You are viewing a plain text version of this content. The canonical link for it is here.
Posted to commits@mxnet.apache.org by GitBox <gi...@apache.org> on 2021/05/26 13:39:33 UTC
[GitHub] [incubator-mxnet] bgawrych opened a new pull request #20312: [FEATURE] Add interleaved batch_dot oneDNN fuses for new GluonNLP models
bgawrych opened a new pull request #20312:
URL: https://github.com/apache/incubator-mxnet/pull/20312
## Description ##
This change utilizes oneDNN matmul primitive capabilities and fuses following sequences of operators:
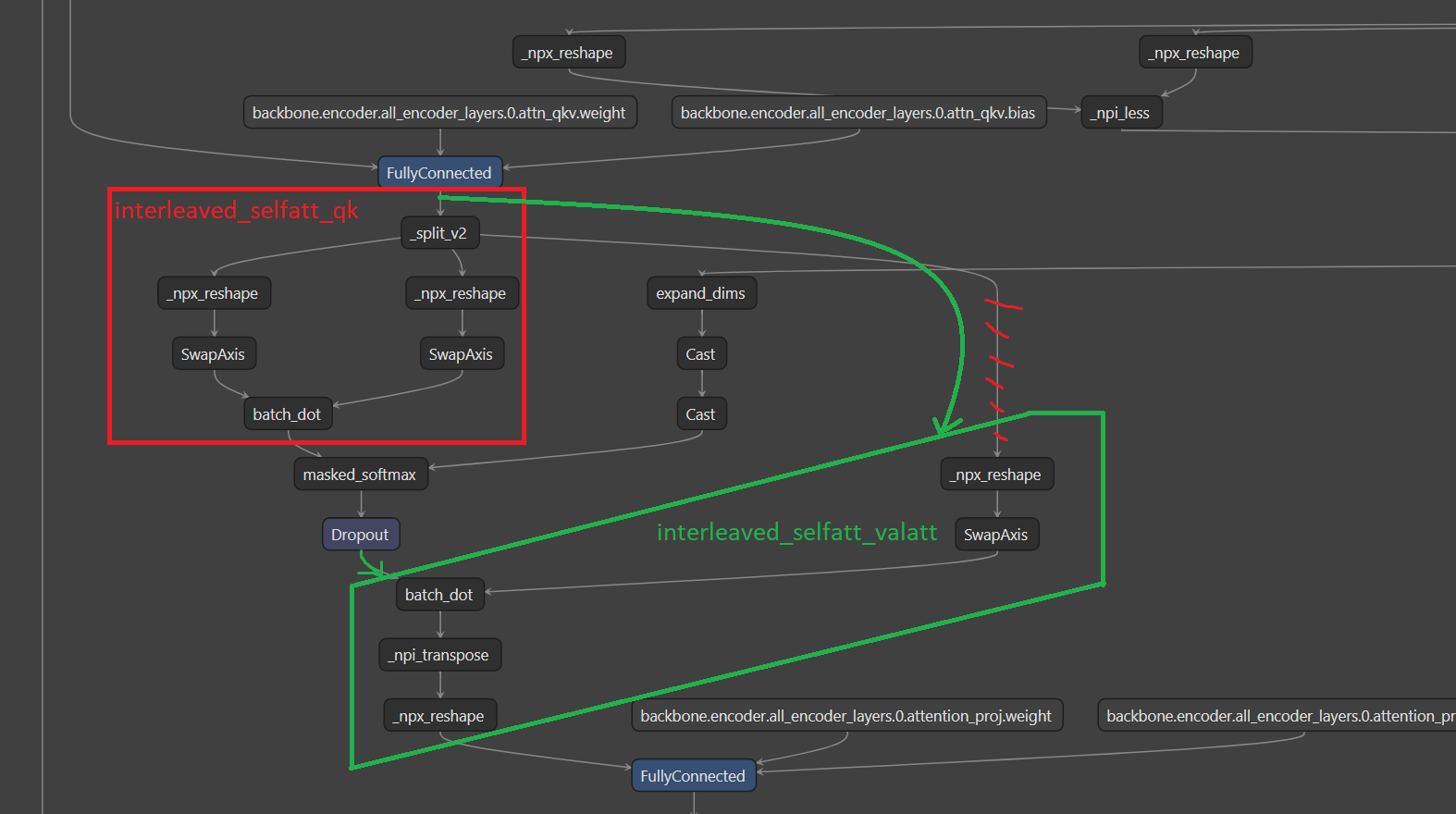
Tested on run_squad.py script from GluonNLP repository (weights downloaded from QA README.md file)

* electra-small quantized with disabled 1st fully connected layer (there is bug not related to this change) - fix incoming
* accuracy drop on bert base is caused because there wasn't custom calibration - without this change quantized accuracy is similar (69.0617)
## Checklist ##
### Essentials ###
- [X] PR's title starts with a category (e.g. [BUGFIX], [MODEL], [TUTORIAL], [FEATURE], [DOC], etc)
- [X] Changes are complete (i.e. I finished coding on this PR)
- [X] All changes have test coverage
--
This is an automated message from the Apache Git Service.
To respond to the message, please log on to GitHub and use the
URL above to go to the specific comment.
