
-<div align=center>
-<img src="/img/2022-03-21/3.png"/>
-</div>
-
-### 多 K8s 集群
-
-K8s 支持多个集群,我们通过 Apache DolphinScheduler 客户端连接到多个 K8s 集群,batch、PROD 等可以搭建多套这K8s 集群,并通过 Namespace 支持多套 K8s 集群。
-
-我们可以编辑所开发的集群,修改所有的属性,如内存等。
-
-在新版中,用户权限的管理位于 user master 中,可以给某个用户授权,允许用户可以向某个 Namespace 上提交任务,并编辑资源。
-
-## 02 持续运行的 K8s 任务
-
-第二部分是关于我们目前已经支持的任务类型。
-
-### 启动不退出的普通镜像,如 ETL 等
-
-比如 ETL 这种提交完之后必须要手动操作才会退出的任务。这种任务一旦提交,就会把数据 sink,这种任务理论上只要不做升级,它永远不会停。
-<div align=center>
-<img src="/img/2022-03-21/4.png"/>
-</div>
-
-这种任务其实调度可能用不到,因为它只有启停这两种状态。所以,我们把它放在一个实时列表中,并做了一套监控。POD是实时运行的状态,主要是通过一个 Fabris operator 进行交互,可以进行动态进行扩展,以提高资源利用率。
-
-### Flink 任务
-
-我们对于 CPU 的管理可以精确到 0.01%,充分利用了 K8s 虚拟 CPU。
-<div align=center>
-<img src="/img/2022-03-21/5.png"/>
-</div>
-<div align=center>
-<img src="/img/2022-03-21/6.png"/>
-</div>
-<div align=center>
-<img src="/img/2022-03-21/7.png"/>
-</div>
-另外,我们也常用 Flink 任务,这是一种基于 ETL 的扩展。Flink 任务界面中包含编辑、查看状态、上线、下线、删除、执行历史,以及一些监控的设计。我们用代理的模式来设计 Flink UI,并开发了权限管控,不允许外部的人随意修改。
-
-Flink 默认了基于 checkpoint 启动,也可以指定一个时间创建,或基于上一次 checkpoint 来提交和启动。
-
-Flink 任务支持多种模式镜像版本,因为 K8s 本身就是运行镜像的,可以直接指定一些镜像来选择使用包,或通过文件上传的方式提交任务。
-
-另外,Batch 类型的任务可能一次运行即结束,或是按照周期来调度,自动执行完后退出,这和 Flink 不太一样,所以对于这种类型的任务,我们还是基于 Apache DolphinScheduler 做。
-
-## 03 K8s 任务的运行
-
-### K8s 任务的工作流调度
-
-我们在最底层增加了一些 Flink 的 batch 和 Spark 的 batch 任务,添加了一些配置,如使用的资源,所运行的 namespace 等。镜像信息可以支持一些自定义参数启动,封装起来后就相当于插件的模式,Apache DolphinScheduler 完美地扩展了它的功能。
-<div align=center>
-<img src="/img/2022-03-21/8.png"/>
-</div>
-
-### Spark 任务
-
-Spark 任务下可以查看 CPU 等信息,上传文件支持 Spark Jar 包,也可以单独上传配置文件。
-<div align=center>
-<img src="/img/2022-03-21/9.png"/>
-</div>
-
-这种多线程的上层,可以大幅提高处理速度。
-
-## 04 其他和规划
-
-### Watch 状态
-
-<div align=center>
-<img src="/img/2022-03-21/10.jpeg"/>
-</div>
-
-除了上述改动,我们还对任务运行状态进行了优化。
-
-当提交任务后,实际情况下运行过程中可能会出现失败,甚至任务的并行度也会基于某些策略发生改变。这时,我们就需要一种 watch 的方式来动态实时地来获取任务状态,并同步给 Apache DolphinScheduler 系统,以保证界面上看到的状态一定是最准确的。
-
-Batch 做不做 watch 都可以,因为这不是一个需要全量监听的独立任务而且 namespace 的资源使用率也是基于 watch 模式,这样就可以保证状态都是准确的。
-
-### 多环境
-
-多环境是指,同一个任务可以推送到不同的 K8s 集群上,比如同一个Flink 任务。
-
-从代码上来说,watch 有两种方式,一种是单独放一些 pod,比如当使用了 K8s 模块时,定义多个 K8s 集群的信息,在每个集群上创建一些watch pod 来监听集群中的任务状态,并做一些代理的功能。另一种是跟随api或单独服务,启动一个监听服务监听所有k8s集群。但这样无法而外做一些k8s内外网络的代理。
-
-Batch 有多种方案,一种是可以基于 Apache DolphinScheduler 自带功能,通过同步的方式进行 watch,这和 Apache DolphinScheduler 比较兼容。关于这方面的工作我们未来可能很快会提交 PR。Spark 使用相同的模式,提供一些 pod 来进行交互,而内部代码我们使用的是 Fabric K8s 的 client。
-
-今后,我们将与 Apache DolphinScheduler 一起共建,陆续支持这里讨论的功能,并和大家分享更多关于我们的工作进展。谢谢大家!
diff --git a/blog/zh-cn/Lizhi-case-study.md b/blog/zh-cn/Lizhi-case-study.md
deleted file mode 100644
index ea716e2..0000000
--- a/blog/zh-cn/Lizhi-case-study.md
+++ /dev/null
@@ -1,135 +0,0 @@
-
-# 荔枝机器学习平台与大数据调度系统“双剑合璧”,打造未来数据处理新模式!
-
-
-<div align=center>
-<img src="https://s1.imgpp.com/2021/11/23/1637566412753.png"/>
-</div>
-
-
-> 编 者 按:在线音频行业在中国仍是蓝海一片。根据 CIC 数据显示,中国在线音频行业市场规模由 2016 年的 16 亿元增长至 2020 年的 131 亿元,复合年增长率为 69.4%。随着物联网场景的普及,音频场景更是从移动端扩展至车载端、智能硬件端、家居端等各类场景,从而最大限度发挥音频载体的伴随性优势。
-
-> 近年来,国内音频社区成功上市的案例接踵而至。其中,于 2020 年在纳斯达克上市的“在线音频”第一股荔枝,用户数早已超过 2 亿。进入信息化时代,音频行业和所有行业一样,面对平台海量 UGC 数据的产生,音频用户和消费者对于信息传递的效率也有着高要求,互联网音频平台也希望信息能够精准、快速地推送给用户,同时保证平台 UGC 内容的安全性。 为了最高效地达到这一目标,利用日益成熟的机器学习技术与大数据调度系统相结合,以更好地海量数据处理任务,成为各大音频平台探索的方向。荔枝的机器学习智能推荐平台就走在探索的最前沿,尝试将机器学习平台与大数据调度系统 DolphinScheduler 相结合。
-
-
-## 01 背景
-
-<div align=center>
-<img src="https://s1.imgpp.com/2021/11/23/radio-g360707f44_1920.md.jpg"/>
-</div>
-
-
-荔枝是一个快速发展的UGC音频社区公司,非常注重 AI 和数据分析两个领域的技术。因为 AI 可以在海量碎片化的声音中找到每个用户喜欢的声音,并将其打造成一个可持续发展的生态闭环。而数据分析可以为公司快速发展的业务提供指引。两者都需要对海量数据做处理,所以需要使用大数据调度系统。荔枝机器学习平台团队尝试利用大数据调度平台的调度能力与机器学习平台的能力相结合,处理每天涌进荔枝平台的海量 UGC 音频内容。
-
-
-在 2020 年初之前,荔枝机器学习平台使用的是 Azkaban 调度系统,但是对于 AI 来说,虽然大数据调度的 sql/shell/python 脚本和其他大数据相关组件也能完成整个AI 流程,但不够易用,也很难复用。机器学习平台是专门为 AI 构建的一套系统,它将 AI 开发范式,即获取数据、数据预处理、模型训练、模型预测、模型评估和模型发布过程抽象成组件,每个组件提供多个实现,用 DAG 串联,使用拖拽和配置的方式,就可以实现低代码开发。
-
-目前,荔枝每天有 1600+ 流程和 12000+ 任务(IDC)在 DolphinScheduler 上顺利运行。
-
-
-## 02 机器学习平台开发中的挑战
-
-荔枝在进行机器学习平台开发时,对于调度系统有一些明确的需求:
-
-1、需要对海量数据存算,比如筛选样本、生成画像、特征预处理、分布式模型训练等;
-2、需要 DAG 执行引擎,将获取数据->数据预处理->模型训练->模型预测->模型评估->模型发布等流程用 DAG 串联起来执行。
-
-而使用之前的 Azkaban 调度系统进行开发时,他们遇到了一些挑战:
-
-挑战1:开发模式繁琐,需要自己打包脚本和构建 DAG 依赖,没有 DAG 拖拽的实现;
-挑战2:组件不够丰富,脚本编写的 AI 组件不够通用,需要重复开发,且易出错;
-挑战3:单机部署,系统不稳定,容易出故障,任务卡顿容易导致下游任务都无法进行。
-
-
-## 03 切换到DolphinScheduler
-
-
-
-在旧系统中踩坑无数后,荔枝机器学习团队,包括推荐系统开发工程师喻海斌、林燕斌、谢焕杰和郭逸飞,决定采用 DolphinScheduler。喻海斌表示,他们团队调度系统的主要使用人员为推荐算法 > 数据分析 > 风控算法 > 业务开发(依次减弱),他们对调度系统了解不多,所以对于一个简单易用,可拖拉拽实现的调度系统的需求比较大。相比之下,DolphinScheduler 完美切合了他们的需求:
-
-
-1、分布式去中心化架构及容错机制,保障系统高可用;
-
-2、可视化 DAG 拖拽 UI,使用简洁方便,迭代效率高;
-
-3、拥有丰富的组件,且可以轻松开发集成自己的组件;
-
-4、社区活跃,无后顾之忧;
-
-5、与机器学习平台运行模式非常接近,使用 DAG 拖拽 UI 编程。
-
-
-## 04 应用案例
-
-
-
-选型 DolphinScheduler 之后,荔枝机器学习平台在其之上进行了二次开发,并将成果应用于实际业务场景汇总,目前主要是在推荐和风控场景。其中,推荐场景包括声音推荐、主播推荐、直播推荐、播客推荐、好友推荐等,风控场景包括支付、广告、评论等场景中的风险管控。
-
-在平台底层,荔枝针对机器学习的五大范式,获取训练样本、数据预处理、模型训练、模型评估、模型发布过程,进行了扩展组件优化。
-
-一个简单的xgboost案例:
-
-<div align=center>
-<img src="https://s1.imgpp.com/2021/11/23/1.png"/>
-</div>
-
-
-### 1、获取训练样本
-
-目前还没有实现像阿里 PAI 那样直接从 Hive 选取数据,再对样本进行 join,union,拆分等操作,而是直接使用 shell 节点把样本处理好。
-
-### 2. 数据预处理
-
-Transformer&自定义预处理配置文件,训练和线上采用同一份配置,获取特征后进行特征预处理。里面包含了要预测的 itemType 及其特征集,用户 userType 及其特征集,关联和交叉的 itemType 及其特征集。定义每个特征预处理的 transformer 函数,支持自定义transformer 和热更新,xgboost 和 tf 模型的特征预处理。经过此节点后,才是模型训练真正要的数据格式。这个配置文件在模型发布时也会带上,以便保持训练和线上预测是一致的。这个文件维护在 DolphinScheduler 的资源中心。
-
-<div align=center>
-<img src="https://s1.imgpp.com/2021/11/23/2.png"/>
-</div>
-
-### 3. Xgboost 训练
-
-支持 w2v、xgboost、tf模型训练组件,训练组件先使用 TensorFlow或 PyTorch封装,再封装成 DolphinScheduler 组件。
-
-例如,xgboost 训练过程中,使用 Python封装好 xgboost训练脚本,包装成 DolphinScheduler 的 xgboost 训练节点,在界面上暴露训练所需参数。经过“训练集数据预处理”输出的文件,经过 hdfs 输入到训练节点。
-
-<div align=center>
-<img src="https://s1.imgpp.com/2021/11/23/3.png"/>
-</div>
-
-
-### 4. 模型发布
-
-发布模型会把模型和预处理配置文件发到 HDFS,同时向模型发布表插入记录,模型服务会自动识别新模型,进而更新模型,对外提供在线预测服务。
-
-<div align=center>
-<img src="https://s1.imgpp.com/2021/11/23/4.png"/>
-</div>
-
-
-喻海斌表示,基于历史和技术原因,目前荔枝还没有做到像阿里 PAI 那样真正的机器学习平台,但其实践经验已经证明基于 DolphinScheduler 可以做到类似的平台效果。
-
-此外,荔枝还基于 DolphinScheduler 进行了很多二次开发,让调度系统更加符合实际业务需求,如:
-
-1. 上线工作流定义的时候弹窗是否上线定时
-2. 增加所有工作流定义的显示页面,以方便查找
- a) 增加工作流定义筛选并跳转到工作流实例页面,并用折线图表示其运行时长的变化
- b) 工作流实例继续下潜到任务实例
-3. 运行时输入参数,还可以进行配置禁用任务节点
-
-
-## 05 基于调度的机器学习平台实现或将成未来趋势
-
-
-
-深度学习是未来的大趋势,荔枝也针对深度学习模型开发了新的组件,目前已完成 tf 整个流程,后续在开发的还有 LR 和 GBDT 模型相关组件。后两者相对简单,上手更快,一般的推荐场景都可以使用,迭代更快,实现之后可以让荔枝机器学习平台更加完善。
-
-荔枝认为,如果调度系统可以在内核稳定性,支持拖拽 UI,方便扩展的组件化,任务插件化,以及和任务参数传递方向上更加出色,基于调度系统实现机器学习平台,未来有可能会成为业界的普遍做法之一。
-
-
-## 06 期待就是我们前进的动力
-
-
-
-用户的期待就是 DolphinScheduler 前进的动力,荔枝也对 DolphinScheduler 的未来发展提出了很多具有指导性意义的建议,如期待 DolphinScheduler 未来可以优化插件机制,精减成一个专注于调度的“微内核”,并集成组件插件化,同时支持 UI 插件化,后台开发可以定制插件的 UI 界面。此外,荔枝还希望 DolphinScheduler 能够优化MySQL 表维护的“字典管理”,包含 key,value,desc 等主要字段,方便用户把一些易变量维护在字典表中,而不是维护在 .properties 文件里,这使得配置可以运行时更新,比如某个阈值,避免新增组件带来的配置参数放在 .properties 文件里导致的臃肿和不易维护等问题。
-
-最后,荔枝也表达了对 DolphinScheduler 寄语的期待,希望社区发展越来越好,探索更加广阔的领域,在海外也能取得更好的成绩,实现可持续的商业化开源!
diff --git a/blog/zh-cn/Meetup_2022_02_26.md b/blog/zh-cn/Meetup_2022_02_26.md
deleted file mode 100644
index 94232f6..0000000
--- a/blog/zh-cn/Meetup_2022_02_26.md
+++ /dev/null
@@ -1,89 +0,0 @@
-# 直播报名火热启动 | 2022 年 Apache DolphinScheduler Meetup 首秀!
