You are viewing a plain text version of this content. The canonical link for it is here.
Posted to commits@tvm.apache.org by GitBox <gi...@apache.org> on 2020/07/26 14:48:07 UTC
[GitHub] [incubator-tvm] Wheest opened a new pull request #6137: Better grouped convolution for CPU targets
Wheest opened a new pull request #6137:
URL: https://github.com/apache/incubator-tvm/pull/6137
This pull request is to replace the current grouped direct convolution algorithm on x86 and Arm targets, with the faster Grouped Spatial Pack Convolutions (GSPC) algorithm.
Here's a performance comparison graph for ResNet34 on a single big core of a Hikey 970 as we increase the number of groups:
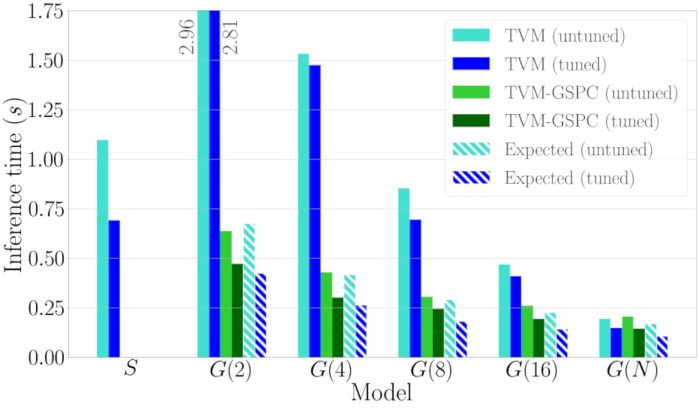
Note that in the untuned case the current depthwise convolution outperforms GSPC, thus I have omitted it from the pull request.
This is my first proper full request to TVM, so I may be have some issues I haven't spotted, or style problems.
In short, this commit adds identical GSPC compute definitions and schedules for x86 and arm_cpu targets for grouped convolutions, as well as updating the Relay operator strategy for each.
----------------------------------------------------------------
This is an automated message from the Apache Git Service.
To respond to the message, please log on to GitHub and use the
URL above to go to the specific comment.
For queries about this service, please contact Infrastructure at:
users@infra.apache.org
[GitHub] [incubator-tvm] Wheest commented on a change in pull request #6137: Better grouped convolution for CPU targets
Posted by GitBox <gi...@apache.org>.
Wheest commented on a change in pull request #6137:
URL: https://github.com/apache/incubator-tvm/pull/6137#discussion_r479231672
##########
File path: topi/python/topi/arm_cpu/group_conv2d.py
##########
@@ -0,0 +1,310 @@
+import tvm
+from tvm import autotvm
+from tvm import te
+from ..util import get_const_tuple
+from ..nn.pad import pad
+from .. import tag
+
+from ..nn.conv2d import group_conv2d_nchw
+from ..nn.util import infer_pad
+from ..nn.conv2d import _get_workload as _get_conv2d_workload
+
+from tvm.autotvm.task.space import SplitEntity, OtherOptionEntity
+
+
+def group_conv2d_nchw(data, kernel, strides, padding, dilation, groups,
+ out_dtype):
+ """Compute group_conv2d with NCHW layout"""
+ return group_conv2d_nchw_spatial_pack(data, kernel, strides, padding,
+ dilation, groups, out_dtype)
+
+
+def schedule_group_conv2d_nchw(outs):
+ """Compute group_conv2d with NCHW layout"""
+ return schedule_group_conv2d_nchwc(outs)
+
+
+def _get_default_config(cfg, data, kernel, strides, padding, groups, out_dtype,
+ layout='NCHW'):
+ """
+ Get default schedule config for the workload
+ """
+ static_data_shape = []
+ for dim in get_const_tuple(data.shape):
+ if isinstance(dim, tvm.tir.Var):
+ static_data_shape.append(1)
+ else:
+ static_data_shape.append(dim)
+ data = te.placeholder(static_data_shape, dtype=data.dtype)
+
+ wkl = _get_conv2d_workload(data, kernel, strides, padding, out_dtype,
+ layout)
+ _fallback_schedule(cfg, wkl)
+
+
+def _fallback_schedule(cfg, wkl):
+ simd_width = 4 # assume ARM SIMD Width is 4
+ HPAD, WPAD = wkl.hpad, wkl.wpad
+ HSTR, WSTR = wkl.hstride, wkl.wstride
+ out_width = (wkl.width + 2 * WPAD - wkl.wkernel) // WSTR + 1
+ G = wkl.groups
+ KPG = wkl.out_filter // G
+ CPG = wkl.in_filter // G
+ oc_bn = 1
+
+ for bn in range(simd_width, 0, -1):
+ if KPG % bn == 0:
+ oc_bn = bn
+ break
+
+ ic_bn = 1
+ for bn in range(oc_bn, 0, -1):
+ if CPG % bn == 0:
+ ic_bn = bn
+ break
+
+ reg_n = 1
+ for n in range(31, 0, -1):
+ if out_width % n == 0:
+ reg_n = n
+ break
+
+ cfg["tile_ic"] = SplitEntity([wkl.in_filter // ic_bn, ic_bn])
+ cfg["tile_oc"] = SplitEntity([wkl.out_filter // oc_bn, oc_bn])
+ cfg["tile_ow"] = SplitEntity([out_width // reg_n, reg_n])
+ cfg["unroll_kw"] = OtherOptionEntity(False)
+
+
+@autotvm.register_topi_compute("group_conv2d_nchw.arm_cpu")
+def group_conv2d_nchw_spatial_pack(cfg, data, kernel, strides, padding,
+ dilation, groups, out_dtype='float32'):
+ assert isinstance(dilation, int) or len(dilation) == 2
+ if isinstance(dilation, int):
+ dilation_h, dilation_w = dilation, dilation
+ else:
+ dilation_h, dilation_w = dilation
+
+ assert isinstance(padding, int) or len(padding) == 2 or len(padding) == 4
+ if isinstance(padding, int):
+ HPAD, WPAD = padding, padding
+ elif len(padding) == 2:
+ HPAD, WPAD = padding
+ else:
+ HPAD, _, WPAD, _ = padding
Review comment:
I've got a suggestion for extending _get_workload. In the new commit `505c127` I've added a 2nd Workload, `Workload_asym`. `_get_workload` takes an optional argument `asymmetric_pad` which means it will return this workload instead. Ideally the old conv2d `Workload` would be deprecated so that all conv2d workloads support asymmetric padding.
----------------------------------------------------------------
This is an automated message from the Apache Git Service.
To respond to the message, please log on to GitHub and use the
URL above to go to the specific comment.
For queries about this service, please contact Infrastructure at:
users@infra.apache.org
[GitHub] [incubator-tvm] FrozenGene commented on a change in pull request #6137: Better grouped convolution for CPU targets
Posted by GitBox <gi...@apache.org>.