For queries about this service, please contact Infrastructure at:
users@infra.apache.org
[GitHub] [incubator-mxnet] bgawrych commented on pull request #20312: [FEATURE] Add interleaved batch_dot oneDNN fuses for new GluonNLP models
Posted by GitBox <gi...@apache.org>.
bgawrych commented on pull request #20312:
URL: https://github.com/apache/incubator-mxnet/pull/20312#issuecomment-861202684
@akarbown Can you review and merge if it's ok?
--
This is an automated message from the Apache Git Service.
To respond to the message, please log on to GitHub and use the
URL above to go to the specific comment.
For queries about this service, please contact Infrastructure at:
users@infra.apache.org
[GitHub] [incubator-mxnet] mxnet-bot commented on pull request #20312: [FEATURE] Add interleaved batch_dot oneDNN fuses for new GluonNLP models
Posted by GitBox <gi...@apache.org>.
mxnet-bot commented on pull request #20312:
URL: https://github.com/apache/incubator-mxnet/pull/20312#issuecomment-870628602
Jenkins CI successfully triggered : [unix-cpu, windows-gpu]
--
This is an automated message from the Apache Git Service.
To respond to the message, please log on to GitHub and use the
URL above to go to the specific comment.
To unsubscribe, e-mail: commits-unsubscribe@mxnet.apache.org
For queries about this service, please contact Infrastructure at:
users@infra.apache.org
[GitHub] [incubator-mxnet] akarbown merged pull request #20312: [FEATURE] Add interleaved batch_dot oneDNN fuses for new GluonNLP models
Posted by GitBox <gi...@apache.org>.
akarbown merged pull request #20312:
URL: https://github.com/apache/incubator-mxnet/pull/20312
--
This is an automated message from the Apache Git Service.
To respond to the message, please log on to GitHub and use the
URL above to go to the specific comment.
To unsubscribe, e-mail: commits-unsubscribe@mxnet.apache.org
For queries about this service, please contact Infrastructure at:
users@infra.apache.org
[GitHub] [incubator-mxnet] szha commented on pull request #20312: [FEATURE] Add interleaved batch_dot oneDNN fuses for new GluonNLP models
Posted by GitBox <gi...@apache.org>.
szha commented on pull request #20312:
URL: https://github.com/apache/incubator-mxnet/pull/20312#issuecomment-853326045
If the tests fail consistently, let's disable these tests and open an issue for tracking them.
--
This is an automated message from the Apache Git Service.
To respond to the message, please log on to GitHub and use the
URL above to go to the specific comment.
For queries about this service, please contact Infrastructure at:
users@infra.apache.org
[GitHub] [incubator-mxnet] bgawrych commented on pull request #20312: [FEATURE] Add interleaved batch_dot oneDNN fuses for new GluonNLP models
Posted by GitBox <gi...@apache.org>.
bgawrych commented on pull request #20312:
URL: https://github.com/apache/incubator-mxnet/pull/20312#issuecomment-852083153
Hi @leezu @szha, is something wrong with master CI? I get always
```
[2021-06-01T09:46:09.673Z] =========================== short test summary info ===========================
[2021-06-01T09:46:09.673Z] FAILED tests/python/gpu/test_operator_gpu.py::test_sparse_nd_pickle - mxnet.b...
[2021-06-01T09:46:09.673Z] FAILED tests/python/gpu/test_operator_gpu.py::test_sparse_nd_save_load[save]
[2021-06-01T09:46:09.673Z] FAILED tests/python/gpu/test_operator_gpu.py::test_sparse_ndarray_load_csr_npz_scipy[save]
```
on windows-gpu - in this PR and in #20227
--
This is an automated message from the Apache Git Service.
To respond to the message, please log on to GitHub and use the
URL above to go to the specific comment.
For queries about this service, please contact Infrastructure at:
users@infra.apache.org
[GitHub] [incubator-mxnet] bgawrych commented on pull request #20312: [FEATURE] Add interleaved batch_dot oneDNN fuses for new GluonNLP models
Posted by GitBox <gi...@apache.org>.
bgawrych commented on pull request #20312:
URL: https://github.com/apache/incubator-mxnet/pull/20312#issuecomment-870628551
@mxnet-bot run ci [unix-cpu, windows-gpu]
--
This is an automated message from the Apache Git Service.
To respond to the message, please log on to GitHub and use the
URL above to go to the specific comment.
To unsubscribe, e-mail: commits-unsubscribe@mxnet.apache.org
For queries about this service, please contact Infrastructure at:
users@infra.apache.org
[GitHub] [incubator-mxnet] mxnet-bot commented on pull request #20312: [FEATURE] Add interleaved batch_dot oneDNN fuses for new GluonNLP models
Posted by GitBox <gi...@apache.org>.
mxnet-bot commented on pull request #20312:
URL: https://github.com/apache/incubator-mxnet/pull/20312#issuecomment-848778364
Hey @bgawrych , Thanks for submitting the PR
All tests are already queued to run once. If tests fail, you can trigger one or more tests again with the following commands:
- To trigger all jobs: @mxnet-bot run ci [all]
- To trigger specific jobs: @mxnet-bot run ci [job1, job2]
***
**CI supported jobs**: [windows-gpu, clang, unix-gpu, edge, centos-gpu, website, sanity, unix-cpu, centos-cpu, miscellaneous, windows-cpu]
***
_Note_:
Only following 3 categories can trigger CI :PR Author, MXNet Committer, Jenkins Admin.
All CI tests must pass before the PR can be merged.