-
-
-<div align=center>
-<img src="/img/2022-02-26/07.png"/>
-</div>
-
-
-各位关注 Apache DolphinScheduler 的小伙伴们大家好呀!相信大家都已经从热闹的春节里回过神来,重新投入到忙碌的工作学习生活中去。海豚调度在这里祝福大家虎年大吉,万事顺利。
-
-在这早春时节, Apache DolphinScheduler 于 2022 年的第一场 Meetup 也是即将到来,相信社区里的小伙伴早就已经翘首以盼了吧!
-
-在此次的Meetup中,四位来自不同公司的嘉宾将会为我们讲述他们在使用 Apache DolphinScheduler 时的心得体会,相信不论你是 Apache Dolphin Scheduler 的使用者抑或是正在观望的朋友都能从中收获到许多。
-
-我们相信未来将会有更多的小伙伴加入我们,也会有越来越多的使用者互相分享实践经验使用和体会, Apache DolphinScheduler 的未来离不开大家的贡献与参与,愿我们一起越变越好。
-
-此次 Apache DolphinScheduler 2月首秀将于2月26日下午14:00准时开播,大家别忘了扫码预约哦~
-
-<div align=center>
-<img src="/img/2022-02-26/09.png"/>
-</div>
-
-
-扫码预约直播
-
-小伙伴们也可以点击 https://www.slidestalk.com/m/679 直达直播预约界面!
-
-
-## 1 活动简介
-
-
-主题:Apache DolphinScheduler 用户实践分享
-时间:2022年2月26日 14:00-17:00
-形式:线上直播
-
-## 02 活动亮点
-
-
-此次 Apache DolphinScheduler 的活动我们将迎来四位分享嘉宾,他们都是来自于360数科、杭州思科以及途家的大咖,相信他们对于 Apache DolphinScheduler的实际体验将会有高度的代表性以及典型性。他们与我们分享的实践经验包含但不限于在 K8S 集群上的探索与应用以及对Alert 模块的改造等,对于解决大家在实际使用中遇到的困难具有很大的意义,希望大家都可以从中得到独属于自己的宝贵财富。
-
-## 03 活动议程
-
-<div align=center>
-<img style="width: 60%;" src="/img/2022-02-26/02.png"/>
-</div>
-
-## 04 议题介绍
-
-<div align=center>
-<img style="width: 300px;height: 300px;margin: 40px 0;" src="/img/2022-02-26/03.png"/>
-</div>
-
-
-刘建敏/360数科/大数据工程师
-
-演讲主题:Apache DolphinScheduler 在360数科的实践
-演讲概要:大数据调度从 Azkaban 到 Apache DolphinScheduler 的演变, Apache DolphinScheduler 的使用与改造。
-
-<div align=center>
-<img style="width: 300px;height: 300px;margin: 40px 0;" src="/img/2022-02-26/04.jpg"/>
-</div>
-
-
-李庆旺/杭州思科/大数据工程师
-
-演讲主题:Apache DolphinScheduler Alert 模块的改造
-演讲概要:切换到 Apache DolphinScheduler 探索,针对于不同的告警场景,对Apache DolphinScheduler 的 Alert 模块进行一些改造和探索。
-
-
-<div align=center>
-<img style="width: 300px;height: 300px;margin: 40px 0;" src="/img/2022-02-26/05.png"/>
-</div>
-
-
-昝绪超/途家/大数据工程师
-
-演讲主题:Apache DolphinScheduler 的探索与应用
-演讲概要:引入 Apache DolphinScheduler ,搭建了途家数据调度系统,完成数据服务的接入,具体包括离线表,邮件,以及数据同步等。详细的介绍了我们对调度系统做了一些功能的开发。
-
-<div align=center>
-<img style="width: 300px;height: 300px;margin: 40px 0;" src="/img/2022-02-26/06.png"/>
-</div>
-
-刘千/杭州思科/大数据工程师
-
-演讲主题:Apache DolphinScheduler 在 K8S 集群上的探索与应用
-演讲概要:切换到 Apache DolphinScheduler 探索,以及在基于 Apache DolphinScheduler二次开发支持任务提交在k8s上。目前可以运行镜像,spark , flink 等任务,以及日志监控报警的探索。
-
-Apache DolphinScheduler 的小伙伴们,我们2月26日下午14:00不见不散!
diff --git a/blog/zh-cn/Twos.md b/blog/zh-cn/Twos.md
deleted file mode 100644
index f91a9dd..0000000
--- a/blog/zh-cn/Twos.md
+++ /dev/null
@@ -1,44 +0,0 @@
-## 恭喜 Apache DolphinScheduler 入选可信开源社区共同体(TWOS)预备成员!
-
-<div align=center>
-<img src="https://s1.imgpp.com/2022/01/10/164179986575876800e959cabce5b.png"/>
-</div>
-
-近日,可信开源社区共同体正式宣布批准 6 位正式成员和 3 位预备成员加入。其中,云原生分布式大数据调度系统 Apache DolphinScheduler 入选,成为可信开源社区共同体预备成员。
-
-<div align=center>
-<img src="https://s1.imgpp.com/2022/01/10/559c8a77c69a15c7e91423b7306b2e37.png"/>
-</div>
-
-Apache DolphinScheduler 是一个分布式易扩展的新一代工作流调度平台,致力于“解决大数据任务之间错综复杂的依赖关系,使整个数据处理过程直观可见”,其强大的可视化 DAG 界面极大地提升了用户体验,配置工作流程无需复杂代码。
-
-自 2019 年 4 月正式对外开源以来,Apache DolphinScheduler 经过数代架构演进,迄今相关开源相关代码累积已获得 7100+ 个 Star,280+ 经验丰富的代码贡献者,110+ 非代码贡献者参与其中,其中也不乏其他 Apache 顶级项目的 PMC 或者 Committer。Apache DolphinScheduler 开源社区不断发展壮大,微信用户群已达 6000+ 人,600 + 家公司及机构已在生产环境中采用 Apache DolphinScheduler。
-
-## TWOS
-
-在“2021OSCAR 开源产业大会”上,中国信通院正式成立可信开源社区共同体(TWOS)。可信开源社区共同体由众多开源项目和开源社区组成,目的是引导建立健康可信且可持续发展的开源社区,旨在搭建交流平台,提供全套的开源风险监测与生态监测服务。
-
-<div align=center>
-<img src="https://s1.imgpp.com/2022/01/10/fae6b468615d4845f2d09d99061e0b6b.png"/>
-</div>
-
-为帮助企业降低开源软件的使用风险,推动建立可信开源生态,中国信通院建立了可信开源标准体系,对企业开源治理能力、开源项目合规性、开源社区成熟度、开源工具检测能力、商业产品开源风险管理能力开展测评。其中,对于开源社区的评估,是开源社区=人+项目+基础设施平台,一个好的开源社区有助于开源项目营造良好的开源生态并扩大影响力。可信开源社区评估从基础设施、社区治理、社区运营与社区开发等角度,梳理开源社区应关注的内容及指标,聚焦于如何构建活跃的开发者生态与可信的开源社区。
-
-经过可信开源社区共同体(TWOS)的重重评估标准的筛选,批准 Apache DolphinScheduler 入选预备成员,证明了其对 Apache DolphinScheduler 的开源运营方式、成熟度及贡献的认可,激励社区提升活跃度。
-
-<div align=center>
-<img src="https://s1.imgpp.com/2022/01/10/86ad880d0f0b069ae3ee5bd3f7265e4a.md.jpg"/>
-</div>
-
-
-可信开源标准体系 开源社区评估标准
-
-2021 年 9 月 17 日,可信开源社区共同体(TWOS)第一批成员加入。目前,可信开源社区共同体包括 openEuler、openGauss、MindSpore、openLookeng 等在内的 25 名正式成员,以及 Apache RocketMQ、Dcloud、Fluid、FastReID 等在内的 27 名预备成员,总数为 52 名:
-
-<div align=center>
-<img src="https://s1.imgpp.com/2022/01/10/9559811cd259449f03e32565a920c0cf.png"/>
-</div>
-
-第二批预备成员仅有两个项目——Apache DolphinScheduler 与阿里云开源云原生态分布式数据库 PolarDB 入选。
-
-Apache DolphinScheduler 社区十分荣幸能够入选可信开源社区共同体(TWOS)预备成员,这是整个行业对 Apache DolphinScheduler 社区建设的肯定与激励,社区将再接再厉,争取早日成为正式成员,与中国信息通信研究院领导的可信开源社区共同体一起,为中国开源生态建设提供更多价值!