FrozenGene commented on a change in pull request #6137:
URL: https://github.com/apache/incubator-tvm/pull/6137#discussion_r477060409
##########
File path: topi/python/topi/arm_cpu/group_conv2d.py
##########
@@ -0,0 +1,310 @@
+import tvm
+from tvm import autotvm
+from tvm import te
+from ..util import get_const_tuple
+from ..nn.pad import pad
+from .. import tag
+
+from ..nn.conv2d import group_conv2d_nchw
+from ..nn.util import infer_pad
+from ..nn.conv2d import _get_workload as _get_conv2d_workload
+
+from tvm.autotvm.task.space import SplitEntity, OtherOptionEntity
+
+
+def group_conv2d_nchw(data, kernel, strides, padding, dilation, groups,
+ out_dtype):
+ """Compute group_conv2d with NCHW layout"""
+ return group_conv2d_nchw_spatial_pack(data, kernel, strides, padding,
+ dilation, groups, out_dtype)
+
+
+def schedule_group_conv2d_nchw(outs):
+ """Compute group_conv2d with NCHW layout"""
+ return schedule_group_conv2d_nchwc(outs)
+
+
+def _get_default_config(cfg, data, kernel, strides, padding, groups, out_dtype,
+ layout='NCHW'):
+ """
+ Get default schedule config for the workload
+ """
+ static_data_shape = []
+ for dim in get_const_tuple(data.shape):
+ if isinstance(dim, tvm.tir.Var):
+ static_data_shape.append(1)
+ else:
+ static_data_shape.append(dim)
+ data = te.placeholder(static_data_shape, dtype=data.dtype)
+
+ wkl = _get_conv2d_workload(data, kernel, strides, padding, out_dtype,
+ layout)
+ _fallback_schedule(cfg, wkl)
+
+
+def _fallback_schedule(cfg, wkl):
+ simd_width = 4 # assume ARM SIMD Width is 4
+ HPAD, WPAD = wkl.hpad, wkl.wpad
+ HSTR, WSTR = wkl.hstride, wkl.wstride
+ out_width = (wkl.width + 2 * WPAD - wkl.wkernel) // WSTR + 1
+ G = wkl.groups
+ KPG = wkl.out_filter // G
+ CPG = wkl.in_filter // G
+ oc_bn = 1
+
+ for bn in range(simd_width, 0, -1):
+ if KPG % bn == 0:
+ oc_bn = bn
+ break
+
+ ic_bn = 1
+ for bn in range(oc_bn, 0, -1):
+ if CPG % bn == 0:
+ ic_bn = bn
+ break
+
+ reg_n = 1
+ for n in range(31, 0, -1):
+ if out_width % n == 0:
+ reg_n = n
+ break
+
+ cfg["tile_ic"] = SplitEntity([wkl.in_filter // ic_bn, ic_bn])
+ cfg["tile_oc"] = SplitEntity([wkl.out_filter // oc_bn, oc_bn])
+ cfg["tile_ow"] = SplitEntity([out_width // reg_n, reg_n])
+ cfg["unroll_kw"] = OtherOptionEntity(False)
+
+
+@autotvm.register_topi_compute("group_conv2d_nchw.arm_cpu")
+def group_conv2d_nchw_spatial_pack(cfg, data, kernel, strides, padding,
+ dilation, groups, out_dtype='float32'):
+ assert isinstance(dilation, int) or len(dilation) == 2
+ if isinstance(dilation, int):
+ dilation_h, dilation_w = dilation, dilation
+ else:
+ dilation_h, dilation_w = dilation
+
+ assert isinstance(padding, int) or len(padding) == 2 or len(padding) == 4
+ if isinstance(padding, int):
+ HPAD, WPAD = padding, padding
+ elif len(padding) == 2:
+ HPAD, WPAD = padding
+ else:
+ HPAD, _, WPAD, _ = padding
Review comment:
I think we should extend `_get_workload` function to support this, do you think so?
----------------------------------------------------------------
This is an automated message from the Apache Git Service.
To respond to the message, please log on to GitHub and use the
URL above to go to the specific comment.
For queries about this service, please contact Infrastructure at:
users@infra.apache.org
[GitHub] [incubator-tvm] FrozenGene commented on a change in pull request #6137: Better grouped convolution for CPU targets
Posted by GitBox <gi...@apache.org>.
FrozenGene commented on a change in pull request #6137:
URL: https://github.com/apache/incubator-tvm/pull/6137#discussion_r463851152
##########
File path: topi/python/topi/arm_cpu/group_conv2d.py
##########
@@ -0,0 +1,310 @@
+import tvm
+from tvm import autotvm
+from tvm import te
+from ..util import get_const_tuple
+from ..nn.pad import pad
+from .. import tag
+
+from ..nn.conv2d import group_conv2d_nchw
+from ..nn.util import infer_pad
+from ..nn.conv2d import _get_workload as _get_conv2d_workload
+
+from tvm.autotvm.task.space import SplitEntity, OtherOptionEntity
+
+
+def group_conv2d_nchw(data, kernel, strides, padding, dilation, groups,
+ out_dtype):
+ """Compute group_conv2d with NCHW layout"""
+ return group_conv2d_nchw_spatial_pack(data, kernel, strides, padding,
+ dilation, groups, out_dtype)
+
+
+def schedule_group_conv2d_nchw(outs):
+ """Compute group_conv2d with NCHW layout"""
+ return schedule_group_conv2d_nchwc(outs)
+
+
+def _get_default_config(cfg, data, kernel, strides, padding, groups, out_dtype,
+ layout='NCHW'):
+ """
+ Get default schedule config for the workload
+ """
+ static_data_shape = []
+ for dim in get_const_tuple(data.shape):
+ if isinstance(dim, tvm.tir.Var):
+ static_data_shape.append(1)
+ else:
+ static_data_shape.append(dim)
+ data = te.placeholder(static_data_shape, dtype=data.dtype)
+
+ wkl = _get_conv2d_workload(data, kernel, strides, padding, out_dtype,
+ layout)
+ _fallback_schedule(cfg, wkl)
+
+
+def _fallback_schedule(cfg, wkl):
+ simd_width = 4 # assume ARM SIMD Width is 4
+ HPAD, WPAD = wkl.hpad, wkl.wpad
+ HSTR, WSTR = wkl.hstride, wkl.wstride
+ out_width = (wkl.width + 2 * WPAD - wkl.wkernel) // WSTR + 1
+ G = wkl.groups
+ KPG = wkl.out_filter // G
+ CPG = wkl.in_filter // G
+ oc_bn = 1
+
+ for bn in range(simd_width, 0, -1):
+ if KPG % bn == 0:
+ oc_bn = bn
+ break
+
+ ic_bn = 1
+ for bn in range(oc_bn, 0, -1):
+ if CPG % bn == 0:
+ ic_bn = bn
+ break
+
+ reg_n = 1
+ for n in range(31, 0, -1):
+ if out_width % n == 0:
+ reg_n = n
+ break
+
