You are viewing a plain text version of this content. The canonical link for it is here.
Posted to commits@mxnet.apache.org by GitBox <gi...@apache.org> on 2018/10/25 21:41:44 UTC
[GitHub] szha closed pull request #12929: Update bilstm integer array
sorting example
szha closed pull request #12929: Update bilstm integer array sorting example
URL: https://github.com/apache/incubator-mxnet/pull/12929
This is a PR merged from a forked repository.
As GitHub hides the original diff on merge, it is displayed below for
the sake of provenance:
As this is a foreign pull request (from a fork), the diff is supplied
below (as it won't show otherwise due to GitHub magic):
diff --git a/example/bi-lstm-sort/README.md b/example/bi-lstm-sort/README.md
index 3bacc868636..f00cc85caa3 100644
--- a/example/bi-lstm-sort/README.md
+++ b/example/bi-lstm-sort/README.md
@@ -1,24 +1,14 @@
-This is an example of using bidirection lstm to sort an array.
+# Bidirectionnal LSTM to sort an array.
-Run the training script by doing the following:
+This is an example of using bidirectionmal lstm to sort an array. Please refer to the notebook.
-```
-python lstm_sort.py --start-range 100 --end-range 1000 --cpu
-```
-You can provide the start-range and end-range for the numbers and whether to train on the cpu or not.
-By default the script tries to train on the GPU. The default start-range is 100 and end-range is 1000.
+We train a bidirectionnal LSTM to sort an array of integer.
-At last, test model by doing the following:
+For example:
-```
-python infer_sort.py 234 189 785 763 231
-```
+`500 30 999 10 130` should give us `10 30 130 500 999`
-This should output the sorted seq like the following:
-```
-189
-231
-234
-763
-785
-```
+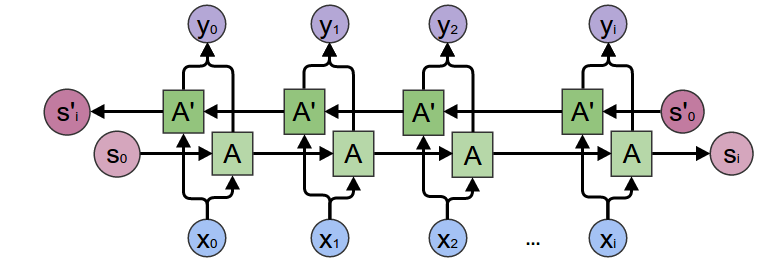
+
+
+([Diagram source](http://colah.github.io/posts/2015-09-NN-Types-FP/))
\ No newline at end of file
diff --git a/example/bi-lstm-sort/bi-lstm-sort.ipynb b/example/bi-lstm-sort/bi-lstm-sort.ipynb
new file mode 100644
index 00000000000..085117674b5
--- /dev/null
+++ b/example/bi-lstm-sort/bi-lstm-sort.ipynb
@@ -0,0 +1,607 @@
+{
+ "cells": [
+ {
+ "cell_type": "markdown",
+ "metadata": {},
+ "source": [
+ "# Using a bi-lstm to sort a sequence of integers"
+ ]
+ },
+ {
+ "cell_type": "code",
+ "execution_count": 1,
+ "metadata": {},
+ "outputs": [],
+ "source": [
+ "import random\n",
+ "import string\n",
+ "\n",
+ "import mxnet as mx\n",
+ "from mxnet import gluon, nd\n",
+ "import numpy as np"
+ ]
+ },
+ {
+ "cell_type": "markdown",
+ "metadata": {},
+ "source": [
+ "## Data Preparation"
+ ]
+ },
+ {
+ "cell_type": "code",
+ "execution_count": 2,
+ "metadata": {},
+ "outputs": [],
+ "source": [
+ "max_num = 999\n",
+ "dataset_size = 60000\n",
+ "seq_len = 5\n",
+ "split = 0.8\n",
+ "batch_size = 512\n",
+ "ctx = mx.gpu() if len(mx.test_utils.list_gpus()) > 0 else mx.cpu()"
+ ]
+ },
+ {
+ "cell_type": "markdown",
+ "metadata": {},
+ "source": [
+ "We are getting a dataset of **dataset_size** sequences of integers of length **seq_len** between **0** and **max_num**. We use **split*100%** of them for training and the rest for testing.\n",
+ "\n",
+ "\n",
+ "For example:\n",
+ "\n",
+ "50 10 200 999 30\n",
+ "\n",
+ "Should return\n",
+ "\n",
+ "10 30 50 200 999"
+ ]
+ },
+ {
+ "cell_type": "code",
+ "execution_count": 3,
+ "metadata": {},
+ "outputs": [],
+ "source": [
+ "X = mx.random.uniform(low=0, high=max_num, shape=(dataset_size, seq_len)).astype('int32').asnumpy()\n",
+ "Y = X.copy()\n",
+ "Y.sort() #Let's sort X to get the target"
+ ]
+ },
+ {
+ "cell_type": "code",
+ "execution_count": 4,
+ "metadata": {},
+ "outputs": [
+ {
+ "name": "stdout",
+ "output_type": "stream",
+ "text": [
+ "Input [548, 592, 714, 843, 602]\n",
+ "Target [548, 592, 602, 714, 843]\n"
+ ]
+ }
+ ],
+ "source": [
+ "print(\"Input {}\\nTarget {}\".format(X[0].tolist(), Y[0].tolist()))"
+ ]
+ },
+ {
+ "cell_type": "markdown",
+ "metadata": {},
+ "source": [
+ "For the purpose of training, we encode the input as characters rather than numbers"
+ ]
+ },
+ {
+ "cell_type": "code",
+ "execution_count": 5,
+ "metadata": {},
+ "outputs": [
+ {
+ "name": "stdout",
+ "output_type": "stream",
+ "text": [
+ "0123456789 \n",
+ "{'0': 0, '1': 1, '2': 2, '3': 3, '4': 4, '5': 5, '6': 6, '7': 7, '8': 8, '9': 9, ' ': 10}\n"
+ ]
+ }
+ ],
+ "source": [
+ "vocab = string.digits + \" \"\n",
+ "print(vocab)\n",
+ "vocab_idx = { c:i for i,c in enumerate(vocab)}\n",
+ "print(vocab_idx)"
+ ]
+ },
+ {
+ "cell_type": "markdown",
+ "metadata": {},
+ "source": [
+ "We write a transform that will convert our numbers into text of maximum length **max_len**, and one-hot encode the characters.\n",
+ "For example:\n",
+ "\n",
+ "\"30 10\" corresponding indices are [3, 0, 10, 1, 0]\n",
+ "\n",
+ "We then one hot encode that and get a matrix representation of our input. We don't need to encode our target as the loss we are going to use support sparse labels"
+ ]
+ },
+ {
+ "cell_type": "code",
+ "execution_count": 6,
+ "metadata": {},
+ "outputs": [
+ {
+ "name": "stdout",
+ "output_type": "stream",
+ "text": [
+ "Maximum length of the string: 19\n"
+ ]
+ }
+ ],
+ "source": [
+ "max_len = len(str(max_num))*seq_len+(seq_len-1)\n",