diff --git a/blog/zh-cn/YouZan-case-study.md b/blog/zh-cn/YouZan-case-study.md
deleted file mode 100644
index 16546c2..0000000
--- a/blog/zh-cn/YouZan-case-study.md
+++ /dev/null
@@ -1,240 +0,0 @@
-
-## 从 Airflow 到 Apache DolphinScheduler,有赞大数据开发平台的调度系统演进
-
-编者按:在不久前的 Apache DolphinScheduler Meetup 2021 上,有赞大数据开发平台负责人 宋哲琦 带来了平台调度系统从 Airflow 迁移到 Apache DolphinScheduler 的方案设计思考和生产环境实践。
-
-这位来自浙江杭州的 90 后年轻人自 2019 年 9 月加入有赞,在这里从事数据开发平台、调度系统和数据同步组件的研发工作。刚入职时,有赞使用的还是同为 Apache 开源项目的 Airflow,但经过调研和生产环境测试,有赞决定切换到 DolphinScheduler。
-
-有赞大数据开发平台如何利用调度系统?为什么决定重新选型为 Apache DolphinScheduler ?让我们跟着他的分享来一探究竟。
-
-## 有赞大数据开发平台(DP平台)
-
-作为一家零售科技 SaaS 服务商,有赞的使命是助力线上商家开店,通过社交营销和拓展全渠道零售业务,搭建数据产品和数字化解决方案,为驱动商家数字增长提供更好的 SaaS 能力。
-
-目前,有赞在数据中台的支撑下已经建立了比较完整的数字产品矩阵:
-
-[](https://imgpp.com/image/ZbGED)
-
-为了支持日益增长的数据处理业务需求,有赞建立了大数据开发平台(以下简称 DP 平台)。这是一个大数据离线开发平台,提供用户大数据任务开发所需的环境、工具和数据。
-
-[](https://imgpp.com/image/ZbZbN)
-
-有赞大数据开发平台架构
-
-有赞大数据开发平台主要由基础组件层、任务组件层、调度层、服务层和监控层五个模块组成。其中,服务层主要负责作业的生命周期管理,基础组件层和任务组件层主要包括大数据开发平台依赖的中间件及大数据组件等基础环境。DP 平台的服务部署主要采用主从模式,Master 节点支持 HA。调度层是在 Airflow 的基础上进行二次开发,监控层对调度集群进行全方位监控和预警。
-
-### 1 调度层架构设计
-
-
-[](https://imgpp.com/image/ZbiQL)
-
-有赞大数据开发平台调度层架构设计
-
-2017 年,我们团队在17年的时候调研了当时的主流的调度系统,最终决定采用 Airflow(1.7)作为 DP 的任务调度模块。根据业务场景实际需求,架构设计方面,我们采用了Airflow + Celery + Redis + MySQL的部署方案,Redis 作为调度队列,通过 Celery 实现任意多台 worker 分布式部署。
-
-在调度节点 HA 设计上,众所周知,Airflow 在 schedule 节点上存在单点问题,为了实现调度的高可用,DP 平台采用了 Airflow Scheduler Failover Controller 这个开源组件,新增了一个 Standby 节点,会周期性地监听 Active 节点的健康情况,一旦发现 Active 节点不可用,则 Standby 切换为 Active,从而保证 schedule 的高可用。
-
-### 2 Worker节点负载均衡策略
-
-另外,由于不同任务占据资源不同,为了更有效地利用资源,DP 平台按照 CPU 密集/内存密集区分任务类型,并安排在不同的 celery 队列配置不同的 slot,保证每台机器 CPU/ 内存使用率保持在合理范围内。
-
-
-## 调度系统升级选型
-
-
-自 2017 年有赞大数据平台 1.0 版本正式上线以来,我们已经在 2018 年 100% 完成了数据仓库迁移的计划,2019 年日调度任务量达 30000+,到 2021 年,平台的日调度任务量已达到 60000+。伴随着任务量的剧增,DP 的调度系统也面临了许多挑战与问题。
-
-### 1 Airflow 的痛点
-
-1. 深度二次开发,脱离社区版本,升级成本高;
-2. Python 技术栈,维护迭代成本高;
-3. 性能问题:
-
-[](https://imgpp.com/image/Zb8yu)
-
-Airflow 的 schedule loop 如上图所示,本质上是对 DAG 的加载解析,将其生成 DAG round 实例执行任务调度。Airflow 2.0 之前的版本是单点 DAG 扫描解析到数据库,这就导致业务增长 Dag 数量较多时,scheduler loop 扫一次 Dag folder 会存在较大延迟(超过扫描频率),甚至扫描时间需要 60-70 秒,严重影响调度性能。
-
-4. 稳定性问题:
-
-Airflow Scheduler Failover Controller 本质还是一个主从模式,standby 节点通过监听 active进程是否存活来判断是否切换,如之前遇到 deadlock 阻塞进程的情况,则会被忽略,进而导致调度故障发生。在生产环境中发生过类似问题后,我们经过排查后发现了问题所在,虽然 Airflow 1.10 版本已经修复了这个问题,但在主从模式下,这个在生产环境下不可忽视的问题依然会存在。
-
-考虑到以上几个痛点问题,我们决定对 DP 平台的调度系统进行重新选型。
-
-在调研对比过程中,Apache DolphinScheduler 进入了我们的视野。同样作为 Apache 顶级开源调度组件项目,我们性能、部署、功能、稳定性和可用性、社区生态等角度对原调度系统和 DolphinScheduler 进行了综合对比。
-
-以下为对比分析结果:
-
-[](https://imgpp.com/image/ZbaBs)
-
-Airflow VS DolphinScheduler
-
-
-### 1 DolphinScheduler 价值评估
-
-[](https://imgpp.com/image/ZbVrt)
-
-如上图所示,经过对 DolphinScheduler 价值评估,我们发现其在相同的条件下,吞吐性能是原来的调度系统的 2 倍,而 2.0 版本后 DolphinScheduler 的性能还会有更大幅度的提升,这一点让我们非常兴奋。
-
-此外,在部署层面,DolphinScheduler 采用的 Java 技术栈有利于ops标准化部署流程,简化发布流程、解放运维人力,且支持Kubernetes、Docker 部署,扩展性更强。
-
-在功能新增上,因为我们在使用过程中比较注重任务依赖配置,而 DolphinScheduler 有更灵活的任务依赖配置,时间配置粒度细化到了时、天、周、月,使用体验更好。另外,DolphinScheduler 的调度管理界面易用性更好,同时支持 worker group 隔离。作为一个分布式调度,DolphinScheduler 整体调度能力随集群规模线性增长,而且随着新特性任务插件化的发布,可自定义任务类型这一点也非常吸引人。
-
-从稳定性与可用性上来说,DolphinScheduler 实现了高可靠与高可扩展性,去中心化的多 Master 多 Worker 设计架构,支持服务动态上下线,自我容错与调节能力更强。
-
-另外一个重要的点是,经过数月的接触,我们发现 DolphinScheduler 社区整体活跃度较高,经常会有技术交流,技术文档比较详细,版本迭代速度也较快。
-
-综上所述,我们决定转向 DolphinScheduler。
-
-
-## DolphinScheduler 接入方案设计
-
-
-决定接入 DolphinScheduler 之后,我们梳理了平台对于新调度系统的改造需求。
-
-总结起来,最重要的是要满足以下几点:
-
-1. 用户使用无感知,平台目前的用户数有 700-800,使用密度大,希望可以降低用户切换成本;
-2. 调度系统可动态切换,生产环境要求稳定性大于一切,上线期间采用线上灰度的形式,希望基于工作流粒度,实现调度系统动态切换;
-3. 测试与发布的工作流配置需隔离,目前任务测试和发布有两套配置文件通过 GitHub维护,线上调度任务配置需要保证数据整个确性和稳定性,需要两套环境进行隔离。
-
-针对以上三点,我们对架构进行了重新设计。
-
-### 1 架构设计
-
-1. 保留现有前端界面与DP API;
-2. 重构调度管理界面,原来是嵌入 Airflow 界面,后续将基于 DolphinScheduler 进行调度管理界面重构;
-3. 任务生命周期管理/调度管理等操作通过 DolphinScheduler API 交互;
-利用 Project 机制冗余工作流配置,实现测试、发布的配置隔离。
-
-[](https://imgpp.com/image/Zbsda)
-
-改造方案设计
-
-架构设计完成之后,进入改造阶段。我们对 DolphinScheduler 的工作流定义、任务执行流程、工作流发布流程都进行了改造,并进行了一些关键功能补齐。
-
-- 工作流定义状态梳理
-
-
-[](https://imgpp.com/image/Zbvzd)
-
-我们首先梳理了 DolphinScheduler 工作流的定义状态。因为 DolphinScheduler 工作的定义和定时管理会区分为上下线状态, 但 DP平台上两者的状态是统一的,因此在任务测试和工作流发布流程中,需要对 DP到DolphinScheduler 的流程串联做相应的改造。
-
-- 任务执行流程改造
-
-首先是任务测试流程改造。在切换到 DolphinScheduler 之后,所有的交互都是基于DolphinScheduler API 来进行的,当在 DP 启动任务测试时,会在 DolphinScheduler 侧生成对应的工作流定义配置,上线之后运行任务,同时调用 DolphinScheduler 的日志查看结果,实时获取日志运行信息。
-
-[](https://imgpp.com/image/Zb6Q0)
-
-- 工作流发布流程改造
-
-其次,针对工作流上线流程,切换到 DolphinScheduler 之后,主要是对工作流定义配置和定时配置,以及上线状态进行了同步。
-
-[](https://imgpp.com/image/Zbx2b)
-
-通过这两个核心流程的改造。工作流的原数据维护和配置同步其实都是基于 DP master来管理,只有在上线和任务运行时才会到调度系统进行交互,基于这点,DP 平台实现了工作流维度下的系统动态切换,以便于后续的线上灰度测试。
-
-### 2 功能补全
-
-此外,DP 平台还进行了一些功能补齐。首先是任务类型的适配。
-
-- 任务类型适配
-
-目前,DolphinScheduler 平台已支持的任务类型主要包含数据同步类和数据计算类任务,如Hive SQL 任务、DataX 任务、Spark 任务等。因为任务的原数据信息是在 DP 侧维护的,因此 DP 平台的对接方案是在 DP 的 master 构建任务配置映射模块,将 DP 维护的 task 信息映射到 DP 侧的 task,然后通过 DolphinScheduler 的 API 调用来实现任务配置信息传递。
-
-[](https://imgpp.com/image/Z8fNH)
-
-因为 DolphinScheduler 已经支持部分任务类型 ,所以只需要基于 DP 平台目前的实际使用场景对 DolphinScheduler 相应任务模块进行定制化改造。而对于 DolphinScheduler 未支持的任务类型,如Kylin任务、算法训练任务、DataY任务等,DP 平台也计划后续通过 DolphinScheduler 2.0 的插件化能力来补齐。
-
-### 3 改造进度
-
-因为 DP 平台上 SQL 任务和同步任务占据了任务总量的 80% 左右,因此改造重点都集中在这几个任务类型上,目前已基本完成 Hive SQL 任务、DataX 任务以及脚本任务的适配改造以及迁移工作。
-
-[](https://imgpp.com/image/Z8dgm)
-
-
-### 4 功能补齐
-
-- Catchup 机制实现调度自动回补
-
-DP 在实际生产环境中还需要一个核心能力,即基于 Catchup 的自动回补和全局补数能力。
-
-Catchup 机制在 DP 的使用场景,是在调度系统异常或资源不足,导致部分任务错过当前调度出发时间,当恢复调度后,会通过Catchup 自动补齐未被触发的调度执行计划。
-
-以下三张图是一个小时级的工作流调度执行的信息实例。
-
-在图 1 中,工作流在 6 点准时调起,每小时调一次,可以看到在 6 点任务准时调起并完成任务执行,当前状态也是正常调度状态。
-
-[](https://imgpp.com/image/Z8IkI)
- 图1
-
-图 2 显示在 6 点完成调度后,一直到 8 点期间,调度系统出现异常,导致 7 点和 8点该工作流未被调起。
-
-[](https://imgpp.com/image/Z8X64)
- 图2
-
-图 3 表示当 9 点恢复调度之后,因为 具有 Catchup 机制,调度系统会自动回补之前丢失的执行计划,实现调度的自动回补。
-
-[](https://imgpp.com/image/Z8tq8)
-图3
-
-此机制在任务量较大时作用尤为显著,当 Schedule 节点异常或核心任务堆积导致工作流错过调度出发时间时,因为系统本身的容错机制可以支持自动回补调度任务,所以无需人工手动补数重跑。
-
-同时,这个机制还应用在了 DP 的全局补数能力中。
-
-- 跨 Dag 全局补数
-
-[](https://imgpp.com/image/Z8BtU)
-DP 平台跨 Dag 全局补数流程
-
-全局补数在有赞的主要使用场景,是用在核心上游表产出中出现异常,导致下游商家展示数据异常时。这种情况下,一般都需要系统能够快速重跑整个数据链路下的所有任务实例。
-
-DP 平台目前是基于 Clear 的功能,通过原数据的血缘解析获取到指定节点和当前调度周期下的所有下游实例,再通过规则剪枝策略过滤部分无需重跑的实例。获取到这些实际列表之后,启动 clear down stream 的清除任务实例功能,再利用 Catchup 进行自动回补。
-
-这个流程实际上是通过 Clear 实现上游核心的全局重跑,自动补数的优势就在于可以解放人工操作。
-
-因为跨 Dag 全局补数能力在生产环境中是一个重要的能力,我们计划在 DolphinScheduler 中进行补齐。
-
-## 现状&规划&展望
-
-
-### 1 DolphinScheduler 接入现状
-
-DP 平台目前已经在测试环境中部署了部分 DolphinScheduler 服务,并迁移了部分工作流。
-
-对接到 DolphinScheduler API 系统后,DP 平台在用户层面统一使用 admin 用户,因为其用户体系是直接在 DP master 上进行维护,所有的工作流信息会区分测试环境和正式环境。
-
-[](https://imgpp.com/image/Zb7rA)
-DolphinScheduler 工作流定义列表
-
-[](https://imgpp.com/image/ZbQlk)
-
-[](https://imgpp.com/image/ZbodC)
-DolphinScheduler 2.0工作流任务节点展示
-
-DolphinScheduler 2.0 整体的 UI 交互看起来更加简洁,可视化程度更高,我们计划直接升级至 2.0 版本。
-
-### 2 接入规划
-
-目前 ,DP 平台还处于接入 DolphinScheduler 的灰度测试阶段,计划于今年 12 月进行工作流的全量迁移,同时会在测试环境进行分阶段全方位测试或调度性能测试和压力测试。确定没有任何问题后,我们会在来年 1 月进行生产环境灰度测试,并计划在 3 月完成全量迁移。
-
-[](https://imgpp.com/image/Zb0z6)
-
-
-### 3 对 DolphinScheduler 的期待
-
-
-未来,我们对 DolphinScheduler 最大的期待是希望 2.0 版本可以实现任务插件化。
-
-[](https://imgpp.com/image/Z8Oae)
-
-目前,DP 平台已经基于 DolphinScheduler 2.0实现了告警组件插件化,可在后端定义表单信息,并在前端自适应展示。
-
-“ 希望 DolphinScheduler 插件化的脚步可以更快一些,以更好地快速适配我们的定制化任务类型。
-——有赞大数据开发平台负责人 宋哲琦”
-
-
-
-
-
-
-
diff --git a/blog/zh-cn/about_blocking_task.md b/blog/zh-cn/about_blocking_task.md
deleted file mode 100644
index dc0e55f..0000000
--- a/blog/zh-cn/about_blocking_task.md
+++ /dev/null