+ cfg["tile_ic"] = SplitEntity([wkl.in_filter // ic_bn, ic_bn])
+ cfg["tile_oc"] = SplitEntity([wkl.out_filter // oc_bn, oc_bn])
+ cfg["tile_ow"] = SplitEntity([out_width // reg_n, reg_n])
+ cfg["unroll_kw"] = OtherOptionEntity(False)
+
+
+@autotvm.register_topi_compute("group_conv2d_nchw.arm_cpu")
+def group_conv2d_nchw_spatial_pack(cfg, data, kernel, strides, padding,
+ dilation, groups, out_dtype='float32'):
+ assert isinstance(dilation, int) or len(dilation) == 2
+ if isinstance(dilation, int):
+ dilation_h, dilation_w = dilation, dilation
+ else:
+ dilation_h, dilation_w = dilation
+
+ assert isinstance(padding, int) or len(padding) == 2 or len(padding) == 4
+ if isinstance(padding, int):
+ HPAD, WPAD = padding, padding
+ elif len(padding) == 2:
+ HPAD, WPAD = padding
+ else:
+ HPAD, _, WPAD, _ = padding
+
+ assert isinstance(strides, int) or len(strides) == 2
+ if isinstance(strides, int):
+ HSTR, WSTR = strides, strides
+ else:
+ HSTR, WSTR = strides
+
+ N, CI, IH, IW = get_const_tuple(data.shape)
+ CO, CIG, KH, KW = get_const_tuple(kernel.shape)
+
+ pad_height = IH + 2 * HPAD
+ pad_width = IW + 2 * WPAD
+
+ dilated_kernel_h = (KH - 1) * dilation_h + 1
+ dilated_kernel_w = (KW - 1) * dilation_w + 1
+ OH = (IH + 2 * HPAD - dilated_kernel_h) // HSTR + 1
+ OW = (IW + 2 * WPAD - dilated_kernel_w) // WSTR + 1
+
+ G = groups
+ KPG = CO // G
+ CPG = CI // G
+
+ cfg.define_split("tile_ic", CI, num_outputs=2)
+ cfg.define_split("tile_oc", CO, num_outputs=2)
+ cfg.define_split("tile_ow", OW, num_outputs=2, filter=lambda y: y.size[-1] <= 64)
+ cfg.define_knob("unroll_kw", [True, False])
+
+ # If no config was set, we can fallback to default config.
+ if cfg.is_fallback:
+ _get_default_config(cfg, te.placeholder((N, CI, IH, IW), dtype=data.dtype),
+ te.placeholder((N, CI // G, KH, KW),
+ dtype=kernel.dtype),
+ strides, padding, groups, out_dtype)
+
+ oc_bn = cfg['tile_oc'].size[-1]
+ ic_bn = cfg['tile_ic'].size[-1]
+ # pack data
+ DOPAD = (HPAD != 0 or WPAD != 0)
+ if DOPAD:
+ data_pad = pad(data, (0, 0, HPAD, WPAD), name="data_pad")
+ else:
+ data_pad = data
+
+ shape = (G, N, CPG // ic_bn,
+ pad_height, ic_bn, pad_width)
+
+ data_vec = te.compute(shape,
+ lambda g, n, C, h, c, w:
+ data_pad[n, C * ic_bn + c + CPG * g, h, w],
+ name='data_vec')
+
+ # pack kernel
+ shape = (G, KPG//oc_bn, CPG//ic_bn,
+ KH, KW, ic_bn, oc_bn)
+ kernel_vec = te.compute(shape,
+ lambda g, CO, CI, h, w, ci, co:
+ kernel[(CO * oc_bn + co + g * KPG),
+ CI * ic_bn + ci, h, w],
+ name='kernel_vec')
+
+ # convolution
+ oshape = (G, N, KPG//oc_bn,
+ OH, OW, oc_bn)
+ unpack_shape = (N, CO, OH, OW)
+
+ ic = te.reduce_axis((0, (CPG)), name='ic')
+ kh = te.reduce_axis((0, KH), name='kh')
+ kw = te.reduce_axis((0, KW), name='kw')
+ idxmod = tvm.tir.indexmod
+ idxdiv = tvm.tir.indexdiv
+
+ conv = te.compute(oshape, lambda g, n, oc_chunk, oh, ow, oc_block:
+ te.sum(data_vec[g, n, idxdiv(ic, ic_bn),
+ oh*HSTR+kh*dilation_h,
+ idxmod(ic, ic_bn),
+ ow*WSTR+kw*dilation_w].astype(out_dtype) *
+ kernel_vec[g, oc_chunk, idxdiv(ic, ic_bn),
+ kh, kw, idxmod(ic, ic_bn),
+ oc_block].astype(out_dtype),
+ axis=[ic, kh, kw]), name='conv')
+
+ unpack = te.compute(unpack_shape,
+ lambda n, c, h, w:
+ conv[idxdiv(c, KPG), n,
+ idxmod(idxdiv(c, oc_bn), (KPG // oc_bn)),
+ h, w,
+ idxmod(idxmod(c, oc_bn), KPG)]
+ .astype(out_dtype),
+ name='output_unpack',
+ tag='group_conv2d_nchw')
+ return unpack
+
+
+@autotvm.register_topi_schedule("group_conv2d_nchw.arm_cpu")
+def schedule_group_conv2d_nchwc(cfg, outs):
+ """Create schedule for tensors"""
+ s = te.create_schedule([x.op for x in outs])
+ scheduled_ops = []
+
+ def traverse(op):
+ """Traverse operators from computation graph"""
+ # inline all one-to-one-mapping operators except the last stage (output)
+ if tag.is_broadcast(op.tag):
+ if op not in s.outputs:
+ s[op].compute_inline()
+ for tensor in op.input_tensors:
+ if isinstance(tensor.op, tvm.te.ComputeOp) and tensor.op not in scheduled_ops:
+ traverse(tensor.op)
+
+ if 'group_conv2d_nchw' in op.tag:
+ output = op.output(0)
+
+ if "tile_ic" not in cfg:
+ return
+ conv_out = op.input_tensors[0]
+ kernel_vec = conv_out.op.input_tensors[1]
+ kernel = kernel_vec.op.input_tensors[0]
+ if isinstance(kernel.op, tvm.te.ComputeOp) and "dilate" in kernel.op.tag:
+ s[kernel].compute_inline()
+ data_vec = conv_out.op.input_tensors[0]
+ data = data_vec.op.input_tensors[0]
+ data_pad = None
+ if isinstance(data.op, tvm.te.ComputeOp) and "pad" in data.op.tag:
+ data_pad = data
+ data = data_pad.op.input_tensors[0]
+
+ _, c, h, w = get_const_tuple(data.shape)
+ _, x, kh, kw = get_const_tuple(kernel.shape)
+
+ args = [s, cfg, data, data_pad, data_vec, kernel_vec, conv_out,
+ output, outs[0]]
+ schedule_conv_sp_grouped(*args)
+
+ scheduled_ops.append(op)
+
+ traverse(outs[0].op)
+ return s
+
+
+def schedule_conv_sp_grouped(s, cfg, data, data_pad, data_vec, kernel_vec,
+ conv_out, output, last,
+ **kwargs):
+ # fetch schedule
+ ic_bn, oc_bn, reg_n, unroll_kw = (cfg["tile_ic"].size[-1], cfg["tile_oc"].size[-1],
+ cfg["tile_ow"].size[-1], cfg["unroll_kw"].val)
+
+ # no stride and padding info here
+ padding = infer_pad(data, data_pad)
+ HPAD, WPAD = padding
+ DOPAD = (HPAD != 0 or WPAD != 0)
+
+ A, W = data, kernel_vec
+ A0, A1 = data_pad, data_vec
+
+ # schedule data
+ if DOPAD:
+ s[A0].compute_inline()
+ groups, batch, ic_chunk, ih, ic_block, iw = s[A1].op.axis
Review comment:
Maybe we should have one compute_at for A1 at CC? Not only just parallel.