+ "print(\"Maximum length of the string: %s\" % max_len)"
+ ]
+ },
+ {
+ "cell_type": "code",
+ "execution_count": 7,
+ "metadata": {},
+ "outputs": [],
+ "source": [
+ "def transform(x, y):\n",
+ " x_string = ' '.join(map(str, x.tolist()))\n",
+ " x_string_padded = x_string + ' '*(max_len-len(x_string))\n",
+ " x = [vocab_idx[c] for c in x_string_padded]\n",
+ " y_string = ' '.join(map(str, y.tolist()))\n",
+ " y_string_padded = y_string + ' '*(max_len-len(y_string))\n",
+ " y = [vocab_idx[c] for c in y_string_padded]\n",
+ " return mx.nd.one_hot(mx.nd.array(x), len(vocab)), mx.nd.array(y)"
+ ]
+ },
+ {
+ "cell_type": "code",
+ "execution_count": 8,
+ "metadata": {},
+ "outputs": [],
+ "source": [
+ "split_idx = int(split*len(X))\n",
+ "train_dataset = gluon.data.ArrayDataset(X[:split_idx], Y[:split_idx]).transform(transform)\n",
+ "test_dataset = gluon.data.ArrayDataset(X[split_idx:], Y[split_idx:]).transform(transform)"
+ ]
+ },
+ {
+ "cell_type": "code",
+ "execution_count": 9,
+ "metadata": {},
+ "outputs": [
+ {
+ "name": "stdout",
+ "output_type": "stream",
+ "text": [
+ "Input [548 592 714 843 602]\n",
+ "Transformed data Input \n",
+ "[[0. 0. 0. 0. 0. 1. 0. 0. 0. 0. 0.]\n",
+ " [0. 0. 0. 0. 1. 0. 0. 0. 0. 0. 0.]\n",
+ " [0. 0. 0. 0. 0. 0. 0. 0. 1. 0. 0.]\n",
+ " [0. 0. 0. 0. 0. 0. 0. 0. 0. 0. 1.]\n",
+ " [0. 0. 0. 0. 0. 1. 0. 0. 0. 0. 0.]\n",
+ " [0. 0. 0. 0. 0. 0. 0. 0. 0. 1. 0.]\n",
+ " [0. 0. 1. 0. 0. 0. 0. 0. 0. 0. 0.]\n",
+ " [0. 0. 0. 0. 0. 0. 0. 0. 0. 0. 1.]\n",
+ " [0. 0. 0. 0. 0. 0. 0. 1. 0. 0. 0.]\n",
+ " [0. 1. 0. 0. 0. 0. 0. 0. 0. 0. 0.]\n",
+ " [0. 0. 0. 0. 1. 0. 0. 0. 0. 0. 0.]\n",
+ " [0. 0. 0. 0. 0. 0. 0. 0. 0. 0. 1.]\n",
+ " [0. 0. 0. 0. 0. 0. 0. 0. 1. 0. 0.]\n",
+ " [0. 0. 0. 0. 1. 0. 0. 0. 0. 0. 0.]\n",
+ " [0. 0. 0. 1. 0. 0. 0. 0. 0. 0. 0.]\n",
+ " [0. 0. 0. 0. 0. 0. 0. 0. 0. 0. 1.]\n",
+ " [0. 0. 0. 0. 0. 0. 1. 0. 0. 0. 0.]\n",
+ " [1. 0. 0. 0. 0. 0. 0. 0. 0. 0. 0.]\n",
+ " [0. 0. 1. 0. 0. 0. 0. 0. 0. 0. 0.]]\n",
+ "<NDArray 19x11 @cpu(0)>\n",
+ "Target [548 592 602 714 843]\n",
+ "Transformed data Target \n",
+ "[ 5. 4. 8. 10. 5. 9. 2. 10. 6. 0. 2. 10. 7. 1. 4. 10. 8. 4.\n",
+ " 3.]\n",
+ "<NDArray 19 @cpu(0)>\n"
+ ]
+ }
+ ],
+ "source": [
+ "print(\"Input {}\".format(X[0]))\n",
+ "print(\"Transformed data Input {}\".format(train_dataset[0][0]))\n",
+ "print(\"Target {}\".format(Y[0]))\n",
+ "print(\"Transformed data Target {}\".format(train_dataset[0][1]))"
+ ]
+ },
+ {
+ "cell_type": "code",
+ "execution_count": 10,
+ "metadata": {},
+ "outputs": [],
+ "source": [
+ "train_data = gluon.data.DataLoader(train_dataset, batch_size=batch_size, shuffle=True, num_workers=20, last_batch='rollover')\n",
+ "test_data = gluon.data.DataLoader(test_dataset, batch_size=batch_size, shuffle=False, num_workers=5, last_batch='rollover')"
+ ]
+ },
+ {
+ "cell_type": "markdown",
+ "metadata": {},
+ "source": [
+ "## Creating the network"
+ ]
+ },
+ {
+ "cell_type": "code",
+ "execution_count": 11,
+ "metadata": {},
+ "outputs": [],
+ "source": [
+ "net = gluon.nn.HybridSequential()\n",
+ "with net.name_scope():\n",
+ " net.add(\n",
+ " gluon.rnn.LSTM(hidden_size=128, num_layers=2, layout='NTC', bidirectional=True),\n",
+ " gluon.nn.Dense(len(vocab), flatten=False)\n",
+ " )"
+ ]
+ },
+ {
+ "cell_type": "code",
+ "execution_count": 12,
+ "metadata": {},
+ "outputs": [],
+ "source": [
+ "net.initialize(mx.init.Xavier(), ctx=ctx)"
+ ]
+ },
+ {
+ "cell_type": "code",
+ "execution_count": 13,
+ "metadata": {},
+ "outputs": [],
+ "source": [
+ "loss = gluon.loss.SoftmaxCELoss()"
+ ]
+ },
+ {
+ "cell_type": "markdown",
+ "metadata": {},
+ "source": [
+ "We use a learning rate schedule to improve the convergence of the model"
+ ]
+ },
+ {
+ "cell_type": "code",
+ "execution_count": 14,
+ "metadata": {},
+ "outputs": [],
+ "source": [
+ "schedule = mx.lr_scheduler.FactorScheduler(step=len(train_data)*10, factor=0.75)\n",
+ "schedule.base_lr = 0.01"
+ ]
+ },
+ {
+ "cell_type": "code",
+ "execution_count": 15,
+ "metadata": {},
+ "outputs": [],
+ "source": [
+ "trainer = gluon.Trainer(net.collect_params(), 'adam', {'learning_rate':0.01, 'lr_scheduler':schedule})"
+ ]
+ },
+ {
+ "cell_type": "markdown",
+ "metadata": {},
+ "source": [
+ "## Training loop"
+ ]
+ },
+ {
+ "cell_type": "code",
+ "execution_count": 16,
+ "metadata": {},
+ "outputs": [
+ {
+ "name": "stdout",
+ "output_type": "stream",
+ "text": [
+ "Epoch [0] Loss: 1.6627886372227823, LR 0.01\n",
+ "Epoch [1] Loss: 1.210370733382854, LR 0.01\n",
+ "Epoch [2] Loss: 0.9692377131035987, LR 0.01\n",
+ "Epoch [3] Loss: 0.7976046623067653, LR 0.01\n",
+ "Epoch [4] Loss: 0.5714595343476983, LR 0.01\n",
+ "Epoch [5] Loss: 0.4458411196444897, LR 0.01\n",
+ "Epoch [6] Loss: 0.36039798817736035, LR 0.01\n",
+ "Epoch [7] Loss: 0.32665719377233626, LR 0.01\n",
+ "Epoch [8] Loss: 0.262064205702915, LR 0.01\n",
+ "Epoch [9] Loss: 0.22285924059279422, LR 0.0075\n",
+ "Epoch [10] Loss: 0.19018426854559717, LR 0.0075\n",
+ "Epoch [11] Loss: 0.1718730723604243, LR 0.0075\n",
+ "Epoch [12] Loss: 0.15736752171670237, LR 0.0075\n",
+ "Epoch [13] Loss: 0.14579375246737866, LR 0.0075\n",
+ "Epoch [14] Loss: 0.13546599733068587, LR 0.0075\n",
+ "Epoch [15] Loss: 0.12490207590955368, LR 0.0075\n",