@@ -1,155 +0,0 @@
-# 关于阻断类任务
-
-## 背景
-
-在工作流的执行中,有时我们并不希望从头一直执行到底,我们希望在工作流中间能够设立一些“检查站”,检查上游任务的执行情况,如果检查满足预期,则继续往下执行;如果检查不通过,就需要暂停当前工作流,告警相关人员进行干预,干预成功后再往下执行。**我们把这些“检查站”的职能称为阻断类任务,而把“检查站”称为阻断节点**。
-
-## 功能展示
-
-阻断类任务的功能设置大致是这样的:用户往DAG中添加阻断节点后,可以在面板设置阻断时机、是否阻断告警、阻断逻辑。当实际结果满足阻断条件时,工作流就会暂停执行,这时需要进行人工干预;否则,工作流就继续执行。
-
-下面通过一个简单的场景来展示阻断类任务的功能。
-
-假设我们有一个如下图所示的工作流
-
-
-
-处在工作流正中的`blocking-01-01`节点即为阻断节点,假设我是这么设置阻断节点的
-
-
-
-我设置的条件是:当阻断任务的前置任务满足阻断条件时,对工作流进行阻断操作,并且当发生阻断时,不进行告警。
-
-现在,我们执行这个工作流,可以发现,工作流在阻断节点处暂停了
-
-
-
-
-
-此时,用户可以选择重跑或继续执行该工作流。如果设置了阻断告警,相关管理人员还会收到通知邮件。
-
-## 技术方案选择
-
-当时主要提出了两种方案:
-
-- 基于现有的节点,赋予其阻断功能
-- 新增一类节点,专门用于阻断任务
-
-两种方案有利有弊,但是可以从以下两个方面进行抉择:
-
-- 功能性方面(基础功能、长远打算)
-- 成本方面(用户成本、开发者成本)
-
-综合考虑,我们选择了**第二种方案**,即新增一类节点,专门用于阻断类任务。
-
-下面我就从上述两个方面,介绍一下为什么第二种方案更好
-
-### 功能性方面
-
-#### 基础功能
-
-衡量一个方案,最最基本的,我认为是功能要达到我们的预期。阻断功能的目标是:在工作流执行过程中,如果遇到阻断任务,且满足用户设定的阻断条件,那么**工作流就暂停执行**,通知用户干预;如果不满足阻断条件,就继续执行。
-
-很显然,**两种方案都能够达到这个基本目标**。但是,对于第二种方案来说,第一种方案能做的,它可以做,且可以做得更好!试考虑以下场景:
-
-> 一天老板提了一个需求,要求我在工作流的中设置一个阻断任务,它的上游有3个分支,对应3个独立的子流程,这个阻断任务需要检查上游3个任务的运行情况,其中任意两个任务检查通过就可以继续执行(后续是单分支的),否则需要对工作流进行暂停。
-
-如果使用方案一,我必然要使用条件节点,但是目前DS中的条件节点,成功分支和失败分支都需要设置,且它们必须不一样,这样DAG才可以保存。我为了实现这个阻断任务,**我必须建立一个无用的分支**(例如使用Shell输出一个hello world)来满足需求。你可能会考虑修改条件节点,让其只有一个分支的情况下才能保存,但是我认为这么做**破坏了条件节点进行分支流转的功能**。
-
-如果使用方案二,一切就变得简单了,对于这个新增节点(阻断节点),我们就没有这个限制了,这个节点具有逻辑判断功能,我既可以让阻断节点支持后继多分支,也可以支持单分支,**即不会破坏现有节点的功能,又完成了我们的阻断目标**。
-
-#### 长远打算
-
-从长远来看,第一种方案,相当于把阻断任务当作了节点的属性进行**捆绑**。假如有一天Apache又发布了一款大数据组件,DS想要支持,在新节点前端编写的时候,依然需要增设阻断的选项。但是使用方案二,就不会存在捆绑问题,一劳永逸,且节点之间各司其职。
-
-### 成本方面
-
-#### 用户成本
-
-用户成本的话,老实说,两个方案用户成本都差不多。用户都要认识什么是阻断任务,如何去设置阻断任务。
-
-#### 开发成本
-
-老实说,预估第二种方案的代码量会比第一种多一些,**但是我觉得这是值得的**。因为第二种方案相比第一种更加灵活,能够更好地完成阻断任务。就好像苹果推出了iPad air 4和iPad Pro 2021,iPad air 4 256GB售价749美元, iPad Pro 2021 11英寸128GB售价799美元,二者相差50$,**但是多加这些钱可以在满足日常需求的情况下,享受到iPad的极致体验,为什么不多付出这些代价呢**?阻断任务也是一样的。
-
-综上,我更推荐第二种方案。
-
-## 技术方案概述
-
-> 本部分只会涉及阻断类任务的**代码主要设计思路**,不会涉及具体的代码,但是文后会给出代码实例的Github。因为ds架构一直在发生变化,在这里讨论具体的代码怎么写没有太大意义。
-
-### 概念
-
-为了方便后文阐述,这里先说明几个概念:
-
-- 阻断节点本身的状态:阻断节点的执行状态,成功 or 取消 or 暂停等
-- 阻断逻辑的状态:阻断节点的前置任务运行结果是否满足用户期望的阻断条件
-
-不难看出,阻断节点本身包含了两种状态。
-
-### 流程图
-
-带阻断类任务的工作流,执行流程图如下
-
-
-
-整个工作流可以以阻断节点为界,分成上游与下游两个部分。首先,先执行上游工作流,当执行到阻断节点时,阻断节点会先判断其前置任务的执行状态是否满足用户设置的预期阻断条件,如果不满足阻断条件,工作流就继续往下执行;如果满足阻断条件,会暂停工作流的执行,并判断用户是否设置阻断告警,如果没有设置,流程结束,否则会调用告警组通知相关人员干预。
-
-### 代码主要设计思路
-
-#### JSON定义
-
-阻断节点的JSON定义是与条件节点类似的,区别在于阻断节点有自己本身的参数,在每个节点的JSON定义中,有一个`params`字段,阻断节点的参数就定义在这里。对于阻断节点,有以下自定义参数:
-
-- `blockingCondition`:阻断时机,取值:BlockingOnSuccess/BlockingOnFailed (阻断逻辑为真阻断/阻断逻辑为假阻断)
-- `alertWhenBlocking`:是否阻断时告警,取值:true/false
-
-```json
- "params": {
- "blockingCondition":"BlockingOnFailed",
- "alertWhenBlocking":true
- }
-```
-
-#### 阻断节点的工作线程类
-
-阻断节点属于ds中“没有实际任务”的节点,条件节点、subProcess节点都是这一类节点。这一类节点都有自己的工作线程类,阻断节点也不例外,也需要一个自己的线程类。
-
-在这个线程类中,主要进行以下操作:
-
-- 获取到阻断节点的参数(需要自己在外部定义一个阻断节点参数类用于映射阻断节点自己的参数)
-- 获取阻断节点前置任务的执行结果
-- 判断前置任务的执行结果,是否满足阻断节点参数中用户设置的阻断条件(阻断逻辑状态)
-- 向工作流调度线程返回阻断节点本身的状态和阻断逻辑状态
-
-#### 阻断节点告警类
-
-这个类比较简单,只需要根据`ProcessAlertContent`模版设置好内容存库就可以了。后续ds的告警插件会自动从数据库查表并发送告警,这一过程不需要我们干预。
-
-#### 工作流调度线程类
-
-工作流调度线程是整个工作流执行的核心,由于阻断节点的存在势必会干预工作流调度,因此这个类是需要做出改变的。
-
-在原有的工作流调度线程中,主要需要进行以下修改:
-
-分两种大情况讨论
-
-- 如果当前任务不是阻断任务
- - 当前任务执行失败:判断其**直接后继**是否是阻断节点,如果是,继续提交后继任务,不走失败策略
- - 其他状态:不用修改代码
-
-- 如果当前任务是阻断任务
- - 当前任务执行成功:拿到阻断节点工作线程类传给调度线程的阻断逻辑状态,然后看阻断逻辑的状态是`true`还是`false`。如果是`false`,什么也不做;如果是`true`,将当前工作流的状态设置为`READY_PAUSE`,并且阻止后续任何任务提交。
- - 其他状态:不用修改代码
-
-> * 阻断节点经过分析,不太可能存在失败状态。阻断节点失败有两种可能,一种是DB宕机,另一种是Master宕机。如果是前者,失败的状态不会被写入数据库,此时整个ds也就瘫痪了;如果是后者,会启动容错策略,此时的任务状态不是失败,而是需要容错。
-> * 当发现满足阻断条件后,阻止后续任何任务提交的原因是:现有的ds架构中,都会维持一个节点提交队列,当这个队列为空时,工作流也就执行结束了,因此,阻止后续任务提交,肯定会在某一个时刻,使这个队列为空,因此工作流肯定可以暂停并停下来。
-
-### 代码示例
-
-- [1.x架构代码示例](https://github.com/mgsky1/dolphinscheduler/tree/add-blocking-task-dev)
-- [2.0架构代码示例](https://github.com/mgsky1/dolphinscheduler/tree/add-blocking-task-dev-new)
-
-## 总结
-
-这个项目的难点并不在coding阶段,而是在方案设计阶段。对于一个idea,往往会有多种方案,如何选择一个合适的方案是十分重要的。例如在这个项目中,面临着在现有节点上修改和新增节点两种方案,它们对应着不同的设计方向。我觉得在遇到这种情况时,需要与社区进行充分讨论,使用一种baseline来衡量每一种方案的利弊,最终做出合适的选择。
\ No newline at end of file
diff --git a/blog/zh-cn/architecture-design.md b/blog/zh-cn/architecture-design.md
deleted file mode 100644
index a9ca539..0000000
--- a/blog/zh-cn/architecture-design.md
+++ /dev/null
@@ -1,304 +0,0 @@
-## 系统架构设计
-在对调度系统架构说明之前,我们先来认识一下调度系统常用的名词
-
-### 1.名词解释
-**DAG:** 全称Directed Acyclic Graph,简称DAG。工作流中的Task任务以有向无环图的形式组装起来,从入度为零的节点进行拓扑遍历,直到无后继节点为止。举例如下图:
-
-<p align="center">
- <img src="/img/dag_examples_cn.jpg" alt="dag示例" width="60%" />
- <p align="center">
- <em>dag示例</em>
- </p>
-</p>
-
-**流程定义**:通过拖拽任务节点并建立任务节点的关联所形成的可视化**DAG**
-
-**流程实例**:流程实例是流程定义的实例化,可以通过手动启动或定时调度生成,流程定义每运行一次,产生一个流程实例
-
-**任务实例**:任务实例是流程定义中任务节点的实例化,标识着具体的任务执行状态
-
-**任务类型**: 目前支持有SHELL、SQL、SUB_PROCESS(子流程)、PROCEDURE、MR、SPARK、PYTHON、DEPENDENT(依赖),同时计划支持动态插件扩展,注意:其中子 **SUB_PROCESS** 也是一个单独的流程定义,是可以单独启动执行的
-
-**调度方式:** 系统支持基于cron表达式的定时调度和手动调度。命令类型支持:启动工作流、从当前节点开始执行、恢复被容错的工作流、恢复暂停流程、从失败节点开始执行、补数、定时、重跑、暂停、停止、恢复等待线程。其中 **恢复被容错的工作流** 和 **恢复等待线程** 两种命令类型是由调度内部控制使用,外部无法调用
-
-**定时调度**:系统采用 **quartz** 分布式调度器,并同时支持cron表达式可视化的生成
-
-**依赖**:系统不单单支持 **DAG** 简单的前驱和后继节点之间的依赖,同时还提供**任务依赖**节点,支持**流程间的自定义任务依赖**
-
-**优先级** :支持流程实例和任务实例的优先级,如果流程实例和任务实例的优先级不设置,则默认是先进先出
-
-**邮件告警**:支持 **SQL任务** 查询结果邮件发送,流程实例运行结果邮件告警及容错告警通知
-
-**失败策略**:对于并行运行的任务,如果有任务失败,提供两种失败策略处理方式,**继续**是指不管并行运行任务的状态,直到流程失败结束。**结束**是指一旦发现失败任务,则同时Kill掉正在运行的并行任务,流程失败结束
-
-**补数**:补历史数据,支持**区间并行和串行**两种补数方式
-
-### 2.系统架构
-
-#### 2.1 系统架构图
-<p align="center">
- <img src="/img/architecture.jpg" alt="系统架构图" width="70%" />
- <p align="center">
- <em>系统架构图</em>
- </p>
-</p>
-
-#### 2.2 架构说明
-
-* **MasterServer**
-
- MasterServer采用分布式无中心设计理念,MasterServer主要负责 DAG 任务切分、任务提交监控,并同时监听其它MasterServer和WorkerServer的健康状态。
- MasterServer服务启动时向Zookeeper注册临时节点,通过监听Zookeeper临时节点变化来进行容错处理。
-
- ##### 该服务内主要包含:
-
- - **Distributed Quartz**分布式调度组件,主要负责定时任务的启停操作,当quartz调起任务后,Master内部会有线程池具体负责处理任务的后续操作
-
- - **MasterSchedulerThread**是一个扫描线程,定时扫描数据库中的 **command** 表,根据不同的**命令类型**进行不同的业务操作
-
- - **MasterExecThread**主要是负责DAG任务切分、任务提交监控、各种不同命令类型的逻辑处理
-
- - **MasterTaskExecThread**主要负责任务的持久化
-
-* **WorkerServer**
-
- WorkerServer也采用分布式无中心设计理念,WorkerServer主要负责任务的执行和提供日志服务。WorkerServer服务启动时向Zookeeper注册临时节点,并维持心跳。
- ##### 该服务包含:
- - **FetchTaskThread**主要负责不断从**Task Queue**中领取任务,并根据不同任务类型调用**TaskScheduleThread**对应执行器。
-
- - **LoggerServer**是一个RPC服务,提供日志分片查看、刷新和下载等功能
-
-* **ZooKeeper**
-
- ZooKeeper服务,系统中的MasterServer和WorkerServer节点都通过ZooKeeper来进行集群管理和容错。另外系统还基于ZooKeeper进行事件监听和分布式锁。
- 我们也曾经基于Redis实现过队列,不过我们希望EasyScheduler依赖到的组件尽量地少,所以最后还是去掉了Redis实现。
-
-* **Task Queue**
-
- 提供任务队列的操作,目前队列也是基于Zookeeper来实现。由于队列中存的信息较少,不必担心队列里数据过多的情况,实际上我们压测过百万级数据存队列,对系统稳定性和性能没影响。
-
-* **Alert**
-
- 提供告警相关接口,接口主要包括**告警**两种类型的告警数据的存储、查询和通知功能。其中通知功能又有**邮件通知**和**SNMP(暂未实现)**两种。
-
-* **API**
-
- API接口层,主要负责处理前端UI层的请求。该服务统一提供RESTful api向外部提供请求服务。
- 接口包括工作流的创建、定义、查询、修改、发布、下线、手工启动、停止、暂停、恢复、从该节点开始执行等等。
-
-* **UI**
-
- 系统的前端页面,提供系统的各种可视化操作界面,详见**[功能介绍]**部分。
-
-#### 2.3 架构设计思想
-
-##### 一、去中心化vs中心化
-
-###### 中心化思想
-
-中心化的设计理念比较简单,分布式集群中的节点按照角色分工,大体上分为两种角色:
-<p align="center">
- <img src="https://analysys.github.io/easyscheduler_docs_cn/images/master_slave.png" alt="master-slave角色" width="50%" />
- </p>
-
-- Master的角色主要负责任务分发并监督Slave的健康状态,可以动态的将任务均衡到Slave上,以致Slave节点不至于“忙死”或”闲死”的状态。
-- Worker的角色主要负责任务的执行工作并维护和Master的心跳,以便Master可以分配任务给Slave。
-
-
-
-中心化思想设计存在的问题:
-
-- 一旦Master出现了问题,则群龙无首,整个集群就会崩溃。为了解决这个问题,大多数Master/Slave架构模式都采用了主备Master的设计方案,可以是热备或者冷备,也可以是自动切换或手动切换,而且越来越多的新系统都开始具备自动选举切换Master的能力,以提升系统的可用性。