##########
File path: topi/python/topi/arm_cpu/group_conv2d.py
##########
@@ -0,0 +1,310 @@
+import tvm
+from tvm import autotvm
+from tvm import te
+from ..util import get_const_tuple
+from ..nn.pad import pad
+from .. import tag
+
+from ..nn.conv2d import group_conv2d_nchw
+from ..nn.util import infer_pad
+from ..nn.conv2d import _get_workload as _get_conv2d_workload
+
+from tvm.autotvm.task.space import SplitEntity, OtherOptionEntity
+
+
+def group_conv2d_nchw(data, kernel, strides, padding, dilation, groups,
+ out_dtype):
+ """Compute group_conv2d with NCHW layout"""
+ return group_conv2d_nchw_spatial_pack(data, kernel, strides, padding,
+ dilation, groups, out_dtype)
+
+
+def schedule_group_conv2d_nchw(outs):
+ """Compute group_conv2d with NCHW layout"""
+ return schedule_group_conv2d_nchwc(outs)
+
+
+def _get_default_config(cfg, data, kernel, strides, padding, groups, out_dtype,
+ layout='NCHW'):
+ """
+ Get default schedule config for the workload
+ """
+ static_data_shape = []
+ for dim in get_const_tuple(data.shape):
+ if isinstance(dim, tvm.tir.Var):
+ static_data_shape.append(1)
+ else:
+ static_data_shape.append(dim)
+ data = te.placeholder(static_data_shape, dtype=data.dtype)
+
+ wkl = _get_conv2d_workload(data, kernel, strides, padding, out_dtype,
+ layout)
+ _fallback_schedule(cfg, wkl)
+
+
+def _fallback_schedule(cfg, wkl):
+ simd_width = 4 # assume ARM SIMD Width is 4
+ HPAD, WPAD = wkl.hpad, wkl.wpad
Review comment:
See below comment. We should support asymmetic padding. i.e. pad_top, pad_left, pad_bottom, pad_right
##########
File path: topi/python/topi/arm_cpu/group_conv2d.py
##########
@@ -0,0 +1,310 @@
+import tvm
+from tvm import autotvm
+from tvm import te
+from ..util import get_const_tuple
+from ..nn.pad import pad
+from .. import tag
+
+from ..nn.conv2d import group_conv2d_nchw
+from ..nn.util import infer_pad
+from ..nn.conv2d import _get_workload as _get_conv2d_workload
+
+from tvm.autotvm.task.space import SplitEntity, OtherOptionEntity
+
+
+def group_conv2d_nchw(data, kernel, strides, padding, dilation, groups,
+ out_dtype):
+ """Compute group_conv2d with NCHW layout"""
+ return group_conv2d_nchw_spatial_pack(data, kernel, strides, padding,
+ dilation, groups, out_dtype)
+
+
+def schedule_group_conv2d_nchw(outs):
+ """Compute group_conv2d with NCHW layout"""
+ return schedule_group_conv2d_nchwc(outs)
+
+
+def _get_default_config(cfg, data, kernel, strides, padding, groups, out_dtype,
+ layout='NCHW'):
+ """
+ Get default schedule config for the workload
+ """
+ static_data_shape = []
+ for dim in get_const_tuple(data.shape):
+ if isinstance(dim, tvm.tir.Var):
+ static_data_shape.append(1)
+ else:
+ static_data_shape.append(dim)
+ data = te.placeholder(static_data_shape, dtype=data.dtype)
+
+ wkl = _get_conv2d_workload(data, kernel, strides, padding, out_dtype,
+ layout)
+ _fallback_schedule(cfg, wkl)
+
+
+def _fallback_schedule(cfg, wkl):
+ simd_width = 4 # assume ARM SIMD Width is 4
+ HPAD, WPAD = wkl.hpad, wkl.wpad
+ HSTR, WSTR = wkl.hstride, wkl.wstride
+ out_width = (wkl.width + 2 * WPAD - wkl.wkernel) // WSTR + 1
+ G = wkl.groups
+ KPG = wkl.out_filter // G
+ CPG = wkl.in_filter // G
+ oc_bn = 1
+
+ for bn in range(simd_width, 0, -1):
+ if KPG % bn == 0:
+ oc_bn = bn
+ break
+
+ ic_bn = 1
+ for bn in range(oc_bn, 0, -1):
+ if CPG % bn == 0:
+ ic_bn = bn
+ break
+
+ reg_n = 1
+ for n in range(31, 0, -1):
+ if out_width % n == 0:
+ reg_n = n
+ break
+
+ cfg["tile_ic"] = SplitEntity([wkl.in_filter // ic_bn, ic_bn])
+ cfg["tile_oc"] = SplitEntity([wkl.out_filter // oc_bn, oc_bn])
+ cfg["tile_ow"] = SplitEntity([out_width // reg_n, reg_n])
+ cfg["unroll_kw"] = OtherOptionEntity(False)
+
+
+@autotvm.register_topi_compute("group_conv2d_nchw.arm_cpu")
+def group_conv2d_nchw_spatial_pack(cfg, data, kernel, strides, padding,
+ dilation, groups, out_dtype='float32'):
+ assert isinstance(dilation, int) or len(dilation) == 2
+ if isinstance(dilation, int):
+ dilation_h, dilation_w = dilation, dilation
+ else:
+ dilation_h, dilation_w = dilation
+
+ assert isinstance(padding, int) or len(padding) == 2 or len(padding) == 4
+ if isinstance(padding, int):
+ HPAD, WPAD = padding, padding
+ elif len(padding) == 2:
+ HPAD, WPAD = padding
+ else:
+ HPAD, _, WPAD, _ = padding
+
+ assert isinstance(strides, int) or len(strides) == 2
+ if isinstance(strides, int):
+ HSTR, WSTR = strides, strides
+ else:
+ HSTR, WSTR = strides
+
+ N, CI, IH, IW = get_const_tuple(data.shape)
+ CO, CIG, KH, KW = get_const_tuple(kernel.shape)
+
+ pad_height = IH + 2 * HPAD
+ pad_width = IW + 2 * WPAD
+
+ dilated_kernel_h = (KH - 1) * dilation_h + 1
+ dilated_kernel_w = (KW - 1) * dilation_w + 1
+ OH = (IH + 2 * HPAD - dilated_kernel_h) // HSTR + 1
+ OW = (IW + 2 * WPAD - dilated_kernel_w) // WSTR + 1
+
+ G = groups
+ KPG = CO // G
+ CPG = CI // G
+
+ cfg.define_split("tile_ic", CI, num_outputs=2)
+ cfg.define_split("tile_oc", CO, num_outputs=2)
+ cfg.define_split("tile_ow", OW, num_outputs=2, filter=lambda y: y.size[-1] <= 64)
+ cfg.define_knob("unroll_kw", [True, False])
+
+ # If no config was set, we can fallback to default config.