--
This is an automated message from the Apache Git Service.
To respond to the message, please log on to GitHub and use the
URL above to go to the specific comment.
For queries about this service, please contact Infrastructure at:
users@infra.apache.org
[GitHub] [incubator-mxnet] bartekkuncer commented on a change in pull request #20312: [FEATURE] Add interleaved batch_dot oneDNN fuses for new GluonNLP models
Posted by GitBox <gi...@apache.org>.
bartekkuncer commented on a change in pull request #20312:
URL: https://github.com/apache/incubator-mxnet/pull/20312#discussion_r643160215
##########
File path: src/operator/subgraph/mkldnn/mkldnn_transformer.cc
##########
@@ -0,0 +1,763 @@
+/*
+* Licensed to the Apache Software Foundation (ASF) under one
+* or more contributor license agreements. See the NOTICE file
+* distributed with this work for additional information
+* regarding copyright ownership. The ASF licenses this file
+* to you under the Apache License, Version 2.0 (the
+* "License"); you may not use this file except in compliance
+* with the License. You may obtain a copy of the License at
+*
+* http://www.apache.org/licenses/LICENSE-2.0
+*
+* Unless required by applicable law or agreed to in writing,
+* software distributed under the License is distributed on an
+* "AS IS" BASIS, WITHOUT WARRANTIES OR CONDITIONS OF ANY
+* KIND, either express or implied. See the License for the
+* specific language governing permissions and limitations
+* under the License.
+*/
+
+#if MXNET_USE_ONEDNN == 1
+
+#include <utility>
+#include <vector>
+#include <string>
+#include "../common.h"
+#include "./mkldnn_transformer-inl.h"
+#include "../../contrib/transformer-inl.h"
+#include "../../tensor/elemwise_unary_op.h"
+
+#include "../../quantization/quantization_utils.h"
+
+// 3 tensors within one (queries key values) =
+#define QKV_NUM 3
+
+namespace mxnet {
+namespace op {
+
+DMLC_REGISTER_PARAMETER(MKLDNNSelfAttParam);
+
+static bool SgMKLDNNSelfAttShape(const NodeAttrs& attrs,
+ mxnet::ShapeVector* in_shape,
+ mxnet::ShapeVector* out_shape) {
+ const auto& params = nnvm::get<MKLDNNSelfAttParam>(attrs.parsed);
+ auto qkv_shape = in_shape->at(0);
+ CHECK_EQ(qkv_shape.ndim(), 3U)
+ << "Input queries_keys_values should be 3D in batch-seq_length-proj_dim, "
+ << "but the given tensor is " << qkv_shape.ndim() << "D";
+
+ if (params.quantized) {
+ CHECK_EQ(in_shape->size(), 3U) << "Input: [queries_keys_values, min_qkv, max_qkv] "
+ << "- currently have " << in_shape->size() << " inputs";
+
+ SHAPE_ASSIGN_CHECK(*in_shape, 1, mxnet::TShape({1}));
+ SHAPE_ASSIGN_CHECK(*in_shape, 2, mxnet::TShape({1}));
+
+ out_shape->resize(3);
+ SHAPE_ASSIGN_CHECK(*out_shape, 0,
+ mxnet::TShape({qkv_shape[0], params.heads, qkv_shape[1], qkv_shape[1]}));
+ if (!params.enable_float_output) {
+ SHAPE_ASSIGN_CHECK(*out_shape, 1, mxnet::TShape({1})); // min output
+ SHAPE_ASSIGN_CHECK(*out_shape, 2, mxnet::TShape({1})); // max output
+ }
+ } else {
+ CHECK_EQ(in_shape->size(), 1U) << "Input:[queries_keys_values] - currently have "
+ << in_shape->size() << " inputs";
+ out_shape->resize(1);
+ SHAPE_ASSIGN_CHECK(*out_shape, 0,
+ mxnet::TShape({qkv_shape[0], params.heads, qkv_shape[1], qkv_shape[1]}));
+ }
+
+ return true;
+}
+
+static bool SgMKLDNNSelfAttQKInferType(const nnvm::NodeAttrs &attrs,
+ std::vector<int> *in_types,
+ std::vector<int> *out_types) {
+ const auto& params = nnvm::get<MKLDNNSelfAttParam>(attrs.parsed);
+ if (params.quantized) {
+ CHECK_EQ(in_types->size(), 3U);
+
+ CHECK(in_types->at(0) == mshadow::kInt8)
+ << "QuantizedSelfAttentionQK only supports int8 input, while "
+ << in_types->at(0) << " is given.";
+
+ TYPE_ASSIGN_CHECK(*in_types, 1, mshadow::kFloat32);
+ TYPE_ASSIGN_CHECK(*in_types, 2, mshadow::kFloat32);
+
+ if (params.enable_float_output) {
+ CHECK_EQ(out_types->size(), 1U);
+ TYPE_ASSIGN_CHECK(*out_types, 0, mshadow::kFloat32);
+ } else {
+ CHECK_EQ(out_types->size(), 3U);