-- 另外一个问题是如果Scheduler在Master上,虽然可以支持一个DAG中不同的任务运行在不同的机器上,但是会产生Master的过负载。如果Scheduler在Slave上,则一个DAG中所有的任务都只能在某一台机器上进行作业提交,则并行任务比较多的时候,Slave的压力可能会比较大。
-
-
-
-###### 去中心化
- <p align="center">
- <img src="https://analysys.github.io/easyscheduler_docs_cn/images/decentralization.png" alt="去中心化" width="50%" />
- </p>
-
-- 在去中心化设计里,通常没有Master/Slave的概念,所有的角色都是一样的,地位是平等的,全球互联网就是一个典型的去中心化的分布式系统,联网的任意节点设备down机,都只会影响很小范围的功能。
-- 去中心化设计的核心设计在于整个分布式系统中不存在一个区别于其他节点的”管理者”,因此不存在单点故障问题。但由于不存在” 管理者”节点所以每个节点都需要跟其他节点通信才得到必须要的机器信息,而分布式系统通信的不可靠行,则大大增加了上述功能的实现难度。
-- 实际上,真正去中心化的分布式系统并不多见。反而动态中心化分布式系统正在不断涌出。在这种架构下,集群中的管理者是被动态选择出来的,而不是预置的,并且集群在发生故障的时候,集群的节点会自发的举行"会议"来选举新的"管理者"去主持工作。最典型的案例就是ZooKeeper及Go语言实现的Etcd。
-
-
-
-- EasyScheduler的去中心化是Master/Worker注册到Zookeeper中,实现Master集群和Worker集群无中心,并使用Zookeeper分布式锁来选举其中的一台Master或Worker为“管理者”来执行任务。
-
-##### 二、分布式锁实践
-
-EasyScheduler使用ZooKeeper分布式锁来实现同一时刻只有一台Master执行Scheduler,或者只有一台Worker执行任务的提交。
-1. 获取分布式锁的核心流程算法如下
- <p align="center">
- <img src="https://analysys.github.io/easyscheduler_docs_cn/images/distributed_lock.png" alt="获取分布式锁流程" width="50%" />
- </p>
-
-2. EasyScheduler中Scheduler线程分布式锁实现流程图:
- <p align="center">
- <img src="https://analysys.github.io/easyscheduler_docs_cn/images/distributed_lock_procss.png" alt="获取分布式锁流程" width="50%" />
- </p>
-
-
-##### 三、线程不足循环等待问题
-
-- 如果一个DAG中没有子流程,则如果Command中的数据条数大于线程池设置的阈值,则直接流程等待或失败。
-- 如果一个大的DAG中嵌套了很多子流程,如下图则会产生“死等”状态:
-
- <p align="center">
- <img src="https://analysys.github.io/easyscheduler_docs_cn/images/lack_thread.png" alt="线程不足循环等待问题" width="50%" />
- </p>
-上图中MainFlowThread等待SubFlowThread1结束,SubFlowThread1等待SubFlowThread2结束, SubFlowThread2等待SubFlowThread3结束,而SubFlowThread3等待线程池有新线程,则整个DAG流程不能结束,从而其中的线程也不能释放。这样就形成的子父流程循环等待的状态。此时除非启动新的Master来增加线程来打破这样的”僵局”,否则调度集群将不能再使用。
-
-对于启动新Master来打破僵局,似乎有点差强人意,于是我们提出了以下三种方案来降低这种风险:
-
-1. 计算所有Master的线程总和,然后对每一个DAG需要计算其需要的线程数,也就是在DAG流程执行之前做预计算。因为是多Master线程池,所以总线程数不太可能实时获取。
-2. 对单Master线程池进行判断,如果线程池已经满了,则让线程直接失败。
-3. 增加一种资源不足的Command类型,如果线程池不足,则将主流程挂起。这样线程池就有了新的线程,可以让资源不足挂起的流程重新唤醒执行。
-
-注意:Master Scheduler线程在获取Command的时候是FIFO的方式执行的。
-
-于是我们选择了第三种方式来解决线程不足的问题。
-
-
-##### 四、容错设计
-容错分为服务宕机容错和任务重试,服务宕机容错又分为Master容错和Worker容错两种情况
-
-###### 1. 宕机容错
-
-服务容错设计依赖于ZooKeeper的Watcher机制,实现原理如图:
-
- <p align="center">
- <img src="https://analysys.github.io/easyscheduler_docs_cn/images/fault-tolerant.png" alt="EasyScheduler容错设计" width="40%" />
- </p>
-其中Master监控其他Master和Worker的目录,如果监听到remove事件,则会根据具体的业务逻辑进行流程实例容错或者任务实例容错。
-
-
-
-- Master容错流程图:
-
- <p align="center">
- <img src="https://analysys.github.io/easyscheduler_docs_cn/images/fault-tolerant_master.png" alt="Master容错流程图" width="40%" />
- </p>
-ZooKeeper Master容错完成之后则重新由EasyScheduler中Scheduler线程调度,遍历 DAG 找到”正在运行”和“提交成功”的任务,对”正在运行”的任务监控其任务实例的状态,对”提交成功”的任务需要判断Task Queue中是否已经存在,如果存在则同样监控任务实例的状态,如果不存在则重新提交任务实例。
-
-
-
-- Worker容错流程图:
-
- <p align="center">
- <img src="https://analysys.github.io/easyscheduler_docs_cn/images/fault-tolerant_worker.png" alt="Worker容错流程图" width="40%" />
- </p>
-
-Master Scheduler线程一旦发现任务实例为” 需要容错”状态,则接管任务并进行重新提交。
-
- 注意:由于” 网络抖动”可能会使得节点短时间内失去和ZooKeeper的心跳,从而发生节点的remove事件。对于这种情况,我们使用最简单的方式,那就是节点一旦和ZooKeeper发生超时连接,则直接将Master或Worker服务停掉。
-
-###### 2.任务失败重试
-
-这里首先要区分任务失败重试、流程失败恢复、流程失败重跑的概念:
-
-- 任务失败重试是任务级别的,是调度系统自动进行的,比如一个Shell任务设置重试次数为3次,那么在Shell任务运行失败后会自己再最多尝试运行3次
-- 流程失败恢复是流程级别的,是手动进行的,恢复是从只能**从失败的节点开始执行**或**从当前节点开始执行**
-- 流程失败重跑也是流程级别的,是手动进行的,重跑是从开始节点进行
-
-
-
-接下来说正题,我们将工作流中的任务节点分了两种类型。
-
-- 一种是业务节点,这种节点都对应一个实际的脚本或者处理语句,比如Shell节点,MR节点、Spark节点、依赖节点等。
-
-- 还有一种是逻辑节点,这种节点不做实际的脚本或语句处理,只是整个流程流转的逻辑处理,比如子流程节等。
-
-每一个**业务节点**都可以配置失败重试的次数,当该任务节点失败,会自动重试,直到成功或者超过配置的重试次数。**逻辑节点**不支持失败重试。但是逻辑节点里的任务支持重试。
-
-如果工作流中有任务失败达到最大重试次数,工作流就会失败停止,失败的工作流可以手动进行重跑操作或者流程恢复操作
-
-
-
-##### 五、任务优先级设计
-在早期调度设计中,如果没有优先级设计,采用公平调度设计的话,会遇到先行提交的任务可能会和后继提交的任务同时完成的情况,而不能做到设置流程或者任务的优先级,因此我们对此进行了重新设计,目前我们设计如下:
-
-- 按照**不同流程实例优先级**优先于**同一个流程实例优先级**优先于**同一流程内任务优先级**优先于**同一流程内任务**提交顺序依次从高到低进行任务处理。
- - 具体实现是根据任务实例的json解析优先级,然后把**流程实例优先级_流程实例id_任务优先级_任务id**信息保存在ZooKeeper任务队列中,当从任务队列获取的时候,通过字符串比较即可得出最需要优先执行的任务
-
- - 其中流程定义的优先级是考虑到有些流程需要先于其他流程进行处理,这个可以在流程启动或者定时启动时配置,共有5级,依次为HIGHEST、HIGH、MEDIUM、LOW、LOWEST。如下图
- <p align="center">
- <img src="https://analysys.github.io/easyscheduler_docs_cn/images/process_priority.png" alt="流程优先级配置" width="40%" />
- </p>
-
- - 任务的优先级也分为5级,依次为HIGHEST、HIGH、MEDIUM、LOW、LOWEST。如下图
- <p align="center">
- <img src="https://analysys.github.io/easyscheduler_docs_cn/images/task_priority.png" alt="任务优先级配置" width="35%" />
- </p>
-
-
-##### 六、Logback和gRPC实现日志访问
-
-- 由于Web(UI)和Worker不一定在同一台机器上,所以查看日志不能像查询本地文件那样。有两种方案:
- - 将日志放到ES搜索引擎上
- - 通过gRPC通信获取远程日志信息
-
-- 介于考虑到尽可能的EasyScheduler的轻量级性,所以选择了gRPC实现远程访问日志信息。
-
- <p align="center">
- <img src="https://analysys.github.io/easyscheduler_docs_cn/images/grpc.png" alt="grpc远程访问" width="50%" />
- </p>
-
-
-- 我们使用自定义Logback的FileAppender和Filter功能,实现每个任务实例生成一个日志文件。
-- FileAppender主要实现如下:
-
- ```java
- /**
- * task log appender
- */
- public class TaskLogAppender extends FileAppender<ILoggingEvent> {
-
- ...
-
- @Override
- protected void append(ILoggingEvent event) {
-
- if (currentlyActiveFile == null){
- currentlyActiveFile = getFile();
- }
- String activeFile = currentlyActiveFile;
- // thread name: taskThreadName-processDefineId_processInstanceId_taskInstanceId
- String threadName = event.getThreadName();
- String[] threadNameArr = threadName.split("-");
- // logId = processDefineId_processInstanceId_taskInstanceId
- String logId = threadNameArr[1];
- ...
- super.subAppend(event);
- }
-}
- ```
-
-
-以/流程定义id/流程实例id/任务实例id.log的形式生成日志
-
-- 过滤匹配以TaskLogInfo开始的线程名称:
-
-- TaskLogFilter实现如下:
-
- ```java
- /**
- * task log filter
- */
-public class TaskLogFilter extends Filter<ILoggingEvent> {
-
- @Override
- public FilterReply decide(ILoggingEvent event) {
- if (event.getThreadName().startsWith("TaskLogInfo-")){
- return FilterReply.ACCEPT;
- }
- return FilterReply.DENY;
- }
-}
- ```
-
-### 总结
-本文从调度出发,初步介绍了大数据分布式工作流调度系统--EasyScheduler的架构原理及实现思路。未完待续
-
-
diff --git a/blog/zh-cn/cicd_workflow.md b/blog/zh-cn/cicd_workflow.md
deleted file mode 100644
index a571f71..0000000
--- a/blog/zh-cn/cicd_workflow.md
+++ /dev/null
@@ -1,287 +0,0 @@
-# 用DolphinScheduler建立java项目的CI/CD工作流
-
-
-### CI/CD 流程介绍
-
-CI/CD中包含一系列步骤,来自动化我们开发阶段的软件交付过程,使其可以频繁地输送准确的代码。CI/CD 的核心概念是持续集成、持续交付和持续部署。作为一个面向开发和运营团队的解决方案,CI/CD 主要针对在集成新代码时所引发的问题。
-
-这里的CI 指持续集成,这个过程是自动化的,以确保团队能够以可靠和可重复的方式构建、测试和打包他们的应用程序。 CI 有助于简化代码更改流程,从而增加开发人员进行更改和改进软件的时间。
-
-CD 指持续交付/持续部署。持续交付代表将完成的代码自动交付到测试和开发等环境中。 CD 为将代码交付到这些环境提供了一种自动化且一致的方式。持续部署是持续交付的下一步。通过自动化测试的每个更改都会自动投入生产环境中,从而在生产环境下部署。
-
-这个针对项目的CI/CD工作流主要有三个:打包(packaging),回滚(roll back),特性发布(feature release)。
-
-打包阶段的主要内容是检查本地代码库是否为最新版本,后进行构建,测试,部署到模拟环境中。若打包顺利完成,本地会产生一个新的版本,并且可以在模拟环境中检查此新版本。
-
-回滚的目的是显示已有的发布版本,调用其中一个选定的版本,将其部署到生产环境。特性发布的内容与回滚大体相似,但不同的是,在这个工作流中,显示已有的特性发布版本,再调用其中一个并部署到生产环境。
-
-### 基于 DS 实现 CI/CD pipeline 实例
-
-基于现有的ds功能,我们找到一个小型项目当作此次尝试的范例。被选中的项目[spring-boot-vuejs](https://github.com/jonashackt/spring-boot-vuejs) 具有前端和后端,可以较为直观的看到部署的结果,它的CI/CD流程也比较简明。这个实例中,我们采用的部署形式是本地部署/单机部署,不考虑到多台机器或者集群的影响。在为项目工作流配置完全局变量和本地变量后,便可以使其上线运行,完成的工作流如下。
-
-##### Packaging
-这个工作流共包含6个shell节点,用于CI/CD流程中的代码获取,构建,测试,打包,预发布(staging)。详见导出的[工作流文件](/img/cicd_workflow/feature_release.json)
-
-
-
-- 节点1: check repo
-这个节点的目的是检查本地是否已经有最新的代码版本,分别通过git clone和git pull获取代码库和更新。环节的开始和结束,均会在日志中有提醒。
-
-```sh
-# Check Java version, Maven version and Git Branch
-echo Show supporting info
-java -version
-mvn --version
-# choose a local directory to store this project
-# cd /opt/repo
-
-echo Start of repo checkout/update
-# Check if project's repo is under the chosen directory.