+ if cfg.is_fallback:
+ _get_default_config(cfg, te.placeholder((N, CI, IH, IW), dtype=data.dtype),
+ te.placeholder((N, CI // G, KH, KW),
+ dtype=kernel.dtype),
+ strides, padding, groups, out_dtype)
+
+ oc_bn = cfg['tile_oc'].size[-1]
+ ic_bn = cfg['tile_ic'].size[-1]
+ # pack data
+ DOPAD = (HPAD != 0 or WPAD != 0)
+ if DOPAD:
+ data_pad = pad(data, (0, 0, HPAD, WPAD), name="data_pad")
+ else:
+ data_pad = data
+
+ shape = (G, N, CPG // ic_bn,
+ pad_height, ic_bn, pad_width)
+
+ data_vec = te.compute(shape,
+ lambda g, n, C, h, c, w:
+ data_pad[n, C * ic_bn + c + CPG * g, h, w],
+ name='data_vec')
+
+ # pack kernel
+ shape = (G, KPG//oc_bn, CPG//ic_bn,
+ KH, KW, ic_bn, oc_bn)
+ kernel_vec = te.compute(shape,
Review comment:
I think we could do this in alter_op_layout for kernel, then we won't need do schedule kernel_vec as it will become tensor when we do inference. Could refer spatial pack of arm conv2d.
##########
File path: topi/python/topi/arm_cpu/group_conv2d.py
##########
@@ -0,0 +1,310 @@
+import tvm
+from tvm import autotvm
+from tvm import te
+from ..util import get_const_tuple
+from ..nn.pad import pad
+from .. import tag
+
+from ..nn.conv2d import group_conv2d_nchw
+from ..nn.util import infer_pad
+from ..nn.conv2d import _get_workload as _get_conv2d_workload
+
+from tvm.autotvm.task.space import SplitEntity, OtherOptionEntity
+
+
+def group_conv2d_nchw(data, kernel, strides, padding, dilation, groups,
+ out_dtype):
+ """Compute group_conv2d with NCHW layout"""
+ return group_conv2d_nchw_spatial_pack(data, kernel, strides, padding,
+ dilation, groups, out_dtype)
+
+
+def schedule_group_conv2d_nchw(outs):
+ """Compute group_conv2d with NCHW layout"""
+ return schedule_group_conv2d_nchwc(outs)
+
+
+def _get_default_config(cfg, data, kernel, strides, padding, groups, out_dtype,
+ layout='NCHW'):
+ """
+ Get default schedule config for the workload
+ """
+ static_data_shape = []
+ for dim in get_const_tuple(data.shape):
+ if isinstance(dim, tvm.tir.Var):
+ static_data_shape.append(1)
+ else:
+ static_data_shape.append(dim)
+ data = te.placeholder(static_data_shape, dtype=data.dtype)
+
+ wkl = _get_conv2d_workload(data, kernel, strides, padding, out_dtype,
+ layout)
+ _fallback_schedule(cfg, wkl)
+
+
+def _fallback_schedule(cfg, wkl):
+ simd_width = 4 # assume ARM SIMD Width is 4
+ HPAD, WPAD = wkl.hpad, wkl.wpad
+ HSTR, WSTR = wkl.hstride, wkl.wstride
+ out_width = (wkl.width + 2 * WPAD - wkl.wkernel) // WSTR + 1
+ G = wkl.groups
+ KPG = wkl.out_filter // G
+ CPG = wkl.in_filter // G
+ oc_bn = 1
+
+ for bn in range(simd_width, 0, -1):
+ if KPG % bn == 0:
+ oc_bn = bn
+ break
+
+ ic_bn = 1
+ for bn in range(oc_bn, 0, -1):
+ if CPG % bn == 0:
+ ic_bn = bn
+ break
+
+ reg_n = 1
+ for n in range(31, 0, -1):
+ if out_width % n == 0:
+ reg_n = n
+ break
+
+ cfg["tile_ic"] = SplitEntity([wkl.in_filter // ic_bn, ic_bn])
+ cfg["tile_oc"] = SplitEntity([wkl.out_filter // oc_bn, oc_bn])
+ cfg["tile_ow"] = SplitEntity([out_width // reg_n, reg_n])
+ cfg["unroll_kw"] = OtherOptionEntity(False)
+
+
+@autotvm.register_topi_compute("group_conv2d_nchw.arm_cpu")
+def group_conv2d_nchw_spatial_pack(cfg, data, kernel, strides, padding,
+ dilation, groups, out_dtype='float32'):
+ assert isinstance(dilation, int) or len(dilation) == 2
+ if isinstance(dilation, int):
+ dilation_h, dilation_w = dilation, dilation
+ else:
+ dilation_h, dilation_w = dilation
+
+ assert isinstance(padding, int) or len(padding) == 2 or len(padding) == 4
+ if isinstance(padding, int):
+ HPAD, WPAD = padding, padding
+ elif len(padding) == 2:
+ HPAD, WPAD = padding
+ else:
+ HPAD, _, WPAD, _ = padding
Review comment:
For tensorflow asymmetic padding, I think this should be pad_top, pad_left, pad_bottom, pad_right = padding; HPAD = pad_top + pad_bottom; WPAD = pad_left + pad_right; After this, we won't be 2 * WPAD / 2 * HPAD when use WPAD / HPAD, just WPAD / HPAD directly
##########
File path: topi/python/topi/arm_cpu/group_conv2d.py
##########
@@ -0,0 +1,310 @@
+import tvm
+from tvm import autotvm
+from tvm import te
+from ..util import get_const_tuple
+from ..nn.pad import pad
+from .. import tag
+
+from ..nn.conv2d import group_conv2d_nchw
+from ..nn.util import infer_pad
+from ..nn.conv2d import _get_workload as _get_conv2d_workload
+
+from tvm.autotvm.task.space import SplitEntity, OtherOptionEntity
+
+
+def group_conv2d_nchw(data, kernel, strides, padding, dilation, groups,
+ out_dtype):
+ """Compute group_conv2d with NCHW layout"""
+ return group_conv2d_nchw_spatial_pack(data, kernel, strides, padding,
+ dilation, groups, out_dtype)
+
+
+def schedule_group_conv2d_nchw(outs):
+ """Compute group_conv2d with NCHW layout"""
+ return schedule_group_conv2d_nchwc(outs)
+
+
+def _get_default_config(cfg, data, kernel, strides, padding, groups, out_dtype,
+ layout='NCHW'):
+ """
+ Get default schedule config for the workload
+ """
+ static_data_shape = []
+ for dim in get_const_tuple(data.shape):
+ if isinstance(dim, tvm.tir.Var):
+ static_data_shape.append(1)
+ else:
+ static_data_shape.append(dim)
+ data = te.placeholder(static_data_shape, dtype=data.dtype)