+ "Epoch [16] Loss: 0.11803316300915133, LR 0.0075\n",
+ "Epoch [17] Loss: 0.10653189395336395, LR 0.0075\n",
+ "Epoch [18] Loss: 0.10514750379197141, LR 0.0075\n",
+ "Epoch [19] Loss: 0.09590611559279422, LR 0.005625\n",
+ "Epoch [20] Loss: 0.08146028108494256, LR 0.005625\n",
+ "Epoch [21] Loss: 0.07707348782965477, LR 0.005625\n",
+ "Epoch [22] Loss: 0.07206193436967566, LR 0.005625\n",
+ "Epoch [23] Loss: 0.07001185417175293, LR 0.005625\n",
+ "Epoch [24] Loss: 0.06797058351578252, LR 0.005625\n",
+ "Epoch [25] Loss: 0.0649358110224947, LR 0.005625\n",
+ "Epoch [26] Loss: 0.06219124286732775, LR 0.005625\n",
+ "Epoch [27] Loss: 0.06075144828634059, LR 0.005625\n",
+ "Epoch [28] Loss: 0.05711334495134251, LR 0.005625\n",
+ "Epoch [29] Loss: 0.054747099572039666, LR 0.00421875\n",
+ "Epoch [30] Loss: 0.0441775271233092, LR 0.00421875\n",
+ "Epoch [31] Loss: 0.041551097910454936, LR 0.00421875\n",
+ "Epoch [32] Loss: 0.04095017269093503, LR 0.00421875\n",
+ "Epoch [33] Loss: 0.04045371045457556, LR 0.00421875\n",
+ "Epoch [34] Loss: 0.038867686657195394, LR 0.00421875\n",
+ "Epoch [35] Loss: 0.038131744303601854, LR 0.00421875\n",
+ "Epoch [36] Loss: 0.039834817250569664, LR 0.00421875\n",
+ "Epoch [37] Loss: 0.03669035941996473, LR 0.00421875\n",
+ "Epoch [38] Loss: 0.03373505967728635, LR 0.00421875\n",
+ "Epoch [39] Loss: 0.03164981273894615, LR 0.0031640625\n",
+ "Epoch [40] Loss: 0.025532766055035336, LR 0.0031640625\n",
+ "Epoch [41] Loss: 0.022659448867148543, LR 0.0031640625\n",
+ "Epoch [42] Loss: 0.02307056112492338, LR 0.0031640625\n",
+ "Epoch [43] Loss: 0.02236944056571798, LR 0.0031640625\n",
+ "Epoch [44] Loss: 0.022204211963120328, LR 0.0031640625\n",
+ "Epoch [45] Loss: 0.02262336903430046, LR 0.0031640625\n",
+ "Epoch [46] Loss: 0.02253308448385685, LR 0.0031640625\n",
+ "Epoch [47] Loss: 0.025286573044797207, LR 0.0031640625\n",
+ "Epoch [48] Loss: 0.02439300988310127, LR 0.0031640625\n",
+ "Epoch [49] Loss: 0.017976388018181983, LR 0.002373046875\n",
+ "Epoch [50] Loss: 0.014343131095805067, LR 0.002373046875\n",
+ "Epoch [51] Loss: 0.013039355582379281, LR 0.002373046875\n",
+ "Epoch [52] Loss: 0.011884741885687715, LR 0.002373046875\n",
+ "Epoch [53] Loss: 0.011438189668858305, LR 0.002373046875\n",
+ "Epoch [54] Loss: 0.011447292693117832, LR 0.002373046875\n",
+ "Epoch [55] Loss: 0.014212571560068334, LR 0.002373046875\n",
+ "Epoch [56] Loss: 0.019900493724371797, LR 0.002373046875\n",
+ "Epoch [57] Loss: 0.02102568301748722, LR 0.002373046875\n",
+ "Epoch [58] Loss: 0.01346214400961044, LR 0.002373046875\n",
+ "Epoch [59] Loss: 0.010107964911359422, LR 0.0017797851562500002\n",
+ "Epoch [60] Loss: 0.008353193600972494, LR 0.0017797851562500002\n",
+ "Epoch [61] Loss: 0.007678258292218472, LR 0.0017797851562500002\n",
+ "Epoch [62] Loss: 0.007262124660167288, LR 0.0017797851562500002\n",
+ "Epoch [63] Loss: 0.00705223578087827, LR 0.0017797851562500002\n",
+ "Epoch [64] Loss: 0.006788556293774677, LR 0.0017797851562500002\n",
+ "Epoch [65] Loss: 0.006473606571238091, LR 0.0017797851562500002\n",
+ "Epoch [66] Loss: 0.006206096486842378, LR 0.0017797851562500002\n",
+ "Epoch [67] Loss: 0.00584477313021396, LR 0.0017797851562500002\n",
+ "Epoch [68] Loss: 0.005648705267137097, LR 0.0017797851562500002\n",
+ "Epoch [69] Loss: 0.006481769871204458, LR 0.0013348388671875003\n",
+ "Epoch [70] Loss: 0.008430448618341, LR 0.0013348388671875003\n",
+ "Epoch [71] Loss: 0.006877245421105242, LR 0.0013348388671875003\n",
+ "Epoch [72] Loss: 0.005671108281740578, LR 0.0013348388671875003\n",
+ "Epoch [73] Loss: 0.004832422162624116, LR 0.0013348388671875003\n",
+ "Epoch [74] Loss: 0.004441103402604448, LR 0.0013348388671875003\n",
+ "Epoch [75] Loss: 0.004216198591475791, LR 0.0013348388671875003\n",
+ "Epoch [76] Loss: 0.004041922989711967, LR 0.0013348388671875003\n",
+ "Epoch [77] Loss: 0.003937713643337818, LR 0.0013348388671875003\n",
+ "Epoch [78] Loss: 0.010251983049068046, LR 0.0013348388671875003\n",
+ "Epoch [79] Loss: 0.01829354052848004, LR 0.0010011291503906252\n",
+ "Epoch [80] Loss: 0.006723233448561802, LR 0.0010011291503906252\n",
+ "Epoch [81] Loss: 0.004397524798170049, LR 0.0010011291503906252\n",
+ "Epoch [82] Loss: 0.0038475305476087206, LR 0.0010011291503906252\n",
+ "Epoch [83] Loss: 0.003591177945441388, LR 0.0010011291503906252\n",
+ "Epoch [84] Loss: 0.003425112014175743, LR 0.0010011291503906252\n",
+ "Epoch [85] Loss: 0.0032633850549129728, LR 0.0010011291503906252\n",
+ "Epoch [86] Loss: 0.0031762316505959693, LR 0.0010011291503906252\n",
+ "Epoch [87] Loss: 0.0030452777096565734, LR 0.0010011291503906252\n",
+ "Epoch [88] Loss: 0.002950224184220837, LR 0.0010011291503906252\n",
+ "Epoch [89] Loss: 0.002821172171450676, LR 0.0007508468627929689\n",
+ "Epoch [90] Loss: 0.002725780961361337, LR 0.0007508468627929689\n",
+ "Epoch [91] Loss: 0.002660556359493986, LR 0.0007508468627929689\n",
+ "Epoch [92] Loss: 0.0026011724946319414, LR 0.0007508468627929689\n",
+ "Epoch [93] Loss: 0.0025355776256703317, LR 0.0007508468627929689\n",
+ "Epoch [94] Loss: 0.0024825221997626283, LR 0.0007508468627929689\n",
+ "Epoch [95] Loss: 0.0024245587435174497, LR 0.0007508468627929689\n",