+ if (params.min_calib_range.has_value() && params.max_calib_range.has_value()) {
+ TYPE_ASSIGN_CHECK(*out_types, 0, mshadow::kInt8);
+ } else {
+ TYPE_ASSIGN_CHECK(*out_types, 0, mshadow::kInt32);
+ }
+ TYPE_ASSIGN_CHECK(*out_types, 1, mshadow::kFloat32);
+ TYPE_ASSIGN_CHECK(*out_types, 2, mshadow::kFloat32);
+ }
+ } else {
+ CHECK_EQ(in_types->size(), 1U);
+ CHECK_EQ(out_types->size(), 1U);
+ TYPE_ASSIGN_CHECK(*in_types, 0, mshadow::kFloat32);
+ TYPE_ASSIGN_CHECK(*out_types, 0, mshadow::kFloat32);
+ }
+
+ return true;
+}
+
+
+class SgMKLDNNSelfAttQKOp {
+ public:
+ explicit SgMKLDNNSelfAttQKOp(const nnvm::NodeAttrs &attrs) :
+ param_(nnvm::get<MKLDNNSelfAttParam>(attrs.parsed)) {}
+
+ void Forward(const OpContext &ctx,
+ const std::vector<NDArray> &inputs,
+ const std::vector<OpReqType> &req,
+ const std::vector<NDArray> &outputs);
+
+ void Backward(const OpContext &ctx,
+ const std::vector<NDArray> &inputs,
+ const std::vector<OpReqType> &req,
+ const std::vector<NDArray> &outputs) {
+ LOG(FATAL) << "Not implemented: subgraph mkldnn self attention qk only supports "
+ "inference computation.";
+ }
+
+ void Initialize(const OpContext &ctx,
+ const std::vector<NDArray> &inputs,
+ const std::vector<OpReqType> &req,
+ const std::vector<NDArray> &outputs);
+
+ bool IsInitialized() {
+ return initialized_;
+ }
+
+ private:
+ bool initialized_{false};
+ MKLDNNSelfAttParam param_;
+ mkldnn_args_map_t args_;
+ std::shared_ptr<dnnl::matmul> fwd_;
+ std::shared_ptr<dnnl::memory> cached_query_mem_;
+ std::shared_ptr<dnnl::memory> cached_key_mem_;
+ std::shared_ptr<dnnl::memory> cached_out_mem_;
+ float min_data_;
+ float max_data_;
+ float min_output_;
+ float max_output_;
+ float data_scale_{0.0f};
+};
+
+static OpStatePtr CreateSgMKLDNNSelfAttQKState(const nnvm::NodeAttrs &attrs,
+ Context ctx,
+ const mxnet::ShapeVector &in_shapes,
+ const std::vector<int> &in_types) {
+ return OpStatePtr::Create<SgMKLDNNSelfAttQKOp>(attrs);
+}
+
+static void SgMKLDNNSelfAttQKForward(const OpStatePtr &state_pointer,
+ const OpContext &ctx,
+ const std::vector<NDArray> &inputs,
+ const std::vector<OpReqType> &req,
+ const std::vector<NDArray> &outputs) {
+ SgMKLDNNSelfAttQKOp &op = state_pointer.get_state<SgMKLDNNSelfAttQKOp>();
+ if (!op.IsInitialized()) {
+ op.Initialize(ctx, inputs, req, outputs);
+ }
+ op.Forward(ctx, inputs, req, outputs);
+}
+
+static bool SgMKLDNNSelfAttStorageType(const nnvm::NodeAttrs &attrs,
+ const int dev_mask,
+ DispatchMode *dispatch_mode,
+ std::vector<int> *in_attrs,
+ std::vector<int> *out_attrs) {
+ return MKLDNNStorageType(attrs, dev_mask, true, dispatch_mode, in_attrs, out_attrs);
+}
+
+void SgMKLDNNSelfAttQKOp::Initialize(const OpContext &ctx,
+ const std::vector<NDArray> &inputs,
+ const std::vector<OpReqType> &req,
+ const std::vector<NDArray> &outputs) {
+ using namespace mkldnn;
+
+ const auto qkv_tensor = inputs[0];
+ const auto out_tensor = outputs[0];
+
+ const auto qkv_dtype = get_mkldnn_type(qkv_tensor.dtype());
+
+ const memory::dim heads = param_.heads;
+ const memory::dim sequences = inputs[0].shape()[0];
+ const memory::dim qkv_seq_len = inputs[0].shape()[1];
+ const memory::dim output_lin_dim = inputs[0].shape()[2];
+ const memory::dim embed_dim = output_lin_dim / QKV_NUM;
+ const memory::dim head_dim = embed_dim / heads;
+ const memory::dim batch_stride = output_lin_dim * qkv_seq_len;
+
+ float min_data = 0.0f;
+ float max_data = 0.0f;
+
+ const auto engine = CpuEngine::Get()->get_engine();
+
+ memory::dims query_dims = {sequences, heads, qkv_seq_len, head_dim};
+ memory::dims key_dims = {sequences, heads, head_dim, qkv_seq_len};
+ memory::dims out_dims = {sequences, heads, qkv_seq_len, qkv_seq_len};
+
+ memory::dims query_strides = {batch_stride, head_dim, output_lin_dim, 1};
+ memory::dims key_strides = {batch_stride, head_dim, 1, output_lin_dim};
+
+ auto query_md = memory::desc(query_dims, qkv_dtype, query_strides);