+
+ wkl = _get_conv2d_workload(data, kernel, strides, padding, out_dtype,
+ layout)
+ _fallback_schedule(cfg, wkl)
+
+
+def _fallback_schedule(cfg, wkl):
+ simd_width = 4 # assume ARM SIMD Width is 4
+ HPAD, WPAD = wkl.hpad, wkl.wpad
+ HSTR, WSTR = wkl.hstride, wkl.wstride
+ out_width = (wkl.width + 2 * WPAD - wkl.wkernel) // WSTR + 1
+ G = wkl.groups
+ KPG = wkl.out_filter // G
+ CPG = wkl.in_filter // G
+ oc_bn = 1
+
+ for bn in range(simd_width, 0, -1):
+ if KPG % bn == 0:
+ oc_bn = bn
+ break
+
+ ic_bn = 1
+ for bn in range(oc_bn, 0, -1):
+ if CPG % bn == 0:
+ ic_bn = bn
+ break
+
+ reg_n = 1
+ for n in range(31, 0, -1):
+ if out_width % n == 0:
+ reg_n = n
+ break
+
+ cfg["tile_ic"] = SplitEntity([wkl.in_filter // ic_bn, ic_bn])
+ cfg["tile_oc"] = SplitEntity([wkl.out_filter // oc_bn, oc_bn])
+ cfg["tile_ow"] = SplitEntity([out_width // reg_n, reg_n])
+ cfg["unroll_kw"] = OtherOptionEntity(False)
+
+
+@autotvm.register_topi_compute("group_conv2d_nchw.arm_cpu")
+def group_conv2d_nchw_spatial_pack(cfg, data, kernel, strides, padding,
+ dilation, groups, out_dtype='float32'):
+ assert isinstance(dilation, int) or len(dilation) == 2
+ if isinstance(dilation, int):
+ dilation_h, dilation_w = dilation, dilation
+ else:
+ dilation_h, dilation_w = dilation
+
+ assert isinstance(padding, int) or len(padding) == 2 or len(padding) == 4
+ if isinstance(padding, int):
+ HPAD, WPAD = padding, padding
+ elif len(padding) == 2:
+ HPAD, WPAD = padding
+ else:
+ HPAD, _, WPAD, _ = padding
+
+ assert isinstance(strides, int) or len(strides) == 2
+ if isinstance(strides, int):
+ HSTR, WSTR = strides, strides
+ else:
+ HSTR, WSTR = strides
+
+ N, CI, IH, IW = get_const_tuple(data.shape)
+ CO, CIG, KH, KW = get_const_tuple(kernel.shape)
+
+ pad_height = IH + 2 * HPAD
+ pad_width = IW + 2 * WPAD
+
+ dilated_kernel_h = (KH - 1) * dilation_h + 1
+ dilated_kernel_w = (KW - 1) * dilation_w + 1
+ OH = (IH + 2 * HPAD - dilated_kernel_h) // HSTR + 1
+ OW = (IW + 2 * WPAD - dilated_kernel_w) // WSTR + 1
+
+ G = groups
+ KPG = CO // G
+ CPG = CI // G
+
+ cfg.define_split("tile_ic", CI, num_outputs=2)
+ cfg.define_split("tile_oc", CO, num_outputs=2)
+ cfg.define_split("tile_ow", OW, num_outputs=2, filter=lambda y: y.size[-1] <= 64)
+ cfg.define_knob("unroll_kw", [True, False])
+
+ # If no config was set, we can fallback to default config.
+ if cfg.is_fallback:
+ _get_default_config(cfg, te.placeholder((N, CI, IH, IW), dtype=data.dtype),
+ te.placeholder((N, CI // G, KH, KW),
+ dtype=kernel.dtype),
+ strides, padding, groups, out_dtype)
+
+ oc_bn = cfg['tile_oc'].size[-1]
+ ic_bn = cfg['tile_ic'].size[-1]
+ # pack data
+ DOPAD = (HPAD != 0 or WPAD != 0)
+ if DOPAD:
+ data_pad = pad(data, (0, 0, HPAD, WPAD), name="data_pad")
+ else:
+ data_pad = data
+
+ shape = (G, N, CPG // ic_bn,
+ pad_height, ic_bn, pad_width)
+
+ data_vec = te.compute(shape,
+ lambda g, n, C, h, c, w:
+ data_pad[n, C * ic_bn + c + CPG * g, h, w],
+ name='data_vec')
+
+ # pack kernel
+ shape = (G, KPG//oc_bn, CPG//ic_bn,
+ KH, KW, ic_bn, oc_bn)
+ kernel_vec = te.compute(shape,
+ lambda g, CO, CI, h, w, ci, co:
+ kernel[(CO * oc_bn + co + g * KPG),
+ CI * ic_bn + ci, h, w],
+ name='kernel_vec')
+
+ # convolution
+ oshape = (G, N, KPG//oc_bn,
+ OH, OW, oc_bn)
+ unpack_shape = (N, CO, OH, OW)
+
+ ic = te.reduce_axis((0, (CPG)), name='ic')
+ kh = te.reduce_axis((0, KH), name='kh')
+ kw = te.reduce_axis((0, KW), name='kw')
+ idxmod = tvm.tir.indexmod
+ idxdiv = tvm.tir.indexdiv
+
+ conv = te.compute(oshape, lambda g, n, oc_chunk, oh, ow, oc_block:
+ te.sum(data_vec[g, n, idxdiv(ic, ic_bn),
+ oh*HSTR+kh*dilation_h,
+ idxmod(ic, ic_bn),
+ ow*WSTR+kw*dilation_w].astype(out_dtype) *
+ kernel_vec[g, oc_chunk, idxdiv(ic, ic_bn),
+ kh, kw, idxmod(ic, ic_bn),
+ oc_block].astype(out_dtype),
+ axis=[ic, kh, kw]), name='conv')
+
+ unpack = te.compute(unpack_shape,
+ lambda n, c, h, w:
+ conv[idxdiv(c, KPG), n,
+ idxmod(idxdiv(c, oc_bn), (KPG // oc_bn)),
+ h, w,
+ idxmod(idxmod(c, oc_bn), KPG)]
+ .astype(out_dtype),
+ name='output_unpack',
+ tag='group_conv2d_nchw')
+ return unpack
+
+
+@autotvm.register_topi_schedule("group_conv2d_nchw.arm_cpu")
+def schedule_group_conv2d_nchwc(cfg, outs):
+ """Create schedule for tensors"""
+ s = te.create_schedule([x.op for x in outs])
+ scheduled_ops = []
+
+ def traverse(op):
+ """Traverse operators from computation graph"""
+ # inline all one-to-one-mapping operators except the last stage (output)
+ if tag.is_broadcast(op.tag):
+ if op not in s.outputs:
+ s[op].compute_inline()
+ for tensor in op.input_tensors:
+ if isinstance(tensor.op, tvm.te.ComputeOp) and tensor.op not in scheduled_ops:
+ traverse(tensor.op)
+
+ if 'group_conv2d_nchw' in op.tag:
+ output = op.output(0)
+
+ if "tile_ic" not in cfg:
+ return
+ conv_out = op.input_tensors[0]
+ kernel_vec = conv_out.op.input_tensors[1]
+ kernel = kernel_vec.op.input_tensors[0]
+ if isinstance(kernel.op, tvm.te.ComputeOp) and "dilate" in kernel.op.tag:
+ s[kernel].compute_inline()
+ data_vec = conv_out.op.input_tensors[0]
+ data = data_vec.op.input_tensors[0]
+ data_pad = None