+ "Epoch [96] Loss: 0.002365282145879602, LR 0.0007508468627929689\n",
+ "Epoch [97] Loss: 0.0023112583984719946, LR 0.0007508468627929689\n",
+ "Epoch [98] Loss: 0.002257173682780976, LR 0.0007508468627929689\n",
+ "Epoch [99] Loss: 0.002162747085094452, LR 0.0005631351470947267\n"
+ ]
+ }
+ ],
+ "source": [
+ "epochs = 100\n",
+ "for e in range(epochs):\n",
+ " epoch_loss = 0.\n",
+ " for i, (data, label) in enumerate(train_data):\n",
+ " data = data.as_in_context(ctx)\n",
+ " label = label.as_in_context(ctx)\n",
+ "\n",
+ " with mx.autograd.record():\n",
+ " output = net(data)\n",
+ " l = loss(output, label)\n",
+ "\n",
+ " l.backward()\n",
+ " trainer.step(data.shape[0])\n",
+ " \n",
+ " epoch_loss += l.mean()\n",
+ " \n",
+ " print(\"Epoch [{}] Loss: {}, LR {}\".format(e, epoch_loss.asscalar()/(i+1), trainer.learning_rate))"
+ ]
+ },
+ {
+ "cell_type": "markdown",
+ "metadata": {},
+ "source": [
+ "## Testing"
+ ]
+ },
+ {
+ "cell_type": "markdown",
+ "metadata": {},
+ "source": [
+ "We get a random element from the testing set"
+ ]
+ },
+ {
+ "cell_type": "code",
+ "execution_count": 17,
+ "metadata": {},
+ "outputs": [],
+ "source": [
+ "n = random.randint(0, len(test_data)-1)\n",
+ "\n",
+ "x_orig = X[split_idx+n]\n",
+ "y_orig = Y[split_idx+n]"
+ ]
+ },
+ {
+ "cell_type": "code",
+ "execution_count": 41,
+ "metadata": {},
+ "outputs": [],
+ "source": [
+ "def get_pred(x):\n",
+ " x, _ = transform(x, x)\n",
+ " output = net(x.as_in_context(ctx).expand_dims(axis=0))\n",
+ "\n",
+ " # Convert output back to string\n",
+ " pred = ''.join([vocab[int(o)] for o in output[0].argmax(axis=1).asnumpy().tolist()])\n",
+ " return pred"
+ ]
+ },
+ {
+ "cell_type": "markdown",
+ "metadata": {},
+ "source": [
+ "Printing the result"
+ ]
+ },
+ {
+ "cell_type": "code",
+ "execution_count": 43,
+ "metadata": {},
+ "outputs": [
+ {
+ "name": "stdout",
+ "output_type": "stream",
+ "text": [
+ "X 611 671 275 871 944\n",
+ "Predicted 275 611 671 871 944\n",
+ "Label 275 611 671 871 944\n"
+ ]
+ }
+ ],
+ "source": [
+ "x_ = ' '.join(map(str,x_orig))\n",
+ "label = ' '.join(map(str,y_orig))\n",
+ "print(\"X {}\\nPredicted {}\\nLabel {}\".format(x_, get_pred(x_orig), label))"
+ ]
+ },
+ {
+ "cell_type": "markdown",
+ "metadata": {},
+ "source": [
+ "We can also pick our own example, and the network manages to sort it without problem:"
+ ]
+ },
+ {
+ "cell_type": "code",
+ "execution_count": 66,
+ "metadata": {},
+ "outputs": [
+ {
+ "name": "stdout",
+ "output_type": "stream",
+ "text": [
+ "10 30 130 500 999 \n"
+ ]
+ }
+ ],
+ "source": [
+ "print(get_pred(np.array([500, 30, 999, 10, 130])))"
+ ]
+ },
+ {
+ "cell_type": "markdown",
+ "metadata": {},
+ "source": [
+ "The model has even learned to generalize to examples not on the training set"
+ ]
+ },
+ {
+ "cell_type": "code",
+ "execution_count": 64,
+ "metadata": {},
+ "outputs": [
+ {
+ "name": "stdout",
+ "output_type": "stream",
+ "text": [
+ "Only four numbers: 105 202 302 501 \n"
+ ]
+ }
+ ],
+ "source": [
+ "print(\"Only four numbers:\", get_pred(np.array([105, 302, 501, 202])))"
+ ]
+ },
+ {
+ "cell_type": "markdown",
+ "metadata": {},
+ "source": [
+ "However we can see it has trouble with other edge cases:"
+ ]
+ },
+ {
+ "cell_type": "code",
+ "execution_count": 63,
+ "metadata": {},
+ "outputs": [
+ {
+ "name": "stdout",
+ "output_type": "stream",
+ "text": [
+ "Small digits: 8 0 42 28 \n",
+ "Small digits, 6 numbers: 10 0 20 82 71 115 \n"
+ ]
+ }
+ ],
+ "source": [
+ "print(\"Small digits:\", get_pred(np.array([10, 3, 5, 2, 8])))\n",
+ "print(\"Small digits, 6 numbers:\", get_pred(np.array([10, 33, 52, 21, 82, 10])))"
+ ]
+ },
+ {
+ "cell_type": "markdown",
+ "metadata": {},
+ "source": [
+ "This could be improved by adjusting the training dataset accordingly"
+ ]
+ }
+ ],
+ "metadata": {
+ "kernelspec": {
+ "display_name": "Python 3",
+ "language": "python",
+ "name": "python3"
+ },
+ "language_info": {
+ "codemirror_mode": {
+ "name": "ipython",
+ "version": 3
+ },
+ "file_extension": ".py",
+ "mimetype": "text/x-python",
+ "name": "python",
+ "nbconvert_exporter": "python",
+ "pygments_lexer": "ipython3",
+ "version": "3.6.4"
+ }
+ },
+ "nbformat": 4,
+ "nbformat_minor": 2
+}
diff --git a/example/bi-lstm-sort/gen_data.py b/example/bi-lstm-sort/gen_data.py
deleted file mode 100644
index 55af1b45554..00000000000
--- a/example/bi-lstm-sort/gen_data.py
+++ /dev/null
@@ -1,37 +0,0 @@
-# Licensed to the Apache Software Foundation (ASF) under one
-# or more contributor license agreements. See the NOTICE file
-# distributed with this work for additional information
-# regarding copyright ownership. The ASF licenses this file
-# to you under the Apache License, Version 2.0 (the
-# "License"); you may not use this file except in compliance
-# with the License. You may obtain a copy of the License at
-#
-# http://www.apache.org/licenses/LICENSE-2.0
-#
-# Unless required by applicable law or agreed to in writing,
-# software distributed under the License is distributed on an
-# "AS IS" BASIS, WITHOUT WARRANTIES OR CONDITIONS OF ANY
-# KIND, either express or implied. See the License for the
-# specific language governing permissions and limitations
-# under the License.