-# If available, pull the latest version, if not, clone
-DIR="${project_folder}"
-if [ -d "$DIR" ]; then
- ### Pull latest if $DIR exists ###
- echo "Pass: ${DIR} exist"
- cd $DIR
- git status
- git pull origin master
- echo spring-boot-vuejs
-else
- ### Clone if $DIR does NOT exist ###
- echo "Error: ${DIR} not found. Clone repo"
- git clone https://github.com/jonashackt/spring-boot-vuejs
- cd spring-boot-vuejs
-fi
-echo End of repo checkout/update
-```
-如果节点运行成功,本地已存在目标代码库,此时可以执行git pull (前提保证本地更新已经提交) 。
-
-
-
-- 节点2: build with java8
-这个节点基于项目使用java的maven框架,进行构建。因为此实例使用的java版本为8和16。以本地默认java版本java8执行命令。
-
-```sh
-pwd
-cd ${project_folder}
-
-JAVA_HOME=${java8_path}
-java -version
-echo $JAVA_HOME
-
-npm config set registry http://registry.npmjs.org
-mvn -B install --no-transfer-progress
-```
-如果节点运行成功,日志中会出现“BUILD SUCCESS”,显示构建完成并且成功。
-
-
-
-- 节点3: test with java8
-这个节点在同一个java版本下运行单元测试。
-
-```sh
-pwd
-cd ${project_folder}
-
-mvn -B verify --no-transfer-progress
-```
-
-如果节点运行成功,日志中会出现“BUILD SUCCESS”,显示测试成功。
-
-
-
-- 节点4: build with java16
-这个节点基于项目使用java的maven框架,进行构建。因为已经用java8进行了构建,所以这里更换为java16后执行构建命令。
-
-```sh
-pwd
-cd ${project_folder}
-
-java -version
-echo $JAVA_HOME
-
-JAVA_HOME=${java16_path}
-export JAVA_HOME;
-echo $JAVA_HOME
-
-npm config set registry http://registry.npmjs.org
-mvn -B install --no-transfer-progress
-```
-
-如果节点运行成功,日志中会出现”BUILD SUCCESS“,显示构建成功。
-
-
-
-- 节点5: test with java16
-这个节点在同一个java版本下运行单元测试。
-
-```sh
-pwd
-cd ${project_folder}
-
-mvn -B verify --no-transfer-progress
-```
-如果节点运行成功,日志中会出现”BUILD SUCCESS“,显示测试成功。
-
-
-
-- 节点6: staging
-这个节点在项目预发布的模拟环境中部署,本地部署到端口 http://localhost:5000 。
-
-```sh
-pwd
-cd ${project_folder}
-
-JAVA_HOME=${java8_path}
-export JAVA_HOME;
-echo $JAVA_HOME
-
-echo START
-nohup java -Djava.security.egd=file:/dev/./urandom -jar ./backend/target/backend-0.0.3-SNAPSHOT.jar --server.port=${staging_port} > buildres_staging.txt 2>&1 &
-echo END
-```
-
-如果节点运行成功,因为使用nohup命令,部署至本地端口的命令在后台进行,日志中会出现“START”和“END”代表此命令的执行情况。日志显示如下
-
-
-
-同时,因为staging_port变量在此处被设置为 localhost:5000 ,所以在本地也可以看到部署完成的页面如下
-
-
-
-##### Rollback
-这个工作流共包含三个shell节点,用于完成项目版本回滚,也相当于CI/CD流程中的生产部署环节。详见导出的[工作流文件](/img/cicd_workflow/rollback.json)
-
-
-
-- 节点1: kill process on port
-这个节点用于关闭要部署的端口的现有进程,为节点3作准备。
-
-```sh
-pwd
-cd ${project_folder}
-
-if [[ $(lsof -ti:${port_number} | wc -l) -gt 0 ]];
- then
- echo process found
- sudo kill -9 $(lsof -ti:${port_number});
- fi
-```
-
-如果节点运行成功,要检查的端口的确有进程,日志中会显示如下图“process found”,之后这些进程会被关闭。
-
-
-
-- 节点2: display available version
-这个节点用于展示本地所有可以被调用的发布版本。假定所有的发布版本都遵循命名格式backend-X.0.0-RELEASE.jar,那么所有包含关键字“RELEASE ”的版本都会被筛选并显示出来,供下一步使用。
-
-```sh
-pwd
-cd ${project_folder}
-
-# see previous releases
-find /opt/repo/spring-boot-vuejs/backend/target -iname '*${keyword}*'
-```
-
-如果节点运行成功,本地所有发布版本都会被列举出来,以供选择调用。
-
-
-
-- 节点3: roll back
-这个节点用于选定回滚的目标版本,并且本地部署到端口。部署成功则可以在本地8098端口显示页面。
-
-```sh
-pwd
-cd ${project_folder}
-
-JAVA_HOME=${java8_path}
-export JAVA_HOME;
-echo $JAVA_HOME
-
-# fill in desired version to roll back to previous release
-echo START
-nohup java -Djava.security.egd=file:/dev/./urandom -jar ./backend/target/backend-1.0.0-RELEASE.jar --server.port=${port_number} > buildres_production.txt 2>&1 &
-echo END
-```
-如果节点运行成功,部署至本地端口的nohup命令在后台进行,日志中出现“START”和“END”代表此命令的执行情况。日志显示如下
-
-
-
-同时,因为port_number变量在此处被设置为localhost:8098,所以在本地也可以看到部署完成的页面如下
-
-
-
-##### Feature Release
-这个工作流共包含三个shell节点,用于完成项目版本回滚,也相当于CI/CD流程中的生产部署环节。详见导出的[工作流文件](/img/cicd_workflow/feature_release.json)
-
-
-
-- 节点1: kill process on port
-这个节点用于关闭要部署的端口的现有进程,为节点3作准备。
-
-```sh
-pwd
-cd ${project_folder}
-
-if [[ $(lsof -ti:${port_number} | wc -l) -gt 0 ]];
- then
- echo process found
- sudo kill -9 $(lsof -ti:${port_number});
- fi
-```
-
-如果要检查的端口的确有进程,日志中会显示如下图“process found”,之后这些进程会被关闭。
-
-
-
-- 节点2: display available version
-这个节点用于展示本地所有可以被调用的特性发布版本。假定所有的特性发布版本都遵循命名格式backend-0.0.1-SNAPSHOT.jar,那么所有包含关键字“SNAPSHOT”的版本都会被筛选并显示出来,供下一步使用。
-
-```sh
-pwd
-cd ${project_folder}
-
-# see previous releases
-find /opt/repo/spring-boot-vuejs/backend/target -iname '*${keyword}*'
-```
-
-如果节点运行成功,本地所有特性发布版本都会被列举出来,以供选择调用。
-
-
-
-- 节点3: release
-这个节点用于选定特性发布的目标版本,并且本地部署到端口。部署成功则可以在本地8098端口显示页面。
-
-```sh
-pwd
-cd ${project_folder}
-
-JAVA_HOME=${java8_path}
-export JAVA_HOME;
-echo $JAVA_HOME
-
-# fill in desired version to roll back to previous release
-echo START
-nohup java -Djava.security.egd=file:/dev/./urandom -jar ./backend/target/backend-0.0.3-SNAPSHOT.jar --server.port=${port_number} > buildres_production.txt 2>&1 &
-echo END
-```
-如果节点运行成功,部署至本地端口的nohup命令在后台进行,日志中出现“START”和“END”代表此命令的执行情况。日志显示如下
-
-
-
-同时,因为port_number变量在此处被设置为 localhost:8098,所以在本地也可以看到部署完成的页面如下
-
-
-
-### 使用DS构建工作流不足之处
-
-##### 项目中觉得使用ds来构建ci/cd 工作流不方便的地方:
-目前DS没有对于工作流或者节点的版本管理,例如不能给一个工作流的当前版本take snapshot,以便后续需要时使用。现在需要通过GitHub、Gitlab或者其他版本管理平台,才能完成例如将单个节点回滚到历史版本的任务。如果用户从DAG中删除一个节点或节点中的任务,并重新部署它,那么与该任务相关的元数据(metadata)就会失去。
-
-在单机部署时,工作流中所有节点并不处在同一个独立的虚拟环境里。每个节点都指向一个/tmp/dolphinscheduler/exec/process/下的不同路径,如果需要获取之前节点的信息,需要指定一个文件夹存储项目的源代码,每次进入文件夹来获取代码。但这也可以通过设置全局变量来解决。
-
-##### 与现有的CI/CD工具相比
-对于***本地部署***的ds来说,虚拟环境完全依赖本机的环境。例如在这次的工作流中,需要提前在本地设置好需要的java版本和maven 版本,虚拟环境中的相应命令才能执行。然而在Github Actions中,运行工作流的环境可以通过yaml文件内的设置,直接拉取对应的Docker image镜像而生成,独立于本地环境。
-
-与Github Actions相比,ds需要手动上线和启动CI/CD工作流,不能用类似on:[push] 这种GitHub event作为触发机制。
-
diff --git a/blog/zh-cn/dolphinscheduler_json.md b/blog/zh-cn/dolphinscheduler_json.md
deleted file mode 100644
index 98e7c8f..0000000
--- a/blog/zh-cn/dolphinscheduler_json.md
+++ /dev/null
@@ -1,799 +0,0 @@
-## dolphinscheduler json拆解
-
-## 1、为什么拆解json
-
-在dolphinscheduler 1.3.x及以前的工作流中的任务及任务关系保存时是以大json的方式保存到数据库中process_definiton表的process_definition_json字段,如果某个工作流很大比如有100或者1000个任务,这个json字段也就非常大,在使用时需要解析json,非常耗费性能,且任务没法重用;基于大json,在工作流版本及任务版本上也没有好的实现方案,否则会导致数据大量冗余。
-
-故社区计划启动json拆解项目,实现的需求目标:
-
-- 大json完全拆分
-- 新增工作流及任务版本
-- 引入全局唯一键(code)
-
-## 2、如何设计拆解后的表
-
-### 1、1.3.6版本工作流
-
-1、比如在当前1.3.6版本创建个a-->b的工作流
-
-
-
-以下是processDefiniton save 接口在controller入口打印的入参日志
-
-```
-create process definition, project name: hadoop, process definition name: ab, process_definition_json: {"globalParams":[],"tasks":[{"type":"SHELL","id":"tasks-77643","name":"a","params":{"resourceList":[],"localParams":[{"prop":"yesterday","direct":"IN","type":"VARCHAR","value":"${system.biz.date}"}],"rawScript":"echo ${yesterday}"},"description":"","timeout":{"strategy":"","interval":null,"enable":false},"runFlag":"NORMAL","conditionResult":{"successNode":[""],"failedNode":[""]},"depen [...]
-```
-
-2、依赖节点的工作流,dep是依赖节点
-
-
-
-
-
-以下是processDefiniton save 接口在controller入口打印的入参日志
-
-```
- create process definition, project name: hadoop, process definition name: dep_c, process_definition_json: {"globalParams":[],"tasks":[{"type":"SHELL","id":"tasks-69503","name":"c","params":{"resourceList":[],"localParams":[],"rawScript":"echo 11"},"description":"","timeout":{"strategy":"","interval":null,"enable":false},"runFlag":"NORMAL","conditionResult":{"successNode":[""],"failedNode":[""]},"dependence":{},"maxRetryTimes":"0","retryInterval":"1","taskInstancePriority":"MEDIUM","wor [...]
-```
-
-
-
-3、条件判断的工作流
-
-
-
-以下是processDefiniton save 接口在controller入口打印的入参日志
-
-```
-create process definition, project name: hadoop, process definition name: condition_test, process_definition_json: {"globalParams":[],"tasks":[{"type":"SHELL","id":"tasks-68456","name":"d","params":{"resourceList":[],"localParams":[],"rawScript":"echo 11"},"description":"","timeout":{"strategy":"","interval":null,"enable":false},"runFlag":"NORMAL","conditionResult":{"successNode":[""],"failedNode":[""]},"dependence":{},"maxRetryTimes":"0","retryInterval":"1","taskInstancePriority":"MEDI [...]