+ auto key_md = memory::desc(key_dims, qkv_dtype, key_strides);
+
+ memory::desc out_md;
+
+ float oscale = 1.0f;
+ if (param_.quantized) {
+ min_data_ = inputs[1].data().dptr<float>()[0];
+ max_data_ = inputs[2].data().dptr<float>()[0];
+ data_scale_ = GetQuantizeScale(qkv_tensor.dtype(), min_data_, max_data_);
+
+ if (param_.min_calib_range.has_value() &&
+ param_.max_calib_range.has_value()) {
+ min_output_ = param_.min_calib_range.value();
+ max_output_ = param_.max_calib_range.value();
+ oscale = GetQuantizeScale(out_tensor.dtype(), min_output_, max_output_) /
+ (data_scale_ * data_scale_);
+ out_md = memory::desc(out_dims, memory::data_type::s8, memory::format_tag::abcd);
+ } else if (param_.enable_float_output) {
+ oscale = 1.0f / (data_scale_ * data_scale_);
+ out_md = dnnl::memory::desc(out_dims, memory::data_type::f32, memory::format_tag::abcd);
+ } else {
+ mshadow::Stream<cpu> *s = ctx.get_stream<cpu>();
+ mxnet_op::Kernel<QuantizationRangeForS8S8MultiplicationStruct, cpu>::Launch(
+ s, 1, &min_output_, &max_output_, &min_data, &max_data, &min_data,
+ &max_data);
+ out_md = dnnl::memory::desc(out_dims, memory::data_type::s32, memory::format_tag::abcd);
+ }
+ } else {
+ out_md = dnnl::memory::desc(out_dims, memory::data_type::f32, memory::format_tag::abcd);
+ }
+
+ dnnl::primitive_attr attr;
+ attr.set_output_scales(0, {oscale});
+ auto matmul_d = matmul::desc(query_md, key_md, out_md);
+ auto matmul_pd = matmul::primitive_desc(matmul_d, attr, engine);
+ fwd_ = std::make_shared<matmul>(matmul_pd);
+
+ MSHADOW_TYPE_SWITCH(inputs[0].dtype(), DType, {
+ DType* query_mem_ptr = inputs[0].data().dptr<DType>();
+ DType* key_mem_ptr = query_mem_ptr + embed_dim;
+ cached_query_mem_ = std::make_shared<memory>(query_md, engine, query_mem_ptr);
+ cached_key_mem_ = std::make_shared<memory>(key_md, engine, key_mem_ptr);
+ });
+
+ MSHADOW_TYPE_SWITCH(outputs[0].dtype(), DType, {
+ cached_out_mem_ = std::make_shared<memory>(out_md, engine, outputs[0].data().dptr<DType>());
+ });
+
+ args_[DNNL_ARG_SRC] = *cached_query_mem_;
+ args_[DNNL_ARG_WEIGHTS] = *cached_key_mem_;
+ args_[DNNL_ARG_DST] = *cached_out_mem_;
+ initialized_ = true;
+}
+
+
+void SgMKLDNNSelfAttQKOp::Forward(const OpContext &ctx,
+ const std::vector<NDArray> &inputs,
+ const std::vector<OpReqType> &req,
+ const std::vector<NDArray> &outputs) {
+ const size_t output_lin_dim = inputs[0].shape()[2];
+ const size_t embed_dim = output_lin_dim / QKV_NUM;
+
+ MSHADOW_TYPE_SWITCH(inputs[0].dtype(), DType, {
+ DType* query_mem_ptr = inputs[0].data().dptr<DType>();
+ DType* key_mem_ptr = query_mem_ptr + embed_dim;
+ cached_query_mem_->set_data_handle(query_mem_ptr);
+ cached_key_mem_->set_data_handle(key_mem_ptr);
+ });
+
+ MSHADOW_TYPE_SWITCH(outputs[0].dtype(), DType, {
+ cached_out_mem_->set_data_handle(outputs[0].data().dptr<DType>());
+ });
+
+ MKLDNNStream::Get()->RegisterPrimArgs(*fwd_, args_);
+ MKLDNNStream::Get()->Submit();
+
+ if (param_.quantized && !param_.enable_float_output) {
+ float* output_min = outputs[1].data().dptr<float>();
+ float* output_max = outputs[2].data().dptr<float>();
+
+ *output_min = min_output_;
+ *output_max = max_output_;
+ }
+}
+
+nnvm::ObjectPtr SgMKLDNNSelfAttQKQuantizedOp(const NodeAttrs& attrs) {
+ nnvm::ObjectPtr node = nnvm::Node::Create();
+ auto const ¶m = nnvm::get<MKLDNNSelfAttParam>(attrs.parsed);
+ node->attrs.op = Op::Get("_sg_mkldnn_selfatt_qk");
+ node->attrs.name = "quantized_" + attrs.name;
+ node->attrs.dict = attrs.dict;
+ node->attrs.dict["heads"] = std::to_string(param.heads);
+ node->attrs.dict["quantized"] = "True";
+ node->attrs.subgraphs.reserve(attrs.subgraphs.size());
+ node->attrs.subgraphs = attrs.subgraphs;
+ node->op()->attr_parser(&(node->attrs));
+ return node;
+}
+
+NNVM_REGISTER_OP(_sg_mkldnn_selfatt_qk)
+.describe(R"code(_sg_mkldnn_selfatt_qk)code" ADD_FILELINE)
+.set_num_inputs([](const NodeAttrs& attrs) {
+ auto const& param = nnvm::get<MKLDNNSelfAttParam>(attrs.parsed);