-
-import random
-
-vocab = [str(x) for x in range(100, 1000)]
-sw_train = open("sort.train.txt", "w")
-sw_test = open("sort.test.txt", "w")
-sw_valid = open("sort.valid.txt", "w")
-
-for i in range(1000000):
- seq = " ".join([vocab[random.randint(0, len(vocab) - 1)] for j in range(5)])
- k = i % 50
- if k == 0:
- sw_test.write(seq + "\n")
- elif k == 1:
- sw_valid.write(seq + "\n")
- else:
- sw_train.write(seq + "\n")
-
-sw_train.close()
-sw_test.close()
-sw_valid.close()
diff --git a/example/bi-lstm-sort/infer_sort.py b/example/bi-lstm-sort/infer_sort.py
deleted file mode 100644
index f81c6c0ec62..00000000000
--- a/example/bi-lstm-sort/infer_sort.py
+++ /dev/null
@@ -1,80 +0,0 @@
-# Licensed to the Apache Software Foundation (ASF) under one
-# or more contributor license agreements. See the NOTICE file
-# distributed with this work for additional information
-# regarding copyright ownership. The ASF licenses this file
-# to you under the Apache License, Version 2.0 (the
-# "License"); you may not use this file except in compliance
-# with the License. You may obtain a copy of the License at
-#
-# http://www.apache.org/licenses/LICENSE-2.0
-#
-# Unless required by applicable law or agreed to in writing,
-# software distributed under the License is distributed on an
-# "AS IS" BASIS, WITHOUT WARRANTIES OR CONDITIONS OF ANY
-# KIND, either express or implied. See the License for the
-# specific language governing permissions and limitations
-# under the License.
-
-# pylint: disable=C0111,too-many-arguments,too-many-instance-attributes,too-many-locals,redefined-outer-name,fixme
-# pylint: disable=superfluous-parens, no-member, invalid-name
-import sys
-import os
-import argparse
-import numpy as np
-import mxnet as mx
-
-from sort_io import BucketSentenceIter, default_build_vocab
-from rnn_model import BiLSTMInferenceModel
-
-TRAIN_FILE = "sort.train.txt"
-TEST_FILE = "sort.test.txt"
-VALID_FILE = "sort.valid.txt"
-DATA_DIR = os.path.join(os.getcwd(), "data")
-SEQ_LEN = 5
-
-def MakeInput(char, vocab, arr):
- idx = vocab[char]
- tmp = np.zeros((1,))
- tmp[0] = idx
- arr[:] = tmp
-
-def main():
- tks = sys.argv[1:]
- assert len(tks) >= 5, "Please provide 5 numbers for sorting as sequence length is 5"
- batch_size = 1
- buckets = []
- num_hidden = 300
- num_embed = 512
- num_lstm_layer = 2
-
- num_epoch = 1
- learning_rate = 0.1
- momentum = 0.9
-
- contexts = [mx.context.cpu(i) for i in range(1)]
-
- vocab = default_build_vocab(os.path.join(DATA_DIR, TRAIN_FILE))
- rvocab = {}
- for k, v in vocab.items():
- rvocab[v] = k
-
- _, arg_params, __ = mx.model.load_checkpoint("sort", 1)
- for tk in tks:
- assert (tk in vocab), "{} not in range of numbers that the model trained for.".format(tk)
-
- model = BiLSTMInferenceModel(SEQ_LEN, len(vocab),
- num_hidden=num_hidden, num_embed=num_embed,
- num_label=len(vocab), arg_params=arg_params, ctx=contexts, dropout=0.0)
-
- data = np.zeros((1, len(tks)))
- for k in range(len(tks)):
- data[0][k] = vocab[tks[k]]
-
- data = mx.nd.array(data)
- prob = model.forward(data)
- for k in range(len(tks)):
- print(rvocab[np.argmax(prob, axis = 1)[k]])
-
-
-if __name__ == '__main__':
- sys.exit(main())
diff --git a/example/bi-lstm-sort/lstm.py b/example/bi-lstm-sort/lstm.py
deleted file mode 100644
index 362481dd09e..00000000000
--- a/example/bi-lstm-sort/lstm.py
+++ /dev/null
@@ -1,175 +0,0 @@
-# Licensed to the Apache Software Foundation (ASF) under one
-# or more contributor license agreements. See the NOTICE file
-# distributed with this work for additional information
-# regarding copyright ownership. The ASF licenses this file
-# to you under the Apache License, Version 2.0 (the
-# "License"); you may not use this file except in compliance
-# with the License. You may obtain a copy of the License at
-#
-# http://www.apache.org/licenses/LICENSE-2.0
-#
-# Unless required by applicable law or agreed to in writing,
-# software distributed under the License is distributed on an
-# "AS IS" BASIS, WITHOUT WARRANTIES OR CONDITIONS OF ANY
-# KIND, either express or implied. See the License for the
-# specific language governing permissions and limitations
-# under the License.
-
-# pylint:skip-file
-import sys
-import mxnet as mx
-import numpy as np
-from collections import namedtuple
-import time
-import math
-LSTMState = namedtuple("LSTMState", ["c", "h"])
-LSTMParam = namedtuple("LSTMParam", ["i2h_weight", "i2h_bias",
- "h2h_weight", "h2h_bias"])
-LSTMModel = namedtuple("LSTMModel", ["rnn_exec", "symbol",
- "init_states", "last_states", "forward_state", "backward_state",
- "seq_data", "seq_labels", "seq_outputs",
- "param_blocks"])
-
-def lstm(num_hidden, indata, prev_state, param, seqidx, layeridx, dropout=0.):
- """LSTM Cell symbol"""
- if dropout > 0.:
- indata = mx.sym.Dropout(data=indata, p=dropout)
- i2h = mx.sym.FullyConnected(data=indata,
- weight=param.i2h_weight,
- bias=param.i2h_bias,
- num_hidden=num_hidden * 4,
- name="t%d_l%d_i2h" % (seqidx, layeridx))
- h2h = mx.sym.FullyConnected(data=prev_state.h,
- weight=param.h2h_weight,
- bias=param.h2h_bias,
- num_hidden=num_hidden * 4,
- name="t%d_l%d_h2h" % (seqidx, layeridx))
- gates = i2h + h2h
- slice_gates = mx.sym.SliceChannel(gates, num_outputs=4,
- name="t%d_l%d_slice" % (seqidx, layeridx))
- in_gate = mx.sym.Activation(slice_gates[0], act_type="sigmoid")
- in_transform = mx.sym.Activation(slice_gates[1], act_type="tanh")
- forget_gate = mx.sym.Activation(slice_gates[2], act_type="sigmoid")
- out_gate = mx.sym.Activation(slice_gates[3], act_type="sigmoid")
- next_c = (forget_gate * prev_state.c) + (in_gate * in_transform)
- next_h = out_gate * mx.sym.Activation(next_c, act_type="tanh")
- return LSTMState(c=next_c, h=next_h)
-
-
-def bi_lstm_unroll(seq_len, input_size,
- num_hidden, num_embed, num_label, dropout=0.):
-
- embed_weight = mx.sym.Variable("embed_weight")
- cls_weight = mx.sym.Variable("cls_weight")
- cls_bias = mx.sym.Variable("cls_bias")
- last_states = []
- last_states.append(LSTMState(c = mx.sym.Variable("l0_init_c"), h = mx.sym.Variable("l0_init_h")))
- last_states.append(LSTMState(c = mx.sym.Variable("l1_init_c"), h = mx.sym.Variable("l1_init_h")))
- forward_param = LSTMParam(i2h_weight=mx.sym.Variable("l0_i2h_weight"),
- i2h_bias=mx.sym.Variable("l0_i2h_bias"),
- h2h_weight=mx.sym.Variable("l0_h2h_weight"),
- h2h_bias=mx.sym.Variable("l0_h2h_bias"))
- backward_param = LSTMParam(i2h_weight=mx.sym.Variable("l1_i2h_weight"),
- i2h_bias=mx.sym.Variable("l1_i2h_bias"),
- h2h_weight=mx.sym.Variable("l1_h2h_weight"),
- h2h_bias=mx.sym.Variable("l1_h2h_bias"))
-