-```
-
-从以上三个案例中,我们知道controller的入口参数的每个参数都可以在t_ds_process_definition表中找到对应,故表中数据如下图
-
-
-
-### 2、拆解后的表设计思路
-
-工作流只是dag的展现形式,任务通过工作流进行组织,组织的同时存在了任务之间的关系,也就是依赖。就好比一个画板,画板上有些图案,工作流就是画板,图案就是任务,图案之间的关系就是依赖。而调度的核心是调度任务,依赖只是表述调度的先后顺序。当前定时还是对整个工作流进行的定时,拆解后就方便对单独任务进行调度。正是基于这个思想设计了拆解的思路,所以这就需要三张表,工作流定义表、任务定义表、任务关系表。
-
-- 工作流定义表:描述工作流的基本信息,比如全局参数、dag中节点的位置信息
-- 任务定义表:描述任务的详情信息,比如任务类别、任务容错信息、优先级等
-- 任务关系表:描述任务的关系信息,比如当前节点、前置节点等
-
-基于这个设计思想再扩展到版本,无非是对于这三张表,每张表新增个保存版本的日志表。
-
-#### 工作流定义表
-
-现在看案例中save接口日志,现有字段(project、process_definition_name、desc、locations、connects),对于json中除了task之外的还剩下
-
-```json
-{"globalParams":[],"tenantId":1,"timeout":0}
-```
-
-所以可知工作流定义表:
-
-```sql
-CREATE TABLE `t_ds_process_definition` (
- `id` int(11) NOT NULL AUTO_INCREMENT COMMENT 'self-increasing id',
- `code` bigint(20) NOT NULL COMMENT 'encoding',
- `name` varchar(200) DEFAULT NULL COMMENT 'process definition name',
- `version` int(11) DEFAULT NULL COMMENT 'process definition version',
- `description` text COMMENT 'description',
- `project_code` bigint(20) NOT NULL COMMENT 'project code',
- `release_state` tinyint(4) DEFAULT NULL COMMENT 'process definition release state:0:offline,1:online',
- `user_id` int(11) DEFAULT NULL COMMENT 'process definition creator id',
- `global_params` text COMMENT 'global parameters',
- `flag` tinyint(4) DEFAULT NULL COMMENT '0 not available, 1 available',
- `locations` text COMMENT 'Node location information',
- `connects` text COMMENT 'Node connection information',
- `warning_group_id` int(11) DEFAULT NULL COMMENT 'alert group id',
- `timeout` int(11) DEFAULT '0' COMMENT 'time out, unit: minute',
- `tenant_id` int(11) NOT NULL DEFAULT '-1' COMMENT 'tenant id',
- `create_time` datetime NOT NULL COMMENT 'create time',
- `update_time` datetime DEFAULT NULL COMMENT 'update time',
- PRIMARY KEY (`id`,`code`),
- UNIQUE KEY `process_unique` (`name`,`project_code`) USING BTREE
-) ENGINE=InnoDB DEFAULT CHARSET=utf8;
-
-CREATE TABLE `t_ds_process_definition_log` (
- `id` int(11) NOT NULL AUTO_INCREMENT COMMENT 'self-increasing id',
- `code` bigint(20) NOT NULL COMMENT 'encoding',
- `name` varchar(200) DEFAULT NULL COMMENT 'process definition name',
- `version` int(11) DEFAULT NULL COMMENT 'process definition version',
- `description` text COMMENT 'description',
- `project_code` bigint(20) NOT NULL COMMENT 'project code',
- `release_state` tinyint(4) DEFAULT NULL COMMENT 'process definition release state:0:offline,1:online',
- `user_id` int(11) DEFAULT NULL COMMENT 'process definition creator id',
- `global_params` text COMMENT 'global parameters',
- `flag` tinyint(4) DEFAULT NULL COMMENT '0 not available, 1 available',
- `locations` text COMMENT 'Node location information',
- `connects` text COMMENT 'Node connection information',
- `warning_group_id` int(11) DEFAULT NULL COMMENT 'alert group id',
- `timeout` int(11) DEFAULT '0' COMMENT 'time out,unit: minute',
- `tenant_id` int(11) NOT NULL DEFAULT '-1' COMMENT 'tenant id',
- `operator` int(11) DEFAULT NULL COMMENT 'operator user id',
- `operate_time` datetime DEFAULT NULL COMMENT 'operate time',
- `create_time` datetime NOT NULL COMMENT 'create time',
- `update_time` datetime DEFAULT NULL COMMENT 'update time',
- PRIMARY KEY (`id`)
-) ENGINE=InnoDB DEFAULT CHARSET=utf8;
-```
-
-从表字段可以看出 日志表仅仅比主表多了两个字段operator、operate_time
-
-
-
-#### 任务定义表
-
-案例中ab工作流task的json
-
-```json
- "tasks": [{
- "type": "SHELL",
- "id": "tasks-77643",
- "name": "a",
- "params": {
- "resourceList": [],
- "localParams": [{
- "prop": "yesterday",
- "direct": "IN",
- "type": "VARCHAR",
- "value": "${system.biz.date}"
- }],
- "rawScript": "echo ${yesterday}"
- },
- "description": "",
- "timeout": {
- "strategy": "",
- "interval": null,
- "enable": false
- },
- "runFlag": "NORMAL",
- "conditionResult": {
- "successNode": [""],
- "failedNode": [""]
- },
- "dependence": {},
- "maxRetryTimes": "0",
- "retryInterval": "1",
- "taskInstancePriority": "MEDIUM",
- "workerGroup": "default",
- "preTasks": []
- }, {
- "type": "SHELL",
- "id": "tasks-99814",
- "name": "b",
- "params": {
- "resourceList": [],
- "localParams": [{
- "prop": "today",
- "direct": "IN",
- "type": "VARCHAR",
- "value": "${system.biz.curdate}"
- }],
- "rawScript": "echo ${today}"
- },
- "description": "",
- "timeout": {
- "strategy": "",
- "interval": null,
- "enable": false
- },
- "runFlag": "NORMAL",
- "conditionResult": {
- "successNode": [""],
- "failedNode": [""]
- },
- "dependence": {},
- "maxRetryTimes": "0",
- "retryInterval": "1",
- "taskInstancePriority": "MEDIUM",
- "workerGroup": "default",
- "preTasks": ["a"]
- }]
-```
-
-dep_c工作流task的json
-
-```json
- "tasks": [{
- "type": "SHELL",
- "id": "tasks-69503",
- "name": "c",
- "params": {
- "resourceList": [],
- "localParams": [],
- "rawScript": "echo 11"
- },
- "description": "",
- "timeout": {
- "strategy": "",
- "interval": null,
- "enable": false
- },
- "runFlag": "NORMAL",
- "conditionResult": {
- "successNode": [""],
- "failedNode": [""]
- },
- "dependence": {},
- "maxRetryTimes": "0",
- "retryInterval": "1",
- "taskInstancePriority": "MEDIUM",
- "workerGroup": "default",
- "preTasks": ["dep"]
- }, {
- "type": "DEPENDENT",
- "id": "tasks-22756",
- "name": "dep",
- "params": {},
- "description": "",
- "timeout": {
- "strategy": "",
- "interval": null,
- "enable": false
- },
- "runFlag": "NORMAL",
- "conditionResult": {
- "successNode": [""],
- "failedNode": [""]
- },
- "dependence": {
- "relation": "AND",
- "dependTaskList": [{
- "relation": "AND",
- "dependItemList": [{
- "projectId": 1,
- "definitionId": 1,
- "depTasks": "b",
- "cycle": "day",
- "dateValue": "today"
- }]
- }]
- },
- "maxRetryTimes": "0",
- "retryInterval": "1",
- "taskInstancePriority": "MEDIUM",
- "workerGroup": "default",
- "preTasks": []
- }]
-```
-
-condition_test工作流task的json
-
-```json
- "tasks": [{
- "type": "SHELL",
- "id": "tasks-68456",
- "name": "d",
- "params": {
- "resourceList": [],
- "localParams": [],
- "rawScript": "echo 11"
- },
- "description": "",
- "timeout": {
- "strategy": "",
- "interval": null,
- "enable": false
- },
- "runFlag": "NORMAL",
- "conditionResult": {
- "successNode": [""],
- "failedNode": [""]
- },
- "dependence": {},
- "maxRetryTimes": "0",
- "retryInterval": "1",
- "taskInstancePriority": "MEDIUM",
- "workerGroup": "default",
- "preTasks": []
- }, {
- "type": "SHELL",
- "id": "tasks-58183",
- "name": "e",
- "params": {
- "resourceList": [],
- "localParams": [],
- "rawScript": "echo 22"
- },
- "description": "",
- "timeout": {
- "strategy": "",
- "interval": null,
- "enable": false
- },
- "runFlag": "NORMAL",
- "conditionResult": {
- "successNode": [""],
- "failedNode": [""]
- },
- "dependence": {},
- "maxRetryTimes": "0",
- "retryInterval": "1",
- "taskInstancePriority": "MEDIUM",
- "workerGroup": "default",
- "preTasks": ["cond"]
- }, {
- "type": "SHELL",
- "id": "tasks-43996",
- "name": "f",
- "params": {
- "resourceList": [],
- "localParams": [],
- "rawScript": "echo 33"
- },
- "description": "",
- "timeout": {
- "strategy": "",
- "interval": null,
- "enable": false
- },
- "runFlag": "NORMAL",
- "conditionResult": {
- "successNode": [""],
- "failedNode": [""]
- },
- "dependence": {},
- "maxRetryTimes": "0",
- "retryInterval": "1",
- "taskInstancePriority": "MEDIUM",
- "workerGroup": "default",
- "preTasks": ["cond"]
- }, {
- "type": "CONDITIONS",
- "id": "tasks-38972",
- "name": "cond",
- "params": {},
- "description": "",
- "timeout": {
- "strategy": "",
- "interval": null,
- "enable": false
- },
- "runFlag": "NORMAL",
- "conditionResult": {
- "successNode": ["e"],
- "failedNode": ["f"]
- },
- "dependence": {
- "relation": "AND",
- "dependTaskList": [{
- "relation": "AND",
- "dependItemList": [{
- "depTasks": "d",
- "status": "SUCCESS"
- }]
- }]
- },
- "maxRetryTimes": "0",
- "retryInterval": "1",
- "taskInstancePriority": "MEDIUM",
- "workerGroup": "default",
- "preTasks": ["d"]
- }]
-```
-
-从案例中可以知道SHELL/DEPENDENT/CONDITIONS类型的节点的json构成(其他任务类似SHELL),preTasks标识前置依赖节点。conditionResult结构比较固定,而dependence结构复杂,DEPENDENT和CONDITIONS类型任务的dependence结构还不一样,所以为了统一,我们将conditionResult和dependence整体放到params中,params对应表字段的task_params。
-
-这样我们就确定了t_ds_task_definition表
-
-```mysql
-CREATE TABLE `t_ds_task_definition` (
- `id` int(11) NOT NULL AUTO_INCREMENT COMMENT 'self-increasing id',
- `code` bigint(20) NOT NULL COMMENT 'encoding',
- `name` varchar(200) DEFAULT NULL COMMENT 'task definition name',
- `version` int(11) DEFAULT NULL COMMENT 'task definition version',
- `description` text COMMENT 'description',
- `project_code` bigint(20) NOT NULL COMMENT 'project code',
- `user_id` int(11) DEFAULT NULL COMMENT 'task definition creator id',
- `task_type` varchar(50) NOT NULL COMMENT 'task type',
- `task_params` text COMMENT 'job custom parameters',
- `flag` tinyint(2) DEFAULT NULL COMMENT '0 not available, 1 available',
- `task_priority` tinyint(4) DEFAULT NULL COMMENT 'job priority',
- `worker_group` varchar(200) DEFAULT NULL COMMENT 'worker grouping',
- `fail_retry_times` int(11) DEFAULT NULL COMMENT 'number of failed retries',
- `fail_retry_interval` int(11) DEFAULT NULL COMMENT 'failed retry interval',
- `timeout_flag` tinyint(2) DEFAULT '0' COMMENT 'timeout flag:0 close, 1 open',
- `timeout_notify_strategy` tinyint(4) DEFAULT NULL COMMENT 'timeout notification policy: 0 warning, 1 fail',
- `timeout` int(11) DEFAULT '0' COMMENT 'timeout length,unit: minute',
- `delay_time` int(11) DEFAULT '0' COMMENT 'delay execution time,unit: minute',
- `resource_ids` varchar(255) DEFAULT NULL COMMENT 'resource id, separated by comma',
- `create_time` datetime NOT NULL COMMENT 'create time',
- `update_time` datetime DEFAULT NULL COMMENT 'update time',
- PRIMARY KEY (`id`,`code`),
- UNIQUE KEY `task_unique` (`name`,`project_code`) USING BTREE
-) ENGINE=InnoDB DEFAULT CHARSET=utf8;
-
-CREATE TABLE `t_ds_task_definition_log` (
- `id` int(11) NOT NULL AUTO_INCREMENT COMMENT 'self-increasing id',
- `code` bigint(20) NOT NULL COMMENT 'encoding',
- `name` varchar(200) DEFAULT NULL COMMENT 'task definition name',
- `version` int(11) DEFAULT NULL COMMENT 'task definition version',