+ if isinstance(data.op, tvm.te.ComputeOp) and "pad" in data.op.tag:
+ data_pad = data
+ data = data_pad.op.input_tensors[0]
+
+ _, c, h, w = get_const_tuple(data.shape)
+ _, x, kh, kw = get_const_tuple(kernel.shape)
+
+ args = [s, cfg, data, data_pad, data_vec, kernel_vec, conv_out,
+ output, outs[0]]
+ schedule_conv_sp_grouped(*args)
+
+ scheduled_ops.append(op)
+
+ traverse(outs[0].op)
+ return s
+
+
+def schedule_conv_sp_grouped(s, cfg, data, data_pad, data_vec, kernel_vec,
+ conv_out, output, last,
+ **kwargs):
+ # fetch schedule
+ ic_bn, oc_bn, reg_n, unroll_kw = (cfg["tile_ic"].size[-1], cfg["tile_oc"].size[-1],
+ cfg["tile_ow"].size[-1], cfg["unroll_kw"].val)
+
+ # no stride and padding info here
+ padding = infer_pad(data, data_pad)
+ HPAD, WPAD = padding
+ DOPAD = (HPAD != 0 or WPAD != 0)
+
+ A, W = data, kernel_vec
+ A0, A1 = data_pad, data_vec
+
+ # schedule data
+ if DOPAD:
+ s[A0].compute_inline()
Review comment:
Consider using compute_at and vectorize data load. According to experiment of https://github.com/apache/incubator-tvm/pull/6095#discussion_r458893850, we should have better performance than compute inline directly.
----------------------------------------------------------------
This is an automated message from the Apache Git Service.
To respond to the message, please log on to GitHub and use the
URL above to go to the specific comment.
For queries about this service, please contact Infrastructure at:
users@infra.apache.org
[GitHub] [incubator-tvm] Wheest commented on pull request #6137: Better grouped convolution for CPU targets
Posted by GitBox <gi...@apache.org>.
Wheest commented on pull request #6137:
URL: https://github.com/apache/incubator-tvm/pull/6137#issuecomment-693632139
@ZihengJiang Have updated linting errors.
Have added asymmetric padding support, however this isn't consistent across the rest of TOPI. Have added a new workload type in `python/tvm/topi/nn/conv2d.py`, that can be adopted across other Conv2D implementations.
Have been looking at suggested scheduled improvements, however have not been able to get any improvements to date.
Have not yet figured out how to do the kernel packing step in `alter_op_layout`, but I think I can do when I have some time next week.
----------------------------------------------------------------
This is an automated message from the Apache Git Service.
To respond to the message, please log on to GitHub and use the
URL above to go to the specific comment.
For queries about this service, please contact Infrastructure at:
users@infra.apache.org
[GitHub] [incubator-tvm] tqchen commented on pull request #6137: Better grouped convolution for CPU targets
Posted by GitBox <gi...@apache.org>.
tqchen commented on pull request #6137:
URL: https://github.com/apache/incubator-tvm/pull/6137#issuecomment-667182671
@Wheest please help to fix the CI lint error
----------------------------------------------------------------
This is an automated message from the Apache Git Service.
To respond to the message, please log on to GitHub and use the
URL above to go to the specific comment.
For queries about this service, please contact Infrastructure at:
users@infra.apache.org
[GitHub] [incubator-tvm] ZihengJiang commented on pull request #6137: Better grouped convolution for CPU targets
Posted by GitBox <gi...@apache.org>.
ZihengJiang commented on pull request #6137:
URL: https://github.com/apache/incubator-tvm/pull/6137#issuecomment-693639876
Sounds good! Just ping the reviewers when you feel it is ready to be reviewed again. Thanks for the works!
----------------------------------------------------------------
This is an automated message from the Apache Git Service.
To respond to the message, please log on to GitHub and use the
URL above to go to the specific comment.
For queries about this service, please contact Infrastructure at:
users@infra.apache.org
[GitHub] [incubator-tvm] tqchen commented on pull request #6137: Better grouped convolution for CPU targets
Posted by GitBox <gi...@apache.org>.
tqchen commented on pull request #6137:
URL: https://github.com/apache/incubator-tvm/pull/6137#issuecomment-667181967
cc @anijain2305 @FrozenGene @mbaret @giuseros it would be great if you can help to review the PR
----------------------------------------------------------------
This is an automated message from the Apache Git Service.
To respond to the message, please log on to GitHub and use the
URL above to go to the specific comment.
For queries about this service, please contact Infrastructure at:
users@infra.apache.org
[GitHub] [incubator-tvm] ZihengJiang commented on pull request #6137: Better grouped convolution for CPU targets
Posted by GitBox <gi...@apache.org>.
ZihengJiang commented on pull request #6137:
URL: https://github.com/apache/incubator-tvm/pull/6137#issuecomment-693627025
Ping for update @FrozenGene @Wheest
----------------------------------------------------------------
This is an automated message from the Apache Git Service.
To respond to the message, please log on to GitHub and use the
URL above to go to the specific comment.