- # embeding layer
- data = mx.sym.Variable('data')
- label = mx.sym.Variable('softmax_label')
- embed = mx.sym.Embedding(data=data, input_dim=input_size,
- weight=embed_weight, output_dim=num_embed, name='embed')
- wordvec = mx.sym.SliceChannel(data=embed, num_outputs=seq_len, squeeze_axis=1)
-
- forward_hidden = []
- for seqidx in range(seq_len):
- hidden = wordvec[seqidx]
- next_state = lstm(num_hidden, indata=hidden,
- prev_state=last_states[0],
- param=forward_param,
- seqidx=seqidx, layeridx=0, dropout=dropout)
- hidden = next_state.h
- last_states[0] = next_state
- forward_hidden.append(hidden)
-
- backward_hidden = []
- for seqidx in range(seq_len):
- k = seq_len - seqidx - 1
- hidden = wordvec[k]
- next_state = lstm(num_hidden, indata=hidden,
- prev_state=last_states[1],
- param=backward_param,
- seqidx=k, layeridx=1,dropout=dropout)
- hidden = next_state.h
- last_states[1] = next_state
- backward_hidden.insert(0, hidden)
-
- hidden_all = []
- for i in range(seq_len):
- hidden_all.append(mx.sym.Concat(*[forward_hidden[i], backward_hidden[i]], dim=1))
-
- hidden_concat = mx.sym.Concat(*hidden_all, dim=0)
- pred = mx.sym.FullyConnected(data=hidden_concat, num_hidden=num_label,
- weight=cls_weight, bias=cls_bias, name='pred')
-
- label = mx.sym.transpose(data=label)
- label = mx.sym.Reshape(data=label, target_shape=(0,))
- sm = mx.sym.SoftmaxOutput(data=pred, label=label, name='softmax')
-
- return sm
-
-
-def bi_lstm_inference_symbol(input_size, seq_len,
- num_hidden, num_embed, num_label, dropout=0.):
- seqidx = 0
- embed_weight=mx.sym.Variable("embed_weight")
- cls_weight = mx.sym.Variable("cls_weight")
- cls_bias = mx.sym.Variable("cls_bias")
- last_states = [LSTMState(c = mx.sym.Variable("l0_init_c"), h = mx.sym.Variable("l0_init_h")),
- LSTMState(c = mx.sym.Variable("l1_init_c"), h = mx.sym.Variable("l1_init_h"))]
- forward_param = LSTMParam(i2h_weight=mx.sym.Variable("l0_i2h_weight"),
- i2h_bias=mx.sym.Variable("l0_i2h_bias"),
- h2h_weight=mx.sym.Variable("l0_h2h_weight"),
- h2h_bias=mx.sym.Variable("l0_h2h_bias"))
- backward_param = LSTMParam(i2h_weight=mx.sym.Variable("l1_i2h_weight"),
- i2h_bias=mx.sym.Variable("l1_i2h_bias"),
- h2h_weight=mx.sym.Variable("l1_h2h_weight"),
- h2h_bias=mx.sym.Variable("l1_h2h_bias"))
- data = mx.sym.Variable("data")
- embed = mx.sym.Embedding(data=data, input_dim=input_size,
- weight=embed_weight, output_dim=num_embed, name='embed')
- wordvec = mx.sym.SliceChannel(data=embed, num_outputs=seq_len, squeeze_axis=1)
- forward_hidden = []
- for seqidx in range(seq_len):
- next_state = lstm(num_hidden, indata=wordvec[seqidx],
- prev_state=last_states[0],
- param=forward_param,
- seqidx=seqidx, layeridx=0, dropout=0.0)
- hidden = next_state.h
- last_states[0] = next_state
- forward_hidden.append(hidden)
-
- backward_hidden = []
- for seqidx in range(seq_len):
- k = seq_len - seqidx - 1
- next_state = lstm(num_hidden, indata=wordvec[k],
- prev_state=last_states[1],
- param=backward_param,
- seqidx=k, layeridx=1, dropout=0.0)
- hidden = next_state.h
- last_states[1] = next_state
- backward_hidden.insert(0, hidden)
-
- hidden_all = []
- for i in range(seq_len):
- hidden_all.append(mx.sym.Concat(*[forward_hidden[i], backward_hidden[i]], dim=1))
- hidden_concat = mx.sym.Concat(*hidden_all, dim=0)
- fc = mx.sym.FullyConnected(data=hidden_concat, num_hidden=num_label,
- weight=cls_weight, bias=cls_bias, name='pred')
- sm = mx.sym.SoftmaxOutput(data=fc, name='softmax')
- output = [sm]
- for state in last_states:
- output.append(state.c)
- output.append(state.h)
- return mx.sym.Group(output)
-
diff --git a/example/bi-lstm-sort/lstm_sort.py b/example/bi-lstm-sort/lstm_sort.py
deleted file mode 100644
index 3d7090a9a35..00000000000
--- a/example/bi-lstm-sort/lstm_sort.py
+++ /dev/null
@@ -1,142 +0,0 @@
-# Licensed to the Apache Software Foundation (ASF) under one
-# or more contributor license agreements. See the NOTICE file
-# distributed with this work for additional information
-# regarding copyright ownership. The ASF licenses this file
-# to you under the Apache License, Version 2.0 (the
-# "License"); you may not use this file except in compliance
-# with the License. You may obtain a copy of the License at
-#
-# http://www.apache.org/licenses/LICENSE-2.0
-#
-# Unless required by applicable law or agreed to in writing,
-# software distributed under the License is distributed on an
-# "AS IS" BASIS, WITHOUT WARRANTIES OR CONDITIONS OF ANY
-# KIND, either express or implied. See the License for the
-# specific language governing permissions and limitations
-# under the License.
-
-# pylint: disable=C0111,too-many-arguments,too-many-instance-attributes,too-many-locals,redefined-outer-name,fixme
-# pylint: disable=superfluous-parens, no-member, invalid-name
-import argparse
-import errno
-import logging
-import os
-import random
-import sys
-
-import numpy as np
-
-import mxnet as mx
-from lstm import bi_lstm_unroll
-from sort_io import BucketSentenceIter, default_build_vocab
-
-head = '%(asctime)-15s %(message)s'
-logging.basicConfig(level=logging.DEBUG, format=head)
-
-TRAIN_FILE = "sort.train.txt"
-TEST_FILE = "sort.test.txt"
-VALID_FILE = "sort.valid.txt"
-DATA_DIR = os.path.join(os.getcwd(), "data")
-SEQ_LEN = 5
-
-
-def gen_data(seq_len, start_range, end_range):
- if not os.path.exists(DATA_DIR):
- try:
- logging.info('create directory %s', DATA_DIR)
- os.makedirs(DATA_DIR)
- except OSError as exc:
- if exc.errno != errno.EEXIST:
- raise OSError('failed to create ' + DATA_DIR)
- vocab = [str(x) for x in range(start_range, end_range)]
- sw_train = open(os.path.join(DATA_DIR, TRAIN_FILE), "w")
- sw_test = open(os.path.join(DATA_DIR, TEST_FILE), "w")
- sw_valid = open(os.path.join(DATA_DIR, VALID_FILE), "w")
-
- for i in range(1000000):
- seq = " ".join([vocab[random.randint(0, len(vocab) - 1)] for j in range(seq_len)])
- k = i % 50
- if k == 0:
- sw_test.write(seq + "\n")
- elif k == 1:
- sw_valid.write(seq + "\n")
- else:
- sw_train.write(seq + "\n")
-
- sw_train.close()
- sw_test.close()
-
-def parse_args():
- parser = argparse.ArgumentParser(description="Parse args for lstm_sort example",
- formatter_class=argparse.ArgumentDefaultsHelpFormatter)
- parser.add_argument('--start-range', type=int, default=100,
- help='starting number of the range')
- parser.add_argument('--end-range', type=int, default=1000,
- help='Ending number of the range')
- parser.add_argument('--cpu', action='store_true',
- help='To use CPU for training')
- return parser.parse_args()
-
-
-def Perplexity(label, pred):
- label = label.T.reshape((-1,))
- loss = 0.