+ if (param.quantized) {
+ return 3;
+ } else {
+ return 1;
+ }
+})
+.set_num_outputs([](const NodeAttrs& attrs) {
+ auto const& param = nnvm::get<MKLDNNSelfAttParam>(attrs.parsed);
+ if (param.quantized && !param.enable_float_output) {
+ return 3;
+ } else {
+ return 1;
+ }
+})
+.set_attr_parser(ParamParser<MKLDNNSelfAttParam>)
+.set_attr<nnvm::FListInputNames>("FListInputNames", [](const NodeAttrs& attrs) {
+ auto const& param = nnvm::get<MKLDNNSelfAttParam>(attrs.parsed);
+ std::vector<std::string> input_names {"queries_keys_values"};
+ if (param.quantized) {
+ input_names.emplace_back("min_qkv");
+ input_names.emplace_back("max_qkv");
+ }
+ return input_names;
+})
+.set_attr<nnvm::FListOutputNames>("FListOutputNames", [](const NodeAttrs& attrs) {
+ auto const& param = nnvm::get<MKLDNNSelfAttParam>(attrs.parsed);
+ std::vector<std::string> output_names {"output"};
+ if (param.quantized && !param.enable_float_output) {
+ output_names.emplace_back("min_output");
+ output_names.emplace_back("max_output");
+ }
+ return output_names;
+})
+.set_attr<mxnet::FInferShape>("FInferShape", SgMKLDNNSelfAttShape)
+.set_attr<nnvm::FInferType>("FInferType", SgMKLDNNSelfAttQKInferType)
+.set_attr<FInferStorageType>("FInferStorageType", SgMKLDNNSelfAttStorageType)
+.set_attr<FCreateOpState>("FCreateOpState", CreateSgMKLDNNSelfAttQKState)
+.set_attr<FStatefulComputeEx>("FStatefulComputeEx<cpu>", SgMKLDNNSelfAttQKForward)
+.set_attr<bool>("TIsMKLDNN", true)
+.set_attr<nnvm::FGradient>("FGradient", MakeZeroGradNodes)
+.set_attr<FQuantizable>("FQuantizable", [](const NodeAttrs& attrs) {
+ return QuantizeType::kMust;
+})
+.set_attr<FQuantizedOp>("FQuantizedOp", SgMKLDNNSelfAttQKQuantizedOp)
+.set_attr<FNeedRequantize>("FNeedRequantize", [](const NodeAttrs& attrs) { return true; })
+.add_argument("queries_keys_values", "NDArray-or-Symbol", "Interleaved queries, keys and values")
+.add_arguments(MKLDNNSelfAttParam::__FIELDS__());
+
+/**********************************_sg_mkldnn_selfatt_valatt**********************************/
+
+static bool SgMKLDNNSelfAttValShape(const NodeAttrs& attrs,
+ mxnet::ShapeVector* in_shape,
+ mxnet::ShapeVector* out_shape) {
+ const auto& params = nnvm::get<MKLDNNSelfAttParam>(attrs.parsed);
+ auto att_shape = in_shape->at(0);
+ auto qkv_shape = in_shape->at(1);
+
+ CHECK_EQ(att_shape.ndim(), 4U)
+ << "Attention maps should be 4D in batch-heads-seq_length-seq_length, "
+ << "but the given tensor is " << att_shape.ndim() << "D";
+
+ CHECK_EQ(qkv_shape.ndim(), 3U)
+ << "Input queries_keys_values should be 3D in batch-seq_length-proj_dim, "
+ << "but the given tensor is " << qkv_shape.ndim() << "D";
+
+ if (params.quantized) {
+ CHECK_EQ(in_shape->size(), 6U) << "Input:[attention, queries_keys_values, "
+ << "attn_min, attn_max, qkv_min, qkv_max] - currently have "
+ << in_shape->size() << " inputs";
+ for (int i = 2; i < 6; i++) {
+ SHAPE_ASSIGN_CHECK(*in_shape, i, mxnet::TShape({1}));
+ }
+
+ out_shape->resize(3);
+ SHAPE_ASSIGN_CHECK(*out_shape, 0,
+ mxnet::TShape({att_shape[0],
+ att_shape[2],
+ att_shape[1] * qkv_shape[2] / params.heads / QKV_NUM}));
+ if (!params.enable_float_output) {
+ SHAPE_ASSIGN_CHECK(*out_shape, 1, mxnet::TShape({1})); // min output
+ SHAPE_ASSIGN_CHECK(*out_shape, 2, mxnet::TShape({1})); // max output
+ }
+ } else {
+ CHECK_EQ(in_shape->size(), 2U) << "Inputs: [queries_keys_values, attention] - currently have "
+ << in_shape->size() << " inputs";
+ auto qkv_shape = in_shape->at(1);
+ auto att_shape = in_shape->at(0);
+ CHECK_EQ(qkv_shape.ndim(), 3U)
+ << "Input queries_keys_values should be 3D in batch-seq_length-proj_dim, "
+ << "but the given tensor is " << qkv_shape.ndim() << "D";
+ out_shape->resize(1);
+ SHAPE_ASSIGN_CHECK(*out_shape, 0,
+ mxnet::TShape({att_shape[0],
+ att_shape[2],
+ att_shape[1] * qkv_shape[2] / params.heads / QKV_NUM}));
+ return true;
+ }
+
+ return true;
+}
+
+static bool SgMKLDNNSelfAttValInferType(const nnvm::NodeAttrs &attrs,