- `description` text COMMENT 'description',
- `project_code` bigint(20) NOT NULL COMMENT 'project code',
- `user_id` int(11) DEFAULT NULL COMMENT 'task definition creator id',
- `task_type` varchar(50) NOT NULL COMMENT 'task type',
- `task_params` text COMMENT 'job custom parameters',
- `flag` tinyint(2) DEFAULT NULL COMMENT '0 not available, 1 available',
- `task_priority` tinyint(4) DEFAULT NULL COMMENT 'job priority',
- `worker_group` varchar(200) DEFAULT NULL COMMENT 'worker grouping',
- `fail_retry_times` int(11) DEFAULT NULL COMMENT 'number of failed retries',
- `fail_retry_interval` int(11) DEFAULT NULL COMMENT 'failed retry interval',
- `timeout_flag` tinyint(2) DEFAULT '0' COMMENT 'timeout flag:0 close, 1 open',
- `timeout_notify_strategy` tinyint(4) DEFAULT NULL COMMENT 'timeout notification policy: 0 warning, 1 fail',
- `timeout` int(11) DEFAULT '0' COMMENT 'timeout length,unit: minute',
- `delay_time` int(11) DEFAULT '0' COMMENT 'delay execution time,unit: minute',
- `resource_ids` varchar(255) DEFAULT NULL COMMENT 'resource id, separated by comma',
- `operator` int(11) DEFAULT NULL COMMENT 'operator user id',
- `operate_time` datetime DEFAULT NULL COMMENT 'operate time',
- `create_time` datetime NOT NULL COMMENT 'create time',
- `update_time` datetime DEFAULT NULL COMMENT 'update time',
- PRIMARY KEY (`id`)
-) ENGINE=InnoDB DEFAULT CHARSET=utf8;
-```
-
-**注意:dev版本和1.3.6版本区别,dev版本已将description换成desc,并且新增了delayTime**
-
-```json
-{
- "globalParams": [],
- "tasks": [{
- "type": "SHELL",
- "id": "tasks-18200",
- "name": "d",
- "code": "",
- "params": {
- "resourceList": [],
- "localParams": [],
- "rawScript": "echo 5"
- },
- "desc": "",
- "runFlag": "NORMAL",
- "conditionResult": {
- "successNode": [
- ""
- ],
- "failedNode": [
- ""
- ]
- },
- "dependence": {},
- "maxRetryTimes": "0",
- "retryInterval": "1",
- "delayTime": "0",
- "timeout": {
- "strategy": "",
- "interval": null,
- "enable": false
- },
- "waitStartTimeout": {},
- "taskInstancePriority": "MEDIUM",
- "workerGroup": "hadoop",
- "preTasks": [],
- "depList": null
- },
- {
- "type": "SHELL",
- "id": "tasks-55225",
- "name": "e",
- "code": "",
- "params": {
- "resourceList": [],
- "localParams": [],
- "rawScript": "echo 6"
- },
- "desc": "",
- "runFlag": "NORMAL",
- "conditionResult": {
- "successNode": [
- ""
- ],
- "failedNode": [
- ""
- ]
- },
- "dependence": {},
- "maxRetryTimes": "0",
- "retryInterval": "1",
- "delayTime": "0",
- "timeout": {
- "strategy": "",
- "interval": null,
- "enable": false
- },
- "waitStartTimeout": {},
- "taskInstancePriority": "MEDIUM",
- "workerGroup": "hadoop",
- "preTasks": [
- "def"
- ],
- "depList": null
- },
- {
- "type": "SHELL",
- "id": "tasks-67639",
- "name": "f",
- "code": "",
- "params": {
- "resourceList": [],
- "localParams": [],
- "rawScript": "echo 7"
- },
- "desc": "",
- "runFlag": "NORMAL",
- "conditionResult": {
- "successNode": [
- ""
- ],
- "failedNode": [
- ""
- ]
- },
- "dependence": {},
- "maxRetryTimes": "0",
- "retryInterval": "1",
- "delayTime": "0",
- "timeout": {
- "strategy": "",
- "interval": null,
- "enable": false
- },
- "waitStartTimeout": {},
- "taskInstancePriority": "MEDIUM",
- "workerGroup": "hadoop",
- "preTasks": [
- "def"
- ],
- "depList": null
- },
- {
- "type": "CONDITIONS",
- "id": "tasks-67387",
- "name": "def",
- "code": "",
- "params": {},
- "desc": "",
- "runFlag": "NORMAL",
- "conditionResult": {
- "successNode": [
- "e"
- ],
- "failedNode": [
- "f"
- ]
- },
- "dependence": {
- "relation": "AND",
- "dependTaskList": [{
- "relation": "AND",
- "dependItemList": [{
- "depTasks": "d",
- "status": "SUCCESS"
- },
- {
- "depTasks": "d",
- "status": "FAILURE"
- }
- ]
- }]
- },
- "maxRetryTimes": "0",
- "retryInterval": "1",
- "delayTime": "0",
- "timeout": {
- "strategy": "",
- "interval": null,
- "enable": false
- },
- "waitStartTimeout": {},
- "taskInstancePriority": "MEDIUM",
- "workerGroup": "hadoop",
- "preTasks": [
- "d"
- ],
- "depList": null
- }
- ],
- "tenantId": 1,
- "timeout": 0
-}
-```
-
-
-
-#### 任务关系表
-
-preTasks标识前置依赖节点,当前节点在关系表中使用postTask标识。由于当前节点肯定存在而前置节点不一定存在,所以post不可能为空,而preTask有可能为空
-
-```mysql
-CREATE TABLE `t_ds_process_task_relation` (
- `id` int(11) NOT NULL AUTO_INCREMENT COMMENT 'self-increasing id',
- `name` varchar(200) DEFAULT NULL COMMENT 'relation name',
- `process_definition_version` int(11) DEFAULT NULL COMMENT 'process version',
- `project_code` bigint(20) NOT NULL COMMENT 'project code',
- `process_definition_code` bigint(20) NOT NULL COMMENT 'process code',
- `pre_task_code` bigint(20) NOT NULL COMMENT 'pre task code',
- `pre_task_version` int(11) NOT NULL COMMENT 'pre task version',
- `post_task_code` bigint(20) NOT NULL COMMENT 'post task code',
- `post_task_version` int(11) NOT NULL COMMENT 'post task version',
- `condition_type` tinyint(2) DEFAULT NULL COMMENT 'condition type : 0 none, 1 judge 2 delay',
- `condition_params` text COMMENT 'condition params(json)',
- `create_time` datetime NOT NULL COMMENT 'create time',
- `update_time` datetime DEFAULT NULL COMMENT 'update time',
- PRIMARY KEY (`id`)
-) ENGINE=InnoDB DEFAULT CHARSET=utf8;
-
-CREATE TABLE `t_ds_process_task_relation_log` (
- `id` int(11) NOT NULL AUTO_INCREMENT COMMENT 'self-increasing id',
- `name` varchar(200) DEFAULT NULL COMMENT 'relation name',
- `process_definition_version` int(11) DEFAULT NULL COMMENT 'process version',
- `project_code` bigint(20) NOT NULL COMMENT 'project code',
- `process_definition_code` bigint(20) NOT NULL COMMENT 'process code',
- `pre_task_code` bigint(20) NOT NULL COMMENT 'pre task code',
- `pre_task_version` int(11) NOT NULL COMMENT 'pre task version',
- `post_task_code` bigint(20) NOT NULL COMMENT 'post task code',
- `post_task_version` int(11) NOT NULL COMMENT 'post task version',
- `condition_type` tinyint(2) DEFAULT NULL COMMENT 'condition type : 0 none, 1 judge 2 delay',
- `condition_params` text COMMENT 'condition params(json)',
- `operator` int(11) DEFAULT NULL COMMENT 'operator user id',
- `operate_time` datetime DEFAULT NULL COMMENT 'operate time',
- `create_time` datetime NOT NULL COMMENT 'create time',
- `update_time` datetime DEFAULT NULL COMMENT 'update time',
- PRIMARY KEY (`id`)
-) ENGINE=InnoDB DEFAULT CHARSET=utf8;
-```
-
-对于依赖关系复杂的场景
-
-
-
-
-
-## 3、API模块如何改造
-
-
-
-- [ ] api模块进行save操作时
-
-1. 通过雪花算法生成13位的数字作为process_definition_code,工作流定义保存至process_definition(主表)和process_definition_log(日志表),这两个表保存的是同样的数据,工作流定义版本为1
-2. 通过雪花算法生成13位的数字作为task_definition_code,任务定义表保存至task_definition(主表)和task_definition_log(日志表),也是保存同样的数据,任务定义版本为1
-3. 工作流任务关系保存在 process_task_relation(主表)和process_task_relation_log(日志表),该表保存的code和version是工作流的code和version,因为任务是通过工作流进行组织,以工作流来画dag。也是通过post_task_code和post_task_version标识dag的当前节点,这个节点的前置依赖通过pre_task_code和pre_task_version来标识,如果没有依赖,pre_task_code和pre_task_version为0
-
-- [ ] api模块进行update操作时,工作流定义和任务定义直接更新主表数据,更新后的数据insert到日志表。关系表主表先删除然后再插入新的关系,关系表日志表直接插入新的关系
-
-- [ ] api模块进行delete操作时,工作流定义、任务定义和关系表直接删除主表数据,日志表数据不变动
-- [ ] api模块进行switch操作时,直接将日志表中对应version数据覆盖到主表
-
-
-
-## 4、数据交互如何改造
-
-
-
-
-
-
-
-- [ ] 在json拆分一期api模块controller层整体未变动,传入的大json还是在service层映射为ProcessData对象。insert或update操作在公共Service模块通过ProcessService.saveProcessDefiniton()入口完成保存数据库操作,按照task_definition、process_task_relation、process_definition的顺序保存。保存时,如果该任务已经存在并且关联的工作流未上线,则更改任务;如果任务关联的工作流已上线,则不允许更改任务
-
-- [ ] api查询操作时,当前还是通过工作流id来查询,在公共Service模块通过ProcessService.genTaskNodeList()入口完成数据组装,还是组装为ProcessData对象,进而生成json返回
-- [ ] Server模块(Master)也是通过公共Service模块ProcessService.genTaskNodeList()获得TaskNodeList生成调度dag,把当前任务所有信息放到 MasterExecThread.readyToSubmitTaskQueue队列,以便生成taskInstance,dispatch给worker
-
-
-
-## 5、当前json还需做什么
-
-- controller对外restAPI接口改造
-- ui模块dag改造
-- ui模块新增task操作页面
-
-
-
-**processDefinition**
-
-| 接口名称 | 参数名称 | 参数说明 | 数据类型 |
-| ----------------- | -------------------- | :--------------------------------: | ---------- |
-| | projectName | 项目名称 | string |
-| | name | 流程定义名称 | string |
-| | description | 流程定义描述信息 | string |
-| | globalParams | 全局参数 | string |
-| save | connects | 流程定义节点图标连接信息(json格式) | string |
-| | locations | 流程定义节点坐标位置信息(json格式) | string |
-| | **timeout** | **超时分钟数** | **int** |
-| | **tenantId** | **租户id** | **int** |
-| | **taskRelationJson** | **任务关系(json格式)** | **string** |
-| -- | -- | -- | -- |
-| | projectName | 项目名称 | string |
-| | **code** | **流程定义code** | **long** |
-| | name | 流程定义名称 | string |
-| | description | 流程定义描述信息 | string |
-| update | releaseState | 发布流程定义,可用值:OFFLINE,ONLINE | ref |
-| | connects | 流程定义节点图标连接信息(json格式) | string |
-| | locations | 流程定义节点坐标位置信息(json格式) | string |
-| | **timeout** | **超时分钟数** | **int** |
-| | **tenantId** | **租户id** | **int** |
-| | **taskRelationJson** | **任务关系(json格式)** | **string** |
-| | -- | -- | -- |
-| | **code** | **流程定义code** | **long** |
-| switch/deleteCode | projectName | 项目名称 | string |
-| | version | 版本号 | string |
-
-备注:taskRelationJson格式:[{"name":"","pre_task_code":0,"pre_task_version":0,"post_task_code":123456789,"post_task_version":1,"condition_type":0,"condition_params":{}},{"name":"","pre_task_code":123456789,"pre_task_version":1,"post_task_code":123451234,"post_task_version":1,"condition_type":0,"condition_params":{}}]
-
-同理其他接口请求参数processDefinitionId换成code
-
-
-
-**schedule**
-
-| 接口名称 | 参数名称 | 参数说明 | 数据类型 |
-| :---------------------: | :---------------------- | ---------------------------------------------------- | -------- |
-| | **code** | **流程定义code** | **long** |
-| | projectName | 项目名称 | string |
-| | failureStrategy | 失败策略,可用值:END,CONTINUE | string |
-| createSchedule | processInstancePriority | 流程实例优先级,可用值:HIGHEST,HIGH,MEDIUM,LOW,LOWEST | string |
-| | schedule | 定时 | string |
-| | warningGroupId | 发送组ID | int |
-| | warningType | 发送策略,可用值:NONE,SUCCESS,FAILURE,ALL | string |
-| | workerGroup | workerGroup | string |
-| -- | -- | -- | -- |
-| | **code** | **流程定义code** | **long** |
-| | projectName | 项目名称 | string |
-| queryScheduleListPaging | pageNo | 页码号 | int |
-| | pageSize | 页大小 | int |
-| | searchVal | 搜索值 | string |
-
-**taskDefinition**(新增)
... 54772 lines suppressed ...