- for i in range(pred.shape[0]):
- loss += -np.log(max(1e-10, pred[i][int(label[i])]))
- return np.exp(loss / label.size)
-
-def main():
- args = parse_args()
- gen_data(SEQ_LEN, args.start_range, args.end_range)
- batch_size = 100
- buckets = []
- num_hidden = 300
- num_embed = 512
- num_lstm_layer = 2
-
- num_epoch = 1
- learning_rate = 0.1
- momentum = 0.9
-
- if args.cpu:
- contexts = [mx.context.cpu(i) for i in range(1)]
- else:
- contexts = [mx.context.gpu(i) for i in range(1)]
-
- vocab = default_build_vocab(os.path.join(DATA_DIR, TRAIN_FILE))
-
- def sym_gen(seq_len):
- return bi_lstm_unroll(seq_len, len(vocab),
- num_hidden=num_hidden, num_embed=num_embed,
- num_label=len(vocab))
-
- init_c = [('l%d_init_c'%l, (batch_size, num_hidden)) for l in range(num_lstm_layer)]
- init_h = [('l%d_init_h'%l, (batch_size, num_hidden)) for l in range(num_lstm_layer)]
- init_states = init_c + init_h
-
- data_train = BucketSentenceIter(os.path.join(DATA_DIR, TRAIN_FILE), vocab,
- buckets, batch_size, init_states)
- data_val = BucketSentenceIter(os.path.join(DATA_DIR, VALID_FILE), vocab,
- buckets, batch_size, init_states)
-
- if len(buckets) == 1:
- symbol = sym_gen(buckets[0])
- else:
- symbol = sym_gen
-
- model = mx.model.FeedForward(ctx=contexts,
- symbol=symbol,
- num_epoch=num_epoch,
- learning_rate=learning_rate,
- momentum=momentum,
- wd=0.00001,
- initializer=mx.init.Xavier(factor_type="in", magnitude=2.34))
-
- model.fit(X=data_train, eval_data=data_val,
- eval_metric = mx.metric.np(Perplexity),
- batch_end_callback=mx.callback.Speedometer(batch_size, 50),)
-
- model.save("sort")
-
-if __name__ == '__main__':
- sys.exit(main())
diff --git a/example/bi-lstm-sort/rnn_model.py b/example/bi-lstm-sort/rnn_model.py
deleted file mode 100644
index 1079e90991b..00000000000
--- a/example/bi-lstm-sort/rnn_model.py
+++ /dev/null
@@ -1,73 +0,0 @@
-# Licensed to the Apache Software Foundation (ASF) under one
-# or more contributor license agreements. See the NOTICE file
-# distributed with this work for additional information
-# regarding copyright ownership. The ASF licenses this file
-# to you under the Apache License, Version 2.0 (the
-# "License"); you may not use this file except in compliance
-# with the License. You may obtain a copy of the License at
-#
-# http://www.apache.org/licenses/LICENSE-2.0
-#
-# Unless required by applicable law or agreed to in writing,
-# software distributed under the License is distributed on an
-# "AS IS" BASIS, WITHOUT WARRANTIES OR CONDITIONS OF ANY
-# KIND, either express or implied. See the License for the
-# specific language governing permissions and limitations
-# under the License.
-
-# pylint: disable=C0111,too-many-arguments,too-many-instance-attributes,too-many-locals,redefined-outer-name,fixme
-# pylint: disable=superfluous-parens, no-member, invalid-name
-import sys
-import numpy as np
-import mxnet as mx
-
-from lstm import LSTMState, LSTMParam, lstm, bi_lstm_inference_symbol
-
-class BiLSTMInferenceModel(object):
- def __init__(self,
- seq_len,
- input_size,
- num_hidden,
- num_embed,
- num_label,
- arg_params,
- ctx=mx.cpu(),
- dropout=0.):
- self.sym = bi_lstm_inference_symbol(input_size, seq_len,
- num_hidden,
- num_embed,
- num_label,
- dropout)
- batch_size = 1
- init_c = [('l%d_init_c'%l, (batch_size, num_hidden)) for l in range(2)]
- init_h = [('l%d_init_h'%l, (batch_size, num_hidden)) for l in range(2)]
-
- data_shape = [("data", (batch_size, seq_len, ))]
-
- input_shapes = dict(init_c + init_h + data_shape)
- self.executor = self.sym.simple_bind(ctx=mx.cpu(), **input_shapes)
-
- for key in self.executor.arg_dict.keys():
- if key in arg_params:
- arg_params[key].copyto(self.executor.arg_dict[key])
-
- state_name = []
- for i in range(2):
- state_name.append("l%d_init_c" % i)
- state_name.append("l%d_init_h" % i)
-
- self.states_dict = dict(zip(state_name, self.executor.outputs[1:]))
- self.input_arr = mx.nd.zeros(data_shape[0][1])
-
- def forward(self, input_data, new_seq=False):
- if new_seq == True:
- for key in self.states_dict.keys():
- self.executor.arg_dict[key][:] = 0.
- input_data.copyto(self.executor.arg_dict["data"])
- self.executor.forward()
- for key in self.states_dict.keys():
- self.states_dict[key].copyto(self.executor.arg_dict[key])
- prob = self.executor.outputs[0].asnumpy()
- return prob
-
-
diff --git a/example/bi-lstm-sort/sort_io.py b/example/bi-lstm-sort/sort_io.py
deleted file mode 100644
index 853d0ee87db..00000000000
--- a/example/bi-lstm-sort/sort_io.py
+++ /dev/null
@@ -1,255 +0,0 @@
-# Licensed to the Apache Software Foundation (ASF) under one
-# or more contributor license agreements. See the NOTICE file
-# distributed with this work for additional information
-# regarding copyright ownership. The ASF licenses this file
-# to you under the Apache License, Version 2.0 (the
-# "License"); you may not use this file except in compliance
-# with the License. You may obtain a copy of the License at
-#
-# http://www.apache.org/licenses/LICENSE-2.0
-#
-# Unless required by applicable law or agreed to in writing,
-# software distributed under the License is distributed on an
-# "AS IS" BASIS, WITHOUT WARRANTIES OR CONDITIONS OF ANY
-# KIND, either express or implied. See the License for the
-# specific language governing permissions and limitations
-# under the License.
-
-# pylint: disable=C0111,too-many-arguments,too-many-instance-attributes,too-many-locals,redefined-outer-name,fixme
-# pylint: disable=superfluous-parens, no-member, invalid-name
-from __future__ import print_function
-import sys
-import numpy as np
-import mxnet as mx
-
-# The interface of a data iter that works for bucketing
-#
-# DataIter
-# - default_bucket_key: the bucket key for the default symbol.