+ std::vector<int> *in_types,
+ std::vector<int> *out_types) {
+ const auto& params = nnvm::get<MKLDNNSelfAttParam>(attrs.parsed);
+
+ if (params.quantized) {
+ CHECK_EQ(in_types->size(), 6U) << "Input:[attention, queries_keys_values, min_att, max_att, "
+ "min_qkv, max_qkv] - currently have "
+ << in_types->size() << " inputs";
+
+ CHECK(in_types->at(0) == mshadow::kUint8)
+ << "QuantizedSelfAttentionQK only supports int8/uint8 input, while "
+ << in_types->at(0) << " is given.";
+ CHECK(in_types->at(1) == mshadow::kInt8 ||
+ in_types->at(1) == mshadow::kUint8)
+ << "QuantizedSelfAttentionQK only supports int8/uint8 input, while "
+ << in_types->at(1) << " is given.";
+ for (int i = 2; i < 6; i++) {
+ TYPE_ASSIGN_CHECK(*in_types, i, mshadow::kFloat32);
+ }
+
+ if (params.enable_float_output) {
+ CHECK_EQ(out_types->size(), 1U);
+ TYPE_ASSIGN_CHECK(*out_types, 0, mshadow::kFloat32);
+ } else {
+ CHECK_EQ(out_types->size(), 3U);
+ if (params.min_calib_range.has_value() && params.max_calib_range.has_value()) {
+ TYPE_ASSIGN_CHECK(*out_types, 0, mshadow::kInt8);
+ } else {
+ TYPE_ASSIGN_CHECK(*out_types, 0, mshadow::kInt32);
+ }
+ TYPE_ASSIGN_CHECK(*out_types, 1, mshadow::kFloat32);
+ TYPE_ASSIGN_CHECK(*out_types, 2, mshadow::kFloat32);
+ }
+ } else {
+ CHECK_EQ(in_types->size(), 2U);
+ CHECK_EQ(out_types->size(), 1U);
+ TYPE_ASSIGN_CHECK(*out_types, 0, mshadow::kFloat32);
+ TYPE_ASSIGN_CHECK(*in_types, 0, mshadow::kFloat32);
+ TYPE_ASSIGN_CHECK(*in_types, 1, mshadow::kFloat32);
+ }
+
+ return true;
+}
+
+nnvm::ObjectPtr SgMKLDNNSelfAttValAttQuantizedOp(const NodeAttrs& attrs) {
+ nnvm::ObjectPtr node = nnvm::Node::Create();
+ auto const ¶m = nnvm::get<MKLDNNSelfAttParam>(attrs.parsed);
+ node->attrs.op = Op::Get("_sg_mkldnn_selfatt_valatt");
+ node->attrs.name = "quantized_" + attrs.name;
+ node->attrs.dict = attrs.dict;
+ node->attrs.dict["heads"] = std::to_string(param.heads);
+ node->attrs.dict["quantized"] = "True";
+ node->attrs.subgraphs.reserve(attrs.subgraphs.size());
+ node->attrs.subgraphs = attrs.subgraphs;
+ node->op()->attr_parser(&(node->attrs));
+ return node;
+}
+
+class MKLDNNSelfAttValAttOp {
+ public:
+ explicit MKLDNNSelfAttValAttOp(const nnvm::NodeAttrs &attrs) :
+ param_(nnvm::get<MKLDNNSelfAttParam>(attrs.parsed)) {}
+
+ void Forward(const OpContext &ctx,
+ const std::vector<NDArray> &inputs,
+ const std::vector<OpReqType> &req,
+ const std::vector<NDArray> &outputs);
+
+ void Backward(const OpContext &ctx,
+ const std::vector<NDArray> &inputs,
+ const std::vector<OpReqType> &req,
+ const std::vector<NDArray> &outputs) {
+ LOG(FATAL) << "Not implemented: subgraph mkldnn self attention val only supports "
+ "inference computation.";
+ }
+
+ void Initialize(const OpContext &ctx,
+ const std::vector<NDArray> &inputs,
+ const std::vector<OpReqType> &req,
+ const std::vector<NDArray> &outputs);
+
+ bool IsInitialized() {
+ return initialized_;
+ }
+
+ private:
+ bool initialized_{false};
+ MKLDNNSelfAttParam param_;
+ mkldnn_args_map_t args_;
+ mkldnn_args_map_t reorder_args;
+ std::shared_ptr<dnnl::matmul> fwd_;
+ std::shared_ptr<dnnl::reorder> reorder_;
+ std::shared_ptr<dnnl::memory> cached_att_mem_;
+ std::shared_ptr<dnnl::memory> cached_value_mem_;
+ std::shared_ptr<dnnl::memory> cached_result_mem_;
+ std::shared_ptr<dnnl::memory> cached_tmp_mem_;
+ std::shared_ptr<dnnl::memory> cached_transposed_mem_; // op output
+ float min_qkv_;
+ float max_qkv_;
+ float min_att_;
+ float max_att_;
+ float min_output_;
+ float max_output_;
+ float qkv_scale_{0.0f};
+ float att_scale_{0.0f};
+};
+
+static OpStatePtr CreateMKLDNNSelfAttValAttState(const nnvm::NodeAttrs &attrs,
+ Context ctx,
+ const mxnet::ShapeVector &in_shapes,
+ const std::vector<int> &in_types) {
+ return OpStatePtr::Create<MKLDNNSelfAttValAttOp>(attrs);
+}
+
+static void MKLDNNSelfAttValAttForward(const OpStatePtr &state_pointer,
+ const OpContext &ctx,
+ const std::vector<NDArray> &inputs,