For queries about this service, please contact Infrastructure at:
users@infra.apache.org
[GitHub] [incubator-tvm] Wheest commented on a change in pull request #6137: Better grouped convolution for CPU targets
Posted by GitBox <gi...@apache.org>.
Wheest commented on a change in pull request #6137:
URL: https://github.com/apache/incubator-tvm/pull/6137#discussion_r475536419
##########
File path: topi/python/topi/arm_cpu/group_conv2d.py
##########
@@ -0,0 +1,310 @@
+import tvm
+from tvm import autotvm
+from tvm import te
+from ..util import get_const_tuple
+from ..nn.pad import pad
+from .. import tag
+
+from ..nn.conv2d import group_conv2d_nchw
+from ..nn.util import infer_pad
+from ..nn.conv2d import _get_workload as _get_conv2d_workload
+
+from tvm.autotvm.task.space import SplitEntity, OtherOptionEntity
+
+
+def group_conv2d_nchw(data, kernel, strides, padding, dilation, groups,
+ out_dtype):
+ """Compute group_conv2d with NCHW layout"""
+ return group_conv2d_nchw_spatial_pack(data, kernel, strides, padding,
+ dilation, groups, out_dtype)
+
+
+def schedule_group_conv2d_nchw(outs):
+ """Compute group_conv2d with NCHW layout"""
+ return schedule_group_conv2d_nchwc(outs)
+
+
+def _get_default_config(cfg, data, kernel, strides, padding, groups, out_dtype,
+ layout='NCHW'):
+ """
+ Get default schedule config for the workload
+ """
+ static_data_shape = []
+ for dim in get_const_tuple(data.shape):
+ if isinstance(dim, tvm.tir.Var):
+ static_data_shape.append(1)
+ else:
+ static_data_shape.append(dim)
+ data = te.placeholder(static_data_shape, dtype=data.dtype)
+
+ wkl = _get_conv2d_workload(data, kernel, strides, padding, out_dtype,
+ layout)
+ _fallback_schedule(cfg, wkl)
+
+
+def _fallback_schedule(cfg, wkl):
+ simd_width = 4 # assume ARM SIMD Width is 4
+ HPAD, WPAD = wkl.hpad, wkl.wpad
+ HSTR, WSTR = wkl.hstride, wkl.wstride
+ out_width = (wkl.width + 2 * WPAD - wkl.wkernel) // WSTR + 1
+ G = wkl.groups
+ KPG = wkl.out_filter // G
+ CPG = wkl.in_filter // G
+ oc_bn = 1
+
+ for bn in range(simd_width, 0, -1):
+ if KPG % bn == 0:
+ oc_bn = bn
+ break
+
+ ic_bn = 1
+ for bn in range(oc_bn, 0, -1):
+ if CPG % bn == 0:
+ ic_bn = bn
+ break
+
+ reg_n = 1
+ for n in range(31, 0, -1):
+ if out_width % n == 0:
+ reg_n = n
+ break
+
+ cfg["tile_ic"] = SplitEntity([wkl.in_filter // ic_bn, ic_bn])
+ cfg["tile_oc"] = SplitEntity([wkl.out_filter // oc_bn, oc_bn])
+ cfg["tile_ow"] = SplitEntity([out_width // reg_n, reg_n])
+ cfg["unroll_kw"] = OtherOptionEntity(False)
+
+
+@autotvm.register_topi_compute("group_conv2d_nchw.arm_cpu")
+def group_conv2d_nchw_spatial_pack(cfg, data, kernel, strides, padding,
+ dilation, groups, out_dtype='float32'):
+ assert isinstance(dilation, int) or len(dilation) == 2
+ if isinstance(dilation, int):
+ dilation_h, dilation_w = dilation, dilation
+ else:
+ dilation_h, dilation_w = dilation
+
+ assert isinstance(padding, int) or len(padding) == 2 or len(padding) == 4
+ if isinstance(padding, int):
+ HPAD, WPAD = padding, padding
+ elif len(padding) == 2:
+ HPAD, WPAD = padding
+ else:
+ HPAD, _, WPAD, _ = padding
Review comment:
Have added this to my working version. However, the support function [_get_workload()](https://github.com/apache/incubator-tvm/blob/master/python/tvm/topi/nn/conv2d.py#L141) throws this information away.
----------------------------------------------------------------
This is an automated message from the Apache Git Service.
To respond to the message, please log on to GitHub and use the
URL above to go to the specific comment.
For queries about this service, please contact Infrastructure at:
users@infra.apache.org
[GitHub] [incubator-tvm] FrozenGene commented on a change in pull request #6137: Better grouped convolution for CPU targets
Posted by GitBox <gi...@apache.org>.
FrozenGene commented on a change in pull request #6137:
URL: https://github.com/apache/incubator-tvm/pull/6137#discussion_r463837025
##########
File path: topi/python/topi/arm_cpu/group_conv2d.py
##########
@@ -0,0 +1,310 @@
+import tvm
+from tvm import autotvm
+from tvm import te
+from ..util import get_const_tuple
+from ..nn.pad import pad
+from .. import tag
+
+from ..nn.conv2d import group_conv2d_nchw
+from ..nn.util import infer_pad
+from ..nn.conv2d import _get_workload as _get_conv2d_workload
+
+from tvm.autotvm.task.space import SplitEntity, OtherOptionEntity
+
+
+def group_conv2d_nchw(data, kernel, strides, padding, dilation, groups,
+ out_dtype):
+ """Compute group_conv2d with NCHW layout"""
+ return group_conv2d_nchw_spatial_pack(data, kernel, strides, padding,
+ dilation, groups, out_dtype)
+
+
+def schedule_group_conv2d_nchw(outs):
+ """Compute group_conv2d with NCHW layout"""
+ return schedule_group_conv2d_nchwc(outs)
+
+
+def _get_default_config(cfg, data, kernel, strides, padding, groups, out_dtype,
+ layout='NCHW'):
+ """
+ Get default schedule config for the workload
+ """
+ static_data_shape = []
+ for dim in get_const_tuple(data.shape):
+ if isinstance(dim, tvm.tir.Var):
+ static_data_shape.append(1)
+ else:
+ static_data_shape.append(dim)
+ data = te.placeholder(static_data_shape, dtype=data.dtype)
+
+ wkl = _get_conv2d_workload(data, kernel, strides, padding, out_dtype,
+ layout)
+ _fallback_schedule(cfg, wkl)
+
+
+def _fallback_schedule(cfg, wkl):
+ simd_width = 4 # assume ARM SIMD Width is 4
+ HPAD, WPAD = wkl.hpad, wkl.wpad
Review comment:
See above comment. We should support asymmetic padding. i.e. pad_top, pad_left, pad_bottom, pad_right
----------------------------------------------------------------
This is an automated message from the Apache Git Service.
To respond to the message, please log on to GitHub and use the
URL above to go to the specific comment.
For queries about this service, please contact Infrastructure at:
users@infra.apache.org