-#
-# DataBatch
-# - provide_data: same as DataIter, but specific to this batch
-# - provide_label: same as DataIter, but specific to this batch
-# - bucket_key: the key for the bucket that should be used for this batch
-
-def default_read_content(path):
- with open(path) as ins:
- content = ins.read()
- content = content.replace('\n', ' <eos> ').replace('. ', ' <eos> ')
- return content
-
-def default_build_vocab(path):
- content = default_read_content(path)
- content = content.split(' ')
-
- words = set([x for x in content if len(x) > 0])
- words = [x for x in words]
- words = sorted(words)
- the_vocab = {}
- idx = 1 # 0 is left for zero-padding
- the_vocab[' '] = 0 # put a dummy element here so that len(vocab) is correct
- for word in words:
- if len(word) == 0:
- continue
- if not word in the_vocab:
- the_vocab[word] = idx
- idx += 1
- return the_vocab
-
-def default_text2id(sentence, the_vocab):
- words = sentence.split(' ')
- words = [the_vocab[w] for w in words if len(w) > 0]
- return words
-
-def default_gen_buckets(sentences, batch_size, the_vocab):
- len_dict = {}
- max_len = -1
- for sentence in sentences:
- words = default_text2id(sentence, the_vocab)
- lw = len(words)
- if lw == 0:
- continue
- if lw > max_len:
- max_len = lw
- if lw in len_dict:
- len_dict[lw] += 1
- else:
- len_dict[lw] = 1
- print(len_dict)
-
- tl = 0
- buckets = []
- for l, n in len_dict.items(): # TODO: There are better heuristic ways to do this
- if n + tl >= batch_size:
- buckets.append(l)
- tl = 0
- else:
- tl += n
- if tl > 0:
- buckets.append(max_len)
- return buckets
-
-
-class SimpleBatch(object):
- def __init__(self, data_names, data, label_names, label, bucket_key):
- self.data = data
- self.label = label
- self.data_names = data_names
- self.label_names = label_names
- self.bucket_key = bucket_key
-
- self.pad = 0
- self.index = None # TODO: what is index?
-
- @property
- def provide_data(self):
- return [(n, x.shape) for n, x in zip(self.data_names, self.data)]
-
- @property
- def provide_label(self):
- return [(n, x.shape) for n, x in zip(self.label_names, self.label)]
-
-class DummyIter(mx.io.DataIter):
- "A dummy iterator that always return the same batch, used for speed testing"
- def __init__(self, real_iter):
- super(DummyIter, self).__init__()
- self.real_iter = real_iter
- self.provide_data = real_iter.provide_data
- self.provide_label = real_iter.provide_label
- self.batch_size = real_iter.batch_size
-
- for batch in real_iter:
- self.the_batch = batch
- break
-
- def __iter__(self):
- return self
-
- def next(self):
- return self.the_batch
-
-class BucketSentenceIter(mx.io.DataIter):
- def __init__(self, path, vocab, buckets, batch_size,
- init_states, data_name='data', label_name='label',
- seperate_char=' <eos> ', text2id=None, read_content=None):
- super(BucketSentenceIter, self).__init__()
-
- if text2id is None:
- self.text2id = default_text2id
- else:
- self.text2id = text2id
- if read_content is None:
- self.read_content = default_read_content
- else:
- self.read_content = read_content
- content = self.read_content(path)
- sentences = content.split(seperate_char)
-
- if len(buckets) == 0:
- buckets = default_gen_buckets(sentences, batch_size, vocab)
- print(buckets)
- self.vocab_size = len(vocab)
- self.data_name = data_name
- self.label_name = label_name
-
- buckets.sort()
- self.buckets = buckets
- self.data = [[] for _ in buckets]
-
- # pre-allocate with the largest bucket for better memory sharing
- self.default_bucket_key = max(buckets)
-
- for sentence in sentences:
- sentence = self.text2id(sentence, vocab)
- if len(sentence) == 0:
- continue
- for i, bkt in enumerate(buckets):
- if bkt >= len(sentence):
- self.data[i].append(sentence)
- break
- # we just ignore the sentence it is longer than the maximum
- # bucket size here
-
- # convert data into ndarrays for better speed during training
- data = [np.zeros((len(x), buckets[i])) for i, x in enumerate(self.data)]
- for i_bucket in range(len(self.buckets)):
- for j in range(len(self.data[i_bucket])):
- sentence = self.data[i_bucket][j]
- data[i_bucket][j, :len(sentence)] = sentence
- self.data = data
-
- # Get the size of each bucket, so that we could sample
- # uniformly from the bucket
- bucket_sizes = [len(x) for x in self.data]
-
- print("Summary of dataset ==================")
- for bkt, size in zip(buckets, bucket_sizes):
- print("bucket of len %3d : %d samples" % (bkt, size))
-
- self.batch_size = batch_size
- self.make_data_iter_plan()
-
- self.init_states = init_states
- self.init_state_arrays = [mx.nd.zeros(x[1]) for x in init_states]
-
- self.provide_data = [('data', (batch_size, self.default_bucket_key))] + init_states
- self.provide_label = [('softmax_label', (self.batch_size, self.default_bucket_key))]
-
- def make_data_iter_plan(self):
- "make a random data iteration plan"
- # truncate each bucket into multiple of batch-size
- bucket_n_batches = []
- for i in range(len(self.data)):
- bucket_n_batches.append(len(self.data[i]) / self.batch_size)
- self.data[i] = self.data[i][:int(bucket_n_batches[i]*self.batch_size)]
-
- bucket_plan = np.hstack([np.zeros(n, int)+i for i, n in enumerate(bucket_n_batches)])
- np.random.shuffle(bucket_plan)
-
- bucket_idx_all = [np.random.permutation(len(x)) for x in self.data]
-
- self.bucket_plan = bucket_plan
- self.bucket_idx_all = bucket_idx_all
- self.bucket_curr_idx = [0 for x in self.data]
-
- self.data_buffer = []
- self.label_buffer = []
- for i_bucket in range(len(self.data)):
- data = np.zeros((self.batch_size, self.buckets[i_bucket]))
- label = np.zeros((self.batch_size, self.buckets[i_bucket]))
- self.data_buffer.append(data)
- self.label_buffer.append(label)
-
- def __iter__(self):
- init_state_names = [x[0] for x in self.init_states]
-
- for i_bucket in self.bucket_plan:
- data = self.data_buffer[i_bucket]
- label = self.label_buffer[i_bucket]
-
- i_idx = self.bucket_curr_idx[i_bucket]
- idx = self.bucket_idx_all[i_bucket][i_idx:i_idx+self.batch_size]
- self.bucket_curr_idx[i_bucket] += self.batch_size
- data[:] = self.data[i_bucket][idx]
-
- for k in range(len(data)):
- label[k] = sorted(data[k])
- #count = len(data[k]) / 2
- #for j in range(count):
- # data[j+count] = data[j]
-
- #label[:, :-1] = data[:, 1:]
- #label[:, -1] = 0
-
- data_all = [mx.nd.array(data)] + self.init_state_arrays
- label_all = [mx.nd.array(label)]
- data_names = ['data'] + init_state_names
- label_names = ['softmax_label']
-
- data_batch = SimpleBatch(data_names, data_all, label_names, label_all,
- self.buckets[i_bucket])
-
- yield data_batch
-
- def reset(self):
- self.bucket_curr_idx = [0 for x in self.data]
----------------------------------------------------------------
This is an automated message from the Apache Git Service.
To respond to the message, please log on GitHub and use the
URL above to go to the specific comment.
For queries about this service, please contact Infrastructure at:
users@infra.apache.org
With regards,
Apache Git Services