+ const std::vector<OpReqType> &req,
+ const std::vector<NDArray> &outputs) {
+ MKLDNNSelfAttValAttOp &op = state_pointer.get_state<MKLDNNSelfAttValAttOp>();
+ if (!op.IsInitialized()) {
+ op.Initialize(ctx, inputs, req, outputs);
+ }
+ op.Forward(ctx, inputs, req, outputs);
+}
+
+void MKLDNNSelfAttValAttOp::Initialize(const OpContext &ctx,
+ const std::vector<NDArray> &inputs,
+ const std::vector<OpReqType> &req,
+ const std::vector<NDArray> &outputs) {
+ using namespace mkldnn;
+
+ const auto attn_tensor = inputs[0];
+ const auto qkv_tensor = inputs[1];
+ const auto out_tensor = outputs[0];
+
+ const auto qkv_dtype = get_mkldnn_type(qkv_tensor.dtype());
+ const auto attn_dtype = get_mkldnn_type(attn_tensor.dtype());
+
+ const memory::dim heads = param_.heads;
+ const memory::dim sequences = qkv_tensor.shape()[0];
+ const memory::dim qkv_seq_len = qkv_tensor.shape()[1];
+ const memory::dim output_lin_dim = qkv_tensor.shape()[2];
+ const memory::dim embed_dim = output_lin_dim / QKV_NUM;
+ const memory::dim head_dim = embed_dim / heads;
+ const memory::dim batch_stride = output_lin_dim * qkv_seq_len;
+
+ const auto engine = CpuEngine::Get()->get_engine();
+
+ memory::dims attn_dims = {sequences, heads, qkv_seq_len, qkv_seq_len};
+ memory::dims value_dims = {sequences, heads, qkv_seq_len, head_dim};
+ memory::dims out_dims = {sequences, heads, qkv_seq_len, head_dim};
+
+ // needed to make transpose on 2nd and 3rd axis with oneDNN
+ memory::dims transpose_dims = {sequences, heads, qkv_seq_len, head_dim, 1};
+
+ memory::dims value_strides = {batch_stride, head_dim, output_lin_dim, 1};
+
+ // for attention tensor just use normal data layout,
+ // for value tensor we need to use strides as input tensor consists of queries, keys and values
+ const auto attn_md = memory::desc(attn_dims, attn_dtype, memory::format_tag::abcd);
+ const auto value_md = memory::desc(value_dims, qkv_dtype, value_strides);
+
+ // result = attn * value
+ // tmp = result + artificial dimension (1) - same memory ptr as result
+ // transpose = transposed tmp - output
+ memory::desc result_md, tmp_md, transpose_md;
+
+ float oscale = 1.0f;
+ auto result_mkldnn_dtype = memory::data_type::f32;
+ if (param_.quantized) {
+ min_att_ = inputs[2].data().dptr<float>()[0];
+ max_att_ = inputs[3].data().dptr<float>()[0];
+ min_qkv_ = inputs[4].data().dptr<float>()[0];
+ max_qkv_ = inputs[5].data().dptr<float>()[0];
+
+ att_scale_ = GetQuantizeScale(mshadow::kUint8, min_att_, max_att_);
+ qkv_scale_ = GetQuantizeScale(mshadow::kInt8, min_qkv_, max_qkv_);
+
+ if (param_.min_calib_range.has_value() &&
+ param_.max_calib_range.has_value()) {
+ min_output_ = param_.min_calib_range.value();
+ max_output_ = param_.max_calib_range.value();
+ oscale = GetQuantizeScale(out_tensor.dtype(), min_output_, max_output_) /
+ (att_scale_ * qkv_scale_);
+ result_mkldnn_dtype = memory::data_type::s8;
+ } else if (param_.enable_float_output) {
+ oscale = 1.0f / (att_scale_ * qkv_scale_);
+ result_mkldnn_dtype = memory::data_type::f32;
+ } else {
+ mshadow::Stream<cpu> *s = ctx.get_stream<cpu>();
+ mxnet_op::Kernel<QuantizationRangeForS8S8MultiplicationStruct, cpu>::Launch(
+ s, 1, &min_output_, &max_output_, &min_att_, &max_att_, &min_qkv_,
+ &max_qkv_);
+ result_mkldnn_dtype = memory::data_type::s32;
+ }
+ } else {
+ result_mkldnn_dtype = memory::data_type::f32;
+ }
+
+ result_md = memory::desc(out_dims, result_mkldnn_dtype, memory::format_tag::abcd);
+ tmp_md = memory::desc(transpose_dims, result_mkldnn_dtype, memory::format_tag::abcde);
+ transpose_md = memory::desc(transpose_dims, result_mkldnn_dtype, memory::format_tag::acbde);;
Review comment:
Doubled ';' at the end of the line.
--
This is an automated message from the Apache Git Service.
To respond to the message, please log on to GitHub and use the
URL above to go to the specific comment.
For queries about this service, please contact Infrastructure at:
users@infra.apache.org